The mechanisms of celastrol in treating papillary thyroid carcinoma based on network pharmacology and experiment verification
Introduction
In recent years, the incidence of thyroid cancer (TC) has increased rapidly, and it has become the most common endocrine system malignant tumor (1). Approximately 85% of TCs are papillary thyroid carcinoma (PTC) (2). Although most early-stage PTC patients have a favorable prognosis after treatment, the likelihood of recurrence is greatly increased if metastasis is already present at the time of diagnosis (3). Metastases are known to cause more than 90% of deaths from cancer (4,5). In addition to the primary tumor cells, multiple stromal cells and inflammatory cells in the tumor microenvironment are also involved in metastasis. These cells can affect the progression of PTC by secreting various chemokines to participate in several mechanisms (6). It has been reported that inflammation can promote the transformation of normal thyroid tissues to malignant tumors by creating an advantageous immune microenvironment (7,8). Consequently, targeted treatment of the inflammatory microenvironment is promising for the prevention and treatment of PTC.
Celastrol, also known as tripterine, is a natural bioactive ingredient isolated from the plant Tripterygium wilfordii. Previous studies have shown that celastrol possesses a good therapeutic effect in many diseases, including Alzheimer disease, bronchial asthma, systemic lupus erythematosus, rheumatoid arthritis, and obesity (9-11). Most intriguingly, celastrol has clearly been demonstrated have anticancer effects in many tumor cells and animal models (12-14). It has been demonstrated that celastrol could inhibit the NLRP3 inflammasome, reduced the potency of macrophage to stimulate migration and invasion of tumor cells (15). Nevertheless, the underlying mechanism of celastrol in PTC is not well clarified.
Network pharmacology (NP) is a new approach that uses bioinformatics to observe the interactions of drugs on diseases, which provides a new logical guide and technical routes for developing and understanding of drugs, especially suitable for complex Traditional Chinese Medicine (TCM) (16). Molecular docking is a method which is most used for calculating protein-ligand interaction (17). Because celastrol has multiple pharmacological effects and multiple targets, its mechanism of action in tumor therapy is difficult to be revealed by traditional research methods. In recent years, many studies have combined bioinformatics and pharmacology through the application of network pharmacology to reveal the mechanism of action of these drugs and systematically elucidate their role in the treatment of diseases (18,19). Therefore, we will use network pharmacology to predict the target of celastrol in PTC and analyze the interaction between target and pathway-related PTC, so as to provide reference for the further study of the material basis and mechanism of anti-PTC. It will provide a new way and method for the research of celastrol. The workflow of this research is shown in Figure 1. We present the following article in accordance with the MDAR reporting checklist (available at http://dx.doi.org/10.21037/atm-21-1854).
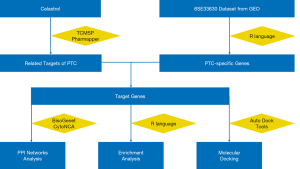
Methods
Screening the differentially expressed genes (DEGs) in PTC
Based on the GPL570-55999 Print_1437 platform, the dataset of gene expression in PTC and normal thyroid tissue, GSE33630, was downloaded from the Gene Expression Omnibus (GEO) online database. There were 105 samples in total, including 60 tumor tissues from PTC surgery patients and 45 normal thyroid tissues from non-malignant surgery patients. The pheatmap and limma packages in R were utilized to assess the results. DEGs between PTC tissue and normal thyroid tissue were checked based on the criteria |logFC| >1 and adjusted P value <0.05. The study was conducted in accordance with the Declaration of Helsinki (as revised in 2013).
Potential targets of celastrol
The celastrol chemical structures were acquired from PubChem (20). The Swiss Target Prediction online database and PharMapper were used to predict the target proteins which corresponded to chemical small molecules.
Protein-protein interaction (PPI) network construction
To visualize and understand the interaction mechanisms of these target proteins, we constructed a PPI network by utilizing the STRING 11.0 database (21). The candidate target proteins were used by STRING to construct and visualize the PPI network based on a minimum interaction value >0.9 (22). In the network, each node represented a target protein and each edge represented the PPI.
