A nomogram for predicting brain metastasis in patients with de novo stage IV breast cancer
Introduction
Breast cancer tumors have the highest incidence of malignancy in women. The overall prognosis of patients with breast cancer in the United States is good, with a 5-year survival rate of 90.2% (1). However, the prognosis of metastatic breast cancer (MBC) is very poor, and brain metastasis (BM) is one of the distant metastatic sites with the worst prognosis. Although the incidence of BM in early breast cancer is less than 3% (2,3), the incidence in patients with MBC is as high as 10–30% (3-5), and the incidence in autopsy reports of patients with MBC exceeds 30% (6). Patients with BM have rapid disease progression, poor quality of life, high mortality, and few effective treatment options, resulting in a median survival time of only 4 weeks for untreated breast cancer patients with BM (6,7).
In recent years, remarkable progress has been made in the treatment of BM, novel targeted therapeutic drugs and locoregional treatment techniques have brought survival benefits to patients with BM, especially in human epidermal growth factor receptor 2 (HER2) positive breast cancer. Small molecule tyrosine kinase inhibitors (TKI) such as tucatinib (8,9) and neratinib (10-12), as well as novel antibody-drug conjugates such as trastuzumab emtansine (T-DM1) (13,14) and trastuzumab deruxtecan (15,16), have shown substantial intracranial efficacy against HER2-positive breast cancer. The application of new locoregional treatments, such as CyberKnife radiosurgery and stereotactic radiotherapy, has significantly improved the prognosis of patients with BM (17). The median survival time of breast cancer patients with BM treated with whole brain radiotherapy and stereotactic radiosurgery can be extended to 4–6 months, and if a single metastatic focus can be surgically removed, the median survival time can be extended to 16 months (6,7,18). Nevertheless, the overall prognosis of BM remains poor, mainly due to late detection. Therefore, early identification of patients with high-risk of BM is the key to improving their outcomes. Clinicians can perform routine laboratory or imaging screening for high-risk patients, which is helpful for early detection, early treatment and even preventive intervention, thus improving the prognosis of these patients.
However, no proven screening method has currently been established for BM. For screening BM in patients with early breast cancer, only routine central nervous system (CNS) symptoms follow up is recommended, no routine laboratory or imaging screening is recommended for patients in the absence of CNS symptoms due to a lack of research confirming associated survival advantages (19). This approach is even applicable to patients with advanced breast cancer, including metastatic HER2-positive and triple-negative breast cancer (TNBC) (20,21). Owing to the low incidence of BM in breast cancer, especially early breast cancer, regular imaging screening for all patients is not cost-effective, and the cumulative radiation is detrimental for the body. In view of this, MRI screening is needed only when patients have CNS symptoms, but a high proportion of patients do not have CNS symptoms in the early stage of BM (6), so the screening model recommended by the guidelines cannot distinguish BM in time. Therefore, researchers have tried to identify pathological and biological parameters associated with BM. However, no highly abundant and recurrent mutations have been proved to be associated with BM in breast cancer, even the ERBB2-activated breast cancer genetically engineered mouse models did not interrogate the presence of brain metastases (22). Previous studies have only found that MBC patients with HER2-positive breast cancer (23), TNBC (24,25), BRCA genes mutation (26,27), diagnosis at young age (28), lung metastasis (29), high histological grade (23), and high proliferative activity (30) are more likely to develop BM.
In our study, we incorporated a large number of MBC cases from the Surveillance, Epidemiology, and End Results (SEER) database with 2 goals in mind. The primary goal was to identify risk factors associated with BM, and the secondary goal was to establish a predictive model for BM and evaluate its performance by examining its correlation with survival and designing a preventive intervention trial. The predictive model established in this study was expected to provide a basis for BM screening and preventive treatment in patients with MBC.
We present the following article in accordance with the TRIPOD reporting checklist (available at http://dx.doi.org/10.21037/atm-21-1808).
