Screening and identification of potential protein biomarkers for the early diagnosis of acute myocardial infarction
Introduction
Coronary artery disease (CAD) is caused by atherosclerosis, often leading to serious cardiac events. If CAD patients are not treated promptly, complete occlusion of the coronary artery may occur, leading to permanent damage (myocardial infarction) (1,2). Acute myocardial infarction (AMI) is one of the most serious types of CAD. In CAD, coronary blood flow is drastically reduced or interrupted, causing severe myocardial ischemia or necrosis (3). AMI is caused by the rupture of an unstable coronary-artery plaque and often occurs due to coronary atherosclerosis stenosis. Unstable plaque caused by coronary atherosclerosis often leads to local erosion and rupture and results in platelet aggregation, thrombus formation, and blockage of the coronary arteries. This ultimately results in myocardial ischemia and hypoxia, and eventually, AMI.
Moreover, AMI, in turn, further accelerates atherosclerosis (4,5). AMI could cause severe complications, such as congestive heart failure and malignant arrhythmia, with high rates of morbidity and mortality (6,7). Timely diagnosis and treatment of AMI can reduce the mortality rate and may improve the prognosis of patients.
At present, the diagnosis of AMI is based on chest pain accompanied by dynamic changes on electrocardiogram (ECG) and protein biomarkers. However, ECG lacks high sensitivity for the detection of AMI. Some patients have no typical clinical manifestations or specific ECG changes in AMI’s early stages (8). Cardiac troponin I (TNI), myoglobin (Mb), and creatine kinase MB (CK-MB) are the most commonly used biomarkers for AMI clinical diagnosis. Among them, cardiac troponin is a highly specific biomarker for the diagnosis of AMI (1). However, the sensitivity is not good in the first few AMI hours, and false-positive values may occur in severe heart failure, arrhythmia, and myocarditis (1). MB can be detected within 3 hours after AMI with good sensitivity. However, the specificity is limited. MB can also be detected in skeletal muscles, and abnormally increased MB levels can be found in diseases such as striated muscle injury (1). CK-MB is the most widely used biomarker for the diagnosis of AMI. However, the sensitivity of CK-MB is poor within 6 hours of onset, and false positive results can occur in skeletal muscle diseases, affecting the specificity of CK-MB (1,9). Also, a single protein serving as a diagnostic biomarker for the disease is not specific enough.
In some cases, it is difficult to distinguish between AMI patients and stable angina pectoris (SAP) patients (10). The clinical symptoms of AMI and SAP are similar. Both of the diseases occur during exertion or agitation and have the symptoms such as chest pain, precordial pain, and feelings of impending death. Chest pain can be manifested as compression pain and oppression. It is difficult to distinguish AMI and SAP by clinical symptoms alone, and it is even more difficult for patients with atypical symptoms. Since SAP patients are often compared and analyzed with AMI patients in studies examining potential biomarkers for the early diagnosis of AMI, SAP and were also selected for comparison with AMI in this study (11). Xu et al. (12) identified several novel AMI biomarker candidates by (iTRAQ)-based quantitative proteomic approach. However, the sample size was too small, the differential diagnosis group (patients with similar symptoms with AMI patients) was lacking, and the differentially expressed proteins were not verified by another protein detection method in a greater number of patients. Therefore, early diagnosis is still the main principle for the treatment of AMI.
In this study, iTRAQ-2DLC-MS/MS was used to screen differentially expressed proteins in the serum of 10 AMI patients (onset time ≤12 h), 10 SAP patients, and 10 healthy controls. Potential biomarkers for the early diagnosis of AMI were validated and analyzed using bioinformatics methods and enzyme linked immunosorbent assay (ELISA) in 30 AMI patients, 30 healthy controls, and 30 SAP patients. All patients’ clinical data were analyzed, as was the relationship between the clinical data and the candidate proteins. Finally, diagnostic models were established. We present the following article in accordance with the STARD reporting checklist (available at http://dx.doi.org/10.21037/atm-20-7891).
Methods
Patients and control subjects
The workflow of this study is illustrated in Figure 1. A total of 90 subjects were included. All of the AMI patients (aged 41–92 years, N=30), SAP patients (aged 30–82 years, N=30), and healthy controls (aged 43–83 years, N=30) were recruited from the Zhejiang hospital (Hangzhou, Zhejiang, China) between January 2014 and December 2016. Patients were diagnosed according to the guidelines of the American Heart Association/American College of Cardiology Foundation (13-15). AMI patients were diagnosed using the changes in myocardial necrotic proteins and met one of the following criteria: (I) showing clear ischemic symptoms; (II) showing dynamic ECG changes with pathological Q wave; (III) showing new ST-T changes or onset of left bundle branch block on ECG; or (IV) showing segmental wall motion disorder on imaging, or with loss of viable myocardium (13,14). Patients with SAP were also diagnosed according to the American Heart Association/American College of Cardiology Foundation guidelines (15,16). Patients with hepatitis B, tuberculosis, diabetes, AIDS, cancer, and immunosuppressive drug users were excluded. Fasting blood samples were collected in the morning from all subjects. 5.0 mL peripheral blood were collected and centrifuged at 956 g at 4 °C for 10 min to separate the serum.
