Genome-wide DNA methylome and whole-transcriptome landscapes of spontaneous intraductal papilloma in tree shrews
Introduction
Intraductal papilloma (IP), a benign tumour that forms in the breast ducts, accounts for approximately 10% of cases of benign breast lesions (1). IP, which is caused by the abnormal proliferation of ductal epithelial cells, most commonly affects women between the ages of 35 and 55 years old (2). Hormones, fertility, and diet are all risk factors that predispose women to the development of IP (3). Because IP is related to atypia, ductal carcinoma in situ (DCIS), and carcinoma, it is classified as a high-risk precursor lesion and carries a 6.3% risk of malignancy (3); upon surgical excision, IP may be upgraded to atypical ductal hyperplasia or DCIS (4). However, at present, the mechanism of breast neoplasms is not fully understood, and multidimensional molecular data from IP patients have not been fully integrated in studies on this topic.
The results of DNA sequencing research have confirmed that tree shrews are closely related to primates (5). Consequently, tree shrews have become an increasingly popular experimental animal model for various human tumours, including lung cancer (6), hepatocellular carcinoma (7), and glioblastoma (8). Genome sequencing of Chinese tree shrews was first accomplished in 2013 and has provided a useful resource for functional genomic studies since (9). A database of the genome sequencing data of tree shrews has also been established (10). Most importantly, in terms of morphology and structure, the mammary glands of tree shrews are similar to those of humans (11). Based on these qualities, tree shrews are ideal experimental animals for studying the pathogenesis of mammary tumours. However, few studies have used tree shrews as a novel breast tumour animal model to examine gene expression patterns and the underlying function of DNA methylation in the tumorigenesis of spontaneous IP.
DNA methylation is one of the epigenetic changes that has been shown to play a key role in the pretranscriptional regulation and inhibition of gene expression in multiple mammalian genomes. The mapping of genome-wide DNA methylation is of great importance to understanding tumorigenesis (12). DNA methylation is implicated in many cancers, including thyroid cancer (13), non-small cell lung cancer (14), and gastric cancer (15), as well as in the development and progression of breast cancer (16). Limited evidence has also indicated that the aberrant methylation of cytosine residues is involved in the development of IP (17). Therefore, delineating the DNA methylation profile and identifying differentially methylated genes (DMGs) in IP would be helpful to understanding the tumorigenesis of papilloma from the perspective of epigenetic regulation.
It is generally believed that the abnormal reprogramming of the whole transcriptome, including genes, long noncoding RNAs (lncRNAs), and circular RNAs (circRNAs), is a crucial process in the occurrence and progression of tumours. Recently, RNA sequencing (RNA-seq) studies of breast cancer (18) have been conducted to inform a deeper understanding of the mechanisms involved, and research on the potential underlying molecular mechanisms influencing breast cancer occurrence and development has been performed in murine mammary tumour models (19). By analysing methylome and transcriptome variations related to the survival status of patients with breast cancer, we can obtain a deeper understanding of the basic biological process of breast cancer based on its genetic aetiology (20). Moreover, the results of analysis of DNA methylation and gene expression have demonstrated that the methylation level of CpGs in breast cancer tissues is significantly higher than that in adjacent normal tissues. Additionally, large numbers of CpGs exhibit a significantly higher methylation level than that found in nearby normal tissues, which is negatively correlated with gene expression (21). Thus, by combining methylation data and gene expression profile data, we can better analyse the regulatory function of methylation to solve existing conundrums.
At present, studies on DNA methylation and the transcriptome in IP are lagging behind those on malignant breast cancer. Therefore, in the present work, we carried out an integrated analysis of genome-wide DNA methylation levels and the whole transcriptome in breast IP in tree shrews. Our study provides new insights into IP in tree shrews, highlights candidate tumorigenesis-eliciting genes, and will contribute to the use of tree shrews in breast tumour animal models. We present the following article in accordance with the MDAR and ARRIVE reporting checklist (available at http://dx.doi.org/10.21037/atm-21-1293).
Methods
Tissue specimens from tree shrews and their histology
Six female tree shrews were obtained from the Institute of Medical Biology, Chinese Academy of Medical Sciences (IMB-CAMS; Kunming, China). Experiments were performed under a project license (No.: DWSP201809003) granted by the animal ethics committee of IMB-CAMS, in compliance with IMB-CAMS guidelines for the care and use of animals.
Breast tumour tissues and normal breast tissues were collected after euthanasia of the animals by intraperitoneal injection of pentobarbital sodium (100 mg/kg). The mammary tumours were surgically removed. A portion of each tissue sample was dissected, fixed in a 4% paraformaldehyde solution, embedded in paraffin, and stained with haematoxylin and eosin (HE) before histological examination by pathologists. Another portion of each tissue sample was immediately frozen in liquid nitrogen and stored at –80 °C for subsequent experiments.
Library construction and whole-genome bisulfite sequencing (WGBS)
Genomic DNA was extracted from the samples using the cetyltrimethylammonium bromide (CTAB) method, and the DNA concentration and integrity were determined with a NanoPhotometer® spectrophotometer (IMPLEN, CA, USA) and agarose gel electrophoresis, respectively. Then, DNA libraries were prepared for bisulfite sequencing. Briefly, genomic DNA was fragmented into 100–300 bp fragments by sonication (Covaris, Massachusetts, USA) and purified with a MiniElute PCR Purification Kit (QIAGEN, MD, USA). The DNA fragments were end-repaired, and a single “A” nucleotide was added to the 3' end of the blunt fragments. Then, the genomic fragments were ligated to methylated sequencing adapters. Fragments with adapters were subjected to bisulfite conversion using the Methylation-Gold kit (ZYMO, CA, USA), and unmethylated cytosines were converted to uracils through sodium bisulfite treatment. Finally, the converted DNA fragments were amplified by polymerase chain reaction (PCR) and sequenced on an Illumina HiSeqTM 2500 instrument.
Methylation level analysis
After data filtering, the acquired clean reads were mapped to the Tupaia chinensis (Chinese tree shrew) reference genome (TupChi_1.0) (GCF_000334495.1) using BSMAP software (v2.90) (22). A custom Perl script (23) was then applied to call methylated cytosines, and a correction algorithm was applied to the methylated cytosine results. Methylation levels were calculated according to the methylated cytosine percentage in the global genome as well as that in variant regions of the genome for each sequence context (CG, CHG, and CHH). Variant methylation patterns in variant genomic regions were estimated by plotting the methylation profiles of the flanking 2 kb regions and the gene body based on the average methylation level for each window.
