This article has an erratum available at: http://dx.doi.org/10.21037/atm-2021-6 the article has been update on 2021-06-03 at here.
Construction of a ceRNA immunoregulatory network related to the development of vascular dementia through a weighted gene coexpression network analysis
Introduction
Vascular dementia (VaD) is the second most common type of dementia. It is related to vascular disease, which leads to dysfunction and damage to the cerebrovascular system, including interruption of cerebral blood flow (CBF), brain damage, and eventual cognitive impairment and memory loss (1). In North America and Europe (2), VaD accounts for approximately 15% to 20% of dementia cases, while in Asia and developing countries this proportion has risen to approximately 30% (3). Essentially, VaD is caused by a decrease in the blood supply to the brain (4) and is due to partial or complete occlusion of cerebral perfusion, which is crucial for memory and cognitive processes. VaD reaches its peak in ischemic brain injury (5). In addition to vascular problems, risk factors such as diabetes, age, smoking, and alcohol cannot be ignored (6). Improving vascular endothelial dysfunction and restoring the increase in oxidative stress and neuroinflammation caused by hypoperfusion may provide a potential treatment strategy for preventing the cognitive dysfunction of VaD. From the morphological and structural point of view, the brain of VaD is mainly manifested as a tendency of brain atrophy, and VaD can lead to the decline of brain function, especially the comprehensive decline of intelligence-related functions, and the syndrome will decline to a certain degree. The current treatment for VaD is symptomatic rather than radical, as the number of known drug targets is insufficient to develop VaD therapies. Currently, no drug has been approved by the US FAD for VaD.
In the different forms of central nervous system (CNS) diseases, neuroimmune disorders are common phenomena (7). Recent research indicates that the immune system plays a vital role in VaD, and immune dysfunction has been shown to exist in VaD (8). The CD4+ CD25+ FoxP3 regulatory T cells present in VaD patients are significantly reduced (9), and monocytes and natural killer (NK) cells are reduced in VaD, but not in Alzheimer‘s disease (AD) (10). In addition, the immune response has recently been shown to be an important factor in promoting the development of cerebral small vessel disease, which also affects the progression of VaD (11).
High-throughput technology has recently gained attention, providing an excellent opportunity to identify VaD immune-related biomarkers (12). Long non-coding RNA (lncRNA) is the largest category of ncRNA with a length >200 nucleotides, but it is no significant protein coding potential (13). Although they are not involved in protein coding, growing evidence shows that lncRNAs have many biological and molecular functions, such as epigenetic regulation, signal transduction, cell differentiation, and immune regulation (14). MicroRNAs (miRNAs, a small family of non-coding RNAs that are important post-transcriptional regulators of gene expression) and lncRNAs are gene regulatory markers that regulate the translation of various proteins and biological processes in health and disease states and play an important role in neurological diseases (15). In 2011, the competitive endogenous RNA (ceRNA) hypothesis proposed attracted much attention (16). In this hypothesis, lncRNA transcripts act as ceRNAs or natural miRNA sponges. They communicate with each other and coregulate mRNAs by competing with shared miRNAs (17). Surprisingly, the amount of research on the association between lncRNAs and VaD is sparse (15).
In this study, we revealed for the first time the molecular mechanism of ceRNA immunoregulation during the progression of VaD. We constructed a VaD-related ceRNA network through a weighted gene coexpression analysis (WGCNA) method to ensure that the nodes were highly interconnected and that the network was highly reliable (18). Then, we performed an immune cell infiltration analysis, constructed a related ceRNA subnet and screened the key factors. Our findings may assist in screening VaD biomarker candidates and therapeutic targets. Our research process is shown in Figure 1.
Methods
Microarray data
From the National Center for Biotechnology Information (NCBI) Gene Expression Omnibus (GEO) database (http://www.ncbi.nlm.nih.gov/geo), which is a public functional genomics data repository, we retrieved and downloaded the VaD microarray expression profile data set (GSE122063 and GSE120584). GSE122063 data, which is the lncRNA and mRNA data set, was collected by researchers at the University of Michigan from the frontal and temporal cortex of VaD, AD, and non-dementia control groups. The total sample size was 136 cases, including 36 cases of VaD and 44 control cases. The data of GSE120584, which is the miRNA data set, was collected from serum samples by researchers at the National Center for Geriatrics in Japan. The sample size was 1,601 cases, including 91 cases of AD, 56 cases of VaD, and 288 control cases. The study was conducted in accordance with the Declaration of Helsinki (as revised in 2013).