Enrichment analysis of Gene Ontology (GO) and Kyoto Encyclopedia of Genes and Genomes (KEGG)
GO enrichment analysis and KEGG pathway annotation were performed using the Bioconductor data package in R software (23,24). Adjusted P values ≤0.05 and subsequent pathways related to PTC were identified based on the pathological and clinical data.
Molecular docking
Based on the crystal structures of the proteins that were downloaded from RCSB Protein Data Bank (PDB) databases, molecular docking studies were performed with select proteins using AutodockTools 1.5.6. Docking results, the score ranging from 0 to 10 indicates that weak to strong combing ability of the proteins, as a negative logarithm of experimental dissociation/inhibition constan value (25).
Cell culture and treatment
PTC cell line BCPAP was obtained from Guangzhou Cellcook Biotech Co. (Guangzhou, China). Cells were cultured in RPMI 1640 (Gibco) supplemented with 10% fetal bovine serum, penicillin/streptomycin (5,000 units/mL, Gibco) and l-glutamine (2 mM, Gibco). The passage number of the cells used for the experiments was approximately 20–30. Celastrol was purchased from Sigma-Aldrich (St. Louis., MO, USA) and was dissolved into DMSO to a final concentration of 50 mM (26).
Quantitative real-time PCR (qRT-PCR)
RT-PCR assays were performed as previously described (27). Briefly, total RNA was extracted from cells using a RNeasy Mini Kit (Qiagen). cDNA synthesis was conducted using a Transcriptor First Strand cDNA Synthesis Kit (Takara) according to the manufacturer’s instructions. PCR was performed with FastStart Universal SYBR Green Master Mix (Takara) on an ABI ViiA7 system. The primers are listed in Table 1.

Full table
Western blot
Cells treated as described above were lysed in modified RIPA buffer comprising 1% PMSF. Equal amounts of total protein were resolved by SDS-PAGE and transferred onto PVDF membranes (Millipore). Then, the membranes were immunoblotted overnight with primary antibodies. The primary antibodies used for the western blot were MMP9 (BOSTER, PB9669; 1:1,000), JUN (BOSTER, BM4168; 1:1,000), ICAM1 (BOSTER, PB9018; 1:1,000), VCAM1 (BOSTER, A01199; 1:1,000) and GAPDH (Cell Signaling Technology, 5174; 1:40,000).
Statistical analysis
The software SPSS 22.0 software (SPSS Inc., Armonk, NY, USA) was used for statistical analysis. All values are presented as the mean ± standard deviation (SD) of three independent replicates. Student’s t-test was performed to compare differences. Significant differences were indicated by P<0.05 and P<0.01.
Results
Acquisition of DEGs in PTC
The 105 samples which were downloaded from the GEO database were separated into two groups, including the normal group containing 45 normal thyroid samples and the tumor group containing 60 PTC samples. A total of 904 DEGs were identified from the tumor group compared with the normal thyroid samples, including 423 up-regulated genes and 481 down-regulated genes. The identified DEGs between normal thyroid samples and the tumor group were presented in a volcano plot (Figure 2A). The heatmap shows the gene expression of DEGs (Figure 2B).
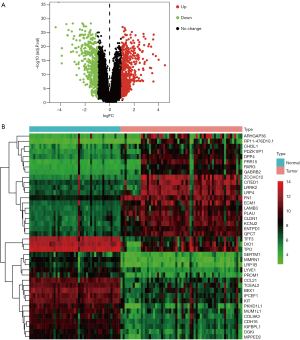
Potential targets of celastrol against PTC
The 2D molecular structure of celastrol is shown in Figure 3A. In total 392 targets of celastrol were identified by using the online database, then 47 common targets associated with both PTC and celastrol were summarized by a Venn diagram (Figure 3B).