Differentially methylated region (DMR) analysis
Methylkit software (v1.4.1) (24) was used for the analysis of differential DNA methylation. To investigate DMRs between the IP and control groups, the minimum read coverage to call the methylation status of a base was set to 4. A 200-bp window was used to scan the whole genome, and the average DNA methylation rate was calculated in each window (a certain type of C). Then, the differences in the methylation level of each sample in each window were compared. The coding genes were divided into 3 regions: 2 kb upstream, gene body, and 2 kb downstream. Then, we analysed the location of the DMR to determine the DMGs. Gene Ontology (GO) and Kyoto Encyclopedia of Genes and Genomes (KEGG) pathway enrichment analyses were performed to explore the functional enrichment of genes influenced by DMRs.
Strand-specific library construction and whole-transcriptome sequencing
First, total RNA was extracted using TRIzol, and ribosomal RNA (rRNA) was removed to retain messenger RNAs (mRNAs) and non-coding RNAs (ncRNAs). The enriched mRNAs and ncRNAs were fragmented into short fragments using fragmentation buffer and then reverse-transcribed into complementary DNA (cDNA) with random primers. Second-strand cDNA was synthesized with DNA polymerase I, RNase H, dNTP (dUTP instead of dTTP) and buffer. Next, the cDNA fragments were purified with a QiaQuick PCR extraction kit, end-repaired, subjected to poly (A) addition, and ligated to Illumina sequencing adapters. The second-strand cDNA was then digested with UNG (uracil-N-glycosylase). Finally, the digested products were size-selected by agarose gel electrophoresis, and subjected to PCR amplification and sequencing on the Illumina HiSeqTM 4000 platform.
Alignment with rRNA and the reference genome
After the filtering of clean reads, Bowtie2 (v2.2.8) (25) was applied to map reads to the rRNA database (ftp://ftp.ncbi.nlm.nih.gov/genbank/), and the rRNA mapped reads were removed. The remaining reads were used for subsequent assembly and analysis. The removed rRNA reads were also mapped to TupChi_1.0 using TopHat2 (v2.1.1) (26). After alignment with TupChi_1.0, the reads mapped to the genomes were removed, and the unmapped reads were collected for circRNA identification. Unmapped reads were aligned to the reference genome with Bowtie2 again, and the enriched unmapped reads were split into smaller segments, which were subsequently used to identify potential splice sites.
Transcript reconstruction
The reconstruction of transcripts was performed with Cufflinks software (27), together with TopHat2, to identify new genes and new splice variants of known genes. The reference annotation-based transcripts (RABT) program was preferred. To make up for the effect of low-coverage sequencing, Cufflinks generated faux reads based on reference. Finally, all reassembled fragments were aligned with reference genes, and similar fragments were discarded.
Identification and annotation of novel transcripts
To identify new transcripts, all reconstructed transcripts were aligned to TupChi_1.0. For the identification of predictable novel genes, the following parameters were applied: length of transcript >200 bp and exon number >2. To acquire protein functional annotations, the novel transcripts were aligned to the Nr, KEGG, and GO databases.
CircRNA identification and database annotation
To identify unique anchor positions within splice sites, 20-mers were extracted from both ends of the unmapped reads and aligned to TupChi_1.0. Anchor reads that aligned in the reverse orientation (head to tail) and showed circRNA splicing were further subjected to find_circ (28) analysis for circRNA identification. Anchor alignments were extended until the complete read was aligned and the breakpoints were flanked by GU/AG splice sites. A candidate circRNA was called if it was supported by at least 2 unique back-spliced reads. The identified circRNAs were also subjected to statistical analysis of their type and length distribution. Finally, the circRNAs were subjected to BLAST searches against circBase (29) for annotation, and those that were unable to be annotated were defined as novel circRNAs.
LncRNA prediction and analysis
Coding-non-coding index (CNCI) (v2) (30) and coding potential calculator (CPC) (31) were applied for evaluation of the protein-coding potential of novel transcripts according to default parameters. To obtain protein annotations, novel transcripts were also mapped to the SwissProt database. Those showing the intersection of neither protein-coding potential nor protein annotation results were selected as lncRNAs. To investigate the interaction between antisense lncRNAs and mRNAs, complementary correlation analysis was performed using RNAplex (32). The program contains the ViennaRNA package (33) and predicts the best base pairing according to thermodynamic structure on the basis of the calculation of minimum free energy.
Quantification of the abundance of transcripts and circRNAs
Transcript abundance was quantified by RSEM (RNA-Seq by Expectation-Maximization) (34). The fragments per kilobase of transcript per million mapped reads (FPKM) method was applied for the normalization of transcript expression levels. Additionally, the reads per million mapped reads (RPM) method was used to scale back-spliced junction reads for circRNA quantification.
Correlation of DNA methylation and gene expression
To identify whether the DNA methylation level in DMRs affects gene expression between groups, genes were classified according to their genomic location, including the ±2 kb flanking regions and gene body region. Spearman’s correlation analysis was used to identify the statistical relationships between gene expression and DNA methylation within the gene body and ±2 kb flanking regions. Rho <0 indicated a negative correlation and Rho >0 indicated a positive correlation. To investigate the underlying functions of DNA methylation which are responsible for differential gene expression, common genes between the DMR-related genes and DEGs were analysed, and GO and KEGG pathway enrichment analyses were conducted for DEGs with DMRs.
Functional enrichment analysis
GO enrichment analysis recognizes the key biological functions of genes by providing all GO terms that are significantly enriched in genes compared to the genomic background, and also enables genes to be filtered according to their biological functions. Genes generally interact with each other to carry out specific biological functions. Pathway-based analysis contributes to further identification of genes’ biological functions, with KEGG being the primary public pathway-related database (35). All genes were mapped to GO terms in the GO database, gene numbers were calculated for each term, and the significantly enriched GO terms were identified. Pathway enrichment analysis revealed signal transduction or metabolic pathways that were significantly enriched in genes compared to the genomic background. To discern whether a set of genes enriched in distinct GO terms/pathways showed significant differences between the 2 groups, a gene set enrichment analysis (GSEA) was carried out using GSEA software (36). Briefly, we input a gene expression matrix and ranked genes by using a SinaltoNoise normalization program. Enrichment scores and P values were calculated using default parameters.