Data preprocessing and the differential analysis
We downloaded GPL16699 and GPL21263 files from GEO. We transformed the probe into the corresponding gene symbol according to the annotation information. We used the “GEO2R” online analysis tool in the GEO database to analyze the difference between the two data sets and normalized them. For the mRNA in the chip, we set the threshold as an adjusted P value <0.05, and for the lncRNA and miRNA in the chip, the threshold was |log2FC| >0.5 and adjusted P value <0.05 (19).
The weighted gene coexpression network
We imported the gene expression matrix of the relevant VaD and control groups into the Image GP tool (http://www.ehbio.com/Cloud_Platform/front/#/) and constructed a coexpression network of the VaD and control groups’ differentially expressed genes through a WGCNA algorithm. We utilized WGCNA to construct a coexpression module, and screen disease candidate targets. The Pearson correlation coefficient was used to calculate the linear correlation between each gene pair. We utilized the power function to transform the matrix into a signed adjacency matrix, built a scale-free network, and then transformed it into a topological overlap matrix (TOM). Then, we performed hierarchical clustering of the highly co-expressed genes. Next, we used the dynamic tree cutting algorithm to cut the clustering tree branches to generate modules. The first principal component is the module eigengene (ME), which summarizes the gene expression overall level of each module. MEs are used to assess module-trait associations. Modular Significance (MS) and Gene Significance (GS) are used to calculate the expression patterns of modules related to disease traits. In this study, the dynamic tree cutting algorithm can be used to detect the module, and the minimum module size was 30 (20).
Module enrichment analysis
We used the Enrichr database (https://maayanlab.cloud/Enrichr/) to analyze each module of the WGCNA results using the Gene Ontology (GO) and Kyoto Encyclopedia of Genes and Genomes (KEGG) pathway analysis to study the function of related mRNAs. We then screened out the immune-related modules for the ceRNA network construction.
Construction of the lncRNA-miRNA-mRNA ceRNA network
According to the theory that lncRNAs act as miRNA sponges to influence miRNAs and further regulate mRNA expression, we constructed a ceRNA network. We used the starBase database (http://starbase.sysu.edu.cn/index.php) and the miRTarbase database (http://mirtarbase.mbc.nctu.edu.tw/php/index.php) (21) to search for mRNAs targeted by differential miRNAs. In order to improve the validity of the search results, we included miRNA-targeted mRNAs in all two databases, and then we cross-referenced these mRNAs with the mRNAs of the immune module and performed a correlation test to confirm that the miRNA and mRNA expressions were negatively correlated. We used the starBase (http://starbase.sysu.edu.cn/index.php) database to predict the lncRNAs regulated by differential miRNAs by taking the intersection with the differential lncRNAs obtained through the gene chip analysis, and then we performed a correlation test to confirm that the miRNA and mRNA expressions were negatively correlated. In the above process, we used the Cytoscape 3.7.2 visualization to construct a ceRNA network diagram.
mRNAs of ceRNA network Enrichment analysis
We used Enrichr to perform the GO and KEGG pathway enrichment analysis on mRNAs in this ceRNA network.
Immune infiltration analysis
ImmuCellAI (http://bioinfo.life.hust.edu.cn/web/ImmuCellAI/) is a method based on gene set characteristics and is used to accurately appraise the abundance of 18 T cell subgroups and 6 other important immune cells from gene microarray, including B cells, NK cells, and monocyte, macrophage, neutrophil and dendritic cells (DCs) (22). We used this tool to investigate the difference in immune cell infiltration between the VaD and control expression groups.
Construction of the macrophage-related ceRNA subnetwork
Pearson’s method was used to analyze the correlation coefficient between the value of the NKT cell infiltration and the expression value of mRNAs and lncRNAs (|R|>0.4, P<0.05) in the ceRNA network. Then we analyzed the correlation between the expression values of the lncRNAs and mRNAs in the results, and finally screened the NKT cell ceRNA sub-network construction with an R value >0.4.
Determination of the regulatory pathways of the key factors
After grouping according to the median expression value of key lncRNAs, we utilized gene set enrichment analysis (GSEA) to analysis the KEGG pathway and GO process of the gene microarray. The standardized enrichment score (NES) was acquired and utilized to identify the key pathways.
