Applications of artificial intelligence in oncologic 18F-FDG PET/CT imaging: a systematic review
Introduction
Positron emission tomography (PET) is a non-invasive functional imaging modality that can provide valuable information regarding the diagnosis and management of patients with cancer (1). Image acquisition is based on detecting photons produced by annihilation of positrons that are emitted from administered radiotracers (1). The most widely used PET-radiotracer in clinical practice is 2-deoxy-2-[18F]fluoro-D-glucose (18F-FDG), which provides an estimation of glucose metabolism in tissues (2). 18F-FDG is the workhorse of oncological PET imaging and is recommended for staging, restaging, therapy response assessment, and recurrence detection of different cancers. In addition, PET imaging allows quantitative assessment of tumor glucose metabolism and provides additional diagnostic and prognostic information which is particularly helpful in monitoring therapy response assessment (3). Different 18F-FDG PET-derived imaging biomarkers have been proposed for this purpose including tumor glycolytic activity as reflected by the maximum standardized uptake value (SUVmax), metabolic tumor volume (MTV), and total lesion glycolysis (TLG) (4).
Artificial intelligence (AI) is a growing research field that has affected our lives in many aspects (5). AI is defined as the study of “intelligent agents” that can perceive the surrounding environment and take actions to maximize the chances of successfully achieving predefined goals (6). Machine learning (ML) is a subset of AI that involves computer algorithms. ML algorithms build a model based on the training data to make predictions or decisions. AI encompasses a broader range of intelligent functions, such as rule-based algorithms that have been used in mammography for computer-aided diagnosis. However, ML algorithms are not rule-based (7). ML is currently improving many aspects of modern society, from image processing to web searches to medical decision support (8). Deep learning (DL) is a type of ML methodology that is based on artificial neural networks. DL is considered representation learning which includes a set of methods that would enable a machine to be fed with raw data which results in automatic detection of the needed representation for classification. A convolutional neural network (CNN) is a type of deep learning architecture inspired by neural system that can learn representations of input data (8). DL is emerging as a promising tool in detection and management of different cancers.
AI can improve different aspects of medical imaging. It can be helpful in optimizing orders, protocoling studies, screening, scheduling, providing clinical decision support, improving image acquisition, quality assessment, and image archiving as well as post-processing approaches including tumor delineation, registration, and quantification. Moreover, it can help to optimize dose estimation, and provide automated detection of abnormalities, compare with prior studies and assess response to therapy, interpret and generate report, and correlate image findings with other clinical data (7). 18F-FDG PET as a diagnostic imaging modality can benefit from AI in all the abovementioned aspects. The result will make the process faster and more accurate.
In this systematic review, we will summarize the relevant literature regarding the application of AI in 18F-FDG PET/CT Imaging of different cancers.
We present the following article in accordance with the PRISMA reporting checklist (available at http://dx.doi.org/10.21037/atm-20-6162).
Methods
A systematic literature review was performed in PubMed, using the following search terms: “(“Positron Emission Tomography Computed Tomography”[Mesh]) AND “Artificial Intelligence”[Mesh]”. No date limit was considered. The search was last updated in August 2020. A total of 65 studies were available to be reviewed against the inclusion criteria. The included studies were those that developed an AI model based on 18F-FDG PET data in cancer to diagnose, differentiate, delineate, stage, assess response to therapy, determine prognosis, or improve image quality. Figure 1 shows the flowchart of study selection process. Fifteen studies were excluded based on the review of the title and abstract. The full text of the remaining 50 studies were reviewed. 18 studies were excluded (13 studies for not including 18F-FDG PET data as the input to the AI model, 2 studies for not evaluating AI models, and 3 studies for being review articles). Thirty-two studies met the inclusion criteria.
Quality assessment
Study quality was evaluated based on QUADAS-2 (supplementary material).
Statistical analysis
Due to considerable heterogeneity among the studies including diversity of the diseases and evaluated outputs, meta-analysis was not performed.
Results
The list of included studies based on the type of disease is provided in Table 1. The most common evaluated cancer with AI models was lung cancer. Other studied cancers included head and neck cancer, lymphoma, pancreatic cancer, breast cancer, cervical cancer, and sarcoma. Herein, we summarize the available literature on the application of AI-based PET/CT algorithms in different cancers.
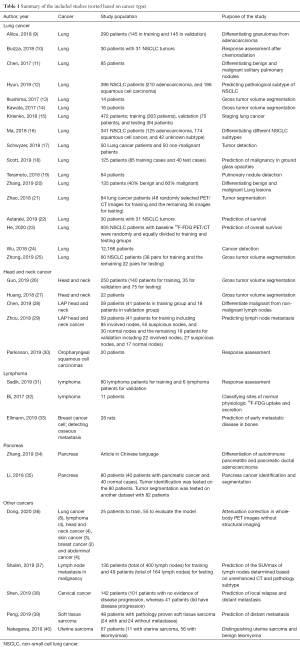
Full table
Lung cancer
18F-FDG PET/CT has been incorporated in routine imaging work-up of lung cancer and plays an important role in the multidisciplinary management of patients. AI techniques were developed regarding different aspects of lung cancer imaging including characterization of malignant pulmonary nodules, tumor detection and delineation, differentiation of lung cancer subtypes, lung cancer staging, and response assessment. In addition, AI-based models show promising value in predicting tumor behavior, response to different therapies, and patients’ survival.
