Probabilistic ratiocination of hepatocellular carcinoma after resection: evaluation of expected to be promising approaches
Introduction
Individualized calculation of mortality risk has gained attention in the precision medicine era due to its supportive guidance for treatment selection and the estimation of survival outcomes (1,2). Among the predictive models for cancer, the Cox proportional hazards regression model has been widely applied for both the identification of significant prognostic factors and the prediction of patient survival outcomes. The results of the Cox proportional hazards regression model are frequently visualized as nomograms for clinical application (3,4). In recent years, the deep learning (DL) approach, which allows computational models composed of multiple processing layers to learn data representations with multilevel abstraction, has been applied in some medical fields, including drug discovery, image evaluation and diagnosis, and genomics (5,6).
Hepatocellular carcinoma (HCC) is the most common primary hepatic tumor, accounting for the majority of primary liver cancers. The global incidence and mortality of HCC are rapidly increasing (7). Most HCCs arise from viral hepatitis, non-alcoholic fatty liver disease, and liver cirrhosis. Thus close surveillance of patients with these conditions would contribute to the early detection of HCC, which in turn could expand the proportion of eligible candidates for surgical resection (8,9). Recently, it has been reported that T1 stage HCC accounts for more than 40% of the total cases (10). Although surgical resection and orthotopic liver transplantation are the standard of care and provide an opportunity for curative treatment of tumors without extrahepatic metastasis, owing to a shortage of organ donors, surgical resection is recommended for resectable cases (11).
Along with advances in the identification of risk factors for the development of HCC and surveillance systems, the effectiveness of surgical resection and the identification of appropriate candidates have become crucial to improving the prognosis of patients with HCC. In the present study, we investigated the derivation of predictive systems composed of clinical and genetic factors using the Cox regression model and DL approaches, with the aim of evaluating the optimal covariates and methodology for patients with HCC undergoing surgical resection. We present the following article in accordance with the TRIPOD reporting checklist (available at http://dx.doi.org/10.21037/atm-20-4828).
Methods
Patients
This was a retrospective, two-center study. The clinical models were derived from patients with HCC who underwent surgical resection at the Eastern Hepatobiliary Surgery Hospital (EHBH), Second Military Medical University (Shanghai, China) between January 2005 and December 2011. The models were validated in patients with HCC who underwent resection at Renji Hospital, School of Medicine, Shanghai Jiao Tong University (Shanghai, China) between January 2004 and December 2012. The enrolled patients had a diagnosis of HCC based on histopathological examination. To be eligible, patients also needed to have an Eastern Cooperative Oncology Group (ECOG) performance status score of 0 or 1, and to have undergone only surgical resection as the initial treatment. Patients who died perioperatively or who had incomplete follow-up or clinical data were excluded from the analysis. Of 2,163 and 601 patients treated at the Eastern Hepatobiliary Surgery Hospital and Renji Hospital, respectively, 1,114 patients and 184 patients were enrolled into the present study (Figure 1). The study was conducted in accordance with the Declaration of Helsinki (as revised in 2013). The study was approved by Eastern Hepatobiliary Surgery Hospital ethics committee (No. 2020024) and individual consent for this retrospective analysis was waived.
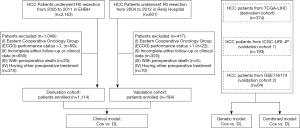
Data sources
For the construction of the genetic and combined clinical and genetic models, gene expression data from 377 patients with HCC were retrieved from The Cancer Genome Atlas (TCGA; https://www.cancer.gov/tcga) Research Network. After screening the available data, 374 patients were enrolled into the analyses for the development of the genetic and combined clinical and genetic models. For validation, we retrieved clinical and RNA sequencing (RNA-seq) data of patients with HCC from the International Cancer Genome Consortium (ICGC; ICGC-LIRI-JP, n=193; validation group 1) and Gene Expression Omnibus (GEO; GSE116174, n=64; validation group 2), respectively (Figure 1).