Methods
Data source and inclusion criteria
The study was conducted in accordance with the Declaration of Helsinki (as revised in 2013). We searched the SEER database released in November 2018 which covers approximately 30% of the U.S. population from 18 registries (1973–2015). Informed consent is not required to extract SEER data because it does not provide identification information. The inclusion criteria were as follows: (I) primary malignant breast cancer diagnosed with distant metastasis from 2010 to 2015, patients before 2010 were excluded because their HER2 statuses were not recorded in the SEER database; (II) female, age at diagnosis ≥18; (III) histologically diagnosed as invasive ductal carcinoma (IDC), invasive lobular carcinoma (ILC), or IDC or ILC mixed with other types of carcinoma; (IV) breast cancer was the only primary malignant tumor; (V) patients who were not diagnosed by autopsy or death certificate; (VI) patients with complete clinicopathological data, those with missing data were excluded. The brain involvements of all patients were assessed at the time of initial diagnosis, so the patients had not received any form of treatment for breast cancer before. The evaluation of all the variables in the study was for the purpose of routine diagnosis and treatment, and the data were collected retrospectively when constructing the nomogram, therefore there was no obvious assessment bias. All the variable definitions in the study can be found in the SEER program coding and staging manual (https://seer.cancer.gov/tools/codingmanuals/). In our study, after excluding patients whose clinicopathological information was not available, we identified 7,154 patients, of whom 422 (5.9%) had clinical evidence of BM, which was regarded as an outcome event. Patients were randomly allocated to a training set and validation set according to the proportion of 7:3, the training set was used to establish the prediction model of BM, and the validation set was used for validation of the model.
Statistical analysis
Pearson chi-square test was used to evaluate the baseline characteristics and test the relationship between BM and clinicopathologic characteristics. Variables that were found to be statistically significant in the Pearson chi-square test were incorporated into multivariate logistic regression analysis. Significant variables in multivariate logistic regression were considered independent predictors of BM and were included in the final multivariate logistic regression model (nomogram).
The nomogram performance was evaluated from the aspects of discrimination, calibration, and clinical utility. The discrimination was quantified with the area under curve (AUC). The calibration was evaluated by the calibration curve using the bootstrapping method (1,000 repeats), which represented the relationship between observation frequencies and prediction probabilities. In order to evaluate the clinical utility of the nomogram, we defined the predictive risk of BM as a new variable (above/ below mean) and performed Cox regression analysis together with other confirmed independent prognostic factors of breast cancer (including liver involvement, surgery, chemotherapy, and so on). Multivariate cox regression analysis was aimed to reveal this comprehensive BM prediction risk as one of the most important variables for predicting survival outcomes. The clinical utility of this nomogram was also demonstrated by conducting a virtual trial in the validation set to prevent BM. We used the nomogram to calculate the individual risk of BM in the validation set and set different thresholds as intervention conditions. We evaluated the health economic value of our model in the prevention of breast cancer BM by estimating the number of patients who needed preventive intervention and the number of BM successfully prevented.
We compared the overall survival (OS) between two groups by Kaplan-Meier curves and log-rank test. All of the statistical methods in our study were performed using the statistical software SPSS version 23 (IBM Corp., Chicago, IL, USA) and R software version 3.6.3 (https://www.R-project.org/). All statistical analyses were 2-sided, and statistical significance was defined at P<0.05. All confidence intervals (CIs) were reported at the 95% confidence level.
Results
Demographic characteristics
Based on the inclusion criteria, we recruited a cohort of 7,154 patients with breast cancer with distant metastasis from the SEER database, among whom 422 patients (5.9%) had clinical evidence of BM. Table 1 shows the clinicopathologic characteristics of the entire cohort, as well as the training and validation sets which were randomly derived from the entire cohort at a 7:3 ratio. We used Pearson chi-square test for the training set and demonstrated that the status of brain involvement was related to variables including age at diagnosis (P=0.038), grade (P=0.043), histological type (P=0.027), tumor size (P=0.034), estrogen receptor (ER) status (P<0.001), progesterone receptor (PR) status (P<0.001), subtype (P<0.001), degree of liver involvement (P=0.004), and degree of lung involvement (P<0.001).