Tumor detection
In a study in 2016, Teramoto et al. evaluated the role of a CNN in automated detection of pulmonary nodules (19), based on 104 18F-FDG PET/CT images. The CNN was used to improve the false positives from their prior study which was published in 2015 based on automated detection of lung nodules using an active contour filter (ACF) (41). ACF is a type of contrast enhancement filter with a deformable kernel. The nodule was detected from CT and PET images separately, based on the ACF and thresholding, respectively. Using the CNN, the false positive detection per case decreased from 72.8 to 4.9. The authors concluded that using CNN technique results in decreased false positives.
In a separate study, the data of 2,789,675 patients from three different hospitals in China were screened (24). 12,186 patients with non-small cell lung cancer (NSCLC) and a total of 48,145 lung images were extracted for ML. The algorithm accuracy in detecting cancer increased by increasing the input cases from 57% for 100 cases to 77% for 3,000 cases.
Tumor delineation
Ikushima et al. showed that an ML approach could be utilized to train delineation of gross tumor volumes (GTV) based on optimum contour selection (OCS) (13). The leave-one-out-by-patient was used for training and testing the data. The GTV contour was determined based on the knowledge of radiation oncologists. A support vector machine (SVM) technique was used to extract the initial GTV regions. The image features were fed into an ML classifier. 3D dice similarity coefficient (DSC) was used to assess the efficacy of the framework. DSC provided the similarity between the GTV contours by radiation oncologists and the framework output. The average DSC for 14 cases with lung cancer was 0.777. The authors concluded that this framework can be utilized in determining GTV contours.
In another study, Kawata et al. used 3 different approaches with pixel-based ML techniques including fuzzy-c-means clustering method (FCM), artificial neural network (ANN), and SVM to develop an automated framework for delineation of the GTV. The morphological and metabolic data of 16 lung cancer patients with PET/CT images were determined based on the knowledge of 2 separate radiation oncologists and were fed into FCM, ANN, and SVM. The PET and diagnostic GTV contoured by radiation oncologists were compared with the output of an automated framework for 3 different ML-based approaches. The similarity between the 2 methods was evaluated based on DSC. FCM-based framework provided the highest DSC (0.79±0.06) in comparison to ANN (0.76±0.14) and SVM (0.73±0.14). The FCM based framework had the highest segmentation accuracy and precision (14).
Zhong et al. fed PET and CT images into 3D DL fully convolutional networks (DFCN) to develop a co-segmentation model based on PET/CT images (25). They used two coupled 3D U-Net models that could communicate to share the information between CT and PET images. 60 NSCLC patients with PET/CT images who had stereotactic body radiation therapy (SBRT) were included. The output was compared with manual segmentations by 3 expert physicians. DFCN co-segmentation based on PET and CT images outperformed the models based on either PET or CT alone.
Staging lung cancer
A CNN has been used to classify lung cancer to T1-T2 or T3-T4 stages based on 18F-FDG PET/CT imaging (15). 472 patients (T1-T2 =353 patients, T3-T4 =119 patients) were divided into training (n=303), validation (n=75), and testing (n=94) groups. The dataset included 3D bounding boxes, cropped around the center of the lesions by 2 nuclear medicine physicians on both PET and CT images, and were fed to a CNN. A fivefold cross-validation strategy was used for the training. Concordance between the algorithm prediction and reference was classified as “Correct” and discordance as “Incorrect”. The accuracy [Correct/(Correct + Incorrect)] in the training, validation, and test sets were 87%, 86%, and 90%, respectively.
Response assessment
The SUVmax from PET and Hounsfield units (HU) from CT scans can be used for response assessment after chemoradiation. Buizza et al. used linear SVMs to describe the temporal and spatial changes of the tumors (10). This model was tested on pre-radiation 18F-FDG PET/CT images as well as images during the first 3 weeks of radiation therapy for 30 patients with non-small cell lung cancer who had sequential or concurrent chemoradiation. 15 patients had concurrent chemoradiation therapy, while the other 15 had sequential chemoradiation with 3 cycles of chemotherapy before radiation. A set of imaging features named longitudinal pattern features (LoP) was designed that provided information about the alterations of spatial intensity as a function of distance from the tumor border. Using SVM, the patients were classified as responders or non-responders. This model was compared to a set of standard radiomics features. Based on the linear SVM, the LoP from both CT and PET images consistently outperformed radiomics results for response assessment.