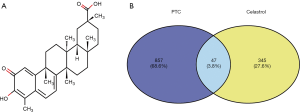
The PPI of candidate targets against PTC
In order to illustrate the relationships between the 47 common target proteins, a PPI network was constructed (Figure 4) which showed the interrelationships in the development and progression of PTC through the PPI network nodes in the disease. There were 47 nodes and 100 edges, the average node degree was 4.26, and the local clustering coefficient was 0.549 in our PPI network. The color of the node showed the degree of node contribution in the network and that there was a positive correlation in the development of PTC. Nodes such as MMP9, JUN, ICAM1, VCAM1, HMOX1, CASP1, and MMP1 were significantly enriched. The top 10 genes in the network ranked by degrees are shown in Table 2. Based on the results, MMP9, JUN, ICAM1, and VCAM1 were selected for further molecular docking experiments.
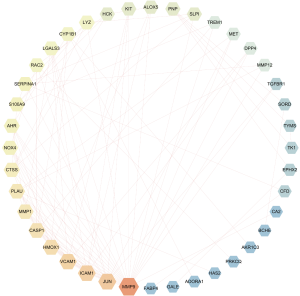
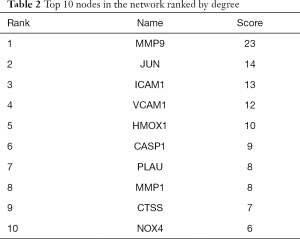
Full table
GO functional and KEGG pathway enrichment analysis
The 47 common genes of celastrol and PTC were further analyzed for biological processes and KEGG pathways. Biological processes with high enrichment scores such as neutrophil activation involved in the immune response, leukocyte migration, and extracellular matrix organization were associated with PTC (Figure 5A). The KEGG analysis results showed that the genes were mainly enriched in the interleukin-17 (IL-17), nuclear factor kappa-B (NF-κB), and tumor necrosis factor (TNF) signaling pathways (Figure 5B). The key pathway as shown in Figure S1.
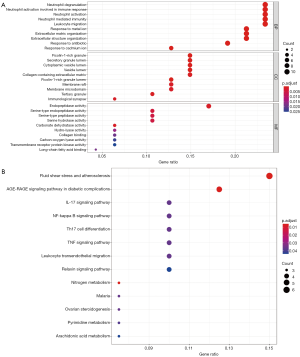
Hub proteins designation and molecular docking analysis
The molecular docking results showed that there was good binding between celastrol and the hub proteins, partially explaining the treatment mechanism of celastrol in PTC. Celastrol inhibited the development of PTC through the regulation of targets such as MMP9, JUN, ICAM1, and VCAM1, which was consistent with the results of NP screening. At the same time, the results of molecular docking verified the reliability of NP. The docking results between celastrol ligand and the PTC target protein receptors are shown in Table 3 and Figure 6.

Full table
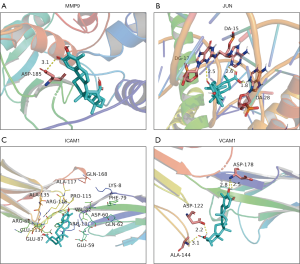
In vitro experiment
In order to clarify the mechanism of celastrol on PTC, the four core genes were measured by using qRT-PCR and western blot methods. The results were shown in Figure 7. Compared with control group, the MMP9, ICAM1, VCAM1 activity of celastrol treatment group has decreased, however the JUN activity of celastrol group has increased significantly, which was consistent with the above results.
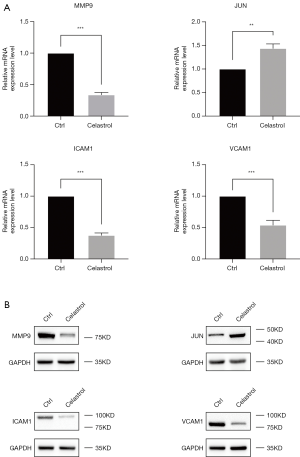
Discussion
Nowadays, research focused on natural products has gained more and more attention (28,29). There are many novel therapeutic drugs derived from natural products that have been decoded through a combination of NP and molecular biological analysis (30). This has provided new insights into the systemic connection between different diseases and therapeutic targets as a whole, and provides a promising and powerful tool to clarify disease mechanisms at a systems level and discover potential active compounds (31).