Model construction and variables
Two methodologies, Cox regression and DL, were applied in the derivation of the models. For Cox models, all variables were tested for statistical significance through univariate analyses, and multivariate analysis was carried out for factors with statistical significance. Only the significant factors identified in the multivariate analysis were selected for the Cox clinical model. For the development of the genetic nomogram, only covariates found to have a significant prognostic impact in the Cox univariate analysis as well as |log2 (fold change)|>0.6 and P<0.05 were considered eligible for inclusion. Multivariate analysis was not carried out for gene expression variables due to there being a large number of variables that limited the evaluation of independent prognostic impact.
For the development of the DL models, 22 demographic and clinical variables were adopted, including sex, age, alpha fetoprotein (AFP), carcinoembryonic antigen (CEA), hepatitis B virus (HBV) and hepatitis C virus (HCV) infection, total bilirubin (TB), albumin, prothrombin time (PT), alanine aminotransferase (ALT), aspartate aminotransferase (AST), liver cirrhosis, tumor size, tumor number, lymph node metastasis, vascular invasion, capsule formation, TNM stage, tumor location, and diabetes mellitus. Among the variables, tumor size and number, vascular invasion, lymph node metastasis, capsule formation, tumor location, and TNM stage were collected from postoperative pathology reports. For the DL genetic model, RNA-seq identified 686 expressive genes that were approved in HUGO Gene Nomenclature Committee (HGNC; https://www.genenames.org) and were subjected to analysis. The full list of the included genes is shown in the supplementary file (https://cdn.amegroups.cn/static/application/3f120d22dea23635dbe86366eed76a7f/atm-20-4828-1.pdf).
In the construction and validation of the combined clinical and genetic models, 7 variables (age, sex, HBV infection, TNM stage, vascular invasion, alcohol consumption, and smoking) were overlapping; thus, these variables were included in the analyses for the Cox combined model and in the development of the DL combined model along with 686 genes. The other non-overlapping variables among the databases were excluded from the analyses.
Statistical analysis
All models were developed for the prediction of overall survival (OS) in HCC patients, which was defined as time from surgery to death. Continuous variables were not categorized in the development of any of the models and were presented as median [interquartile range (IQR)]. There were no missing values; any patient with missing data was excluded from the analyses. Kaplan-Meier estimation was performed using the log-rank test for the evaluation of cumulative events. Internal validation was defined and performed through random sampling of 100 patients for 4 times per model. The performances of the models were assessed by receiver operating characteristic (ROC) curve analysis with area under the ROC curve (AUC) and calibration plots. Between-model comparisons were carried out by calculating the net reclassification index (NRI) and integrated discrimination improvement (IDI). The models were developed with 2 major aims: discrimination and individualized provision of probability. Discrimination was carried out by halving according to the risk probability. P values <0.5 were considered to be statistically significant. All statistical analyses were performed using the R Project for Statistical Computing (v3.5.3; https://www.r-project.org). The DL models were derived using TensorFlow (v1.2.1), on servers equipped with the dual-core Intel (R) Core (TM) i7-4650U CPU @1.70 Ghz 2.30 GHz, 8 GB RAM, and Intel (R) HD Graphics 5000 using Python (v3.7.3; https://www.python.org).
Results
Patient characteristics
All derivation and validation patients were Chinese patients with a median age of 53 (IQR, 45–59) and 51 (IQR, 45–59) years, respectively (Table S1). Of the patients, 15% were female, and 10% had diabetes mellitus. Both HBV infection (88.2% in the derivation cohort; 90.2% in the validation cohort) and liver cirrhosis (69.4% in the derivation cohort; 86.4% in the validation cohort) were prevalent, supporting the theory of three-step development of HCC from HBV infection to liver cirrhosis to HCC. However, the prevalence of HCV infection was 0.9% and 2.2% in the derivation cohort and validation cohort, respectively.