Differentiating benign lesions from malignancies
Schwyzer et al. studied the role of an ANN in differentiating lung cancer from non-malignant lung lesions (17). 50 patients with lung cancer and 50 patients without pulmonary malignancy were included in the study. A pre-trained deep residual neural network was used for 3936 slices of JPEG images converted from reconstructed 2D PET images. Besides the standard reconstruction of PET images (named PET100%), two other reconstructions were created to simulate lower injected doses (PET10% simulating tenfold and PET3.3% simulating thirtyfold decrease in injected dose). The AUC of the algorithm for PET100% was 0.989 (95% CI: 0.983-0.995), for PET10% was 0.983 (95% CI: 0.977-0.989), and for PET3.3% was 0.970 (95% CI: 0.961-0.978). There was no significant difference between the AUC for PET100% and PET 10%, however, the PET3.3% AUC was significantly lower than PET100%. The sensitivity of PET100%, PET10%, and PET3.3% was 95.9%, 93.6%, and 91.5% respectively, and specificities were 98.1%, 94.3%, and 94.2% respectively. This study showed that DL can provide automated lung cancer detection based on PET images even at much lower effective radiation doses.
Dual time point imaging (DTPI) has recently been suggested to improve the accuracy of differentiating benign and malignant lesions on 18F-FDG PET scans. This is achieved by acquiring additional delayed scans (11). Retention Index (RI) can be calculated by subtraction of the early SUV from the delayed SUV divided by the early SUV. Solitary pulmonary nodules (SPNs) can be detected on CT scan and may represent benign or neoplastic lesions. In a study, Chen at all used DTPI to differentiate malignant and benign SPNs based on texture features in delayed 18F-FDG PET/CT images in patients from granuloma-endemic regions. They used an SVM model for early CT (eCT), early PET (ePET), early PET+CT (ePET+CT), delayed PET (dPET), early and delayed PET+CT (edPET+CT), early SUVmax, and RI. dPET and edPET+CT showed the highest accuracy (both 0.86) and specificity (both 0.91) in differentiating benign and malignant SPNs.
Alilou et al. suggested that quantitative vessel tortuosity (QVT) as a novel CT biomarker can differentiate granulomas from adenocarcinomas (9). To assess the nodular vasculature tortuosity, curvature, and branching, 35 QVT features were extracted from non-contrast CT images. Both granulomas and adenocarcinoma can present with 18F-FDG avidity and spiculated margins. 290 patients with PET/CT were divided in half, 145 images were used for training and the other 145 cases were reserved for validation. The top informative and stable QVT characteristics in conjunction with an ML classifier provided an AUC of 0.85 in the validation dataset while the AUC for a radiologist and pulmonologist were 0.61 and 0.60, respectively. According to the authors QVT features outperformed conventional radiomics features.
In another recent study, Zhang et al. used the radiomics features from CT images, and metabolic variables from the PET data of 135 patients with lung lesions and pathologic diagnoses (40% benign and 60% malignant) to develop an SVM with a five-fold cross-validation approach to differentiate benign and malignant lung lesions (20). The AUC as a measurement of diagnostic performance for CT-radiomics, PET-metabolic data, and the combination were 0.820±0.053, 0.874±0.081, and 0.887±0.046, respectively.
In a recent study, an artificial network was developed that was based on imaging features (including the ratio of the SUVmax of the lesion to SUV of the liver, mean diameter of lesions, and morphology of the lesion) and clinical data (including age and history of lung cancer) to predict the likelihood of malignancy of pure ground-glass opacities (18). 125 cases (85 training cases and 40 test cases) with pure ground-glass opacities on 18F-FDG PET/CT images were included in the study. 85 patients were used randomly for training, and 40 cases were included in the testing group. The predictive role of the ANN to detect malignant lesions was compared with the estimations of two blinded experts. The ANN demonstrated an excellent predictive value with an AUC of 0.98±0.02.
Differentiating different subtypes of non-small cell lung carcinoma
In order to differentiate different types of NSCLC, 341 18F-FDG PET/CT images from patients with NSCLC including 125 with adenocarcinoma, 174 with squamous cell cancer, and 42 with unknown subtype were included in a study by Ma et al. (16). Texture features for ROIs from the fused PET/CT images were calculated. Moreover, to improve tumor classification and segmentation, the grayscale PET and CT images were converted to color images. Discriminatory analysis was performed based on an SVM ML algorithm to assess tumor subtype prediction. The best classifier performance was achieved by combining both texture and color data that resulted in AUC of 0.89 (95% CI, 0.78-1.00).
In a different PET-based study, 396 patients with pathology-proven NSCLC (210 with adenocarcinoma and 186 with squamous cell carcinoma) with pre-therapeutic 18F-FDG PET/CT were studied (12). 40 radiomic features from PET images and 4 from clinical data were analyzed with 5 different ML algorithms, including logistic regression, random forest, naive Bayes, neural network, and SVM. The best 5 predictors of adenocarcinoma included sex, SUVmax, total lesion glycolysis, gray-level zone length nonuniformity, and gray-level nonuniformity for zone. The logistic regression outperformed the other 4 models with AUC value of 0.859.
Survival and prognosis
Astaraki et al. trained a linear SVM to utilize a novel feature set based on PET and CT images to predict early survival in patients with NSCLS (22). 30 patients with 31 NSCLC tumors were included in the study. The proposed feature set was named size-aware longitudinal pattern (SALoP) and would quantify the spatial and temporal variations in structural and functional imaging of the lesion. In addition to SALoP, a set of PET and CT radiomics features recommended by the Image Biomarker Standardization Initiative (IBSI) were extracted. Patients were followed for up to 2 years after the last radiation therapy. The binary overall survival status after 2 years was considered as the endpoint. The SALoP outperformed the radiomics approach in predicting the prognosis.