In the current study, publicly available databases with information on PTC were integrated to predict the potential therapeutic targets of celastrol and their interactions. These results showed that 47 target genes were up- or down-regulated by celastrol in PTC. After PPI network analysis, MMP9, JUN, ICAM1, and VCAM1 were identified as the key genes with the highest degrees. Modifications in matrix metalloproteinases 9 (MMP9), also known as 92-kDa gelatinase B type IV collagenase, play a significant role in various human tumors, including morphogenesis, differentiation, angiogenesis, metastasis, and tissue remodeling during tumor invasion (32,33). Previous studies have reported that MMP9 is overexpressed in PTC tissues, and targeted inhibition of MMP9 reduced migration and invasion in PTC cells (34). JUN, a leucine zipper protein dimer also known as AP-1, regulates many important cell processes, including cell proliferation, survival, apoptosis, invasion, and metastasis (35). In PTC, the up-regulation of JUN expression played a potential role in proliferation and transformation (36). Intercellular adhesion molecule 1 (ICAM1), a member of the immunoglobulin superfamily, is considered to play an important role in the inflammatory response and immune processes (37). It was demonstrated that the overexpression of ICAM1 was associated with extrathyroidal invasion and lymph node metastasis (38). Vascular cell adhesion molecular 1 (VCAM1), also known as CD106, is also an important member of immunoglobulin superfamily. It has been reported that VCAM1 overexpression can promote thyroid tumor cell migration and invasion in vitro (39).
On the basis of GO enrichment analysis, the BP terms of target gene enrichment mainly focus on the response to various materials and the biochemical processes of different substances. Neutrophil activation involved in the immune response, leukocyte migration, and extracellular matrix organization are all important in PTC tumorigenesis (40). Some of the enriched MF terms were related to inflammation, for instance endopeptidase activity, serine-type endopeptidase activity, and carbon-oxygen lyase activity. KEGG enrichment analysis suggested that the celastrol pharmacological action in PTC is closely related to well-known pathways which are thought to be associated with tumors, such as the IL-17, TNF, and NF-κB signaling pathways. The proinflammatory cytokine IL-17 was shown to be significantly up-regulated in PTC tissues (41). The overexpression of IL-17 induces MHC class I expression in PTC and facilitates tumor antigenicity via the PD-1/PD-L1 signaling pathway (42). TNF-α is an important cytokine associated with cell growth, differentiation, and apoptosis (43), and also plays a significant role in regulating the adhesion and migration of PTC cells (44). Serum TNF-α level can serve as an indicator of the risk of benign thyroid nodule cancerization, and may be a predictive factor in the occurrence and development of TC and the prognosis of TC patients (45). NF-κB transcription factors regulate cell proliferation, invasion, migration, and EMT processes of PTC cells, and is a key regulatory factor (46). It has been revealed that targeted inhibition of the NF-κB signaling pathway can have a good therapeutic effect against PTC by suppressing proliferation and accelerating apoptosis (47).
These results indicate that celastrol can inhibit PTC through the above mechanisms, which are closely associated with the key target proteins, biological processes, and tumor signaling pathways described in this study. Further experiments and clinical validation are still required to verify our findings.
Conclusions
In our study, we first clarified the pharmacological effects of celastrol against PTC by using NP analysis. A total of 47 target genes including MMP9, JUN, ICAM1, and VCAM1 were shown to be the hub target proteins for the anticancer effects of celastrol. On the other hand, functional enrichment analysis including GO and KEGG demonstrated that proteins targeted by celastrol were markedly enriched in immune microenvironment-related signaling pathways such as IL-17, NF-κB, and TNF. This research provides a theoretical basis for further studies of celastrol in PTC.
Acknowledgments
Funding: This work was supported by grants from the National Natural Science Foundation of China (81872169), Tianjin Key Research and Development Program Science and Technology Support Key Projects (17YFZCSY00690), Tianjin Municipal Science and Technology Project (19JCYBJC27400) and Beijing-Tianjin-Hebei Basic Research Cooperation Project (20JCZXJC00120).