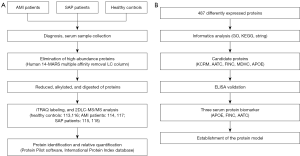
This study was performed following the Declaration of Helsinki (as revised in 2013). The Ethics Committee approved this study of Zhejiang University (China) (No. 2015007). Written informed consent was obtained from all participants.
iTRAQ 2D LC-MS/MS
There were three experimental groups in this study: AMI patients, SAP patients, and healthy controls. Ten serum samples from each group were randomly selected and divided into two biological replicates, and then the serum samples of each biological replicate were pooled in equal amounts, respectively. Each serum mixture’s high-abundance proteins were removed by using a Human 14-Multiple Affinity Removal System (MARS) multiple affinity removal liquid chromatography (LC) column (Agilent Technologies, Santa Clara, CA, USA). The total protein in each group was determined by the Bradford protein assay; 100 µg protein samples from each sample were then reduced, alkylated, digested with trypsin, and labeled with the iTRAQ-isotope (17,18). Experimental groups were labeled as follows: healthy controls, 113, 116; AMI patients, 114, 117; and SAP patients, 115, 118. The labeled proteins were pooled and desalted with Sep-Pak Vac C18 (Waters, Milford, MA, USA) and dried by rotation vacuum concentrator (Christ RVC 2–25, Christ, Osterode, Germany).
Mixed peptides were separated using the strong cation exchange LC column (strong cation exchange, SCX, 2.1 mm × 100 mm, 5 µm, 200 A, Polysulfoethyl column) (Nest Group, Southborough, MA, USA) by applying a 20AD LC system (SHIMADZU, Kyoto, Japan). Subsequently, peptides were loaded into the column and eluted stepwise with buffer A (25% acetonitrile, 10 mM KH2PO4, pH 2.6) at a flow rate of 200 µL/min, and a linear gradient (0–80%) of buffer B (25% acetonitrile, 10 mM KH2PO4, 350 mM KCL, pH 2.6). Twenty fractions were collected from the SCX column and were then injected into the ZORBAX 300SB-C18 reverse-phase (RP) column (5 µm, 300Å, 0.1×150 mm, Microm, USA). The flow rate of buffer A (5% CAN, 0.1% formic acid) in the RP separation was 300 nL/min, and elution was performed with a linear gradient of buffer B (95% acetonitrile ACN, 0.1% formic acid) over 90 min. Peptides were analyzed using the Triple TOF 5600 system (Applied Biosystems, USA) with the information-dependent acquisition mode (IDA). In the IDA mode, the mass-spectrometer (MS) range was 400–1,500 m/z, and up to 20 most-intense multiply charged ions were sequentially selected for production analysis with a mass range of 100–2,000 m/z. All samples were performed and analyzed in duplicate (19,20).
Protein identification and relative quantification
Protein Pilot software 4.2 beta (ABI, USA) and the International Protein Index database (version 3.87, HU-MAN) were used to search and identify proteins. The ProGroup algorithm of the Protein Pilot software was applied to eliminate redundant hits and generate the minimal number of identified proteins (21). Meanwhile, to minimize false positives, more than two peptides with a 95% confidence interval with unused ProtScore ≥1.3 were set as cutoff values during protein identification. The error factor and P value were calculated using ProteinPilot software, and the data was considered reliable at P value <0.05 and error factor <2. Proteins with fold changes ratio <0.50 (down-regulated) or >1.60 (up-regulated) were selected.
Informatics analysis
The functional distribution of differentially expressed proteins was analyzed by Gene Ontology (GO), including molecular function, cellular component, and biological process. The signaling pathway enrichment of differentially expressed proteins was acquired by searching against the Kyoto Encyclopedia of Genes and Genomes (KEGG) database. The functional interaction between proteins was analyzed with STRING software (http://string-db.org/), and functions were also searched through the UniportKB database.
ELISA analysis
Human Apolipoprotein E ELISA (APOE) Kit (Abcam, Cambridge, MA, USA; SwissProt: P02649; dilution factor: 1:400), Human Fibronectin (FINC) ELISA Kit (Abcam, Cambridge, MA, USA; SwissProt: P02751; dilution factor: 1:16,000), and Human aspartate aminotransferase (AATC) ELISA Kit (CUSABIO Biotech, Wuhan, Hubei, China; SwissProt: P17174; dilution factor: 1:10) were used to test the concentrations of proteins in different subjects (N=90; 30 AMI patients, 30 SAP patients, and 30 healthy controls).
ELISA assay was performed in duplicate according to the manufacturer’s instructions. The protein concentration was calculated by applying a four-parameter logistic curve of Microplate Manager 6 software (Bio-Rad, Inc., Hercules, CA, USA).
Statistical analysis
GraphPad Prism 5.0 (GraphPad Software, Inc., La Jolla, CA USA) was applied for statistical analysis. Parametric data were presented as mean ± standard deviation (SD) and were analyzed by t-test for the means and chi-square test for the composition ratios. P<0.05 was considered statistically significant. Nonparametric data were presented as median ± interquartile range (IQR) and were analyzed by the Mann-Whitney U test (for two groups) and Kruskal-Wallis H test (for three or more groups). Spearman correlation between candidate proteins and clinical indices were also performed using SPSS software. Receiver operating characteristic curve (ROC) curves were analyzed by MedCalc 11.4 software (Version 12.3.0, Belgium), and the area under the curve (AUC) value and the logistic regression equation were also generated.
Results
Protein identification and relative quantification
A total of 109 proteins were identified to be differentially expressed in the AMI group than the healthy control group, including 81 up-regulated and 28 down-regulated differentially expressed proteins. A total of 115 proteins were found to be differentially expressed in the AMI group than the SAP group, including 74 up-regulated and 41 down-regulated differentially expressed proteins. The results also showed that, compared with the healthy control and SAP groups, there were 39 differentially expressed proteins in the AMI group. Among them, 29 differentially expressed proteins were up-regulated (Table 1), and 10 differentially expressed proteins were down-regulated (Table 2).