Statistical analysis
Mean ± SEM and multiple t tests for methylation levels were calculated using GraphPad Prism 8 (GraphPad, San Diego, USA). The DMRs in each sequence context (CG, CHG, and CHH) were identified based on the following criteria: for CG, CHG, CHH, and all C, the number of CG, CHG, CHH, and all C sites in each window needed to be ≥5, 5, 15, and 20, respectively; the absolute value of the difference in the methylation ratio needed to be ≥0.25, 0.25, 0.15, and 0.2, respectively; and Q≤0.05 was required in all cases. The edgeR package was used to determine the transcripts and circRNAs that were differentially expressed between the 2 groups. For each comparison, we identified mRNAs with a fold change (FC) ≥2 and a false discovery rate (FDR) <0.05 as DEGs, and circRNAs/lncRNAs with a FC ≥2 and a P value <0.05 as differentially expressed circRNAs/lncRNAs. GO terms/KEGG pathways meeting the criterion that calculated P values were subjected to FDR correction, with a P value ≤0.05 as the threshold, were defined as significantly enriched GO terms/KEGG pathways in genes.
Results
Diagnosis and pathologic identification of spontaneous breast IP in tree shrews
With respect to the basic pathology of tree shrew breast IPs, the ductal epithelium of the breast showed papillary hyperplasia, the nipple varied in size, and the cells showed no atypia, which is similar to human pathology. The tumours were expected to be benign (Figure 1A). In contrast, examination of normal tree shrew mammary glands showed the breast acini and ducts to have a normal structure, and no degeneration, necrosis, or inflammatory cell infiltration was observed (Figure 1B). We confirmed that 3 of the spontaneous mammary tumours collected from females in the closed colony of tree shrews were breast IPs. The selected tree shrews were divided into 2 groups: the IP group (n=3), comprising tree shrews with IPs (IP-1, IP-2, and IP-3); and the control group (n=3), comprising tree shrews with healthy mammary gland tissues (control-1, control-2, and control-3). The results indicated that the selected IP tree shrews and normal tree shrews were appropriate for subsequent analyses.
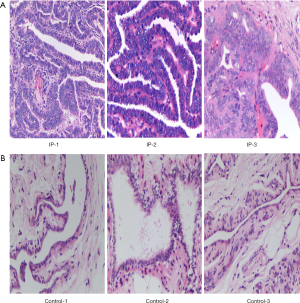
Genome-wide DNA methylation profiling of breast IPs and normal mammary gland tissues of tree shrews
To investigate methylation patterns during IP development in tree shrews, we analysed genome-wide DNA methylation (DNAm) levels in tissues from the IP group and control group by WGBS with >99% conversion efficiency (Table S1). The genome of the normal mammary gland tissue sample (control group) presented ~4.39% methylated cytosines (mCs), and the IP sample presented ~4.41% mCs among the total sequenced C sites, reflecting the degree of genome methylation. The methylation levels of CG, CHH, and CHG (in which H is A, C, or T) sites were distinct. The genome-wide mC levels were detected as 88.08%±1.76% for CG, 2.52%±0.32% for CHG, and 9.40%±1.45% for CHH in the control group, and 90.19%±0.54% for CG, 2.07%±0.04% for CHG, and 8.41%±0.19% for CHH in the IP group. Thus, the proportions of these contexts were analogous between groups (Figure 2A).
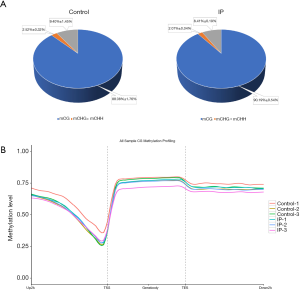
Epigenetic variation among DNA sequences, especially CpG DNA methylation, is an important type of variation that modulates gene expression under different physiological and pathological conditions. Our results showed that in the IP group, the CG methylation levels were higher, the CHG and CHH methylation levels were lower, and the number of mCG sites was increased compared with the control group, whereas no significant difference was found in other types of sites. To further compare the distribution of coding genes as well as the methylation levels of diverse functional genomic elements between the 2 groups, we analysed the DNA methylation pattern in 3 distinct regions of transcriptional elements: the upstream 2k region [2,000 bp before the transcription start site (TSS) of the gene], the gene body region, and the downstream 2k region (2,000 bp after the transcription termination site). The distribution characteristics of DNA methylation levels in distinct functional regions can aid in the understanding of the characteristics of DNA methylation modifications in different regions at the whole-genome level.
The obtained DNA methylation profiles showed that the methylation level in the CG context was higher than those in the CHG and CHH contexts. The DNA methylation level in the CG context was highest in the gene body region. DNA methylation was moderately high in the upstream 2K start site, decreased dramatically from the upstream 2k region to the TSS, increased sharply from the TSS to the gene body region, maintained the highest level in the gene body region, and then decreased slightly in the downstream 2K region (Figure 2B).
A total of 3,486 differentially methylated CG regions, 82 CHG regions, and 361 CHH regions were identified. In the CG context, 3,486 DMRs, located in 701 genes, were identified between the IP and control groups (Q<0.05); among them, 705 showed increased methylation and 2,781 showed decreased methylation in IP tissues compared with control tissues. The union of the DMRs of all samples was taken, and a heat map of the CG methylation rates of regions was drawn (Figure 3A). The results revealed that the CG methylation rates showed discrepant levels in the IP and control group samples.
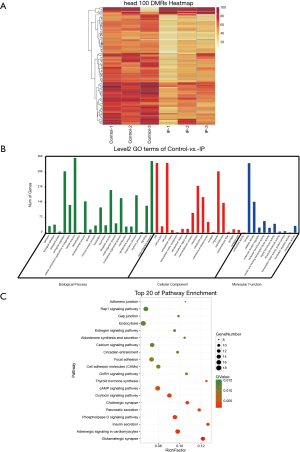
To further elucidate the biological functions of the DMGs, we performed GO and KEGG pathway analyses. The GO term analysis revealed that the putative target genes of KLF5 were associated with terms such as developmental process (P adjust =0.0056) and single-organism developmental process (P adjust =0.0103) in the biological process (BP) category, binding (P=0.0063) in the molecular function (MF) category, and membrane (P=0.0045) in the cellular component (CC) category (Figure 3B). KEGG pathway analysis showed that 15 DMGs were associated with the oxytocin signalling pathway (Q=0.0027), and 10 DMGs were associated with the oestrogen signalling pathway (Q=0.0113) (Figure 3C). Taken together, these gene annotation results revealed that the majority of DMGs showing increased methylation or decreased methylation were mapped to the gene body region, suggesting that DMGs play critical roles in IP.