Footnote
Reporting Checklist: The authors have completed the MDAR reporting checklist. Available at http://dx.doi.org/10.21037/atm-21-1854
Conflicts of Interest: All authors have completed the ICMJE uniform disclosure form (available at http://dx.doi.org/10.21037/atm-21-1854). The authors have no conflicts of interest to declare.
Ethical Statement: The authors are accountable for all aspects of the work in ensuring that questions related to the accuracy or integrity of any part of the work are appropriately investigated and resolved. The study was conducted in accordance with the Declaration of Helsinki (as revised in 2013).
Open Access Statement: This is an Open Access article distributed in accordance with the Creative Commons Attribution-NonCommercial-NoDerivs 4.0 International License (CC BY-NC-ND 4.0), which permits the non-commercial replication and distribution of the article with the strict proviso that no changes or edits are made and the original work is properly cited (including links to both the formal publication through the relevant DOI and the license). See: https://creativecommons.org/licenses/by-nc-nd/4.0/.
References
- Coca-Pelaz A, Shah JP, Hernandez-Prera JC, et al. Papillary Thyroid Cancer-Aggressive Variants and Impact on Management: A Narrative Review. Adv Ther 2020;37:3112-28. [Crossref] [PubMed]
- Davies L, Welch HG. Increasing incidence of thyroid cancer in the United States, 1973-2002. JAMA 2006;295:2164-7. [Crossref] [PubMed]
- Abdullah MI, Junit SM, Ng KL, et al. Papillary Thyroid Cancer: Genetic Alterations and Molecular Biomarker Investigations. Int J Med Sci 2019;16:450-60. [Crossref] [PubMed]
- Guan X. Cancer metastases: challenges and opportunities. Acta Pharm Sin B 2015;5:402-18. [Crossref] [PubMed]
- Monteiro J, Fodde R. Cancer stemness and metastasis: therapeutic consequences and perspectives. Eur J Cancer 2010;46:1198-203. [Crossref] [PubMed]
- Urra S, Fischer MC, Martinez JR, et al. Differential expression profile of CXCR3 splicing variants is associated with thyroid neoplasia. Potential role in papillary thyroid carcinoma oncogenesis? Oncotarget 2017;9:2445-67. [Crossref] [PubMed]
- Guarino V, Castellone MD, Avilla E, et al. Thyroid cancer and inflammation. Mol Cell Endocrinol 2010;321:94-102. [Crossref] [PubMed]
- Ward LS. Immune response in thyroid cancer: widening the boundaries. Scientifica (Cairo) 2014;2014:125450 [Crossref] [PubMed]
- Liu J, Lee J, Salazar Hernandez MA, et al. Treatment of obesity with celastrol. Cell 2015;161:999-1011. [Crossref] [PubMed]
- Sharma S, Mishra R, Walker BL, et al. Celastrol, an oral heat shock activator, ameliorates multiple animal disease models of cell death. Cell Stress Chaperones 2015;20:185-201. [Crossref] [PubMed]
- Kannaiyan R, Shanmugam MK, Sethi G. Molecular targets of celastrol derived from Thunder of God Vine: potential role in the treatment of inflammatory disorders and cancer. Cancer Lett 2011;303:9-20. [Crossref] [PubMed]
- Zhang CJ, Zhu N, Long J, et al. Celastrol induces lipophagy via the LXRalpha/ABCA1 pathway in clear cell renal cell carcinoma. Acta Pharmacol Sin 2020; Epub ahead of print. [Crossref] [PubMed]
- Liu M, Fan Y, Li D, et al. Ferroptosis inducer erastin sensitizes NSCLC cells to celastrol through activation of the ROS-mitochondrial fission-mitophagy axis. Mol Oncol 2021; Epub ahead of print. [Crossref] [PubMed]