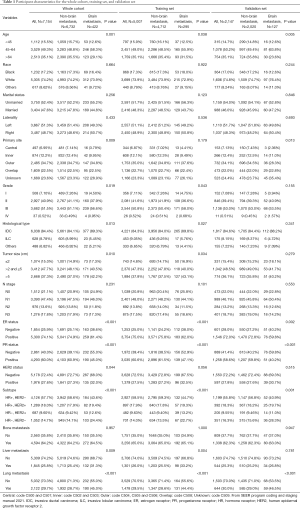
Full table
Multivariate logistic regression results
Variables that were found to be statistically significant in the Pearson chi-square test were incorporated into multivariate logistic regression analysis in Table 2. In multivariate logistic regression analysis, BM was independently associated with age at diagnosis, tumor size, subtype, and the degree of lung involvement.
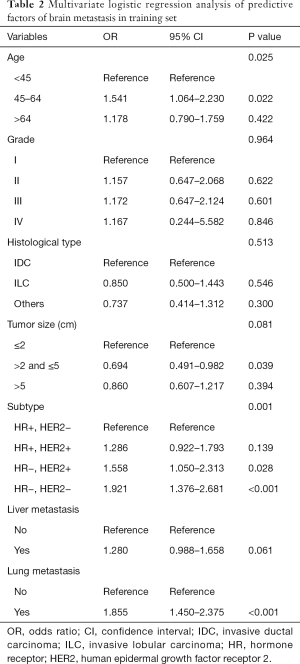
Full table
Nomogram construction
We used the results of the multivariate logistic regression in Table 2 to construct a nomogram to predict the risk of BM (Figure 1). By summing the scores of each variable, we can predict the probability of BM in a specific patient. Interestingly, the relationship between age and BM was neither a straightforward positive nor a negative correlation. The results showed that patients aged 45–64 had the highest risk of BM, followed by patients over 64 years old, and the risk was lowest for patients under 45 years old. Similarly, tumors smaller than 2 cm had the highest risk of BM, followed by tumors over 5 cm, and those sized >2 cm and ≤5 cm had the lowest risk.
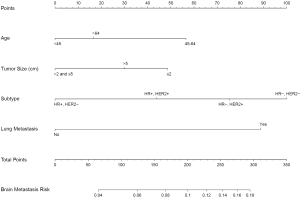
Nomogram performance
The nomogram performance at predicting BM was evaluated according to discrimination, calibration, and clinical utility. The discrimination was evaluated with the ROC curves of the training set (Figure 2A) and the validation set (Figure 2B). The nomogram had discriminatory ability with an area under curve (AUC) of 0.640 [95% confidence interval (CI): 0.607 to 0.673] in the training set, and 0.644 (95% CI: 0.595 to 0.693) in the validation set.
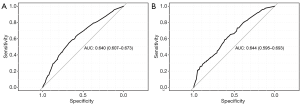
Then, we performed the calibration of the nomogram internally by 1,000 bootstrap resamples with a calibration plot in the training set (Figure 3). Both the bias-corrected curve and the apparent curve were close to the ideal curve, demonstrating that the nomogram fitted well internally.
To evaluate the clinical utility of the nomogram, we defined the predictive risk of BM as a new variable and performed Cox regression analysis together with other confirmed independent prognostic factors of breast cancer, including the degree of liver involvement, surgery, chemotherapy, and so on (Table 3). Multivariate cox regression analysis showed that the BM prediction risk obtained from the nomogram was one of the most significant variables in predicting OS [P<0.001, hazard ratio (HR): 1.499, 95% CI: 1.403 to 1.602].
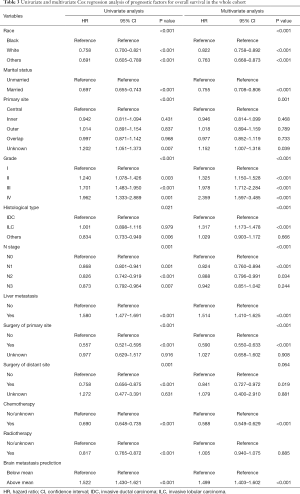
Full table
We then grouped participants according to the predicted risk of BM and observed significant differences between the two groups on the Kaplan-Meier curves (Figure 4, P<0.001). The results suggested that this predictive risk of BM could act as a prognostic indicator, participants with a higher predictive risk of BM had worse prognoses.