Statistical analysis
In this study, we used the online analysis tool GEO2R of the GEO database to analyze the difference of related chips, and used WGCNA to analyze the mRNAs matrix. Subsequently, we applied enrichment analysis to related mRNAs, and performed ImmuCellAI immune infiltration analysis on mRNAs. Finally, we used Pearson correlation analysis to analyze the ceRNA network, and finally performed GSEA analysis on the mRNA matrix.
Results
Differentially expressed mRNAs, lncRNAs, and miRNAs in VaD
We used the online analysis tool GEO2R of the GEO database to analyze the difference between the GSE122063 and GSE120584 data sets. After batch correction, we obtained 6,876 VaD-related differential mRNAs using an adjusted P value <0.05, of which 3,178 were upregulated and 3,698 were downregulated. In addition, we also obtained 94 differential miRNAs based on an adjusted P value <0.05 and |log2FC| >0.5, of which 89 were upregulated and 5 were downregulated (the related heat map and volcano map are shown in Figure 2A and B). We also obtained 1,895 differential lncRNAs, of which 925 were upregulated and 970 were downregulated (the related heat map and volcano map are shown in Figure 2C and D).
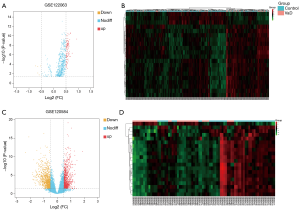
Coexpression module construction and immune module identification
We used the WGCNA algorithm to construct a coexpression network and modules for the VaD and control group samples. Based on the scale-free topological structure of R2=0.85, the Pearson correlation matrix of the gene was transformed into a strengthened adjacency matrix according to the power r=6 (Figure 3A,B). All selected genes were clustered using the dissimilarity measurement method based on the topological overlap matrix (TOM), and the tree was divided into six modules with different colors based on the dynamic tree cutting algorithm (Figure 3C). Then we calculated and plotted the relationship between each module and its corresponding clinical features (Figure 3D). We used the Enrichr database to perform the enrichment analysis of KEGG and GO for each module. The KEGG pathway mainly included staphylococcus aureus infection; leishmaniasis; inflammatory bowel disease (IBD); phagosome; and tuberculosis (Figure 4A). It can be seen that the blue module may be the key module of the immune response, and the blue module contains a total of 393 mRNAs. Through enrichment analysis, we found that the GO biological process of the blue module mainly included the cytokine-mediated signaling pathway (GO:0019221); neutrophil activation involved in immune response (GO:0002283); neutrophil degranulation (GO:0043312); neutrophil-mediated immunity (GO:0002446); and inflammatory response (GO:0006954) (Figure 4B).
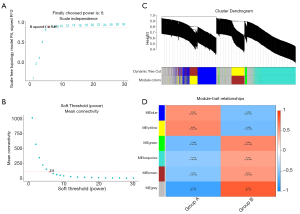
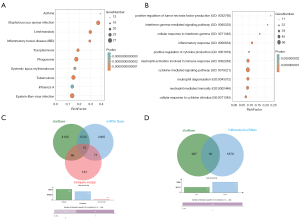
Construction of the lncRNA-miRNA-mRNA ceRNA network
We predicted the mRNAs regulated by differential miRNAs through the starBase and miRTarbase databases, including 8,001 in the starBase database and 6,336 in the miRTarbase database. We selected the mRNAs contained in the two databases and intersected the immune-related modules, and a total of 75 mRNAs were obtained (Figure 4C). In addition, we obtained 46 mRNAs after correlation analysis. We predicted the miRNA-related lncRNAs through the starBase database, and obtained a total of 983 lncRNAs, and then intersected these with the differential lncRNAs to get a total of 16 lncRNAs (Figure 4D). A total of nine lncRNAs were obtained after correlation analysis. We used Cytoscape 3.7.2 to construct the relevant ceRNA network (Figure 5A).
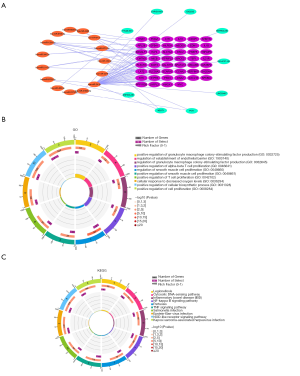
Enrichment analysis
We used the Enrichr database to perform the enrichment analysis of GO and KEGG for the mRNAs. Through enrichment analysis, we found that the GO biological process of the blue module mainly included regulation of smooth muscle cell proliferation (GO:0048660); positive regulation of cell proliferation (GO:0008284); positive regulation of smooth muscle cell proliferation (GO:0048661); regulation of establishment of endothelial barrier (GO:1903140); and positive regulation of granulocyte macrophage colony-stimulating factor production (GO:0032725) (Figure 5B). The KEGG pathway mainly included the NF-kappa B signaling pathway; Epstein-Barr virus infection; TNF signaling pathway; legionellosis; and the cytosolic DNA-sensing pathway (Figure 5C).