In terms of patient characteristics, the cohort (TCGA-LIHC, n=374) used for construction of the genetic models was 32.4% female and had a median age of 61 years (IQR, 52–69 years). Of the patients in this cohort, 42.5% had HBV infection, and there was a predominance of TNM stages I–II (74.3%; Table S2). Validation cohort 1 (ICGC-LIRI-JP, n=193) had a relatively high age (median, 69 years; IQR, 62–74 years) and the majority of patients were male (74.6%). In this cohort, 72.0% of patients did not have HBV infection, and there was a high rate of alcohol consumption (59.6%), smoking (59.1%), and vascular invasion (31.6%). Validation cohort 2 (GSE116174, n=64) had a median age of 54 years (IQR, 49–62 years), and 9.4% of patients were female. This cohort showed a high prevalence of HBV infection (73.4%), but a low rate of alcohol consumption (20.3%). Collectively, the characteristics of the training cohort and the 2 validation cohorts were different with the aim to challenge generalization.
Cox clinical model
For the development of the Cox clinical model, univariate and multivariate analyses were carried out for the derivation set (EHBH, n=1,114). Univariate analyses identified ALT, AST, TB, PT, albumin, AFP, tumor size, tumor number, tumor location (left lobe), vascular invasion, lymph node metastasis, and TNM stage to be significant prognostic factors for OS (Table S3). Among these significant prognostic factors, TB [hazard ratio (HR), 1.01; 95% CI, 1.00–1.01; P=0.001], PT (HR, 1.14; 95% CI, 1.04–1.24; P=0.003), tumor size (HR, 1.16; 95% CI, 1.12–1.19; P<0.001), tumor number (HR, 1.48; 95% CI, 1.18–1.86; P=0.001), vascular invasion (HR, 2.22; 95% CI, 1.65–2.99; P<0.001), and lymph node metastasis (HR, 1.99; 95% CI, 1.22–3.24; P=0.006). The derived nomogram for probabilistic ratiocination and the discrimination of risk groups (Figure 2A). The consistency between predicted probability and actual proportion of survival is proved by calibration plot in training (Figure 2B). Sensitivity and specificity of training performance are evaluated by receiver operating curve (AUC: 0.75; Figure 2C).
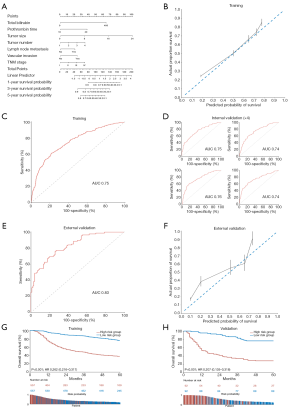
When internal validation by random sampling was carried out, the model’s performance remained significantly predictive (AUC: 0.74–0.76; Figure 2D). In the external validation cohort (patients from Renji Hospital, n=184), both ROC analysis (AUC: 0.83; Figure 2E) and the calibration plot revealed an excellent predictive performance of the model (Figure 2F). Kaplan-Meier estimation of high- and low-risk groups stratified according to the median risk revealed the HR to be 0.262 (95% CI, 0.216–0.317; P<0.001) in the training set (Figure 2G). In the validation set, the HR was 0.207 (95% CI, 0.135–0.318; P<0.001; Figure 2H). The between-group OS differed by 37% and 46% at 1 year and 5 years, respectively.
DL clinical model
The DL clinical model was developed by adopting a DL neural network, composed of 1 input, 4 hidden, and 1 output layers, to 22 clinical factors listed in the Methods section (Figure 3A). The derivative performance was comparable to that of the Cox clinical model in terms of the calibration plot (Figure 3B) and AUC (0.77; Figure 3C).