In another study, 935 NSCLC patients with baseline 18F-FDG PET/CT were randomly and equally divided into training and testing groups (23). An ML random survival forest (RSF) model was developed to predict overall survival. Input variables included demographic information, TNM staging, treatment category, PET-derived data including maximum standard uptake value of whole-body tumor (SUVmaxWB), and whole-body metabolic tumor volume (MTVwb). The best performance was associated with TNM staging, MTVwb, and treatment category, indicating the prognostic value of these variables.
Head and neck cancer
The use of 18F-FDG PET/CT imaging in the work-up of head and neck cancer patients has greatly increased in recent decades, in various clinical settings including staging, re-staging, radiotherapy planning, and outcome assessment.
Tumor delineation
An automated deep learning method has been studied to contour the GTV in head and neck cancer (27). A deep CNN was developed and trained based on the reconstructed 2D PET/CT images of 22 newly diagnosed patients with head and neck cancer (Figure S1) from two different centers. Tumor segmentation based on the deep CNN method was compared against the manual segmentation by an oncologist and a radiologist. There was a high correlation between GTVs delineated by experts and those generated by CNN.
In another study, the therapeutic planning CT, as well as 18F-FDG PET/CT images of patients with head and neck cancer were used to develop a deep dense multi-modality network to segment the GTV of the lesion (26). 250 patients with head and neck cancer were included in the study. 140 patient scans were used for training, 35 scans for validation, and 75 scans for testing. The output segmentation of the Dense-Net framework with CT images, PET images, and PET+CT images were compared with manual segmentations by radiation oncologists. Based on the results of five-fold cross-validation, the multi-modality dense-net (including PET and CT images) had better performance in contrast to CT alone, PET alone, and the state-of-the-art framework (3D-U-Net).
Differentiation of malignant from non-malignant lymph nodes
Chen et al. developed a hybrid model including many-objective radiomics (MaO-radiomics) and 3D CNN to classify normal, suspicious, and malignant lymph nodes in patients with head and neck cancer (28). Fifty-nine patients with head and neck cancer with pretherapeutic PET/CT images were included. The first 41 patients were included in the training group (a total of 170 nodes, including 85 involved lymph nodes, 55 suspicious nodes, and 30 normal nodes). Validation was done on the data of 18 independent patients (a total of 66 nodes, including 22 involved, 27 suspicious, and 17 normal). Reference was developed based on nodal status determination by a radiation oncologist and a nuclear medicine radiologist. To fully utilize spatial contextual data, evidential reasoning (ER) was used to fuse the output of the CNN with radiomics information. The accuracy of the hybrid model was 0.88 which was higher than radiomics alone (0.75).
Response assessment
AI-algorithms can play an important role in therapy response assessment and monitoring changes in tumors over time, by identifying PET-based radiomic features with high discriminative capabilities across images over time. Radiomic features of PET can predict response to chemoradiation in patients with head and neck cancer (42).
Delineation of post-therapeutic biologic tumor volume in the 18F-FDG PET images of patients with oropharyngeal squamous cell carcinoma is challenging secondary to decreased 18F-FDG avidity and smaller volumes. Parkinson et al. developed an ML model for biological tumor volume delineation after one cycle of chemotherapy (30). 20 patients with oropharyngeal squamous cell cancer who had 18F-FDG PET/CT at baseline and 3 weeks post-chemotherapy were included. An ML model including Automatic decision Tree-based Learning Algorithm for Advanced image Segmentation (ATLAAS) algorithm was developed. The output was compared against manual segmentation by a nuclear medicine physician and an oncologist. The DSC value for ATLAAS was 0.72 which was higher than other studied models including 60% peak thresholding (PT60), adaptive thresholding (AT), and watershed thresholding (WT).
Lymphoma
18F-FDG PET/CT imaging has been incorporated in the staging workup and therapy response assessment of Hodgkin lymphoma and certain types of non-Hodgkin lymphoma for more than a decade. To date, only a few studies about the application of AI in the imaging of lymphoma have been published. In an experiment on 40 whole-body lymphoma PET/CT exams, Bi et al. proposed that a deep CNN can be used to classify sites of normal physiologic 18F-FDG uptake and excretion in 18F-FDG PET images (32). They used multi-scale superpixel-based encoding (MSE) to group different fragments of normal 18F-FDG uptake into larger segments which would allow the CNN to extract the highly discriminating features. The accuracy of the model was higher than other existing methods, such as patch-SVM, to classify the normal 18F-FDG uptake regions.