Immune cell abundance analysis
ImmuCellAI was used to estimate the abundance of 24 immune cells in the GSE cohort based on microarray data. Among them, CD4_naive, natural regulatory T (nTreg), iTreg, Th1, natural killer T (NKT) cells, mucosal-associated invariant T cells (MAIT), B cells, monocytes, macrophages, natural killer (NK) cells, neutrophils, and infiltration scores were significantly different between the VaD and control groups. Of these, the difference in NKT cells was the most significant, with the VaD group showing significantly less abundance than the control group (P=2.2e-8) (Figure 6A).
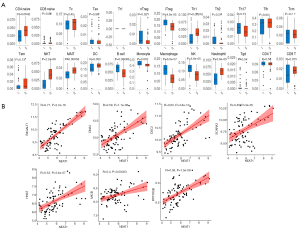
Construction of the NKT cell-related ceRNA sub-network
We used GSE122063 to identify key genes related to the ImmuCellAI infiltration score of the NKT cells. After correlation analysis, it was found that one lncRNA and 14 mRNA had a strong correlation with NKT cell infiltration (https://cdn.amegroups.cn/static/public/atm-21-1717-1.zip). The subsequent correlation analysis established the NKT cell ceRNA sub-network constructed with B4GALT1, PPP1R3B, MICB, HHAT, DSC2, DNA2, SCARA3, and lncRNA NEAT1 (Figure 6B), and indicated that lncRNA NEAT1 may be the key lncRNA identified in our research.
GSEA analysis
We applied GSEA analysis to GSE122063 to clarify the regulatory pathways of the key prognostic factors through median grouping of lncRNA NEAT1 (Figure 7A). It can be seen that these key factors are closely related to immunity, and surprisingly, compared with the lncRNA low expression group, we found that the significantly enriched signaling pathways included the NATURAL_KILLER_CELL, and TOLL_LIKE_RECEPTOR_SIGNALING_PATHWAY (Figure 7B), and GO analysis also shows that lncRNA is closely related to the immune process (Figure 7C).
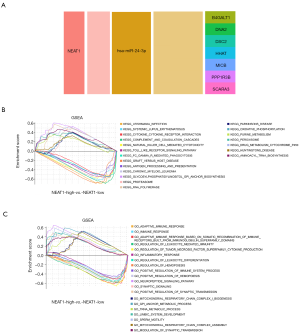
Discussion
VaD refers to a syndrome associated with clinical stroke or subclinical cerebrovascular injury, which affects at least one cognitive function. The disease is a heterogeneous encephalopathy, accounting for more than 20% of dementia cases (23). Studies have shown that effective treatment of VaD is more challenging than AD. The number of known drug targets is insufficient to develop VaD therapies (4). Therefore, the identification of new therapeutic factors is especially meaningful.
Immune regulation plays an important role in VaD. There is no research on VaD related to NKT cells. However, previous studies have shown that the mechanisms mediated by NKT cells are pro-inflammatory and immune regulatory, including the protective effect of B cell regulation. The production of sex antibodies, and the destructive effects of suppressing autoimmunity through cytokine production, DC crosstalk, and T-/B-cell interaction (24) is consistent with our immune infiltration results. In this study, we found a CeRNA immunoregulatory network in VaD. Then we established a CeRNA subnet related to NKT cells and identified several key factors, including B4GALT1, PPP1R3B, MICB, HHAT, DSC2, DNA2, and lncRNA NEAT1, which could be used as potential therapeutic targets for VaD.
