Construction and validation of a prognostic signature using CNV-driven genes for hepatocellular carcinoma
Introduction
Hepatocellular carcinoma (HCC) is a lethal malignancy and accounts for approximately 85% to 90% of primary liver cancers (1,2). Although targeted therapy and immunotherapy have emerged as potential therapies, curative therapies for HCC remain limited (3). Moreover, high post-operative recurrence rates and rare complete cures make it difficult for achieving long term survival. A study on natural history of HCC indicated that patients with advanced stage (Barcelona Clinic Liver Cancer Stage C) had a survival of only 3.4 months if untreated (4). HCC develops following a step-wise manner with abundant genetic and epigenetic molecular alterations (5). Therefore, it is crucial to achieve a better understanding of the underlying molecular mechanism that drives HCC occurrence and development. Exploring prediction model based on the factors that drive HCC can be useful for individualized therapy option and prognosis prediction for HCC patients.
As critical subclasses of somatic mutations, copy number variations (CNVs) refer to duplications or deletions of DNA segments, which are greater than 1 kb compared to a reference genome (6). CNVs account for the accumulation of genomic DNA aberrations, and play important role in cancer pathogenesis. Notably, CNVs can result in activation of oncogenes or inactivation of tumor suppressor genes, which drives cancer development (7,8). Multiple CNVs have been reported to be implicated in the pathogenesis and prognosis of cancers including HCC (9-12). Frequent CNVs of subpopulations of cancer cells were reported to contribute to HCC heterogeneity, indicating a critical role of CNVs in HCC development and progression (13). However, most previous studies focused on CNVs or transcriptome alterations separately, and a comprehensive study of how CNVs drives HCC is still lacking. Combining analysis of CNVs and corresponding gene expression will promote more accurate identification of the specific cancer signatures for HCC. In this study, we used transcriptomic and CNVs profiles to identify CNV-driven genes and aimed to construct a prognostic model for HCC. Our research may contribute to better understanding of the underlying mechanisms, and provide novel therapeutic targets for HCC treatment. We present the following article in accordance with the TRIPOD reporting checklist (available at http://dx.doi.org/10.21037/atm-20-7101).
Methods
Data collection
Gene expression profiles (374 tumor samples and 50 normal samples) and DNA CNVs data (379 HCC samples and 389 nontumor samples) of HCC patients were obtained from The Cancer Genome Atlas (TCGA) (https://portal.gdc.cancer.gov/, up to November 1, 2019). The corresponding clinical parameters were also obtained. HCC RNA-sequencing data were analyzed using the Illumina HiSeq 2000 RNA Sequencing platform, and CNVs data were analyzed with the Affymetrix SNP 6.0 platform. For validation cohort, RNA-sequencing profiles of 232 HCC patients with survival time and status were downloaded from the International Cancer Genome Consortium (ICGC) (https://dcc.icgc.org/, up to April 3, 2019). All analyses were performed according to relevant regulations and guidelines. The study was conducted in accordance with the Declaration of Helsinki (as revised in 2013).
Identification of differentially expressed genes (DEGs) between tumor and normal tissues
To identify genes critical for HCC development, we used the “edgeR” R package to select DEGs between tumor and nontumor samples from TCGA (14). The |log2 (fold change [FC])| >2 and false discovery rate (FDR) <0.01were used as cutoff value for screening DEGs.
Integrative analysis of gene expression and DNA CNVs
Genes in CNV regions were annotated using Genome Research Consortium Human build 38 (GRCh38) as reference genome. The copy variation ratios of the genes both in normal and tumor samples were calculated and the gene-CNV matrix was constructed for Chi-square test. CNVs alteration rates between normal and tumor samples were then compared using Chi-square test, and CNVs data with adjusted P values less than 0.05 were chosen for next analysis. Then the CNVs data and DEGs data of the same sample were merged to construct a matrix. By using Kolmogorov-Smirnov test, those genes showing the same tendency both in CNVs and differential gene expression were selected as CNV-driven genes. Moreover, the differential expression of CNV-driven genes between tumor and normal samples was compared by utilizing the Wilcoxon rank-sum test method.