DEGs between the IP group and the control group
To systematically describe the transcriptome landscape of the IP group and the control group, whole-transcriptome sequencing was performed. After the removal of low-quality reads from each library, the clean reads were combined and aligned to TupChi_1.0, resulting in the identification of 10,051 known mRNAs, 25,481 novel mRNAs, 1,022 known lncRNAs, 1,617 novel lncRNAs, and 10,602 new circRNAs in the IP group and the control group in total. We further compared the transcriptomic landscapes of the normal mammary gland and IP tumour tissues of tree shrews. A total of 39 upregulated DEGs and 23 downregulated DEGs were identified in IP tumours compared with normal mammary glands (|log2FC| ≥1 & FDR <0.05) (Figure 4A). Among these DEGs, the expression levels of ST14, PSMF1, and TNFSF11 in the IP group were more than 15-fold higher than those in the control group. In the control group, the levels of FAM192A and Psmc5 were 15-fold higher, respectively, than those in the IP group.
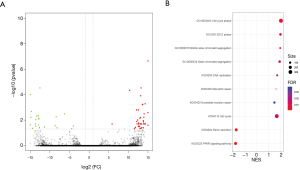
GO analysis of the DEGs showed that TNFSF11 participates in tumour necrosis factor-mediated signalling pathway and cellular response to tumour necrosis factor (P=0.0042); GATA-binding protein 3 (GATA3), EPHA2, and PTPRG are involved in regulation of epithelial cell migration (P=0.0072); STAG2, TEX14, PPP1R9B, and TPR are involved in regulation of cell cycle processes (P=0.0087); and TNFSF11, and EPHA2 are involved in epithelial cell proliferation (P=0.0091). The KEGG analysis revealed that ACSL1 and APOA5 participate in PPAR signalling pathway (P=0.0367). Furthermore, GSEA was utilized to analyse groups of functionally relevant genes in the IP group compared to the control group (Figure 4B). In the IP group, under the BP category, the upregulation of cell cycle phase- and S phase-related terms in the biological phase subcategory, and mitotic sister chromatid segregation and sister chromatid segregation in the cellular component organization or biogenesis subcategory was observed. Among the DEGs identified in the IP group, the upregulated genes were involved in replication and repair, including DNA replication, mismatch repair and nucleotide excision repair, as well as in cell growth and death, including the cell cycle. Meanwhile, the downregulated genes were involved in the endocrine system, including the renin secretion and Peroxisome proliferators-activated receptor (PPAR) signalling pathway (Figure S1). Overall, the enrichment analysis of GO terms and pathways demonstrated that many of the DEGs identified in the IP group are involved in tumorigenesis.
The lncRNA expression profile is distinct between IP and normal mammary gland tissues of tree shrews
Compared with the control group, 50 differentially expressed lncRNAs (DElncRNAs), of which 39 were upregulated and 11 were downregulated, were identified in the IP tissue samples (|log2FC| ≥1 & P<0.05) (Figure 5A). In the IP group, the most significantly upregulated lncRNAs were TCONS_00002926, TCONS_00077528, XR_001369927.1, TCONS_00037489, and XR_333956.1, while the most significantly downregulated lncRNAs were TCONS_00016941, XR_334171.1, TCONS_00135842, TCONS_00077456, and TCONS_00094673. To reveal the functions of the lncRNAs, the complementary correlation of antisense lncRNAs and mRNAs was predicted. All antisense target genes of the DElncRNAs were subjected to GO and KEGG pathway analyses to determine their functions (Figure 5B,C). Various relevant GO terms in the BP category were observed, including blood vessel endothelial cell migration (P=3.99E−05), epithelial cell migration (P=0.0012), epithelium migration (P=0.0012), and negative regulation of apoptotic process (P=0.0016). KEGG pathway analysis revealed that Jak-STAT signalling pathway (P=0.0087), PPAR signalling pathway (P=0.0233), and oestrogen signalling pathway (P=0.0292) were associated with the DElncRNAs.
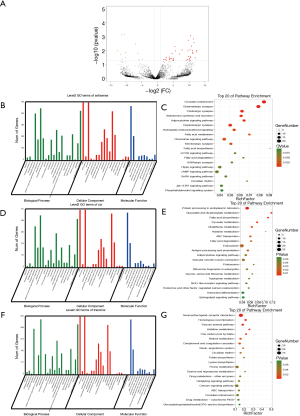
The 2nd function of lncRNAs, when located less than 10 kb upstream/downstream from a gene, is to act as cis-regulators of their neighbouring genes on the same strand. A large number of enriched GO terms were observed in the BP category, including branching morphogenesis of an epithelial tube (P=0.0001), serine phosphorylation of STAT protein (P=0.0002), negative regulation of cell growth (P=0.0017), and execution phase of apoptosis (P=0.0019) (Figure 5D). The KEGG pathway analysis revealed that the DElncRNAs were associated with the pathways of protein processing in endoplasmic reticulum (P=0.0017), glyoxylate and dicarboxylate metabolism (P=0.0018), antigen processing and presentation (P=0.0250), and NOD-like receptor signalling (P=0.0483) (Figure 5E).
The 3rd function of lncRNAs is the trans-regulation of non-adjoining co-expressed genes. To determine the target genes of the lncRNAs, the correlation between lncRNA and mRNA expression was analysed, and GO function and KEGG pathway enrichment analyses of protein-coding genes with an absolute correlation >0.9 were performed. In the BP category, they included mesenchymal stem cell differentiation (P=0.0032), extrinsic apoptotic signalling pathway (P=0.0070), recombinational repair (P=0.0086), and execution phase of apoptosis (P=0.0125) (Figure 5F). The DElncRNA trans-regulated co-expressed genes were significantly enriched in KEGG pathways including homologous recombination (P=0.0004), histidine metabolism (P=0.0022), and renin-angiotensin system (P=0.0244) (Figure 5G). These results suggested that the DElncRNAs might be involved in the tumorigenesis of IP in tree shrews via the regulation of tumorigenesis-related genes and signalling pathways.