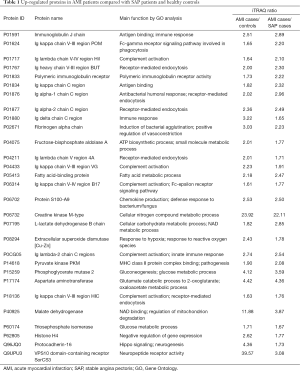
Full table
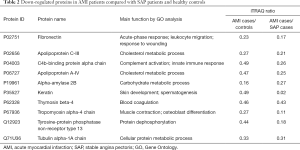
Full table
Bioinformatics analysis
GO functional annotation analysis showed that most of the differentially expressed proteins were involved in the biological process (32, 5.09%), cellular process (26, 4.13%), and biological regulation (25, 3.97%). Proteins related to the cellular component were distributed in the cell (40, 6.36%), extracellular region (36, 5.72%), and organelles (34, 5.41%). Proteins related to the molecular function were involved in binding (32, 5.09%), catalytic activity (20, 3.18%), and enzyme regulation activity (7, 1.11%) (Figure 2A). KEGG analysis showed that most proteins were involved in the glycolysis/gluconeogenesis metabolic pathway (7, 13.73%), the pyruvate metabolism pathway (3, 5.88%), and regulation of the actin cytoskeleton cellular pathway (3, 5.88%) (Figure 2B). Protein-protein functional interactions were also observed among all differentially expressed proteins (Figure S1). FINC, creatine kinase M-type (KCRM), AATC, and malate dehydrogenase (MDHC) were selected as candidate proteins, combined with the functions of differential proteins and their fold changes between the experimental groups. APOE was associated with the disease functionally and was also listed as a candidate protein, although the difference in APOE level was not significant enough between the experimental groups.
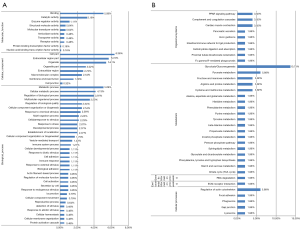
Verification of differentially expressed proteins by ELISA
Differentially expressed proteins were validated in AMI patients (N=30), SAP patients (N=30) and healthy controls (N=30). The results showed that the serum levels of APOE protein (81.17±46.86 µg/mL) were significantly higher in AMI patients than in healthy controls (57.97±22.56 µg/mL) (P=0.0172, Figure 3A). The serum levels of AATC protein in AMI patients (11,760.03±17,780.05 mU/mL) were markedly higher than those in healthy controls (495.36±1,728.08 mU/mL) and SAP patients (562.06±841.30 mU/mL) (P<0.0001 and P<0.0001, respectively, Figure 3B). The serum levels of FINC in AMI patients (41.93±31.41 ng/mL) were notably lower than those in SAP patients (68.55±35.33 ng/mL) (P=0.0044). In addition, the FINC levels in SAP patients were also considerably higher than those in healthy controls (44.84±25.66 ng/mL) (P=0.0043, Figure 3C).
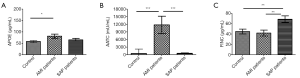
AMI patients were divided into different groups according to their clinical characteristics, including age, gender, ST-segment elevation, the position of lesion vessel (left main coronary artery, anterior descending artery, right coronary artery, or left circumflex artery), and the serum levels of APOE, AATC, and FINC were analyzed. The results showed that the serum levels of FINC in AMI patients were significantly higher in those aged <65 years (92.31±61.78 µg/mL) than in those aged ≥65 years (70.83±25.10 µg/mL) (P=0.047, Table S1). The serum levels of APOE protein in AMI patients with anterior descending artery lesion (73.84±37.11) were markedly lower than those in patients with non-anterior descending artery lesion (123.30±78.46) (P=0.041, Table S1).
Clinical data analysis
There were no significant differences in the general characteristics, such as age, gender, body mass index (BMI), smoking, and drinking, between AMI patients (N=30), SAP patients (N=30), and healthy controls (N=30). A comparison of clinical indicators, including blood glucose, glycosylated hemoglobin (HbA1c), triglyceride (TG), total serum cholesterol (TC), high-density lipoprotein (HDL), low-density lipoprotein (LDL), creatinine (cRE), white blood cells (WBC), platelet, C-reactive protein (CRP), hemoglobin, alanine aminotransferase (ALT), AST, troponin I (TNI), CK, CK-MB, lactate dehydrogenase (LDH), systolic blood pressure (SBP), diastolic blood pressure (DBP), and heart rate was performed in each experimental group. The results showed that the values of glucose (P<0.0001), HbA1c (P=0.003), cRE (P=0.002), WBC (P<0.0001), CRP (P<0.0001), ALT (P<0.0001), AST (P<0.0001), TNI (P<0.0001), CK (P<0.0001), CK-MB (P<0.0001), and LDH (P<0.0001) in AMI patients were significantly higher than those in the healthy controls. Moreover, the values of glucose (P<0.0001), WBC (P<0.0001), CRP (P=0.032), ALT (P<0.0001), AST (P<0.0001), TNI (P<0.0001), CK (P<0.0001), CK-MB (P<0.0001), and LDH (P<0.0001) were notably higher in AMI patients than those in SAP patients. In addition, the value of TG in AMI patients was substantially higher than in SAP patients (P=0.018) (Table 3).