Development and validating the risk prognostic model
Prognostic CNV-driven genes were screened to construct a prognostic prediction model for the TCGA set. We employed univariate Cox proportional-hazards regression analyses to evaluate the associations between CNV-driven DEGs and prognosis. Genes with a P<0.0001 in univariate Cox regression analysis were selected for subsequent analysis. Least Absolute Shrinkage and Selection Operator (LASSO) regression analysis was employed to remove redundant variables and minimize overfitting (15). Then multivariate Cox proportional-hazards regression analysis was conducted to generate coefficients that were used as weights in the prognostic model. The prognostic prediction model including eight genes was built through a linear combination of mRNA expression level. The risk score = (0.06124 × CDCA8 mRNA level) + (0.05817 × AKR1B15 mRNA level) + (0.07457 × EZH2 mRNA level) + (0.02522 × EPS8L3 mRNA level) + (0.05672 × CBX2 mRNA level) + (0.02529 × TRIM16L mRNA level) + (0.11022 × FLVCR1 mRNA level) + (0.11982 × GPRIN1 mRNA level). Based on the risk score model, patients were divided into two groups with high- or low-risks. The optimal risk score cutoff value was obtained using X-tile software (16). Kaplan-Meier (KM) and log-rank methods were used to compare the overall survival (OS) between the two subgroups. The receiver operating characteristic (ROC) curves were plotted, and the external validation of the predictive model was conducted in the ICGC database.
Independence of risk score from other clinical features
In TCGA group, 235 patients with both clinical information and corresponding gene expression were included in the analysis. In ICGC group, 232 patients were included for independence analysis. Univariate and multivariate analyses of OS were employed to evaluate whether the risk score was independent of other clinical features.
Functional enrichment analysis and genome annotation
To explore the underlying biological functions, we performed gene ontology (GO) and Kyoto encyclopedia of genes and genomes (KEGG) pathway enrichment analyses for CNV-driven genes. ClusterProfiler package (17) in R was used to plot the results.
Association between CNV-driven genes prognostic signature and tumor-infiltrating immune cells
Tumor Immune Estimation Resource (TIMER) is a comprehensive database for analyzing tumor-infiltrating immune cells (18). We utilized this database to estimate the abundance of major immune cell subpopulations in tumor immune microenvironment (CD4+ T cells, CD8+ T cells, B cells, macrophages, neutrophils, and dendritic cells) in HCC. The correlations between risk score and tumor-infiltrating immune cells were analyzed with Pearson test. Moreover, the expression levels of key immune checkpoint genes between high- and low-risk patients were compared by using Wilcoxon rank-sum test.
Statistical analysis
All the analyses were performed with R software (version 3.6.2). Unless otherwise specified, a P value less than 0.05 was considered statistically significant.
Results
DEGs in HCC
After data was collected as described in Methods, 3,598 DEGs between tumor and nontumor samples were identified. Among these DEGs, 3,298 genes were upregulated and 300 genes were downregulated. These DEGs were used for further analysis.
Identification of CNV-driven genes in HCC patients
By applying Chi-square test, 16,644 HCC-related CNV genes were identified (adjusted P<0.05). The distribution of HCC-related CNVs in the chromosomes is shown in Figure 1. Then CNV-driven genes were screened using Kolmogorov-Smirnov test. The Kolmogorov-Smirnov test identified 568 CNV-driven genes for HCC (Table S1). To illustrate the functional characteristics and biological effects of these CNV-driven genes, GO and KEGG analyses were conducted (Figure 2A,B). Results showed CNV-driven genes were significantly enriched in categories associated with cell division and proliferation, such as “nuclear division”, “chromosome, centromeric region”, and “ligand-gated ion channel activity”. These results indicate that the CNV-driven genes are involved in the dysregulation of tumor cell proliferation, and are critical in the molecular mechanisms of HCC development. Results from KEGG analysis showed that the top six signaling pathways were cell cycle, melanoma, p53 signaling pathway, mineral absorption, oocyte meiosis and gastric cancer. Most of these signaling pathways are involved in tumor initiation and progression, indicating that the CNV-driven genes are critical in the molecular mechanisms of HCC development.