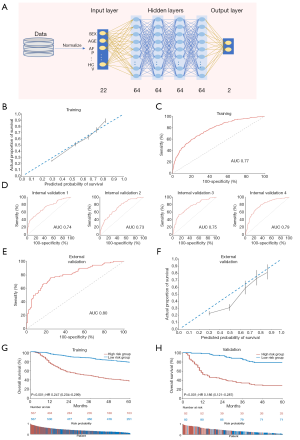
Internal validation by random sampling 4 times (n=100) revealed an AUC of 0.73–0.79 (Figure 3D). In the external validation set, an AUC of 0.80 (Figure 3E) and the calibration plot (Figure 3F) indicated an excellent performance. Evaluation of the cumulative events among probability-bisected risk groups demonstrated the model to have significant discriminatory power in both the training (HR, 0.247; 95% CI, 0.204–0.299; P<0.001; Figure 3G) and validation (HR, 0.186; 95% CI, 0.121–0.287; P<0.001; Figure 3H) sets. The differences in the probability of 1-year and 5-year OS were 41% and 50% between the high- and low-risk groups in the validation set; which was 4% larger compared to the Cox clinical model.
Cox genetic model
To develop a Cox-based genetic nomogram, RNA-seq-based 686 genes were evaluated using Cox univariate analysis. The inclusion criteria for the stratification of covariate genes were set as |log2 (fold change)|>0.6 and P<0.05. Of the 686 genes, the following 6 significantly prognostic genes met the inclusion criteria: NLRP5 [HR, 1.41; 95% CI, 1.24–1.59; P<0.001; log2 (fold change)=0.81], MAGEB6 [HR, 1.17; 95% CI, 1.07–1.28; P=0.001; log2 (fold change)=0.81], SGCZ [HR, 1.16; 95% CI, 1.06–1.26; P=0.001; log2 (fold change) =0.78], STARD6 [HR, 1.32; 95% CI, 1.18–1.47; P<0.001; log2 (fold change) =0.70], ZNF560 [HR, 1.09; 95% CI, 1.01–1.17; P=0.026; log2 (fold change) =0.65], and AKNAD1 [HR, 1.44; 95% CI, 1.23–1.68; P<0.001; log2 (fold change) =0.61]. The selected genes were enrolled in the development of the Cox genetic nomogram (Figure 4A). However, the derived model generally predicted a higher probability of survival compared to the actual proportion of survival (Figure 4B). The model also had acceptable sensitivity and specificity, with an AUC of 0.65 (Figure 4C).
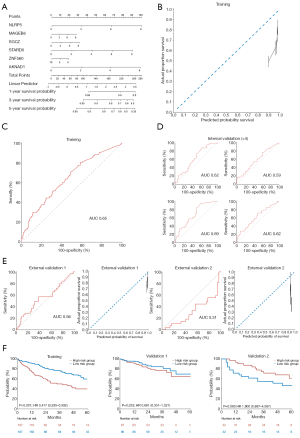
Internal validation showed that the AUC values of the model ranged between 0.59 and 0.69 (Figure 4D). In accordance with the derivation set, the predicted probability of survival was higher in both the validation 1 and validation 2 datasets, and the AUC was found to be 0.56 and 0.31, respectively (Figure 4E). Furthermore, Kaplan-Meier analysis of the 2 validation cohorts indicated that the model did not have a significantly effective performance (Figure 4F).
DL genetic model
After the failure of the Cox regression model and fold change to achieve statistical significance, we generated a DL genetic model based on all 686 genes with 7 layers, including 1 input, 5 hidden, and 1 output layer (Figure 5A). The use of numerous gene covariates resulted in a significantly improved derivation, as confirmed by ROC analysis (AUC: 0.95; Figure 5B) and the calibration plot (Figure 5C).