Response assessment
The Lugano classification is the standardized 18F-FDG-PET-based classification system recommended for interim, and end-of-treatment response assessment of lymphomas (43). The Lugano classification recommends the five-point Deauville scale to assign metabolic response categories. In Deauville scale, 18F-FDG uptake of each lesion is graded in relation to the reference regions of the normal mediastinum (blood pool) and liver (43). Thus, a major step in evaluating response to therapy in lymphoma is to determine the liver and mediastinal blood pool references. Sadik et al. showed that an AI-based model can be trained to perform automated segmentation of liver and mediastinal blood pool in CT images and then transfer the ROI to PET images to calculate the SUV of the reference regions. 80 patients with lymphoma who had undergone 18F-FDG PET/CT were included in this study. The automated quantification of the reference levels was comparable with manually segmented regions by experienced radiologists (31).
Tumor burden
In addition, some studies suggested that 18F-FDG PET-based radiomics features combining metrics reflecting tumor burden (metabolic tumor volume) and tumor dissemination can provide prognostic information and improve risk stratification at staging (44,45). One of the recently introduced radiomic features to quantify tumor dissemination is Dmax, which is the distance between the two lesions that were farthest apart (44) (Figure S2). Tumor delineation and quantification have been time-consuming, particularly in those with advanced disease and multiple lesions (45). The application of ML and AI can optimize precise volumetric delineation of tumors and reduce observational oversights.
Pancreas
Detection of pancreatic cancer in non-contrast CT might be challenging, and 18F-FDG PET helps in the detection and delineation of hypermetabolic tumors. Figure S3 demonstrates 18F-FDG PET/CT images of a patient with adenocarcinoma where the border of tumor in non-contrast CT is not clearly visible while the FDG avid tumor is conspicuous. Li et al. in 2018 developed a computer-aided diagnosis model for pancreatic cancer in 18F-FDG PET/CT images (35). This model included 3 steps. First, a pancreas ROI was segmented using simple linear iterative clustering (SLIC) on CT. The second step was to extract pancreas features from CT and PET images by dual threshold principal component analysis (DT-PCA). The third step was to perform pancreatic cancer classification by hybrid feedback-support vector machine-random forest (HFP-SVM-RF) which would differentiate cancer from normal pancreas based on using different SVMs. The model was tested on 80 patients (40 with pancreatic cancer and 40 normal cases) with 18F-FDG PET/CT for identification of pancreatic cancer. Segmentation was tested on 82 cases from another dataset. The average accuracy for pancreatic cancer identification was 96.47%. Segmentation was tested on 82 publicly available scans from the National Institute of Health and the resultant Dice Index (DI) and Jaccard Index (JI) were 78.9% and 65.4%, respectively. DI and JI provide information about the similarity between computer-aided segmentation and ground truth contour.
In another article (in Chinese), the authors extracted the CT and PET features of patients with autoimmune pancreatitis and pancreatic ductal adenocarcinoma. An SVM classifier was developed that could distinguish autoimmune pancreatitis from cancer (34). Only the abstract of the study with English language was reviewed for this manuscript.
Breast cancer cells and bone metastatic disease
Ellmann et al. studied whether an ML model named model-averaged neural network (avNNET) could predict early metastatic disease in rats with bone metastases (33). MDA-MB-231 breast cancer cells were injected in the right superficial epigastric artery of 28 rats to evaluate bone metastases in the right hind leg. 18F-FDG PET/CT and MRI were acquired on days 0, 10, 20, and 30 after the injection. 18 rats developed metastatic disease at days 20 or 30. There was no discernable abnormality in the PET or MRI scans on day 10. Training was based on imaging features of 18F-FDG PET and MRI images on day 10. The model predicted macro-metastases with an accuracy of 85.7%.
Cervical cancer
In a recent study, Shen et al. included 142 cervical cancer patients with 18F-FDG PET/CT to develop a deep learning model for prediction of local relapse and distant metastases (38). VOIs were centered by SUVmax and cropped by dimensions of 7 cm x 7 cm x 7 cm on PET images. Data augmentation was utilized to compensate for the lack of sufficient training data. A k-fold cross-validation strategy was used. The deep learning model demonstrated an accuracy of 89% for predicting local recurrence and 87% for predicting distant metastases.
Soft tissue sarcoma
The 18F-FDG PET/CT images of 48 patients with pathology-proven soft tissue sarcoma from a publicly available dataset (24 with distant metastases and 24 without metastatic disease) were included in a study to develop a deep multi-modality collaborative learning (DMCL) model to predict distant metastases (39). The model provided higher accuracy, sensitivity, and AUC in comparison with other state-of-the-art models including single-modality PET CNN, and multi-modality CNN.
Uterine sarcoma
67 patients (11 with uterine sarcoma, 56 with leiomyomas) who had contrast-enhanced pelvic 3T MRI with DWI and 18F-FDG PET/CT within 2 months before surgery were included in the study (40). The goal of the study was to develop an ML method to distinguish between uterine sarcoma and benign leiomyoma. In patients with more than one lesion, the largest lesion was used for analysis. Logistic regression was used to develop the ML models. LR model based on SUVmax outperformed different univariate MRI models including LR models based on T2WI, mean ADC, and minimum ADC. Multivariate LR model with mean ADC and normalized T2WI signal performed better than the output based on SUVmax.