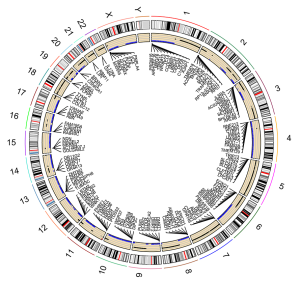
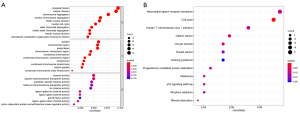
Screening of prognostic CNV-driven genes associated with survival
Wilcoxon rank-sum test was used to analyze the difference of the 568 CNV-driven genes between tumor and non-tumor tissues (FDR <0.05 and |log2 [FC]| >1), and 373 differentially expressed CNV-driven genes were finally selected (Table S2). Of the 373 CNV-driven genes, 63 CNV-driven genes were identified as potential prognostic biomarkers for OS after univariate analysis (P<0.0001, Table S3).
Generating and evaluating the HCC prognosis prediction model
The 63 selected CNV-driven genes were analyzed by LASSO regression method. Eight genes appeared 800 times of a total of 1000 repetitions and were detected as prognostic genes for building risk score (Figure 3A,B). Then, using the coefficients from multivariate Cox regression, we built a model based on the eight CNV-driven genes (Table S4). Hazard ratios of all the eight CNV-driven genes were greater than 1, indicating these genes were associated with shorter OS of HCC patients. Based on the optimal cutoff value of 2.43 for risk score, the patients were grouped into two subgroups with high- and low-risks respectively. Patients with high-risk scores had significantly shorter OS (HR =6.14, 95% CI: 2.72–13.86, P<0.001) than patients in low-risk group (Figure 4A). The risk score distributions and expression of CNV-driven genes were plotted (Figure 4B,C,D). Expression levels for the eight CNV-driven genes increased as risk scores, indicating these CNV-driven genes were high risk factors for OS. The area under the ROC curve (AUC) curve for the 3-year OS was 0.704 (Figure S1A). The risk prognosis model was validated using external independent data from ICGC datasets. HCC patients in validating cohort were designated into high- and low-risk groups using the same risk score formula and cutoff obtained from the TCGA group. Compared to the low-risk group, high-risk group showed significantly poorer OS (HR =3.23, 95% CI: 1.17–8.92, P<0.001) (Figure 4E). The risk score distribution, vital statuses of patients, and expression levels of CNV-driven genes were shown in Figure 4F,G,H. The AUC of the 3-year OS was 0.768 for HCC patient in ICGC dataset (Figure S1B).
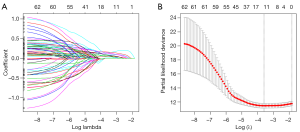
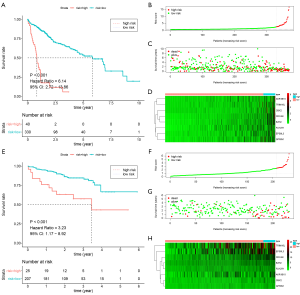
Independent of the prognostic model from other clinical features in TCGA and ICGC
Univariate and multivariate Cox proportional-hazards model were used to determine whether the risk score prognostic model was independent of clinical and pathological parameters (Tables 1,2). Among 235 patients in TCGA datasets, univariate analyses indicated that T category (primary tumor), TNM stage, and risk score were significantly correlated with OS (P<0.001). Multivariate analysis showed that the CNV-driven genes prognostic risk score was the only significant independent predictor for OS (P<0.001). Among 232 patients in ICGC, univariate analysis showed that risk score (P<0.001) and TNM stage (P<0.001) were related with OS, and further multivariate analysis showed risk score was still a predictor for OS independent of TNM stage (P<0.001).