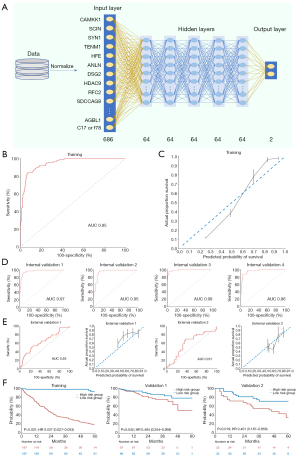
Random sampling showed the DL genetic model to have great effectiveness (AUC: 0.95–0.99; Figure 5D). In both external validation cohort 1 (AUC: 0.65) and cohort 2 (AUC: 0.61), the model’s performance was excellent compared to that of the Cox genetic model (Figure 5E). Discrimination of the training set was significant (HR, 0.037; 95% CI, 0.027–0.053; P<0.001; Figure 5F). The DL genetic model could also significantly stratify patients into high- and low-risk groups in the 2 external validation cohorts.
Cox combined model
Considering recent reports that simultaneous evaluation of clinical and genetic factors may be promising for achieving precise prediction of survival, a combined clinical and genetic model was developed using the Cox model-stratified genes and significant clinical independent prognostic factors (Figure 6A). To identify independent clinical prognostic factors, univariate and multivariate analyses were carried out for 7 variables (selected based on overlapping variables between the TCGA-LIHC, ICGC-LIRI-JP, and GSE116174 datasets), including age, sex, HBV infection, alcohol consumption, smoking, and TNM stage in the TCGA-LIHC dataset (n=374). TNM stage (HR, 1.52; 95% CI, 1.19–1.94; P=0.001) was found to be an independent prognostic factor (Table S4). Therefore, the Cox combined model was generated with 6 pre-identified genes, including NLRP5, MAGEB6, SGCZ, STARD6, and ZNF560, and TNM stage (Figure 6A). Despite the addition of clinical factors, the predicted probability of survival remained higher than the actual proportion of survival (Figure 6B). In addition, the ROC analysis revealed an AUC of 0.67 (Figure 6C).
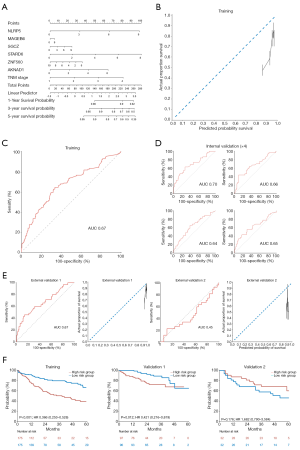
In the internal validation set, the AUC ranged from 0.63 to 0.70 (Figure 6D). However, the performance of the model in one of the validation cohorts was poor, as shown by calibration plots and ROC (AUC: 0.45; Figure 6E). The model showed significant power to discriminate between risk groups in validation group 1 (HR, 0.421; 95% CI, 0.216–0.819; P=0.012); however, its performance in validation group 2 was not significant (HR, 1.682; 95% CI, 0.790–3.584; P=0.176; Figure 6F).
DL combined model
The DL-based combined clinical and genetic model was developed using 7 overlapping clinical variables and 686 genes (Figure 7A). ROC analysis (Figure 7B) and the calibration plot (Figure 7C) showed the model to have excellent precision and discrimination.
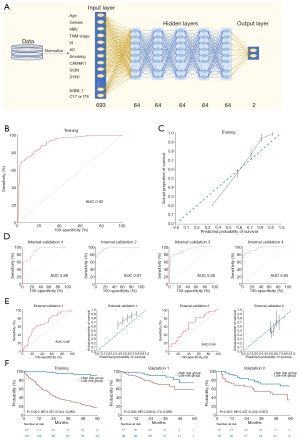
In the internal validation set, the AUCs ranged from 0.89 to 0.97 (Figure 7D). Unexpectedly, in the external validation set, the model’s performance was shown to be effective (AUC: 0.68 and 0.64; Figure 7E). When the survival curves were drawn and evaluated using the log-rank test, the patients could be significantly stratified into high- and low-risk groups in both validation group 1 (HR, 0.338; 95% CI, 0.174–0.658; P=0.002) and validation group 2 (HR, 0.437; 95% CI, 0.204–0.937; P=0.031; Figure 7F).