The circRNA expression profile differs between IP and normal mammary gland tissues of tree shrews
As shown in Figure 6A, we identified 32 differentially expressed circRNAs (DEcircRNAs), including 25 upregulated circRNAs and 7 downregulated circRNAs, between the IP and control groups (|log2FC|≥1 & P<0.05). Heat map analysis of the DEcircRNAs showed that the IP tissues could be separated from the normal mammary gland tissues by the DEcircRNAs. The most significantly upregulated circRNAs in the IP group were novel_circ_004184, novel_circ_001608, novel_circ_007270, novel_circ_004893, and novel_circ_004886, while novel_circ_007552, novel_circ_007844, novel_circ_002826, novel_circ_005946 and novel_circ_009457 were identified as downregulated circRNAs.
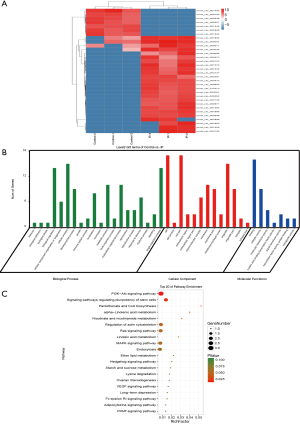
The gene of origin of a circRNA is its parental gene. Therefore, we carried out a functional enrichment analysis of parental genes to investigate the putative functions of DEcircRNAs. GO analysis revealed that the parental genes of the DEcircRNAs were enriched in the BP terms of branch elongation of an epithelium (P=0.0161), negative regulation of cell proliferation (P=0.0173), positive regulation of epithelial cell proliferation (P=0.0233), vasculogenesis (P=0.0276), and gland morphogenesis (P=0.0290). The PI3K-Akt signalling (P=0.0139) and Ras signalling (P=0.0509) pathways were identified as being significant in the KEGG enrichment analysis (Figure 6B, C). Taken together, these results suggested that these DEcircRNAs may affect IP tumour development by influencing gene expression.
Integrated analysis of the methylome and transcriptome data
To examine whether differences in DNA methylation could be the basis of the observed gene expression differences between tree shrews in the IP and control groups, we analysed the correlation between gene expression and DNA methylation data in the 2 groups. The results indicated a considerable regulatory effect of DNA methylation in the modulation of gene expression. In both the IP and control groups, the methylation levels in the upstream 2K (Pearson’s R =−0.0383 and −0.0192, respectively) and gene body (Pearson’s R =−0.0922 and −0.0737, respectively) were negatively correlated with expression levels. In the control group, the methylation levels in the downstream 2K regions were also negatively correlated with expression levels (Pearson’s R =−0.0013); however, in the IP group, a positive correlation was observed (Pearson’s R =0.0030) (Figure 7A,B).
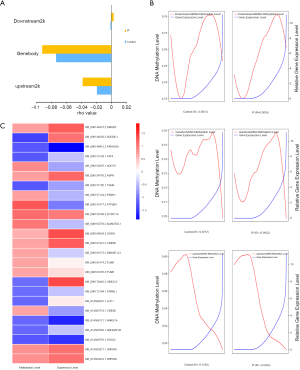
In addition, DMGs and DEGs were compared through integrated methylomic and transcriptomic analysis, which revealed 25 genes with differential methylation and expression according to both RNA-seq (P<0.05) and WGBS (Q<0.05) (Figure 7C). Among these genes, the number of genes exhibiting DMRs in the upstream 2K, gene body, and downstream 2K regions was 1, 23, and 1, respectively. The GO analysis of the 25 genes which were both DEGs and DMGs showed that 15 genes showed enrichment in 193 BP terms (P<0.05). Among these genes, ATPase plasma membrane Ca2+ transporting 4 (ATP2B4) was involved in regulation of calcium-mediated signalling (P=0.0008), calcium-mediated signalling (P=0.0022), negative regulation of catabolic processes (P=0.0031), and negative regulation of calcium-mediated signalling (P=0.0061); PDZ domain-containing 1 (PDZK1) was involved in positive regulation of transmembrane transport (P=0.0014), positive regulation of transport (P=0.0031), and regulation of transmembrane transport (P=0.0126); Lymphocyte cytosolic protein 1 (LCP1) was involved in regulation of intracellular transport (P=0.0034) and regulation of cellular localization (P=0.0151); and PDZK1 and LCP1 were involved in regulation of transport (P=0.0135). The KEGG analysis of the 25 genes which were both DEGs and DMGs showed that 9 genes were enriched in 19 signalling pathways (P<0.05). Subsequent analysis identified 9 genes with an inverse relationship between the degree of DNA methylation and gene expression in gene body regions (Table 1), which were related to signal transduction and the endocrine system. Three differentially over-methylated and downregulated genes (ADCY5, ATP2B4, and CREB5) were associated with signal transduction pathways, including cAMP signalling pathway (P=3.13E−06), cGMP-PKG signalling pathway (P=6.76E−05), TNF signalling pathway (P=0.0118), and AMPK signalling pathway (P=0.0131). Furthermore, ADCY5 and CREB5 were involved in insulin secretion (P=0.0001) and oestrogen signalling pathway (P=0.0002) in endocrine system. Thus, we integrated the gene expression and DNA methylation maps and identified protein-coding genes with underlying changes related to DNA methylation in IP; the resulting alterations probably induce the development of breast tumours.
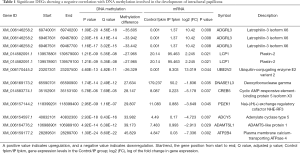
Full table
Discussion
In this work, we have provided an expanded overview of the DNA methylation levels and transcriptomic characteristics of 3 tumour tissue samples of spontaneous breast IP and 3 normal mammary gland tissues from tree shrews, in which gene expression was analysed. Tree shrews are considered to have potential as an animal model for the study of mammary tumours, including both spontaneous and induced models. Daino et al. used a microarray to obtain the DNA methylation and expression profiles of γ-ray-induced mammary carcinomas in rats. They found polycomb repressive complex 2 (PRC2) mediated aberrant DNA methylation and a consequent dysregulation of downstream gene targets during carcinogenesis (37). In this study, we obtained the genomic DNA methylation profiles of normal mammary gland and IP tissues from tree shrews. The DNA methylation levels were reduced in tree shrews with IP compared with healthy tree shrews. Also, GATA3 was upregulated 12.2-fold in IP tissues compared with normal mammary gland tissues; however, no difference was found in DMRs. The different results between these 2 studies may be due to the differences in the experimental animals used and the types of mammary tumours examined.