As far as we know, this is the first study using a WGCNA method to construct a lncRNA-related ceRNA network in VaD, to construct its immune-related ceRNA, and to screen the key factors. We first determined the immune-related modules in the WGCNA results through enrichment analysis, and constructed the immune response-related ceRNA network, and then enriched the mRNAs in the network to confirm the regulatory function of the network. In the established NKT cell-related ceRNA sub-network, we found that lncRNA NEAT1 may be the key lncRNA for immune regulation. The research on human NEAT1 mainly focuses on its oncogene role in various cancers (25). One study has shown that lncRNA NEAT1 is an epigenetic inhibitor of hippocampal-dependent long-term memory formation and suggests a potential relationship with neuroinflammation (26). Another study showed that the expression of lncRNA NEAT1 was significantly upregulated in patients with ischemic stroke and aggravated cerebral ischemia-reperfusion (27). In addition, knockdown of lncRNA NEAT1 inhibits the activity of the pro-inflammatory AKT/STAT3 pathway, and significantly reduces the differentiation and apoptosis of microglia to the pro-inflammatory (M1) phenotype (28). Furthermore, the sponge mechanism of miR-24-3p can exacerbate cerebral ischemia-reperfusion injury (29).
Most of the mRNAs in the ceRNA subnetwork associated with NKT cells may also play an important role in VaD. B4GALT1 is a member of the b-1 4-galactosyltransferase gene (B4GALT) family and plays an important role in many biological events, including morphogenesis, mammalian fertilization, brain development, and cell adhesion (30). This gene may also play an important role in thrombosis. A recent study reported that the expression and activity of B4GALT1 mRNA in platelets were significantly increased, indicating that the gene was upregulated during late thrombosis and platelet release, thus positioning the gene as a regulatory gene for late thrombosis, possibly at the platelet release level (31). This suggests that the formation of thrombus may have an important influence on the progression of VaD. The protein phosphatase 1 regulatory subunit 3B gene (PPP1R3B) is located on chromosome 8p. The previous two GWASs indicate that PPP1R3B polymorphism is related to blood lipid traits. In animal studies, a high expression of PPP1R3B can affect blood lipid traits. In addition, it was found that PPP1R3B variants affect cardiovascular events and atherosclerosis (32), and these factors may cause vascular disease, which in turn may cause VaD. There are relatively few studies on the MICB gene related to VaD. The human genome encodes eight functional “stress-inducing ligands”: MICA, MICB, and ULBP1-6. All of these are recognized by a single receptor NKG2D, which is expressed on natural killer (NK) cells, cytotoxic T cells, and other T cell subpopulations (33). HHAT is an enzyme in the endoplasmic reticulum, which can palmitate hedgehog protein, and HHAT participates in the expression of the sonic hedgehog signaling pathway (34). Hedgehog is involved in almost all planned cell growth and survival mode of vertebrates, and plays an important role in the development of the forebrain and midbrain (35). Desmocollin 3 (DSC3) is a member of the desmocollin family (36), and DSC2 is a transmembrane protein that connects with the other three DSCs to ensure the integrity of myocardial structure and function and is also involved in cell signal transmission and apoptosis (37). The current research on DNA replication helicase/nuclease 2 (DNA2) is mainly focused on tumors, and there is currently no research on VaD. DNA2 is a mediator of genome stability and is required for proper Okazaki fragment maturation, for DSB repair, and for protection of replication forks. In addition, loss or mutation of DNA2 may inhibit DNA repair pathways in cells (38). Lipid metabolism plays an important role in vascular diseases such as atherosclerosis (39). A study has shown that high levels of receptor class A member 3 (SCARA3) methylation are associated with metabolic disorders, including type 2 diabetes and atherosclerosis, and SCARA3 may contribute to obesity and obesity related metabolic complications in an age-related manner (40). The potential role of these mRNAs in VaD needs to be further explored.
We also performed a GSEA analysis to determine the key regulatory pathways of lncRNA NEAT1. Natural killer (NK) cells are a type of cytotoxic lymphocyte that can infiltrate the ischemic brain and exacerbate neuronal cell death (41). Recent research indicates that NK cells play a key role in neurogenesis and cognitive decline during normal brain aging (42). It can be seen that lncRNAs may mediate the cytotoxicity of NK cells to aggravate the progression of VaD, and at the same time, the effect of NKT cells may be inhibited. Toll-like receptor (TLR) is an important pattern recognition receptor of the innate immune system, which triggers the inflammatory cascade by recognizing pathogens and related molecular patterns of damage (43). Toll-like receptor 2 (TLR2) and TLR4 are the two most important members of the TLR family. Previous studies have shown that TLR2 and TLR4 play a key role in inflammation after ischemic brain injury (44). Generally, TLR2 and TLR4 can be activated by a large number of exogenous and endogenous danger signal molecules, and ultimately promote the production of inflammatory cytokines, such as interleukin-6 (IL-6) and tumor necrosis factor-α (TNF-α) (45). Studies have shown that the TLR4 pathway induces the production of inflammatory cytokines, which leads to the development of cerebrovascular diseases during VaD (46). In addition, another study also showed that selective destruction of the TLR2-MyD88 interaction can inhibit inflammation in mice and improve their memory and learning ability (47). Therefore, reducing neuroinflammation by inhibiting the toll-like pathway may be an effective strategy for VaD.