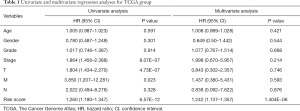
Full table
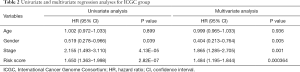
Full table
Analysis of the tumor-infiltrating cells and immune genes with the CNV-driven risk signature
Tumor-infiltrating cells play critical roles in tumor immune balance and associate with cancer development. To explore whether the CNV-driven genes prognostic model was associated tumor-infiltrating cells, we analyzed the relationship between risk score and six immune cell subsets. Pearson correlation tests showed that the abundance of CD8+ T cells, dendritic cells, neutrophils and macrophages were positively correlated with risk scores (P<0.05, Figure 5). Of note, the Pearson correlation coefficient is largest in correlation between neutrophils and risk score, and the weakest correlation is observed between CD8+ T cells and risk score. There were no relations between risk score and B cells or CD4+ T cells. These results indicate that increased infiltrating neutrophils are associated with poorer survival and play a negative role in HCC immune balance. We further assessed the critical immune checkpoint genes expression between patients in high- and low-risk groups. As shown in Figure 6, high-risk cohort had higher expression levels of CTLA4, TIM-3, LAG3 and CD39 compared to those in the low-risk cohort (P<0.05). These results suggest that high-risk patients had higher immunoinhibitory gene expression, and may benefit from immunotherapy based on immune checkpoint inhibitors.
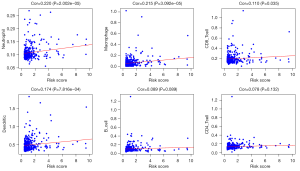
Discussion
HCC remains a major cause of cancer-related deaths in the world, causing one of the highest public health burdens (19,20). Advancement in molecular analyses has facilitated deep understanding of the HCC mutation landscape and characteristics. Studies indicated that hepatocarcinogenesis was a multistep and multifactorial process caused by frequent aberrant gene alterations, including single nucleotide mutations and CNVs (21). Therefore, understanding the roles of CNVs in driving hepatocarcinogenesis is crucial for HCC prevention, treatment, and prognosis prediction.
Integrated genomic analysis can be an effective and essential method for identification of novel cancer driver genes. For instance, the widespread use of high-throughput sequencing has enabled more efficient and comprehensive analysis of CNVs, and provides opportunities for revealing new genes underlying the development of HCC (22). CNVs in oncogenes and tumor suppressor genes are involved in HCC malignant proliferation and transformation. Previous studies on HCC showed that oncogenic driver genes CCND1 and FGF19 had increased amplifications of copy numbers (23), while tumor suppressor genes CDKN2A and CDKN2B contained high frequency of deletions (12). Analysis of recurrent CNVs can also help to identify potential novel biomarkers such as IRF2, which is unique to hepatitis virus B-related HCC (24).
In this work, we conducted an integrative analysis of CNVs and gene expression profiles aiming to identify CNV-driven genes that associated HCC survival, and built a prognostic signature with CNV-driven genes. In multivariate Cox proportional-hazards analysis, the prognostic risk score proved to be an independent predictor for OS. Survival analysis showed the risk score prediction model had robust distinguishing ability, and might help to improve individualized prediction of OS in HCC patients.
Recent research results have highlighted the roles of tumor-infiltrating immune cells in HCC immune tolerance and survival prognosis (25,26). Since CNVs can result in alterations of key genes responsible for cancer immune surveillance, we therefore investigated the associations between tumor-infiltrating immune cells, immune checkpoint genes, and the risk score. The results showed there was the strongest positive correlation between neutrophils and risk score, indicating increased infiltrating neutrophils were risk factor for survival. Previous studies have demonstrated that neutrophil activation could drive tumor progression and metastasis (27), and infiltrating neutrophils contributed to HCC progression, and associated with poor prognosis (28). These studies were consistent with our findings and implied that our risk prognostic signature was closely related with tumor immune microenvironment. Moreover, we explored the expression of immune checkpoint genes that had been proved critical in HCC. Based on the risk score, the gene expression of CTLA-4, TIM-3, LAG3, and CD39 were significantly higher in patients with high-risk. The high expression of immune checkpoint genes may be responsible for poorer survival of high-risk group. The prognostic signature can be utilized to identify high-risk populations who may benefit from cancer immunotherapy such as immune checkpoint inhibitors.
Our study has some limitations. Since the data is retrospective, the results need to be further confirmed in prospective studies. Moreover, the tumor-infiltrating immune cells and related genes expression remain to be further validated by experimental methods.