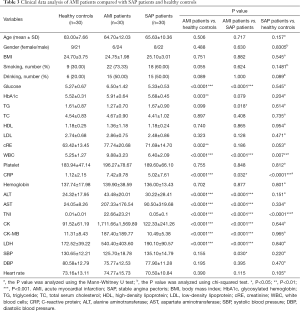
Full table
Spearman correlation analysis showed that in AMI patients, the level of APOE protein was positively correlated with HbA1c (r=0.397, P=0.040); the level of AATC protein was negatively correlated with HDL (r=−0.402, P=0.038); and the level of FINC protein was positively correlated with WBC and BMI (r=0.379, P=0.039; r=0.406, P=0.026) (Table 4).
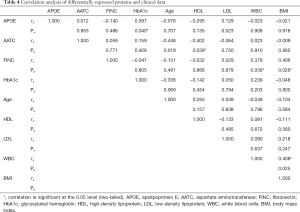
Full table
ROC analysis and decision tree establishment
APOE and AATC proteins were combined to discriminate between AMI patients and healthy controls, with an AUC value of 0.935, a sensitivity of 96.20%, and a specificity of 90.00%. The logistic regression equation was as follows: Logit (p) =−2.945+0.026*(APOE)+0.0006*(AATC). Also, AATC and FINC proteins were combined to discriminate between AMI and SAP patients, with an AUC value of 0.908, a sensitivity of 85.20%, and a specificity of 79.30%. The logistic regression equation was as follows: Logit (p) =0.177−0.001*(AATC)+0.030*(FINC).
Logistic regression analysis results showed that when AATC was combined with LDH and CK to establish a diagnostic model to discriminate between AMI patients and healthy controls, the AUC value was 0.993, and the sensitivity and specificity values were 96.20% and 96.30%, respectively. The logistic regression equation was as follows: Logit (p) =1.548+0.0004*(AATC)−0.049*(LDH)+0.026*(CK). The results also showed that when AATC and CK were combined to establish a diagnostic model to discriminate between AMI patients and SAP patients, the AUC value was 0.975, and the sensitivity and specificity values were 85.20% and 79.30%, respectively. The logistic regression equation was as follows: Logit (p) =−3.322+0.0009*(AATC)+0.0041*(CK). The ROC curve is shown in Figure 4.
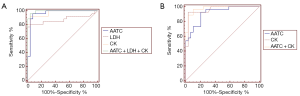
Discussion
AMI is a cardiovascular disease with high morbidity and mortality, which could pose a significant threat to patients’ health with impaired function of the heart (22-24). Therefore, early and accurate diagnosis of AMI is critical. In this study, several differentially expressed serum proteins were identified in patients with AMI by applying iTRAQ-2DLC-MS/MS and ELISA. Patients’ clinical data were also analyzed, and differentially expressed proteins were combined with clinical indicators to construct diagnostic models for AMI.
Several novel AMI protein biomarker candidates have been screened in plasma (12). However, clinically, diagnostic biomarkers for detecting AMI are usually tested in the serum, the separated supernatant after blood coagulation, while plasma is the separated supernatant after blood anticoagulation anticoagulant. Coagulation factors (such as coagulation factors I, II, V, etc.) lack the serum. Thrombosis occurs during the formation and development of AMI. For example, a thrombus could form based on coronary atherosclerosis and could also form as a result of AMI due to persistent coronary artery spasm. When screening the diagnostic biomarkers for AMI, it is important to exclude the influence of coagulation factors and reflect myocardial damage to the greatest extent. Therefore, it is better to use a serum to screen candidate protein biomarkers (25,26).
The clinical analysis demonstrated that the levels of glucose, WBC, CRP, ALT, AST, TNI, CK, CK-MB, and LDH in AMI patients were significantly higher than those in healthy controls and SAP patients. It has been reported that glucose metabolism in AMI patients can induce hyperglycemia (27). The increased blood glucose concentration in AMI patients is associated with the risk of left ventricular failure and death (27,28). Myocardial infarction is an ischemic wound that can recruit many WBC from the blood circulation (29). The elevated WBC levels can be used to predict the progression of AMI (30,31). Therefore, increased blood glucose and WBC levels may be associated with an increased risk of AMI. The increased CRP protein level is associated with the activation of the complement system and endothelial dysfunction in AMI (32). Studies have shown that ALT and AST levels can be elevated in AMI patients with ST-segment elevation (33). ALT and AST are biomarkers of hepatocyte injury, which is caused by a sharp decrease in blood pump induced by pump failure or recessive arrhythmia (34). TNI, CK, CK-MB, and LDH are increased in AMI. However, TNI and CK levels can reach a peak after 6–24 h of cardiac injury (35). CK-MB can increase 4–6 h after AMI and reach a peak within 24 h (1). LDH is a glycolysis metabolic enzyme, and the levels of LDH could elevate in myocardial infarction patients (36). The proteins mentioned above have been demonstrated to serve as single protein biomarkers in the diagnosis of AMI. However, a single biomarker’s sensitivity and specificity in diagnosing the disease can be limited, and the combination of multiple biomarkers is often more accurate (37,38).