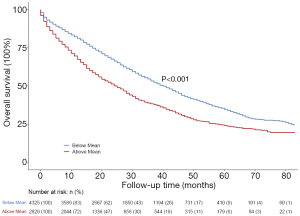
The clinical utility of this nomogram was also demonstrated by conducting a virtual trial in the validation set to prevent BM. Our nomogram could be used to identify a small subset of patients at high risk of BM to receive prophylactic intervention. We used the nomogram to predict individual risk of BM and set different thresholds as intervention conditions. Table 4 shows how we evaluated the health economic value of our model in preventing BM by estimating the number of participants who needed preventive intervention and the number of BM successfully prevented. If all patients were given prophylactic treatment without selection, although the prevention of BM could have been maximized, many patients would have been overtreated. If we only included patients with a predictive risk of BM >5% in our nomogram, only 54.3% (1,165/2,147) of the population would be treated, but 70.9% (90/127) of subsequent BM would be potentially prevented, with the actual BM rate decreasing to 46.5% of the original, with the assumption that prophylactic intervention could reduce the risk of BM by 75%. Table 4 displays several thresholds set for prophylactic treatment and the corresponding effects on the prevention of BM.
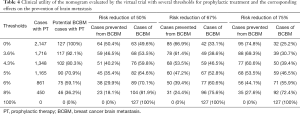
Full table
Discussion
BM is associated with declined quality of life caused by progressive neurologic impairment, which has been an increasingly serious problem in the treatment of breast cancer. In today's clinical practice, clinicians and researchers are increasingly interested in predictive models designed to predict the occurrence of clinical events or therapeutic effects. Nomogram is such a tool to predict personalized risk for patients and provide a basis for clinical decision-making. In our study, we summarized the specific clinicopathological characteristics of BM and developed a nomogram to predict BM in de novo stage IV breast cancer patients, thus helping clinicians identify groups at high-risk of BM and enabling the undertaking of early preventive interventions to improve their prognoses.
We have drawn some interesting conclusions from this nomogram. The most significant predictors in the nomogram were age, tumor size, subtype, and the degree of lung involvement. So far, the relationship between age and BM has been inconclusive. Some studies have indicated that younger patients experience a higher risk of developing BM (31-33). On the contrary, other studies have shown that aging increases the risk of BM (30,34). Different from these prior studies, the relationship between age and BM in our study was neither a straightforward positive nor negative correlation. The results showed that patients aged 45–64 had the highest risk of BM, followed by patients over 64 years old, and the lowest risk was at under 45 years old. The small number of young patients in the SEER database is a possible reason for this difference of age correlation with BM. Thus, it is necessary to carry out studies on larger populations in the future, especially in China, where young patients with breast cancer account for a higher proportion of the broader population (35). Nevertheless, our results were still supported by a previous large retrospective study which covered a population-based sample of 238,726 patients diagnosed with invasive breast cancer between 2010 and 2013 in US for whom the presence or absence of BM at diagnosis was known. Of the total of 238,726 patients, 5.68% of patients were aged 18–40, 41.13% were aged 41–60, 43.44% were aged 61–80, and 9.75% were older than 80. Consistent results were obtained in this study that patients between the ages of 41 and 60 had the highest risk of BM, followed by patients over the age of 60, and patients under the age of 41 (36). Tumor size was statistically an independent factor in our model, small tumors had the highest risk of BM, followed by large tumors, and intermediate-sized tumors had the lowest risk. This conclusion was controversial to common sense because many prior studies have demonstrated that progressively increasing tumor size was associated with an increased risk of BM (37,38). However, our conclusion was supported by the study of Tham et al., in which the tumor size was closely related to BM, but the risk of BM did not always increase or decrease with tumor size (30). In our study, patients with TNBC had the highest risk of BM, followed by HR−/HER2+, HR+/HER2+, and HR+/HER2− had the lowest risk. The association between subtype and BM has been extensively discussed in previous studies (31,32,36,38,39), which was consistent with the conclusion of our study. Besides, the results of our study showed that patients with lung metastasis had a higher risk of BM. However, patients with liver or bone metastasis were not associated with a significant increase in the probability of BM. Slimane et al. also drew the same conclusion in their study (29). The mechanisms and clinical implications behind this phenomenon require further investigation. As for the pathological type of breast cancer, among the existing studies on the risk factors of BM, only two studies have reported that pathological type is related to BM. Similar conclusions were drawn from these two retrospective studies, suggesting that IDC histology is associated with higher risk for BM (30,40). Consistent with most relative studies, our study has not found a significant relationship between pathological type and BM, so we believe that pathological type is not related to BM.