Conclusions
In summary, our study identified CNV-driven genes that related to HCC survival. A prognostic prediction model was established based on CNV-driven genes. Further analyses indicated that tumor-infiltrating immune cells and altered immune checkpoint genes might account for the model’s prognostic capacity. These results contribute to the understanding of hepatocarcinogenesis from view of CNVs, and may improve outcome prediction for patients with HCC.
Acknowledgments
We thank Yu Lin (Statistician, Shenzhen Withsum Technology Limited) for assistance with the data interpretation.
Funding: This work was supported by the International Science and Technology Cooperation Projects, No. 2016YFE0107100; Capital Special Research Project for Health Development, No. 2014-2-4012; Beijing Natural Science Foundation, No. L172055 and No. 7192158; National Ten-thousand Talent Program, the Fundamental Research Funds for the Central Universities, No. 3332018032; and CAMS Innovation Fund for Medical Science (CIFMS), No. 2017-I2M-4-003 and No. 2018-I2M-3-001.
Footnote
Reporting Checklist: The authors have completed the TRIPOD reporting checklist. Available at http://dx.doi.org/10.21037/atm-20-7101
Conflicts of Interest: All authors have completed the ICMJE uniform disclosure form (available at http://dx.doi.org/10.21037/atm-20-7101). The authors have no conflicts of interest to declare.
Ethical Statement: The authors are accountable for all aspects of the work in ensuring that questions related to the accuracy or integrity of any part of the work are appropriately investigated and resolved. The study was conducted in accordance with the Declaration of Helsinki (as revised in 2013). The data used in the current study are obtained from The Cancer Genome Atlas database (TCGA) and the International Cancer Genome Consortium (ICGC), which are open to the public under some guidelines. Therefore, it is confirmed that all written informed consent was achieved and no ethical approval was needed.
Open Access Statement: This is an Open Access article distributed in accordance with the Creative Commons Attribution-NonCommercial-NoDerivs 4.0 International License (CC BY-NC-ND 4.0), which permits the non-commercial replication and distribution of the article with the strict proviso that no changes or edits are made and the original work is properly cited (including links to both the formal publication through the relevant DOI and the license). See: https://creativecommons.org/licenses/by-nc-nd/4.0/.
References
- Llovet JM, Zucman-Rossi J, Pikarsky E, et al. Hepatocellular carcinoma. Nat Rev Dis Primers 2016;2:16018. [Crossref] [PubMed]
- Villanueva A. Hepatocellular Carcinoma. N Engl J Med 2019;380:1450-62. [Crossref] [PubMed]
- Li ZL, Han J, Liu K, et al. Association of family history with long-term prognosis in patients undergoing liver resection of HBV-related hepatocellular carcinoma. Hepatobiliary Surg Nutr 2019;8:88-100. [Crossref] [PubMed]
- Khalaf N, Ying J, Mittal S, et al. Natural History of Untreated Hepatocellular Carcinoma in a US Cohort and the Role of Cancer Surveillance. Clin Gastroenterol Hepatol 2017;15:273-81.e1. [Crossref] [PubMed]
- Sia D, Villanueva A, Friedman SL, et al. Liver Cancer Cell of Origin, Molecular Class, and Effects on Patient Prognosis. Gastroenterology 2017;152:745-61. [Crossref] [PubMed]
- Sebat J, Lakshmi B, Troge J, et al. Large-scale copy number polymorphism in the human genome. Science 2004;305:525-8. [Crossref] [PubMed]
- Nik-Zainal S, Davies H, Staaf J, et al. Landscape of somatic mutations in 560 breast cancer whole-genome sequences. Nature 2016;534:47-54. [Crossref] [PubMed]