Studies not based on specific cancer subtype
Shaish et al. proposed whether a CNN could predict the SUVmax based on non-contrast CT images in PET/CT studies (37). A total of 2017 patients with pathologically proven malignancy were screened for lymph nodes. Two blinded radiologists selected one to ten lymph nodes from the non-contrast CT images. The SUVmax of the lymph nodes was recorded. 400 lymph nodes from 136 patients were utilized for training and 164 lymph nodes from 49 patients were used for testing. The non-contrast CT images and the pathology subtypes were used as input for the training. The developed CNN predicted the SUVmax with moderate accuracy.
A DL approach can perform attenuation correction in whole-body PET images without accompanying structural imaging (36). Attenuation correction is a major step in the post-acquisition processing of PET images. Structural imaging improves the attenuation correction and results in improved diagnostic value of PET images. Dong et al. used 25 whole-body PET images to train a deep learning cycle generative adversarial network (CycleGAN) to perform attenuation correction. The patients had different cancers including lung, head and neck, skin, breast, and lymphoma. Leave-one-out strategy was used. To create the training dataset, for each pair of non-attenuation corrected (NAC) and attenuation corrected (AC) PET images, 3D patches of NAC and AC PET were extracted. The AC PET patch was considered as the deep learning-based target of the NAC PET patch. After training, NAC PET images were fed to make DL-based attenuation correction (DL-AC) PET images. AC images were reconstructed by patch fusion. 55 whole-body PET/CT images were used for evaluation of the model. AC and DL-AC PET showed no significant difference in intensity changes. This method showed excellent quantification reliability and accuracy and can be used in single PET scans as well as hybrid PET/CT or PET/MRI studies.
Discussion and conclusions
Some limitations should be considered when using 18F-FDG PET in practice. The main limitation is its low specificity, which might be a challenge in distinguishing recurrence from inflammation/infection or active fibrosis in the post-surgical or post-radiation status. 18F-FDG PET is less sensitive in detecting small neoplasms or neoplasms with low metabolic rate (for example mucinous adenocarcinoma) and presence of high background tissue uptake (for instance brain and kidney) (3).
AI modeling based on 18F-FDG PET images is a major topic of interest with a growing number of relevant publications in recent years. AI models are valuable in detection, segmentation, post-therapeutic evaluation, pathology prediction, and survival estimation. Moreover, they can improve the quality of 18F-FDG PET imaging by improving attenuation correction regardless of the presence of accompanying structural imaging.
The routine implementation of AI-based models and 18F-FDG PET-derived radiomics in clinical practice is currently limited, due to lack of standardized, reproducible, generalizable, and precise techniques. Regarding the ML-based models, there are a few challenging steps still required to be achieved. Optimization of the ML models requires recruiting higher numbers of patients/images as input to the ML algorithms or using data augmentation methods. For this purpose, gathering reliable and valid databases could be a major challenge. Another major step would be to assess the institution-based ML models in other centers to evaluate the universal reliability and accuracy of these models. By improving the reliability and validity of the ML algorithms, in near future, we will hopefully see the integration of the ML-based models into the current report generation workflow to assist the radiologists/nuclear medicine physicians in creating high-quality reports.
Acknowledgments
Funding: None.
Footnote
Provenance and Peer Review: This article was commissioned by the editorial office, Annals of Translational Medicine for the series “Artificial Intelligence in Molecular Imaging”. The article has undergone external peer review.
Reporting Checklist: The authors have completed the PRISMA reporting checklist. Available at http://dx.doi.org/10.21037/atm-20-6162
Conflicts of Interest: All authors have completed the ICMJE uniform disclosure form (available at http://dx.doi.org/10.21037/atm-20-6162). The series “Artificial Intelligence in Molecular Imaging” was commissioned by the editorial office without any funding or sponsorship. SPR served as the unpaid Guest Editor of the series and serves as an unpaid editorial board member of Annals of Translational Medicine from Mar 2020 to Feb 2022. The authors have no other conflicts of interest to declare.
Ethical Statement: The authors are accountable for all aspects of the work in ensuring that questions related to the accuracy or integrity of any part of the work are appropriately investigated and resolved.
Open Access Statement: This is an Open Access article distributed in accordance with the Creative Commons Attribution-NonCommercial-NoDerivs 4.0 International License (CC BY-NC-ND 4.0), which permits the non-commercial replication and distribution of the article with the strict proviso that no changes or edits are made and the original work is properly cited (including links to both the formal publication through the relevant DOI and the license). See: https://creativecommons.org/licenses/by-nc-nd/4.0/.
References
- Kapoor V, McCook BM, Torok FS. An introduction to PET-CT imaging. Radiographics 2004;24:523-43. [Crossref] [PubMed]
- Weber WA. Quantitative analysis of PET studies. Radiother Oncol 2010;96:308-10. [Crossref] [PubMed]
- Almuhaideb A, Papathanasiou N, Bomanji J. 18F-FDG PET/CT imaging in oncology. Ann Saudi Med 2011;31:3-13. [Crossref] [PubMed]
- Lee JW, Lee SM. Radiomics in Oncological PET/CT: Clinical Applications. Nucl Med Mol Imaging 2018;52:170-89. [Crossref] [PubMed]
- Weidlich V, Weidlich GA. Artificial Intelligence in Medicine and Radiation Oncology. Cureus 2018;10:e2475 [Crossref] [PubMed]
- Russell S, Norvig P. Artificial intelligence: a modern approach. 4 ed. Pearson; 2002.