Our nomogram has the following clinical application value by the identification of BM high-risk patients. First, clinicians can screen brain MRI regularly for high-risk patients to achieve early diagnosis and treatment. Second, in the aspect of systematic treatment, appropriate treatment lines of anticancer drugs can be moved forward for high-risk patients to prevent BM, especially in HER2-positive breast cancer, due to the excellent efficacy of novel targeted drugs. Third, in the aspect of local treatment, prophylactic cranial irradiation (PCI) is currently recommended for patients with small-cell lung cancer due to the significant rate of occult BM, resulting in reduced incidence of BM and improved survival outcomes (41-43). Although no guidelines recommended PCI for breast cancer to prevent BM currently, future randomized clinical trials of PCI for BM prevention might be undertaken in selected patients since high-risk groups can be identified by our nomogram. In our study, the health economic value of this nomogram in predicting BM has already been demonstrated by estimating the number of patients who needed preventive intervention and the number of patients whose BM were successfully prevented.
Our study had some major strengths and prominent observations. First, this was the first exploration of establishing a prediction model of BM using the SEER database which included about 30% of the US population, the clinicopathological features of the patients with BM we described were highly generalized and may better reflect population experience than previous studies limited to data from a single cancer center. Second, previous studies using clinicopathological features of the whole breast cancer population to predict BM had great limitations, because these features overlap with factors that increase the risk of distant metastasis, such as high histological grade and tumor stage. Our study attempted to overcome this by incorporating de novo MBC patients from the SEER database to balance and eliminate the influence of distant metastasis-related features, and to summarize the specific clinicopathological characteristics related to BM.
We acknowledge some notable limitations in our study. First of all, SEER data do not contain follow-up information about recurrence and metastasis of breast cancer, so we could only describe whether patients had BM at the time of initial diagnosis, but could not include patients who developed BM at the later stage of the disease course. Second, since the guidelines do not recommend routine BM screening for patients without CNS symptoms, we might well have underestimated the actual BM rate in newly diagnosed breast cancer. Third, the probability provided by our nomogram using a logistic regression model was binary and not time-related because we only knew the metastasis status at diagnosis. Fourth, the performance of our predictive model (with an AUC of around 0.64) was reasonable, but not great. This was due to a lack of information in the SEER database, such as disease recurrence, subsequent sites of disease involvement, and the treatment before BM. In view of the fact that this was the first exploration of establishing a prediction model of BM using the SEER database, our nomogram would represent a good compromise. Fifth, since our study was a retrospective study, the conclusions raised still need to be further verified in prospective studies with a larger amount of data.
Acknowledgments
We gratefully acknowledge the efforts of the Surveillance, Epidemiology, and End Results (SEER) registries for the establishment and management of the database.
Funding: This study was supported by National Key R&D Program of China (2016YFC0901302) and Interdisciplinary Clinical Research Project of Peking University First Hospital (2019CR38).
Footnote
Reporting Checklist: The authors have completed the TRIPOD reporting checklist. Available at http://dx.doi.org/10.21037/atm-21-1808
Conflicts of Interest: All authors have completed the ICMJE uniform disclosure form (available at http://dx.doi.org/10.21037/atm-21-1808). The authors have no conflicts of interest to declare.
Ethical Statement: The authors are accountable for all aspects of the work in ensuring that questions related to the accuracy or integrity of any part of the work are appropriately investigated and resolved. All the procedures followed were in accordance with the Helsinki Declaration of the World Medical Association (as revised in 2013). The data released by the SEER database was publicly available and therefore did not require informed patient consent.
Open Access Statement: This is an Open Access article distributed in accordance with the Creative Commons Attribution-NonCommercial-NoDerivs 4.0 International License (CC BY-NC-ND 4.0), which permits the non-commercial replication and distribution of the article with the strict proviso that no changes or edits are made and the original work is properly cited (including links to both the formal publication through the relevant DOI and the license). See: https://creativecommons.org/licenses/by-nc-nd/4.0/.