Between-model comparison
For between-model comparison, the IDI and NRI were evaluated for each model and compared between the DL and Cox approaches (Table 1). The DL approach comprehensively improved model performance compared to the Cox approach, except in validation group 2, which could be due to limited sample size. However, DL still improved risk reclassification in validation group 2 by 61%. The IDI for DL vs. Cox was 0.35 to 0.41 in the derivation set. In the validation set, the most significant improvement in both IDI and NRI was found for the clinical factor-based models. Improvements in discrimination and risk reclassification were increased for the combined models compared to the genetic models. Collectively, the DL approach had better IDI and NRI than the Cox approach for both model training and performance.
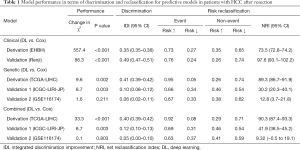
Full table
Discussion
Clinical, genetic, and combined clinical and genetic models were developed using Cox regression and DL. Model validation demonstrated significant differences in predictive performance depending on the selection of covariates and methodology. The Cox model, which consisted of TB, PT, tumor size and number, lymph node metastasis, vascular invasion, and TNM stage, and the DL clinical model, which consisted of 22 clinical factors, effectively achieved precise survival prediction in patients with HCC after resection.
In recent years, a number of gene signatures have been developed and reported to be predictive of prognosis in various cancers, suggesting their potential application value in clinical practice (12-15). In contrast to previous literature, the adoption of Cox regression and expression fold change in stratified significant prognostic genes had no significant impact on survival prediction in patients with HCC after resection. Instead, the enrollment of 686 genes was highly effective in the training of the DL model, which was also validated to be significantly predictive in two different cohorts. From this point of view, previous models for which an excellent performance has been confirmed in one validation dataset may require further validation before general application. Furthermore, the accuracy of the DL genetic model in survival prediction increased when it was trained with additional clinical factors, suggesting that simultaneous evaluation of clinical and genetic factors may be promising for the precise prediction of survival. Therefore, comprehensive enrollment of clinical and genetic covariates using the DL approach may be promising for the implementation of precise survival prediction.
Generalization of predictive models to real-world practice is challenging due to the diverse factors that are not incorporated into the prediction models, such as proficiency of the surgeon, general medical level, and lifestyle and socio-environmental factors. These factors may contribute to the disparity in the identification of prognostic factors. Indeed, independent prognostic factors vary significantly in identical disease and treatment settings at different hospitals. For example, numerous studies have reported that tumor size, which is commonly involved in staging systems for HCC, is not an independent prognostic factor for HCC after resection (16,17). Therefore, considering disparities in prognostic factors influenced by external factors, the performance of a model is likely to be most effective in the center from which the model was derived. In the present study, the Cox clinical and DL clinical models were developed and validated in patients from the same region, while the genetic models were developed and validated in different cohorts from different regions. The generalizability of the clinical factor-derived models has not been evaluated. Future studies are needed to confirm the applicability of the Cox clinical and DL clinical models in order to compare their generalizability.
Prediction models can provide guidance in many ways, including for the identification of patients who require preventative interventions, early detection of disease, treatment effectiveness, stratification of patients at risk of recurrence or death, and the estimation of risk probabilities (18-22). The derived models are capable of time-dependent risk probability estimation for the prediction of survival and resection effectiveness in patients with HCC after resection. In this way, individuals who are at high risk of short-term or long-term mortality can be identified, and more intensive follow-up, preventative treatment, and more advanced examination at intervals can be considered.