- Choy G, Khalilzadeh O, Michalski M, et al. Current Applications and Future Impact of Machine Learning in Radiology. Radiology 2018;288:318-28. [Crossref] [PubMed]
- LeCun Y, Bengio Y, Hinton G. Deep learning. Nature 2015;521:436-44. [Crossref] [PubMed]
- Alilou M, Orooji M, Beig N, et al. Quantitative vessel tortuosity: A potential CT imaging biomarker for distinguishing lung granulomas from adenocarcinomas. Sci Rep 2018;8:15290. [Crossref] [PubMed]
- Buizza G, Toma-Dasu I, Lazzeroni M, et al. Early tumor response prediction for lung cancer patients using novel longitudinal pattern features from sequential PET/CT image scans. Phys Med 2018;54:21-9. [Crossref] [PubMed]
- Chen S, Harmon S, Perk T, et al. Diagnostic classification of solitary pulmonary nodules using dual time (18)F-FDG PET/CT image texture features in granuloma-endemic regions. Sci Rep 2017;7:9370. [Crossref] [PubMed]
- Hyun SH, Ahn MS, Koh YW, et al. A Machine-Learning Approach Using PET-Based Radiomics to Predict the Histological Subtypes of Lung Cancer. Clin Nucl Med 2019;44:956-60. [Crossref] [PubMed]
- Ikushima K, Arimura H, Jin Z, et al. Computer-assisted framework for machine-learning-based delineation of GTV regions on datasets of planning CT and PET/CT images. J Radiat Res 2017;58:123-34. [Crossref] [PubMed]
- Kawata Y, Arimura H, Ikushima K, et al. Impact of pixel-based machine-learning techniques on automated frameworks for delineation of gross tumor volume regions for stereotactic body radiation therapy. Phys Med 2017;42:141-9. [Crossref] [PubMed]
- Kirienko M, Sollini M, Silvestri G, et al. Convolutional Neural Networks Promising in Lung Cancer T-Parameter Assessment on Baseline FDG-PET/CT. Contrast Media Mol Imaging 2018;2018:1382309 [Crossref] [PubMed]
- Ma Y, Feng W, Wu Z, et al. Intra-tumoural heterogeneity characterization through texture and colour analysis for differentiation of non-small cell lung carcinoma subtypes. Phys Med Biol 2018;63:165018 [Crossref] [PubMed]
- Schwyzer M, Ferraro DA, Muehlematter UJ, et al. Automated detection of lung cancer at ultralow dose PET/CT by deep neural networks - Initial results. Lung Cancer 2018;126:170-3. [Crossref] [PubMed]
- Scott JA, McDermott S, Kilcoyne A, et al. Comparison of (18)F-FDG avidity at PET of benign and malignant pure ground-glass opacities: a paradox? Part II: artificial neural network integration of the PET/CT characteristics of ground-glass opacities to predict their likelihood of malignancy. Clin Radiol 2019;74:692-6. [Crossref] [PubMed]
- Teramoto A, Fujita H, Yamamuro O, et al. Automated detection of pulmonary nodules in PET/CT images: Ensemble false-positive reduction using a convolutional neural network technique. Med Phys 2016;43:2821-7. [Crossref] [PubMed]
- Zhang R, Zhu L, Cai Z, et al. Potential feature exploration and model development based on 18F-FDG PET/CT images for differentiating benign and malignant lung lesions. Eur J Radiol 2019;121:108735 [Crossref] [PubMed]
- Zhao X, Li L, Lu W, et al. Tumor co-segmentation in PET/CT using multi-modality fully convolutional neural network. Phys Med Biol 2018;64:015011 [Crossref] [PubMed]
- Astaraki M, Wang C, Buizza G, et al. Early survival prediction in non-small cell lung cancer from PET/CT images using an intra-tumor partitioning method. Phys Med 2019;60:58-65. [Crossref] [PubMed]
- He J, Zhang JX, Chen CT, et al. The Relative Importance of Clinical and Socio-demographic Variables in Prognostic Prediction in Non-Small Cell Lung Cancer: A Variable Importance Approach. Med Care 2020;58:461-7. [Crossref] [PubMed]
- Wu J, Tan Y, Chen Z, et al. Decision based on big data research for non-small cell lung cancer in medical artificial system in developing country. Comput Methods Programs Biomed 2018;159:87-101. [Crossref] [PubMed]
- Zhong Z, Kim Y, Plichta K, et al. Simultaneous cosegmentation of tumors in PET-CT images using deep fully convolutional networks. Med Phys 2019;46:619-33. [Crossref] [PubMed]
- Guo Z, Guo N, Gong K, et al. Gross tumor volume segmentation for head and neck cancer radiotherapy using deep dense multi-modality network. Phys Med Biol 2019;64:205015 [Crossref] [PubMed]