In this study, the differentially expressed proteins in AMI patients, such as KCRM, AATC, and FINC, were mainly concentrated in the function of metabolic processes, catalytic activities, and enzyme regulatory activities. This indicates that the serum levels of proteins involved in the function of metabolism, catalysis, and enzyme-modulating activity may change within 12 h from the onset of AMI. Also, pathway enrichment analysis showed that differentially expressed proteins in AMI patients were mainly concentrated in metabolic pathways, including glycolysis/gluconeogenesis metabolism and pyruvate metabolism. This indicates that the serum levels of proteins linked to metabolic pathways may change within 12 h from the onset of AMI. The results of iTRAQ 2D LC-MS/MS analysis showed that the levels of CK-MB protein in AMI patients were significantly higher than those in healthy controls and SAP patients, which is consistent with the results of CK-MB in AMI. We also found that the serum levels of MDHC in AMI patients were markedly higher than those in healthy controls and SAP patients. Gopcevic et al. (39) found that serum dehydrogenase activity could increase significantly in AMI patients than in healthy controls. These findings were consistent with the results of the present study. Also, the levels of APOE in AMI patients were notably higher than those in healthy controls, the levels of AATC in AMI patients were considerably higher than those in healthy controls and SAP patients, and the levels of FINC in AMI patients were significantly lower than those in SAP patients.
APOE, also known as apolipoprotein E, is the main ligand for the clearance of LDL receptors and chylomicron residues, affecting the occurrence of CAD (40,41). APOE gene polymorphism is closely related to the susceptibility and prognosis of CAD (42,43). Baum et al. (44) found that APOE gene polymorphism was associated with myocardial infarction and ischemic stroke risk. Johansson et al. (45) reported that the elevated serum level of APOE is an independent correlative factor for the occurrence of infarction among females. Zhou et al. (46) found that APOE deficiency in mice impaired infarct healing after myocardial infarction and pointed out that APOE protein could limit myocardial injury after myocardial infarction by regulating the formation of neutrophil extracellular traps. APOE protein is also thought to have a role in atherogenesis (47). In this study, the serum levels of APOE were markedly elevated in AMI patients; however, they were significantly lower in AMI patients with anterior descending branch lesions than in other AMI patients. This indicates that the serum content of APOE in AMI patients was abnormal, and the level of APOE may be associated with vascular lesions in the anterior descending branch. The increased APOE protein level may relate to the formation of infarction and the existence of atherogenesis in the patients. It has also been demonstrated that the level of APOE is positively correlated with the level of HbA1c in AMI patients, and the HbA1c levels were also notably higher in AMI patients than the healthy controls. The concentration of HbA1c is an important predictor of cardiac adverse reactions in non-diabetic AMI patients (48). All AMI patients included in this study were non-diabetic; although, the relationship between the concentration of APOE and the prognosis of the disease requires further study.
The activity of AATC, also known as aspartate aminotransferase, rises during myocardial injury and necrosis, and the serum level of AATC is one of the protein biomarkers for assessing myocardial damage (49). It has been reported that the increased content of AATC is positively correlated with the risk of early-onset and severity of CAD (50). It has also been demonstrated that the increased level of AATC is related to ST-segment elevation and vessel diameter stenosis in AMI patients (51). In this study, the serum levels of AATC in AMI patients were significantly higher than those in the healthy controls and SAP patients. There was no notable difference in the serum levels of AATC between SAP patients and healthy controls. The results indicated severe myocardial injury in AMI patients within 12 h after onset of AMI, while no such changes were observed in SAP patients. In this study, the results of AST were consistent with the results of AATC, which further confirms the experiment’s reliability. Also, there was a negative correlation between AATC and HDL in this experiment. HDL plays a role in cholesterol transporting and scavenging and has protective effects on blood vessels (52), which has predictive and protective effects in AMI patients (53). However, the results showed no significant difference in the HDL levels in AMI patients compared with those in the healthy controls and SAP patients, indicating that the protective effect of HDL in AMI patients may not be significant within 12 h after onset of the disease.
FINC, also known as fibronectin, is a high molecular weight glycoprotein found in body fluids and extracellular matrices and is mainly produced by endothelial cells. FINC is involved in many biological processes such as cell growth, hemostasis, adhesion, migration, and wound repair between the cell and its interstitial collagen network (54,55). It has been reported that the plasma level of FINC could increase considerably in CAD patients, and thus, FINC may serve as a biomarker for predicting CAD (55,56). The increased FINC level in CAD patients may be related to the disease’s lipid composition (56). In this study, the levels of FINC in SAP patients were markedly higher than those in healthy controls and AMI patients. However, there was no significant difference in the levels of FINC between AMI patients and healthy controls. Serebruany et al. (57) found that the plasma level of FINC increased significantly in a pig model of AMI. These results differed from our findings, which may be due to the differences between species. A significant positive correlation was also found between FINC and the value of WBC and BMI in AMI patients in this study. The abnormal WBC levels in AMI patients may be associated with the risk of acute complications in patients (30,31). Meanwhile, the abnormal elevation of BMI value in AMI patients may be associated with an acute risk of cardiovascular disease (58). However, in this study, no significant change was observed in the BMI value, which may be due to the short onset time.
The differentially expressed proteins (APOE, AATC, and FINC) were combined with clinical indicators by logistic regression analysis to establish diagnostic models. When AATC was combined with LDH and CK, the model could be used to discriminate between AMI patients and healthy controls, with an AUC value of 0.993 and a sensitivity and specificity of 96.2% and 96.3%, respectively. When AATC and CK were combined, the model could be used to discriminate between AMI patients and SAP patients, with an AUC value of 0.975 and a sensitivity and specificity of 85.2% and 79.30%, respectively. All patients included in this study were within 12 h from onset (59), and further research will be performed using animal models to explore the relationship between candidate proteins and disease time.