This study has some underlying limitations that should be addressed. The training and validation datasets for the clinical and genetic models were different; thus, comparison of covariate selection among clinical factors and gene expression requires further confirmation. Future prospective studies are needed to evaluate the predictive effectiveness of gene expression and clinical factors in the same study cohort. Also, the cost-effectiveness of RNA-seq for the provision of gene expression data is necessary for clinical practice, but it was not evaluated in this study. The web-based tool for the DL model was not developed due to insufficient precision and prediction, which limits external access. However, despite these limitations, this study is the first to evaluate DL approaches and compare them with conventional methodologies (Cox regression), along with examining the clinical and genetic factors.
Conclusions
In conclusion, in recent years, with the continuous development of genome sequencing, genetic markers have been proven to be effective in predicting the prognosis of a variety of tumors. In clinical practice, the COX model is very mature and accurate in identifying clinical variables that are predicative of prognosis. However, the Cox model is suboptimal for identifying genetic variables for predicting prognosis. By contrast, the DL approach seems to be promising in achieving general application of the prediction model. In addition, the performance of the DL genetic model for survival prediction was enhanced when additionally trained with clinical factors, highlighting the notion that precise survival prediction may be achieved with simultaneous evaluation of clinical and genetic factors. Thus, a comprehensive approach that enrolls both clinical and genetic covariates using the DL technique may be promising in implementing precision survival prediction. For sure, given the cost of obtaining genetic variables, it is of great significance to choose a reasonable prediction model.
Acknowledgments
Funding: This work was supported by the National Natural Science Foundation of China (81970453, 81772529), National Natural Science Foundation of China Youth Program (82000483, 81802983), Science and Technology Innovation Action Plan & Academic/Technical Leader Project of Shanghai (20XD1405100), National Science and Technology Cooperation Program of the Ministry of Science and Technology (2011DFA32980), and the National Key Basic Research Program of China 973 Program (2012CB526706).
Footnote
Reporting Checklist: The authors have completed the TRIPOD reporting checklist. Available at http://dx.doi.org/10.21037/atm-20-4828
Data Sharing Statement: Available at http://dx.doi.org/10.21037/atm-20-4828
Conflicts of Interest: All authors have completed the ICMJE uniform disclosure form (available at http://dx.doi.org/10.21037/atm-20-4828). The authors have no conflicts of interest to declare.
Ethical Statement: The authors are accountable for all aspects of the work in ensuring that questions related to the accuracy or integrity of any part of the work are appropriately investigated and resolved. The study was conducted in accordance with the Declaration of Helsinki (as revised in 2013). The study was approved by Eastern Hepatobiliary Surgery Hospital ethics committee (No. 2020024) and individual consent for this retrospective analysis was waived.
Open Access Statement: This is an Open Access article distributed in accordance with the Creative Commons Attribution-NonCommercial-NoDerivs 4.0 International License (CC BY-NC-ND 4.0), which permits the non-commercial replication and distribution of the article with the strict proviso that no changes or edits are made and the original work is properly cited (including links to both the formal publication through the relevant DOI and the license). See: https://creativecommons.org/licenses/by-nc-nd/4.0/.