In our research, for the first time, a ceRNA network related to a VaD immune response was constructed, and a sub-network related to NKT cells was constructed. However, our research still has certain limitations. We have not carried out any verification and mechanism experiments. In addition, there has been little research on the role of many of the mRNAs involved in VaD.
Conclusions
In conclusion, our research innovatively connects ceRNA to immunomodulation in the development of VaD. A decrease of NKT cells, and an increased expression of B4GALT1, PPP1R3B, MICB, HHAT, DSC2, DNA2, SCARA3, and lncRNA NEAT1 may have a significant correlation with the progression of VaD. These results will assist in a better understanding of the immunomodulatory mechanism of VaD from the perspective of ceRNA and may provide candidate therapeutic targets.
Acknowledgments
Funding: This research was funded by the National Natural Science Foundation of China (81800740) and the Natural Science Foundation of Shandong Province (ZR2020MH361).
Footnote
Conflicts of Interest: All authors have completed the ICMJE uniform disclosure form (available at http://dx.doi.org/10.21037/atm-21-1717). The authors have no conflicts of interest to declare.
Ethical Statement: The authors are accountable for all aspects of the work in ensuring that questions related to the accuracy or integrity of any part of the work are appropriately investigated and resolved. The study was conducted in accordance with the Declaration of Helsinki (as revised in 2013).
Open Access Statement: This is an Open Access article distributed in accordance with the Creative Commons Attribution-NonCommercial-NoDerivs 4.0 International License (CC BY-NC-ND 4.0), which permits the non-commercial replication and distribution of the article with the strict proviso that no changes or edits are made and the original work is properly cited (including links to both the formal publication through the relevant DOI and the license). See: https://creativecommons.org/licenses/by-nc-nd/4.0/.
References
- Poh L, Fann DY, Wong P, et al. AIM2 inflammasome mediates hallmark neuropathological alterations and cognitive impairment in a mouse model of vascular dementia. Mol Psychiatry 2020; Epub ahead of print. [Crossref] [PubMed]
- Wu YT, Fratiglioni L, Matthews FE, et al. Dementia in western Europe: epidemiological evidence and implications for policy making. Lancet Neurol 2016;15:116-24. Erratum in: Lancet Neurol 2016;15:135. [Crossref] [PubMed]
- Wolters FJ, Ikram MA. Epidemiology of Vascular Dementia. Arterioscler Thromb Vasc Biol 2019;39:1542-9. [Crossref] [PubMed]
- Shi H, Dong C, Wang M, et al. Exploring the mechanism of Yizhi Tongmai decoction in the treatment of vascular dementia through network pharmacology and molecular docking. Ann Transl Med 2021;9:164. [Crossref] [PubMed]
- Vijayan M, Reddy PH. Stroke, Vascular Dementia, and Alzheimer's Disease: Molecular Links. J Alzheimers Dis 2016;54:427-43. [Crossref] [PubMed]
- Li C, Wang Y, Yan XL, et al. Pathological changes in neurovascular units: Lessons from cases of vascular dementia. CNS Neurosci Ther 2021;27:17-25. [Crossref] [PubMed]
- Cacabelos R, Torrellas C, Fernández-Novoa L, et al. Histamine and Immune Biomarkers in CNS Disorders. Mediators Inflamm 2016;2016:1924603 [Crossref] [PubMed]
- Guo Z, Peng X, Li HY, et al. Evaluation of Peripheral Immune Dysregulation in Alzheimer's Disease and Vascular Dementia. J Alzheimers Dis 2019;71:1175-86. [Crossref] [PubMed]
- D'Angelo C, Goldeck D, Pawelec G, et al. Exploratory study on immune phenotypes in Alzheimer's disease and vascular dementia. Eur J Neurol 2020;27:1887-94. [Crossref] [PubMed]