Conclusions
This study identified three differentially expressed proteins (APOE, AATC, and FINC) identified by iTRAQ-2D LC-MS/MS and ELISA. Protein models were constructed, and logistic regression equations were obtained by combining the clinical indicators. We found that the combination of AATC, LDH, and CK could discriminate between AMI patients and healthy controls, and the combination of AATC and CK could discriminate between AMI patients and SAP patients with a better diagnostic value. Therefore, our findings provide an experimental basis for the construction of early diagnostic models for AMI and can be of great significance for early diagnosis in AMI patients.
Acknowledgments
The authors thank all the blood donors in this study. We also thank Xiao-Wei Liu, Guang-Zhong Zeng, and Li-Jiang Tang at Zhejiang Hospital for the collection of clinical data in this study.
Funding: This work was supported by grants obtained from the National Natural Science Foundation of China (81772266), the Natural Science Foundation of Guangdong Province (2017A030311014), the Guangzhou Science and Technology Project (201804010369), and the Key Construction Foundation of Guangdong High-level Hospital. The funders had no role in the study design, data collection and analysis, decision to publish, or preparation of the manuscript.
Footnote
Reporting Checklist: The authors have completed the STARD reporting checklist. Available at http://dx.doi.org/10.21037/atm-20-7891
Data Sharing Statement: Available at http://dx.doi.org/10.21037/atm-20-7891
Conflicts of Interest: All authors have completed the ICMJE uniform disclosure form (available at http://dx.doi.org/10.21037/atm-20-7891). The authors have no conflicts of interest to declare.
Ethical Statement: The authors are accountable for all aspects of the work in ensuring that questions related to the accuracy or integrity of any part of the work are appropriately investigated and resolved. This study was conducted in accordance with Declaration of Helsinki (as revised in 2013). The study was approved by the Ethics Committee review board of Zhejiang University (China) (No. 2015007), and the written informed consent was obtained from all of the participants.
Open Access Statement: This is an Open Access article distributed in accordance with the Creative Commons Attribution-NonCommercial-NoDerivs 4.0 International License (CC BY-NC-ND 4.0), which permits the non-commercial replication and distribution of the article with the strict proviso that no changes or edits are made and the original work is properly cited (including links to both the formal publication through the relevant DOI and the license). See: https://creativecommons.org/licenses/by-nc-nd/4.0/.
References
- Ye XD, He Y, Wang S, et al. Heart-type fatty acid binding protein (H-FABP) as a biomarker for acute myocardial injury and long-term post-ischemic prognosis. Acta Pharmacol Sin 2018;39:1155-63. [Crossref] [PubMed]
- Guo W, Yang D, Wu D, et al. Hyperuricemia and long-term mortality in patients with acute myocardial infarction undergoing percutaneous coronary intervention. Ann Transl Med 2019;7:636. [Crossref] [PubMed]
- Gao F, Su Q, Yang W, et al. Functional variants in the LC3B gene promoter in acute myocardial infarction. J Cell Biochem 2018;119:7339-49. [Crossref] [PubMed]
- Wang H, Eitzman DT. Acute myocardial infarction leads to acceleration of atherosclerosis. Atherosclerosis 2013;229:18-22. [Crossref] [PubMed]
- Dutta P, Courties G, Wei Y, et al. Myocardial infarction accelerates atherosclerosis. Nature 2012;487:325-9. [Crossref] [PubMed]
- Nawaz W, Khan FU, Khan MZ, et al. Exo-organoplasty interventions: A brief review of past, present and future directions for advance heart failure management. Biomed Pharmacother 2017;88:162-72. [Crossref] [PubMed]
- White HD, Chew DP. Acute myocardial infarction. Lancet 2008;372:570-84. [Crossref] [PubMed]
- Fathil MF, Md Arshad MK, Gopinath SC, et al. Diagnostics on acute myocardial infarction: Cardiac troponin biomarkers. Biosens Bioelectron 2015;70:209-20. [Crossref] [PubMed]
- Harris BM, Nageh T, Marsden JT, et al. Comparison of cardiac troponin T and I and CK-MB for the detection of minor myocardial damage during interventional cardiac procedures. Ann Clin Biochem 2000;37:764-9. [Crossref] [PubMed]
- Ding Y, Svingen GF, Pedersen ER, et al. Plasma Glycine and Risk of Acute Myocardial Infarction in Patients With Suspected Stable Angina Pectoris. J Am Heart Assoc 2015;5:e002621 [PubMed]
- Li P, Li SY, Liu M, et al. Value of the expression of miR-208, miR-494, miR-499 and miR-1303 in early diagnosis of acute myocardial infarction. Life Sci 2019;232:116547 [Crossref] [PubMed]
- Xu S, Jiang J, Zhang Y, et al. Discovery of potential plasma protein biomarkers for acute myocardial infarction via proteomics. J Thorac Dis 2019;11:3962-72. [Crossref] [PubMed]