- Hoang PH, Dobbins SE, Cornish AJ, et al. Whole-genome sequencing of multiple myeloma reveals oncogenic pathways are targeted somatically through multiple mechanisms. Leukemia 2018;32:2459-70. [Crossref] [PubMed]
- Liu W, Sun J, Li G, et al. Association of a germ-line copy number variation at 2p24.3 and risk for aggressive prostate cancer. Cancer Res 2009;69:2176-9. [Crossref] [PubMed]
- Diskin SJ, Hou C, Glessner JT, et al. Copy number variation at 1q21.1 associated with neuroblastoma. Nature 2009;459:987-91. [Crossref] [PubMed]
- Park RW, Kim TM, Kasif S, et al. Identification of rare germline copy number variations over-represented in five human cancer types. Mol Cancer 2015;14:25. [Crossref] [PubMed]
- Wang K, Lim HY, Shi S, et al. Genomic landscape of copy number aberrations enables the identification of oncogenic drivers in hepatocellular carcinoma. Hepatology 2013;58:706-17. [Crossref] [PubMed]
- Hou Y, Guo H, Cao C, et al. Single-cell triple omics sequencing reveals genetic, epigenetic, and transcriptomic heterogeneity in hepatocellular carcinomas. Cell Res 2016;26:304-19. [Crossref] [PubMed]
- Robinson MD, McCarthy DJ, Smyth GK. edgeR: a Bioconductor package for differential expression analysis of digital gene expression data. Bioinformatics 2010;26:139-40. [Crossref] [PubMed]
- Tibshirani R. The lasso method for variable selection in the Cox model. Stat Med 1997;16:385-95. [Crossref] [PubMed]
- Camp RL, Dolled-Filhart M, Rimm DL. X-tile: a new bio-informatics tool for biomarker assessment and outcome-based cut-point optimization. Clin Cancer Res 2004;10:7252-9. [Crossref] [PubMed]
- Yu G, Wang LG, Han Y, et al. clusterProfiler: an R package for comparing biological themes among gene clusters. Omics 2012;16:284-7. [Crossref] [PubMed]
- Li T, Fan J, Wang B, et al. TIMER: A Web Server for Comprehensive Analysis of Tumor-Infiltrating Immune Cells. Cancer Res 2017;77:e108-10. [Crossref] [PubMed]
- Bray F, Ferlay J, Soerjomataram I, et al. Global cancer statistics 2018: GLOBOCAN estimates of incidence and mortality worldwide for 36 cancers in 185 countries. CA Cancer J Clin 2018;68:394-424. [Crossref] [PubMed]
- Xie DY, Ren ZG, Zhou J, et al. 2019 Chinese clinical guidelines for the management of hepatocellular carcinoma: updates and insights. Hepatobiliary Surg Nutr 2020;9:452-63. [Crossref] [PubMed]
- Niu ZS, Niu XJ, Wang WH. Genetic alterations in hepatocellular carcinoma: An update. World J Gastroenterol 2016;22:9069-95. [Crossref] [PubMed]
- Chen CF, Hsu EC, Lin KT, et al. Overlapping high-resolution copy number alterations in cancer genomes identified putative cancer genes in hepatocellular carcinoma. Hepatology 2010;52:1690-701. [Crossref] [PubMed]
- Sawey ET, Chanrion M, Cai C, et al. Identification of a therapeutic strategy targeting amplified FGF19 in liver cancer by Oncogenomic screening. Cancer Cell 2011;19:347-58. [Crossref] [PubMed]
- Guichard C, Amaddeo G, Imbeaud S, et al. Integrated analysis of somatic mutations and focal copy-number changes identifies key genes and pathways in hepatocellular carcinoma. Nat Genet 2012;44:694-8. [Crossref] [PubMed]
- Zheng C, Zheng L, Yoo JK, et al. Landscape of Infiltrating T Cells in Liver Cancer Revealed by Single-Cell Sequencing. Cell 2017;169:1342-56.e16. [Crossref] [PubMed]
- Zhang Z, Ma L, Goswami S, et al. Landscape of infiltrating B cells and their clinical significance in human hepatocellular carcinoma. Oncoimmunology 2019;8:e1571388 [Crossref] [PubMed]
- Singel KL, Segal BH. Neutrophils in the tumor microenvironment: trying to heal the wound that cannot heal. Immunol Rev 2016;273:329-43. [Crossref] [PubMed]
- Zhou SL, Yin D, Hu ZQ, et al. A Positive Feedback Loop Between Cancer Stem-Like Cells and Tumor-Associated Neutrophils Controls Hepatocellular Carcinoma Progression. Hepatology 2019;70:1214-30. [Crossref] [PubMed]