- Busse M, Michler E, von Hoff F, et al. Alterations in the Peripheral Immune System in Dementia. J Alzheimers Dis 2017;58:1303-13. [Crossref] [PubMed]
- Fu Y, Yan Y. Emerging Role of Immunity in Cerebral Small Vessel Disease. Front Immunol 2018;9:67. [Crossref] [PubMed]
- Layton CJ, McMahon PL, Greenleaf WJ. Large-Scale, Quantitative Protein Assays on a High-Throughput DNA Sequencing Chip. Mol Cell 2019;73:1075-1082.e4. [Crossref] [PubMed]
- Pierce JB, Feinberg MW. Long Noncoding RNAs in Atherosclerosis and Vascular Injury: Pathobiology, Biomarkers, and Targets for Therapy. Arterioscler Thromb Vasc Biol 2020;40:2002-17. [Crossref] [PubMed]
- Mercer TR, Dinger ME, Mattick JS. Long non-coding RNAs: insights into functions. Nat Rev Genet 2009;10:155-9. [Crossref] [PubMed]
- Vijayan M, Reddy PH. Non-Coding RNAs Based Molecular Links in Type 2 Diabetes, Ischemic Stroke, and Vascular Dementia. J Alzheimers Dis 2020;75:353-83. [Crossref] [PubMed]
- Salmena L, Poliseno L, Tay Y, et al. A ceRNA hypothesis: the Rosetta Stone of a hidden RNA language? Cell 2011;146:353-8. [Crossref] [PubMed]
- Kim MS, Pinto SM, Getnet D, et al. A draft map of the human proteome. Nature 2014;509:575-81. [Crossref] [PubMed]
- Liu Y, Liu N, Liu Q. Constructing a ceRNA-immunoregulatory network associated with the development and prognosis of human atherosclerosis through weighted gene co-expression network analysis. Aging (Albany NY) 2021;13:3080-100. [Crossref] [PubMed]
- Xu M, Li Z, Yang L, et al. Elucidation of the Mechanisms and Molecular Targets of Sanhuang Xiexin Decoction for Type 2 Diabetes Mellitus Based on Network Pharmacology. Biomed Res Int 2020;2020:5848497 [Crossref] [PubMed]
- Yin X, Wang P, Yang T, et al. Identification of key modules and genes associated with breast cancer prognosis using WGCNA and ceRNA network analysis. Aging (Albany NY) 2020;13:2519-38. [Crossref] [PubMed]
- Riffo-Campos ÁL, Riquelme I, Brebi-Mieville P. Tools for Sequence-Based miRNA Target Prediction: What to Choose? Int J Mol Sci 2016;17:1987. [Crossref] [PubMed]
- Miao YR, Zhang Q, Lei Q, et al. ImmuCellAI: A Unique Method for Comprehensive T-Cell Subsets Abundance Prediction and its Application in Cancer Immunotherapy. Adv Sci (Weinh) 2020;7:1902880 [Crossref] [PubMed]
- Gorelick PB, Scuteri A, Black SE, et al. Vascular contributions to cognitive impairment and dementia: a statement for healthcare professionals from the American Heart Association/American Stroke Association. Stroke 2011;42:2672-713. [Crossref] [PubMed]
- Seidel A, Seidel CL, Weider M, et al. Influence of Natural Killer Cells and Natural Killer T Cells on Periodontal Disease: A Systematic Review of the Current Literature. Int J Mol Sci 2020;21:9766. [Crossref] [PubMed]
- Yu X, Li Z, Zheng H, et al. NEAT1: A novel cancer-related long non-coding RNA. Cell Prolif 2017;50:e12329 [Crossref] [PubMed]
- Butler AA, Johnston DR, Kaur S, et al. Long noncoding RNA NEAT1 mediates neuronal histone methylation and age-related memory impairment. Sci Signal 2019;12:eaaw9277 [Crossref] [PubMed]
- Shen S, Ma L, Shao F, et al. Long Non-Coding RNA (lncRNA) NEAT1 Aggravates Cerebral Ischemia-Reperfusion Injury by Suppressing the Inhibitory Effect of miR-214 on PTEN. Med Sci Monit 2020;26:e924781 [Crossref] [PubMed]
- Ni X, Su Q, Xia W, et al. Knockdown lncRNA NEAT1 regulates the activation of microglia and reduces AKT signaling and neuronal apoptosis after cerebral ischemic reperfusion. Sci Rep 2020;10:19658. [Crossref] [PubMed]