- Fan L, Meng H, Guo X, et al. Differential gene expression profiles in peripheral blood in Northeast Chinese Han people with acute myocardial infarction. Genet Mol Biol 2018;41:59-66. [Crossref] [PubMed]
- O’Gara PT, Kushner FG, Ascheim DD, et al. 2013 ACCF/AHA guideline for the management of ST-elevation myocardial infarction: a report of the American College of Cardiology Foundation/American Heart Association Task Force on Practice Guidelines. J Am Coll Cardiol 2013;61:e78-e140. [Crossref] [PubMed]
- Fihn SD, Gardin JM, Abrams J, et al. 2012 ACCF/AHA/ACP/ AATS/PCNA/SCAI/STS Guideline for the diagnosis and management of patients with stable ischemic heart disease: a report of the American College of Cardiology Foundation/American Heart Association Task Force on Practice Guidelines, and the American College of Physicians, American Association for Thoracic Surgery, Preventive Cardiovascular Nurses Association, Society for Cardiovascular Angiography and Interventions, and Society of Thoracic Surgeons. J Am Coll Cardiol 2012;60:e44-e164. [Crossref] [PubMed]
- Cui Y, Song J, Li S, et al. Plasmatic MicroRNA Signatures in Elderly People with Stable and Unstable Angina. Int Heart J 2018;59:43-50. [Crossref] [PubMed]
- Jia J, Nie Y, Geng L, et al. Identification of HO-1 as a novel biomarker for graft acute cellular rejection and prognosis prediction after liver transplantation. Ann Transl Med 2020;8:221. [Crossref] [PubMed]
- Liu XX, Tang L, Ge R, et al. iTRAQ-based quantitative proteomic analysis of the anti-apoptotic effect of hyperin, which is mediated by Mcl-1 and Bid, in H2O2-injured EA. hy926 cells. Int J Mol Med 2016;37:1083-90. [Crossref] [PubMed]
- Xu DD, Deng DF, Li X, et al. Discovery and identification of serum potential biomarkers for pulmonary tuberculosis using iTRAQ-coupled two-dimensional LC-MS/MS. Proteomics 2014;14:322-31. [Crossref] [PubMed]
- Yang Y, Mu J, Chen G, et al. iTRAQ-based quantitative proteomic analysis of cerebrospinal fluid reveals NELL2 as a potential diagnostic biomarker of tuberculous meningitis. Int J Mol Med 2015;35:1323-32. [Crossref] [PubMed]
- Wang YS, Cao R, Jin H, et al. Altered protein expression in serum from endometrial hyperplasia and carcinoma patients. J Hematol Oncol 2011;4:15. [Crossref] [PubMed]
- Han Z, Zhang L, Yuan L, et al. Change of plasma microRNA-208 level in acute myocardial infarction patients and its clinical significance. Ann Transl Med 2015;3:307. [PubMed]
- Sanaani A, Yandrapalli S, Jolly G, et al. Correlation between electrocardiographic changes and coronary findings in patients with acute myocardial infarction and single-vessel disease. Ann Transl Med 2017;5:347. [Crossref] [PubMed]
- Xin Y, Yang C, Han Z. Circulating miR-499 as a potential biomarker for acute myocardial infarction. Ann Transl Med 2016;4:135. [Crossref] [PubMed]
- Finn AV, Nakano M, Narula J, et al. Concept of vulnerable/unstable plaque. Arterioscler Thromb Vasc Biol 2010;30:1282-92. [Crossref] [PubMed]
- Wang JC, Normand SL, Mauri L, et al. Coronary artery spatial distribution of acute myocardial infarction occlusions. Circulation 2004;110:278-84. [Crossref] [PubMed]
- Norhammar A, Tenerz A, Nilsson G, et al. Glucose metabolism in patients with acute myocardial infarction and no previous diagnosis of diabetes mellitus: a prospective study. Lancet 2002;359:2140-4. [Crossref] [PubMed]
- Bellodi G, Manicardi V, Malavasi V, et al. Hyperglycemia and prognosis of acute myocardial infarction in patients without diabetes mellitus. Am J Cardiol 1989;64:885-8. [Crossref] [PubMed]
- Sager HB, Heidt T, Hulsmans M, et al. Targeting Interleukin-1β Reduces Leukocyte Production After Acute Myocardial Infarction. Circulation 2015;132:1880-90. [Crossref] [PubMed]
- Zhu G, Yao Y, Pan L, et al. Reduction of Leukocyte Counts by Hydroxyurea Improves Cardiac Function in Rats with Acute Myocardial Infarction. Med Sci Monit 2015;21:3941-7. [Crossref] [PubMed]
- Betgem RP, de Waard GA, Nijveldt R, et al. Intramyocardial haemorrhage after acute myocardial infarction. Nat Rev Cardiol 2015;12:156-67. [Crossref] [PubMed]
- Fordjour PA, Wang Y, Shi Y, et al. Possible mechanisms of C-reactive protein mediated acute myocardial infarction. Eur J Pharmacol 2015;760:72-80. [Crossref] [PubMed]
- Moon J, Kang W, Oh PC, et al. Serum transaminase determined in the emergency room predicts outcomes in patients with acute ST-segment elevation myocardial infarction who undergo primary percutaneous coronary intervention. Int J Cardiol 2014;177:442-7. [Crossref] [PubMed]
- Kavoliuniene A, Vaitiekiene A, Cesnaite G. Congestive hepatopathy and hypoxic hepatitis in heart failure: a cardiologist's point of view. Int J Cardiol 2013;166:554-8. [Crossref] [PubMed]
- Kruse JM, Enghard P, Schröder T, et al. Weak diagnostic performance of troponin, creatine kinase and creatine kinase-MB to diagnose or exclude myocardial infarction after successful resuscitation. Int J Cardiol 2014;173:216-21. [Crossref] [PubMed]