In their study, Shao et al. (38) identified 17 Krüppel-like factors from Chinese tree shrews. KLF5 encodes a member of the zinc finger protein KLF subfamily which acts as transcriptional activator by binding directly to a specific recognition motif in the promoters of target genes, through which it plays roles in both promoting and suppressing cell proliferation. Tupaia belangeri (Tb) KLF5, which is similar to human Krüppel-like factor (hKLF) hKLF5, significantly promotes cell proliferation, playing a pro-proliferative and oncogenic role in breast cancer (39). These findings suggested that tree shrews may serve as an alternative animal model in breast cancer studies related to KLF5 (38). Although KLF family members were not included among the DEGs in this study, DNA methylation analysis showed that KLF5 displayed significant over-methylated among the identified DMRs.
To obtain more in-depth insights into the molecular mechanisms underlying tumorigenesis, we carried out a correlation analysis of the RNA-seq and WGBS data. The results suggested an inverse correlation of PDZK1, ATP2B4, and LCP1 gene expression with DNA methylation between the IP and control groups. PDZK1 encodes a PDZ domain-containing scaffolding protein (40). Genome-wide association study of a large cohort identified rs12405132 of PDZK1 as a new susceptibility locus in breast cancer; hence, PDZK1 is a potential interacting gene in breast cancer (41). In primary breast cancers, PDZK1 is an oestrogen-regulated gene, the overexpression of which is found in oestrogen receptor (ER)-positive breast cancers (42). PDZK1 was identified as a marker of oestrogen-regulated gene expression in a study examining the relationship between the menstrual cycle and ER-positive breast cancer (43). Dunbier et al. further verified that in postmenopausal patients with primary ER-positive breast cancer, the expression of PDZK1 was strongly related to plasma oestradiol level (44). PDZK1 exhibits epithelial expression with a primarily cytosolic subcellular localization, and its expression is indirectly modulated by ER-α stimulation (45).
ATP2B4 encodes plasma membrane calcium ATPase isoform 4 (PMCA4b), which belongs to the P-type primary ion transport ATPase family. The ATP2B4 protein, which is located primarily in the plasma membrane, is expressed in normal breast tissue and plays a key role in the plasma membrane Ca2+ pump in the maintenance of mammary epithelial Ca2+ homeostasis (46). The PMCA4 protein is found in normal breast ductal epithelia; however, as reported by Varga et al., a variety of factors, including hormonal imbalances, epigenetic modifications, and impairment of protein trafficking may lead to a loss of PMCA4b in breast cancer (47). The same study showed that the regulation of Ca2+ signalling through increased PMCA4b expression may be conducive to the normal development of the breast epithelium. Consistent with the results of this previous study, we found that the expression of ATP2B4 mRNA was downregulated 7.3-fold in our IP group, while methylation was increased. In breast cancer treatment, the targeting of PMCA4 may enhance the effectiveness of breast cancer therapies which act by promoting cell death pathways (48). The targeted regulation of PMCA4 functionality may give rise to novel therapeutic methods to attenuate or facilitate new vessel formation in breast cancer, which is associated with angiogenesis (49).
LCP1 is an L-plastin protein-coding gene and a member of the actin-binding protein family. It is conserved during eukaryote evolution, and its expression is found in most tissues of higher eukaryotes. LCP1 plays a critical role in T-cell activation, and is associated with Nuclear factor kappa-B (NF-κB) signalling, calcium ion binding, and actin binding. The induction of L-plastin expression is concomitant with tumorigenesis in solid tissues. A negative effect of LCP1 on breast cancer progression has been evidenced, and LCP1 inhibition results in the migration, invasion, and proliferation of breast cancer (50). Mutations in LCP1 have been reported as putative cancer drivers on the basis of whole-exome sequencing in independent benzo[a]pyrene (BaP)-derived post-stasis human mammary epithelial cell strains (51). L-plastin is a protein that exerts a cell-protective effect against TNF cytotoxicity in breast cancer cell lines (52). The actin-binding protein LCP1/L-PLASTIN has been verified to participate in CXCL12/CXCR4 signalling in breast cancer cells (53).
Therefore, we concluded that PDZK1, ATP2B4, and LCP1 might be key regulatory genes during the development of spontaneous IP in tree shrews. In addition, the DNA methylation of these genes may be a crucial functional regulator of tumorigenesis. Nevertheless, the epigenetic mechanisms participating in the modulation of these genes as well as genetic regions associated with the development of IP require further exploration.
Conclusions
Overall, our findings systematically demonstrate the changes in mRNA, lncRNA, and circRNA, and facilitate the characterization of the genome-wide DNA methylation profiles of IP tissue and normal mammary gland tissue in tree shrews, thus providing valuable evidence for an improved understanding of the development of mammary tumours. Our results also show that DNA methylation influences the expression of genes associated with the development of spontaneous IP in tree shrews. Such analyses greatly improve the progress in exploring the characteristics of DNA methylation in the development of breast IP and provide new directions for the study of epigenetic markers and target genes in spontaneous mammary tumours.
Acknowledgments
We thank Prof. Yunzhang Hu and Dr. Jiandong Shi (Institute of Medical Biology, Chinese Academy of Medical Sciences) for critical reading and constructive suggestions on this manuscript as well as Guangzhou Genedenovo Biotechnology Co., Ltd. for assisting in the sequencing and bioinformatics analyses.
Funding: This work was supported by Yunnan Science and Technology Talent and Platform Program (No. 2017HC019; 2018HB071), Yunnan Key Laboratory of Vaccine Research and Development on Severe Infectious Diseases (No. KF2015-01), the Yunnan Health Training Project of High Level Talents (No. D-2018026), and The Kunming Science and Technology Innovation Team (2019-1-R-24483).
Footnote
Reporting Checklist: The authors have completed the MDAR and ARRIVE reporting checklist. Available at http://dx.doi.org/10.21037/atm-21-1293
Data Sharing Statement: Available at http://dx.doi.org/10.21037/atm-21-1293
Conflicts of Interest: All authors have completed the ICMJE uniform disclosure form (available at http://dx.doi.org/10.21037/atm-21-1293). The authors have no conflicts of interest to declare.