- Kuai F, Zhou L, Zhou J, et al. Long non-coding RNA THRIL inhibits miRNA-24-3p to upregulate neuropilin-1 to aggravate cerebral ischemia-reperfusion injury through regulating the nuclear factor κB p65 signaling. Aging (Albany NY) 2021;13:9071-84. [Crossref] [PubMed]
- Wang B, Wu S, Fang Y, et al. The AKR1C3/AR-V7 complex maintains CRPC tumour growth by repressing B4GALT1 expression. J Cell Mol Med 2020;24:12032-43. [Crossref] [PubMed]
- Giannini S, Lee-Sundlov MM, Rivadeneyra L, et al. β4GALT1 controls β1 integrin function to govern thrombopoiesis and hematopoietic stem cell homeostasis. Nat Commun 2020;11:356. [Crossref] [PubMed]
- Li WJ, Yin RX, Huang JH, et al. Association between the PPP1R3B polymorphisms and serum lipid traits, the risk of coronary artery disease and ischemic stroke in a southern Chinese Han population. Nutr Metab (Lond) 2018;15:27. [Crossref] [PubMed]
- Schmiedel D, Mandelboim O. NKG2D Ligands-Critical Targets for Cancer Immune Escape and Therapy. Front Immunol 2018;9:2040. [Crossref] [PubMed]
- Rallis A, Navarro JA, Rass M, et al. Hedgehog Signaling Modulates Glial Proteostasis and Lifespan. Cell Rep 2020;30:2627-2643.e5. [Crossref] [PubMed]
- Agha Z, Iqbal Z, Azam M, et al. Exome sequencing identifies three novel candidate genes implicated in intellectual disability. PLoS One 2014;9:e112687 [Crossref] [PubMed]
- Cui T, Yang L, Ma Y, et al. Desmocollin 3 has a tumor suppressive activity through inhibition of AKT pathway in colorectal cancer. Exp Cell Res 2019;378:124-30. [Crossref] [PubMed]
- Iyer VR, Chin AJ. Arrhythmogenic right ventricular cardiomyopathy/dysplasia (ARVC/D). Am J Med Genet C Semin Med Genet 2013;163C:185-97. [Crossref] [PubMed]
- Han Y, Zhang Z, Wang Z, et al. Increased Expression of DNA2 Was Linked to Poor Prognosis in Breast Cancer. Dis Markers 2021;2021:8860728 [Crossref] [PubMed]
- Zhang Z, Salisbury D, Sallam T. Long Noncoding RNAs in Atherosclerosis: JACC Review Topic of the Week. J Am Coll Cardiol 2018;72:2380-90. [Crossref] [PubMed]
- Peng H, Guo Q, Su T, et al. Identification of SCARA3 with potential roles in metabolic disorders. Aging (Albany NY) 2020;13:2149-67. [Crossref] [PubMed]
- Dou B, Li S, Wei L, et al. Astragaloside IV suppresses post-ischemic natural killer cell infiltration and activation in the brain: involvement of histone deacetylase inhibition. Front Med 2021;15:79-90. [Crossref] [PubMed]
- Jin WN, Shi K, He W, et al. Neuroblast senescence in the aged brain augments natural killer cell cytotoxicity leading to impaired neurogenesis and cognition. Nat Neurosci 2021;24:61-73. [Crossref] [PubMed]
- Aravalli RN, Peterson PK, Lokensgard JR. Toll-like receptors in defense and damage of the central nervous system. J Neuroimmune Pharmacol 2007;2:297-312. [Crossref] [PubMed]
- Wang YC, Lin S, Yang QW. Toll-like receptors in cerebral ischemic inflammatory injury. J Neuroinflammation 2011;8:134. [Crossref] [PubMed]
- Wang L, Yang JW, Lin LT, et al. Acupuncture Attenuates Inflammation in Microglia of Vascular Dementia Rats by Inhibiting miR-93-Mediated TLR4/MyD88/NF-κB Signaling Pathway. Oxid Med Cell Longev 2020;2020:8253904 [Crossref] [PubMed]
- Belkhelfa M, Beder N, Mouhoub D, et al. The involvement of neuroinflammation and necroptosis in the hippocampus during vascular dementia. J Neuroimmunol 2018;320:48-57. [Crossref] [PubMed]
- Rangasamy SB, Jana M, Roy A, et al. Selective disruption of TLR2-MyD88 interaction inhibits inflammation and attenuates Alzheimer's pathology. J Clin Invest 2018;128:4297-312. [Crossref] [PubMed]
(English Language Editor: D. Fitzgerald)