- Mazhar F, Tariq SR, Bashir F. Age- and gender-based studies of trace metal levels and various enzymes associated with myocardial infarction. Biol Trace Elem Res 2011;140:139-50. [Crossref] [PubMed]
- Xu D, Li Y, Li X, et al. Serum protein S100A9, SOD3, and MMP9 as new diagnostic biomarkers for pulmonary tuberculosis by iTRAQ-coupled two-dimensional LC-MS/MS. Proteomics 2015;15:58-67. [Crossref] [PubMed]
- Jiang TT, Shi LY, Wei LL, et al. Serum amyloid A, protein Z, and C4b-binding protein β chain as new potential biomarkers for pulmonary tuberculosis. PLoS One 2017;12:e0173304 [Crossref] [PubMed]
- Gopcevic K, Rovcanin B, Kekic D, et al. Matrix metalloproteinases and membrane damage markers in sera of patients with acute myocardial infarction. Mol Cell Biochem 2011;350:163-8. [Crossref] [PubMed]
- Onat A, Can G, Ornek E, et al. High serum apolipoprotein E determines hypertriglyceridemic dyslipidemias, coronary disease and apoA-I dysfunctionality. Lipids 2013;48:51-61. [Crossref] [PubMed]
- Bennet AM, Di Angelantonio E, Ye Z, et al. Association of apolipoprotein E genotypes with lipid levels and coronary risk. JAMA 2007;298:1300-11. [Crossref] [PubMed]
- Wang XL, McCredie RM, Wilcken DE. Polymorphisms of the apolipoprotein E gene and severity of coronary artery disease defined by angiography. Arterioscler Thromb Vasc Biol 1995;15:1030-4. [Crossref] [PubMed]
- Brscic E, Bergerone S, Gagnor A, et al. Acute myocardial infarction in young adults: prognostic role of angiotensin-converting enzyme, angiotensin II type I receptor, apolipoprotein E, endothelial constitutive nitric oxide synthase, and glycoprotein IIIa genetic polymorphisms at medium-term follow-up. Am Heart J 2000;139:979-84. [Crossref] [PubMed]
- Baum L, Ng HK, Wong KS, et al. Associations of apolipoprotein E exon 4 and lipoprotein lipase S447X polymorphisms with acute ischemic stroke and myocardial infarction. Clin Chem Lab Med 2006;44:274-81. [Crossref] [PubMed]
- Johansson S, Bondjers G, Fager G, et al. Serum lipids and apolipoprotein levels in women with acute myocardial infarction. Arteriosclerosis 1988;8:742-9. [Crossref] [PubMed]
- Zhou Z, Zhang S, Ding S, et al. Excessive Neutrophil Extracellular Trap Formation Aggravates Acute Myocardial Infarction Injury in Apolipoprotein E Deficiency Mice via the ROS-Dependent Pathway. Oxid Med Cell Longev 2019;2019:1209307 [Crossref] [PubMed]
- Vaisar T, Pennathur S, Green PS, et al. Shotgun proteomics implicates protease inhibition and complement activation in the antiinflammatory properties of HDL. J Clin Invest 2007;117:746-56. [Crossref] [PubMed]
- Chen CL, Yen DH, Lin CS, et al. Glycated hemoglobin level is an independent predictor of major adverse cardiac events after nonfatal acute myocardial infarction in nondiabetic patients: A retrospective observational study. Medicine (Baltimore) 2017;96:e6743 [Crossref] [PubMed]
- Yu B, Wang W. Cardioprotective Effects of Morroniside in Rats Following Acute Myocardial Infarction. Inflammation 2018;41:432-6. [Crossref] [PubMed]
- Masoudkabir F, Karbalai S, Vasheghani-Farahani A, et al. The association of liver transaminase activity with presence and severity of premature coronary artery disease. Angiology 2011;62:614-9. [Crossref] [PubMed]
- Lofthus DM, Stevens SR, Armstrong PW, et al. Pattern of liver enzyme elevations in acute ST-elevation myocardial infarction. Coron Artery Dis 2012;23:22-30. [Crossref] [PubMed]
- Wang P, Wang Y, Ma W, et al. High-density lipoprotein cholesterol and intracoronary thrombosis burden. Coron Artery Dis 2013;24:1-5. [Crossref] [PubMed]
- Park JS, Cha KS, Lee HW, et al. Predictive and protective role of high-density lipoprotein cholesterol in acute myocardial infarction. Cardiol J 2019;26:176-85. [PubMed]
- Pearlstein E. Plasma membrane glycoprotein which mediates adhesion of fibroblasts to collagen. Nature 1976;262:497-500. [Crossref] [PubMed]
- Ozcelik F, Erdogan O, Aktoz M, et al. Diagnostic value of plasma fibronectin level in predicting the presence and severity of coronary artery disease. Ann Hematol 2009;88:249-53. [Crossref] [PubMed]
- Song KS, Kim HK, Shim W, et al. Plasma fibronectin levels in ischemic heart disease. Atherosclerosis 2001;154:449-53. [Crossref] [PubMed]
- Serebruany VL, Solomon SR, Herzog WR, et al. Plasma fibronectin during myocardial ischemia-reperfusion: effects of magnesium, diltiazem, and a novel Mac-1 inhibitor. Am J Hematol 1998;57:309-14. [Crossref] [PubMed]
- Herrmann J, Gersh BJ, Goldfinger JZ, et al. Body mass index and acute and long-term outcomes after acute myocardial infarction (from the Harmonizing Outcomes With Revascularization and Stents in Acute Myocardial Infarction Trial). Am J Cardiol 2014;114:9-16. [Crossref] [PubMed]
- van der Linden N, Wildi K, Twerenbold R, et al. Combining High-Sensitivity Cardiac Troponin I and Cardiac Troponin T in the Early Diagnosis of Acute Myocardial Infarction. Circulation 2018;138:989-99. [Crossref] [PubMed]
(English Language Editors: A. Kassem and J. Chapnick)