References
- Kinnier CW, Asare EA, Mohanty S, et al. Risk prediction tools in surgical oncology. J Surg Oncol 2014;110:500-8. [Crossref] [PubMed]
- Grant SW, Collins GS, Nashef SAM. Statistical Primer: developing and validating a risk prediction model. Eur J Cardiothorac Surg 2018;54:203-8. [Crossref] [PubMed]
- Huang YQ, Liang CH, He L, et al. Development and Validation of a Radiomics Nomogram for Preoperative Prediction of Lymph Node Metastasis in Colorectal Cancer. J Clin Oncol 2016;34:2157-64. [Crossref] [PubMed]
- Wei S, Zang J, Jia Y, et al. A Gene-Related Nomogram for Preoperative Prediction of Lymph Node Metastasis in Colorectal Cancer. J Invest Surg 2020;33:715-22. [Crossref] [PubMed]
- LeCun Y, Bengio Y, Hinton GJN. Deep learning. Nature 2015;521:436-44. [Crossref] [PubMed]
- Hamet P, Tremblay J. Artificial intelligence in medicine. Metabolism 2017;69S:S36-40. [Crossref] [PubMed]
- Siegel RL, Miller KD, Jemal A. Cancer statistics, 2019. CA Cancer J Clin 2019;69:7-34. [Crossref] [PubMed]
- Forner A, Reig M, Bruix JJL. Hepatocellular carcinoma. Lancet 2018;391:1301-14. [Crossref] [PubMed]
- Tang A, Hallouch O, Chernyak V, et al. Epidemiology of hepatocellular carcinoma: target population for surveillance and diagnosis. Abdom Radiol (NY) 2018;43:13-25. [Crossref] [PubMed]
- Beal EW, Mehta R, Merath K, et al. Outcomes After Resection of Hepatocellular Carcinoma: Intersection of Travel Distance and Hospital Volume. J Gastrointest Surg 2019;23:1425-34. [Crossref] [PubMed]
- Bodzin AS, Baker TB. Liver Transplantation Today: Where We Are Now and Where We Are Going. Liver Transpl 2018;24:1470-5. [Crossref] [PubMed]
- Liu GM, Zeng HD, Zhang CY, et al. Identification of a six-gene signature predicting overall survival for hepatocellular carcinoma. Cancer Cell Int 2019;19:138. [Crossref] [PubMed]
- Chen K, He Y, Liu Y, et al. Gene signature associated with neuro-endocrine activity predicting prognosis of pancreatic carcinoma. Mol Genet Genomic Med 2019;7:e00729 [Crossref] [PubMed]
- Yang H, Zhou L, Chen J, et al. A four-gene signature for prognosis in breast cancer patients with hypermethylated IL15RA. Oncol Lett 2019;17:4245-54. [Crossref] [PubMed]
- Gao Z, Zhang D, Duan Y, et al. A five-gene signature predicts overall survival of patients with papillary renal cell carcinoma. PLoS One 2019;14:e0211491 [Crossref] [PubMed]
- Rungsakulkij N, Suragul W, Mingphruedhi S, et al. Prognostic factors in patients with HBV-related hepatocellular carcinoma following hepatic resection. Infect Agent Cancer 2018;13:20. [Crossref] [PubMed]
- You DD, Kim DG, Seo CH, et al. Prognostic factors after curative resection hepatocellular carcinoma and the surgeon's role. Ann Surg Treat Res 2017;93:252-9. [Crossref] [PubMed]
- Härmälä S, O'Brien A, Parisinos C, et al. Development and validation of a prediction model to estimate the risk of liver cirrhosis in primary care patients with abnormal liver blood test results: protocol for an electronic health record study in Clinical Practice Research Datalink. Diagn Progn Res 2019;3:10. [Crossref] [PubMed]
- Jia X, Baig M, Mirza F, et al. A Cox-Based Risk Prediction Model for Early Detection of Cardiovascular Disease: Identification of Key Risk Factors for the Development of a 10-Year CVD Risk Prediction. Adv Prev Med 2019;2019:8392348 [Crossref] [PubMed]
- Flatley C, Gibbons K, Hurst C, et al. Cross-validated prediction model for severe adverse neonatal outcomes in a term, non-anomalous, singleton cohort. BMJ Paediatr Open 2019;3:e000424 [Crossref] [PubMed]
- Jeong S, Cheng Q, Huang L, et al. Risk stratification system to predict recurrence of intrahepatic cholangiocarcinoma after hepatic resection. BMC Cancer 2017;17:464. [Crossref] [PubMed]
- Tsujikawa H, Tanaka S, Matsukuma Y, et al. Development of a risk prediction model for infection-related mortality in patients undergoing peritoneal dialysis. PLoS One 2019;14:e0213922 [Crossref] [PubMed]