- Si H, Wang H, Xiao H, et al. Anti-Tumor Effect of Celastrol on Hepatocellular Carcinoma by the circ_SLIT3/miR-223-3p/CXCR4 Axis. Cancer Manag Res 2021;13:1099-111. [Crossref] [PubMed]
- Lee HE, Lee JY, Yang G, et al. Inhibition of NLRP3 inflammasome in tumor microenvironment leads to suppression of metastatic potential of cancer cells. Sci Rep 2019;9:12277. [Crossref] [PubMed]
- Chandran U, Mehendale N, Tillu G, et al. Network Pharmacology of Ayurveda Formulation Triphala with Special Reference to Anti-Cancer Property. Comb Chem High Throughput Screen 2015;18:846-54. [Crossref] [PubMed]
- Zhou C, Liu L, Zhuang J, et al. A Systems Biology-Based Approach to Uncovering Molecular Mechanisms Underlying Effects of Traditional Chinese Medicine Qingdai in Chronic Myelogenous Leukemia, Involving Integration of Network Pharmacology and Molecular Docking Technology. Med Sci Monit 2018;24:4305-16. [Crossref] [PubMed]
- Wu XM, Wu CF. Network pharmacology: a new approach to unveiling Traditional Chinese Medicine. Chin J Nat Med 2015;13:1-2. [Crossref] [PubMed]
- Chen L, Lv D, Wang D, et al. A novel strategy of profiling the mechanism of herbal medicines by combining network pharmacology with plasma concentration determination and affinity constant measurement. Mol Biosyst 2016;12:3347-56. [Crossref] [PubMed]
- Kim S, Thiessen PA, Bolton EE, et al. PubChem Substance and Compound databases. Nucleic Acids Res 2016;44:D1202-13. [Crossref] [PubMed]
- Szklarczyk D, Morris JH, Cook H, et al. The STRING database in 2017: quality-controlled protein-protein association networks, made broadly accessible. Nucleic Acids Res 2017;45:D362-D368. [Crossref] [PubMed]
- Merico D, Isserlin R, Stueker O, et al. Enrichment map: a network-based method for gene-set enrichment visualization and interpretation. PLoS One 2010;5:e13984 [Crossref] [PubMed]
- Kanehisa M, Goto S. KEGG: kyoto encyclopedia of genes and genomes. Nucleic Acids Res 2000;28:27-30. [Crossref] [PubMed]
- Yu G, Wang LG, Han Y, et al. clusterProfiler: an R package for comparing biological themes among gene clusters. OMICS 2012;16:284-7. [Crossref] [PubMed]
- Hsin KY, Ghosh S, Kitano H. Combining machine learning systems and multiple docking simulation packages to improve docking prediction reliability for network pharmacology. PLoS One 2013;8:e83922 [Crossref] [PubMed]
- Ni H, Han Y, Jin X. Celastrol inhibits colon cancer cell proliferation by downregulating miR-21 and PI3K/AKT/GSK-3beta pathway. Int J Clin Exp Pathol 2019;12:808-16. [PubMed]
- Fedele C, Ran H, Diskin B, et al. SHP2 Inhibition Prevents Adaptive Resistance to MEK Inhibitors in Multiple Cancer Models. Cancer Discov 2018;8:1237-49. [Crossref] [PubMed]
- Hiebl V, Ladurner A, Latkolik S, et al. Natural products as modulators of the nuclear receptors and metabolic sensors LXR, FXR and RXR. Biotechnol Adv 2018;36:1657-98. [Crossref] [PubMed]
- Liang JW, Wang MY, Olounfeh KM, et al. Network pharmacology-based identifcation of potential targets of the flower of Trollius chinensis Bunge acting on anti-inflammatory effectss. Sci Rep 2019;9:8109. [Crossref] [PubMed]
- Li XJ, Jiang ZZ, Zhang LY. Triptolide: progress on research in pharmacodynamics and toxicology. J Ethnopharmacol 2014;155:67-79. [Crossref] [PubMed]