Ethical Statement: The authors are accountable for all aspects of the work in ensuring that questions related to the accuracy or integrity of any part of the work are appropriately investigated and resolved. Experiments were performed under a project license (No.: DWSP201809003) granted by the animal ethics committee of IMB-CAMS, in compliance with IMB-CAMS guidelines for the care and use of animals.
Open Access Statement: This is an Open Access article distributed in accordance with the Creative Commons Attribution-NonCommercial-NoDerivs 4.0 International License (CC BY-NC-ND 4.0), which permits the non-commercial replication and distribution of the article with the strict proviso that no changes or edits are made and the original work is properly cited (including links to both the formal publication through the relevant DOI and the license). See: https://creativecommons.org/licenses/by-nc-nd/4.0/.
References
- Karadeniz E, Arslan S, Akcay MN, et al. Papillary Lesions of Breast. Chirurgia (Bucur) 2016;111:225-9. [PubMed]
- Soran A, Balkan M, Harlak A, et al. Complicated solitary intraductal papilloma of the breast. Int J Clin Pract 2008;62:160-1. [Crossref] [PubMed]
- Jung SY, Kang HS, Kwon Y, et al. Risk factors for malignancy in benign papillomas of the breast on core needle biopsy. World J Surg 2010;34:261-5. [Crossref] [PubMed]
- Eiada R, Chong J, Kulkarni S, et al. Papillary lesions of the breast: MRI, ultrasound, and mammographic appearances. AJR Am J Roentgenol 2012;198:264-71. [Crossref] [PubMed]
- Janecka JE, Miller W, Pringle TH, et al. Molecular and genomic data identify the closest living relative of primates. Science 2007;318:792-4. [Crossref] [PubMed]
- Ye L, He M, Huang Y, et al. Tree shrew as a new animal model for the study of lung cancer. Oncol Lett 2016;11:2091-5. [Crossref] [PubMed]
- Yang C, Ruan P, Ou C, et al. Chronic hepatitis B virus infection and occurrence of hepatocellular carcinoma in tree shrews (Tupaia belangeri chinensis). Virol J 2015;12:26. [Crossref] [PubMed]
- Tong Y, Hao J, Tu Q, et al. A tree shrew glioblastoma model recapitulates features of human glioblastoma. Oncotarget 2017;8:17897-907. [Crossref] [PubMed]
- Fan Y, Huang ZY, Cao CC, et al. Genome of the Chinese tree shrew. Nat Commun 2013;4:1426. [Crossref] [PubMed]
- Fan Y, Yu D, Yao YG. Tree shrew database (TreeshrewDB): a genomic knowledge base for the Chinese tree shrew. Sci Rep 2014;4:7145. [Crossref] [PubMed]
- Xia HJ, He BL, Wang CY, et al. PTEN/PIK3CA genes are frequently mutated in spontaneous and medroxyprogesterone acetate-accelerated 7,12-dimethylbenz(a)anthracene-induced mammary tumours of tree shrews. Eur J Cancer 2014;50:3230-42. [Crossref] [PubMed]
- Yang Y, Wu L, Shu XO, et al. Genetically Predicted Levels of DNA Methylation Biomarkers and Breast Cancer Risk: Data From 228 951 Women of European Descent. J Natl Cancer Inst 2020;112:295-304. [Crossref] [PubMed]
- Park JL, Jeon S, Seo EH, et al. Comprehensive DNA Methylation Profiling Identifies Novel Diagnostic Biomarkers for Thyroid Cancer. Thyroid 2020;30:192-203. [Crossref] [PubMed]
- Vrba L, Oshiro MM, Kim SS, et al. DNA methylation biomarkers discovered in silico detect cancer in liquid biopsies from non-small cell lung cancer patients. Epigenetics 2020;15:419-30. [Crossref] [PubMed]
- Peng Y, Wu Q, Wang L, et al. A DNA methylation signature to improve survival prediction of gastric cancer. Clin Epigenetics 2020;12:15. [Crossref] [PubMed]
- Xu Z, Sandler DP, Taylor JA. Blood DNA Methylation and Breast Cancer: A Prospective Case-Cohort Analysis in the Sister Study. J Natl Cancer Inst 2020;112:87-94. [Crossref] [PubMed]
- Lehmann U, Langer F, Feist H, et al. Quantitative assessment of promoter hypermethylation during breast cancer development. Am J Pathol 2002;160:605-12. [Crossref] [PubMed]
- Bao Y, Wang L, Shi L, et al. Transcriptome profiling revealed multiple genes and ECM-receptor interaction pathways that may be associated with breast cancer. Cell Mol Biol Lett 2019;24:38. [Crossref] [PubMed]
- Mei Y, Yang JP, Lang YH, et al. Global expression profiling and pathway analysis of mouse mammary tumor reveals strain and stage specific dysregulated pathways in breast cancer progression. Cell Cycle 2018;17:963-73. [Crossref] [PubMed]
- Kuang Y, Wang Y, Zhai W, et al. Genome-Wide Analysis of Methylation-Driven Genes and Identification of an Eight-Gene Panel for Prognosis Prediction in Breast Cancer. Front Genet 2020;11:301. [Crossref] [PubMed]
- Liu X, Peng Y, Wang J. Integrative analysis of DNA methylation and gene expression profiles identified potential breast cancer-specific diagnostic markers. Biosci Rep 2020;40:BSR20201053 [Crossref] [PubMed]
- Xi Y, Li W. BSMAP: whole genome bisulfite sequence MAPping program. BMC Bioinformatics 2009;10:232. [Crossref] [PubMed]
- Lister R, Pelizzola M, Dowen RH, et al. Human DNA methylomes at base resolution show widespread epigenomic differences. Nature 2009;462:315-22. [Crossref] [PubMed]
- Akalin A, Kormaksson M, Li S, et al. methylKit: a comprehensive R package for the analysis of genome-wide DNA methylation profiles. Genome Biol 2012;13:R87. [Crossref] [PubMed]
- Langmead B, Salzberg SL. Fast gapped-read alignment with Bowtie 2. Nat Methods 2012;9:357-9. [Crossref] [PubMed]