- Huang B, Chen Z, Wu PM, et al. Fully Automated Delineation of Gross Tumor Volume for Head and Neck Cancer on PET-CT Using Deep Learning: A Dual-Center Study. Contrast Media Mol Imaging 2018;2018:8923028 [Crossref] [PubMed]
- Chen L, Zhou Z, Sher D, et al. Combining many-objective radiomics and 3D convolutional neural network through evidential reasoning to predict lymph node metastasis in head and neck cancer. Phys Med Biol 2019;64:075011 [Crossref] [PubMed]
- Zhou Z, Chen L, Sher D, et al. Predicting Lymph Node Metastasis in Head and Neck Cancer by Combining Many-objective Radiomics and 3-dimensioal Convolutional Neural Network through Evidential Reasoning. Annu Int Conf IEEE Eng Med Biol Soc 2018;2018:1-4. [Crossref] [PubMed]
- Parkinson C, Evans M, Guerrero-Urbano T, et al. Machine-learned target volume delineation of (18)F-FDG PET images after one cycle of induction chemotherapy. Phys Med 2019;61:85-93. [Crossref] [PubMed]
- Sadik M, Lind E, Polymeri E, et al. Automated quantification of reference levels in liver and mediastinal blood pool for the Deauville therapy response classification using FDG-PET/CT in Hodgkin and non-Hodgkin lymphomas. Clin Physiol Funct Imaging 2019;39:78-84. [Crossref] [PubMed]
- Bi L, Kim J, Kumar A, et al. Automatic detection and classification of regions of FDG uptake in whole-body PET-CT lymphoma studies. Comput Med Imaging Graph 2017;60:3-10. [Crossref] [PubMed]
- Ellmann S, Seyler L, Evers J, et al. Prediction of early metastatic disease in experimental breast cancer bone metastasis by combining PET/CT and MRI parameters to a Model-Averaged Neural Network. Bone 2019;120:254-61. [Crossref] [PubMed]
- Zhang Y, Cheng C, Liu Z, et al. Differentiation of autoimmune pancreatitis and pancreatic ductal adenocarcinoma based on multi-modality texture features in (18)F-FDG PET/CT. Sheng Wu Yi Xue Gong Cheng Xue Za Zhi 2019;36:755-62. [PubMed]
- Li S, Jiang H, Wang Z, et al. An effective computer aided diagnosis model for pancreas cancer on PET/CT images. Comput Methods Programs Biomed 2018;165:205-14. [Crossref] [PubMed]
- Dong X, Lei Y, Wang T, et al. Deep learning-based attenuation correction in the absence of structural information for whole-body positron emission tomography imaging. Phys Med Biol 2020;65:055011 [Crossref] [PubMed]
- Shaish H, Mutasa S, Makkar J, et al. Prediction of Lymph Node Maximum Standardized Uptake Value in Patients With Cancer Using a 3D Convolutional Neural Network: A Proof-of-Concept Study. AJR Am J Roentgenol 2019;212:238-44. [Crossref] [PubMed]
- Shen WC, Chen SW, Wu KC, et al. Prediction of local relapse and distant metastasis in patients with definitive chemoradiotherapy-treated cervical cancer by deep learning from [(18)F]-fluorodeoxyglucose positron emission tomography/computed tomography. Eur Radiol 2019;29:6741-9. [Crossref] [PubMed]
- Peng Y, Bi L, Guo Y, et al. Deep multi-modality collaborative learning for distant metastases predication in PET-CT soft-tissue sarcoma studies. Annu Int Conf IEEE Eng Med Biol Soc 2019;2019:3658-88. [Crossref] [PubMed]
- Nakagawa M, Nakaura T, Namimoto T, et al. A multiparametric MRI-based machine learning to distinguish between uterine sarcoma and benign leiomyoma: comparison with (18)F-FDG PET/CT. Clin Radiol 2019;74:167.e1-e7. [Crossref] [PubMed]
- Teramoto A, Adachi H, Tsujimoto M, et al., editors. Automated detection of lung tumors in PET/CT images using active contour filter. Medical Imaging 2015: Computer-Aided Diagnosis; 2015: International Society for Optics and Photonics.
- Beichel RR, Ulrich EJ, Smith BJ, et al. FDG PET based prediction of response in head and neck cancer treatment: Assessment of new quantitative imaging features. PLoS One 2019;14:e0215465 [Crossref] [PubMed]
- Sheikhbahaei S, Mena E, Pattanayak P, et al. Molecular Imaging and Precision Medicine: PET/Computed Tomography and Therapy Response Assessment in Oncology. PET Clin 2017;12:105-18. [Crossref] [PubMed]
- Cottereau AS, Nioche C, Dirand AS, et al. (18)F-FDG PET Dissemination Features in Diffuse Large B-Cell Lymphoma Are Predictive of Outcome. J Nucl Med 2020;61:40-5. [Crossref] [PubMed]
- Voltin CA, Mettler J, Grosse J, et al. FDG-PET Imaging for Hodgkin and Diffuse Large B-Cell Lymphoma-An Updated Overview. Cancers (Basel) 2020;12:601. [Crossref] [PubMed]