- Huang J, Cheung F, Tan HY, et al. Identification of the active compounds and significant pathways of yinchenhao decoction based on network pharmacology. Mol Med Rep 2017;16:4583-92. [Crossref] [PubMed]
- López-Otín C, Overall CM. Protease degradomics: a new challenge for proteomics. Nat Rev Mol Cell Biol 2002;3:509-19. [Crossref] [PubMed]
- Egeblad M, Werb Z. New functions for the matrix metalloproteinases in cancer progression. Nat Rev Cancer 2002;2:161-74. [Crossref] [PubMed]
- Zarkesh M, Zadeh-Vakili A, Akbarzadeh M, et al. The role of matrix metalloproteinase-9 as a prognostic biomarker in papillary thyroid cancer. BMC Cancer 2018;18:1199. [Crossref] [PubMed]
- Ye N, Ding Y, Wild C, et al. Small molecule inhibitors targeting activator protein 1 (AP-1). J Med Chem 2014;57:6930-48. [Crossref] [PubMed]
- Xiao C, Huang Y, Gao Q, et al. Expression of activator protein-1 in papillary thyroid carcinoma and its clinical significance. World J Surg Oncol 2019;17:25. [Crossref] [PubMed]
- Pober JS, Gimbrone MA Jr, Lapierre LA, et al. Overlapping patterns of activation of human endothelial cells by interleukin 1, tumor necrosis factor, and immune interferon. J Immunol 1986;137:1893-6. [PubMed]
- Zhang KE, Ge SJ, Lin XY, et al. Intercellular adhesion molecule 1 is a sensitive and diagnostically useful immunohistochemical marker of papillary thyroid cancer (PTC) and of PTC-like nuclear alterations in Hashimoto's thyroiditis. Oncol Lett 2016;11:1722-30. [Crossref] [PubMed]
- Chen S, Su X, Jiang X, et al. VCAM-1 Upregulation Contributes to Insensitivity of Vemurafenib in BRAF-Mutant Thyroid Cancer. Transl Oncol 2020;13:441-51. [Crossref] [PubMed]
- Xie Z, Li X, He Y, et al. Immune Cell Confrontation in the Papillary Thyroid Carcinoma Microenvironment. Front Endocrinol (Lausanne) 2020;11:570604 [Crossref] [PubMed]
- Cui G, Yang H, Zhao J, et al. Elevated proinflammatory cytokine IL-17A in the adjacent tissues along the adenoma-carcinoma sequence. Pathol Oncol Res 2015;21:139-46. [Crossref] [PubMed]
- Han LT, Hu JQ, Ma B, et al. IL-17A increases MHC class I expression and promotes T cell activation in papillary thyroid cancer patients with coexistent Hashimoto's thyroiditis. Diagn Pathol 2019;14:52. [Crossref] [PubMed]
- Aggarwal BB, Gupta SC, Kim JH. Historical perspectives on tumor necrosis factor and its superfamily: 25 years later, a golden journey. Blood 2012;119:651-65. [Crossref] [PubMed]
- Lv N, Gao Y, Guan H, et al. Inflammatory mediators, tumor necrosis factor-alpha and interferon-gamma, induce EMT in human PTC cell lines. Oncol Lett 2015;10:2591-7. [Crossref] [PubMed]
- Kobawala TP, Trivedi TI, Gajjar KK, et al. Significance of TNF-alpha and the Adhesion Molecules: L-Selectin and VCAM-1 in Papillary Thyroid Carcinoma. J Thyroid Res 2016;2016:8143695 [PubMed]
- Zhou B, Xu J, Chen Y, et al. miR-200b/c-RAP1B axis represses tumorigenesis and malignant progression of papillary thyroid carcinoma through inhibiting the NF-kappaB/Twist1 pathway. Exp Cell Res 2020;387:111785 [Crossref] [PubMed]
- Zhao S, Wang Q, Li Z, et al. LDOC1 inhibits proliferation and promotes apoptosis by repressing NF-kappaB activation in papillary thyroid carcinoma. J Exp Clin Cancer Res 2015;34:146. [Crossref] [PubMed]
(English Language Editor: C. Betlazar-Maseh)