- Kim D, Pertea G, Trapnell C, et al. TopHat2: accurate alignment of transcriptomes in the presence of insertions, deletions and gene fusions. Genome Biol 2013;14:R36. [Crossref] [PubMed]
- Trapnell C, Roberts A, Goff L, et al. Differential gene and transcript expression analysis of RNA-seq experiments with TopHat and Cufflinks. Nat Protoc 2012;7:562-78. [Crossref] [PubMed]
- Memczak S, Jens M, Elefsinioti A, et al. Circular RNAs are a large class of animal RNAs with regulatory potency. Nature 2013;495:333-8. [Crossref] [PubMed]
- Glazar P, Papavasileiou P, Rajewsky N. circBase: a database for circular RNAs. RNA 2014;20:1666-70. [Crossref] [PubMed]
- Sun L, Luo H, Bu D, et al. Utilizing sequence intrinsic composition to classify protein-coding and long non-coding transcripts. Nucleic Acids Res 2013;41:e166 [Crossref] [PubMed]
- Kong L, Zhang Y, Ye ZQ, et al. CPC: assess the protein-coding potential of transcripts using sequence features and support vector machine. Nucleic Acids Res 2007;35:W345-9 [Crossref] [PubMed]
- Tafer H, Hofacker IL. RNAplex: a fast tool for RNA-RNA interaction search. Bioinformatics 2008;24:2657-63. [Crossref] [PubMed]
- Lorenz R, Bernhart SH, Honer Zu Siederdissen C, et al. ViennaRNA Package 2.0. Algorithms Mol Biol 2011;6:26. [Crossref] [PubMed]
- Li B, Dewey CN. RSEM: accurate transcript quantification from RNA-Seq data with or without a reference genome. BMC Bioinformatics 2011;12:323. [Crossref] [PubMed]
- Kanehisa M, Araki M, Goto S, et al. KEGG for linking genomes to life and the environment. Nucleic Acids Res 2008;36:D480-4. [Crossref] [PubMed]
- Subramanian A, Tamayo P, Mootha VK, et al. Gene set enrichment analysis: a knowledge-based approach for interpreting genome-wide expression profiles. Proc Natl Acad Sci U S A 2005;102:15545-50. [Crossref] [PubMed]
- Daino K, Nishimura M, Imaoka T, et al. Epigenetic dysregulation of key developmental genes in radiation-induced rat mammary carcinomas. Int J Cancer 2018;143:343-54. [Crossref] [PubMed]
- Shao M, Ge GZ, Liu WJ, et al. Characterization and phylogenetic analysis of Kruppel-like transcription factor (KLF) gene family in tree shrews (Tupaia belangeri chinensis). Oncotarget 2017;8:16325-39. [Crossref] [PubMed]
- Liu R, Shi P, Zhou Z, et al. Krupple-like factor 5 is essential for mammary gland development and tumorigenesis. J Pathol 2018;246:497-507. [Crossref] [PubMed]
- Kocher O, Krieger M. Role of the adaptor protein PDZK1 in controlling the HDL receptor SR-BI. Curr Opin Lipidol 2009;20:236-41. [Crossref] [PubMed]
- Michailidou K, Beesley J, Lindstrom S, et al. Genome-wide association analysis of more than 120,000 individuals identifies 15 new susceptibility loci for breast cancer. Nat Genet 2015;47:373-80. [Crossref] [PubMed]
- Ghosh MG, Thompson DA, Weigel RJ. PDZK1 and GREB1 are estrogen-regulated genes expressed in hormone-responsive breast cancer. Cancer Res 2000;60:6367-75. [PubMed]
- Haynes BP, Ginsburg O, Gao Q, et al. Menstrual cycle associated changes in hormone-related gene expression in oestrogen receptor positive breast cancer. NPJ Breast Cancer 2019;5:42. [Crossref] [PubMed]
- Dunbier AK, Anderson H, Ghazoui Z, et al. Relationship between plasma estradiol levels and estrogen-responsive gene expression in estrogen receptor-positive breast cancer in postmenopausal women. J Clin Oncol 2010;28:1161-7. [Crossref] [PubMed]
- Kim H, Abd Elmageed ZY, Ju J, et al. PDZK1 is a novel factor in breast cancer that is indirectly regulated by estrogen through IGF-1R and promotes estrogen-mediated growth. Mol Med 2013;19:253-62. [Crossref] [PubMed]
- Varga K, Paszty K, Padanyi R, et al. Histone deacetylase inhibitor- and PMA-induced upregulation of PMCA4b enhances Ca2+ clearance from MCF-7 breast cancer cells. Cell Calcium 2014;55:78-92. [Crossref] [PubMed]
- Varga K, Hollosi A, Paszty K, et al. Expression of calcium pumps is differentially regulated by histone deacetylase inhibitors and estrogen receptor alpha in breast cancer cells. BMC Cancer 2018;18:1029. [Crossref] [PubMed]
- Curry MC, Luk NA, Kenny PA, et al. Distinct regulation of cytoplasmic calcium signals and cell death pathways by different plasma membrane calcium ATPase isoforms in MDA-MB-231 breast cancer cells. J Biol Chem 2012;287:28598-608. [Crossref] [PubMed]
- Baggott RR, Alfranca A, Lopez-Maderuelo D, et al. Plasma membrane calcium ATPase isoform 4 inhibits vascular endothelial growth factor-mediated angiogenesis through interaction with calcineurin. Arterioscler Thromb Vasc Biol 2014;34:2310-20. [Crossref] [PubMed]
- Pillar N, Polsky AL, Shomron N. Dual inhibition of ABCE1 and LCP1 by microRNA-96 results in an additive effect in breast cancer mouse model. Oncotarget 2019;10:2086-94. [Crossref] [PubMed]
- Severson PL, Vrba L, Stampfer MR, et al. Exome-wide mutation profile in benzo[a]pyrene-derived post-stasis and immortal human mammary epithelial cells. Mutat Res Genet Toxicol Environ Mutagen 2014;775-776:48-54. [Crossref] [PubMed]
- Janji B, Vallar L, Al Tanoury Z, et al. The actin filament cross-linker L-plastin confers resistance to TNF-alpha in MCF-7 breast cancer cells in a phosphorylation-dependent manner. J Cell Mol Med 2010;14:1264-75. [Crossref] [PubMed]
- Inaguma S, Riku M, Ito H, et al. GLI1 orchestrates CXCR4/CXCR7 signaling to enhance migration and metastasis of breast cancer cells. Oncotarget 2015;6:33648-57. [Crossref] [PubMed]
(English Language Editor: J. Reynolds)