References
- Allemani C, Matsuda T, Di Carlo V, et al. Global surveillance of trends in cancer survival 2000-14 (CONCORD-3): analysis of individual records for 37 513 025 patients diagnosed with one of 18 cancers from 322 population-based registries in 71 countries. Lancet 2018;391:1023-75. [Crossref] [PubMed]
- Arvold ND, Oh KS, Niemierko A, et al. Brain metastases after breast-conserving therapy and systemic therapy: incidence and characteristics by biologic subtype. Breast Cancer Res Treat 2012;136:153-60. [Crossref] [PubMed]
- Barnholtz-Sloan JS, Sloan AE, Davis FG, et al. Incidence proportions of brain metastases in patients diagnosed (1973 to 2001) in the Metropolitan Detroit Cancer Surveillance System. J Clin Oncol 2004;22:2865-72. [Crossref] [PubMed]
- Frisk G, Svensson T, Bäcklund LM, et al. Incidence and time trends of brain metastases admissions among breast cancer patients in Sweden. Br J Cancer 2012;106:1850-3. [Crossref] [PubMed]
- Smedby KE, Brandt L, Bäcklund ML, et al. Brain metastases admissions in Sweden between 1987 and 2006. Br J Cancer 2009;101:1919-24. [Crossref] [PubMed]
- Lin NU, Bellon JR, Winer EP. CNS metastases in breast cancer. J Clin Oncol 2004;22:3608-17. [Crossref] [PubMed]
- Klos KJ, O'Neill BP. Brain metastases. Neurologist 2004;10:31-46. [Crossref] [PubMed]
- Lin NU, Borges V, Anders C, et al. Intracranial Efficacy and Survival With Tucatinib Plus Trastuzumab and Capecitabine for Previously Treated HER2-Positive Breast Cancer With Brain Metastases in the HER2CLIMB Trial. J Clin Oncol 2020;38:2610-9. [Crossref] [PubMed]
- Murthy RK, Loi S, Okines A, et al. Tucatinib, Trastuzumab, and Capecitabine for HER2-Positive Metastatic Breast Cancer. N Engl J Med 2020;382:597-609. [Crossref] [PubMed]
- Awada A, Colomer R, Inoue K, et al. Neratinib Plus Paclitaxel vs Trastuzumab Plus Paclitaxel in Previously Untreated Metastatic ERBB2-Positive Breast Cancer: The NEfERT-T Randomized Clinical Trial. JAMA Oncol 2016;2:1557-64. [Crossref] [PubMed]
- Freedman RA, Gelman RS, Anders CK, et al. TBCRC 022: A Phase II Trial of Neratinib and Capecitabine for Patients With Human Epidermal Growth Factor Receptor 2-Positive Breast Cancer and Brain Metastases. J Clin Oncol 2019;37:1081-9. [Crossref] [PubMed]
- Saura C, Oliveira M, Feng YH, et al. Neratinib Plus Capecitabine Versus Lapatinib Plus Capecitabine in HER2-Positive Metastatic Breast Cancer Previously Treated With ≥ 2 HER2-Directed Regimens: Phase III NALA Trial. J Clin Oncol 2020;38:3138-49. [Crossref] [PubMed]
- Krop IE, Lin NU, Blackwell K, et al. Trastuzumab emtansine (T-DM1) versus lapatinib plus capecitabine in patients with HER2-positive metastatic breast cancer and central nervous system metastases: a retrospective, exploratory analysis in EMILIA. Ann Oncol 2015;26:113-9. [Crossref] [PubMed]
- Montemurro F, Delaloge S, Barrios CH, et al. Trastuzumab emtansine (T-DM1) in patients with HER2-positive metastatic breast cancer and brain metastases: exploratory final analysis of cohort 1 from KAMILLA, a single-arm phase IIIb clinical trial. Ann Oncol 2020;31:1350-8. [Crossref] [PubMed]
- Modi S, Park H, Murthy RK, et al. Antitumor Activity and Safety of Trastuzumab Deruxtecan in Patients With HER2-Low-Expressing Advanced Breast Cancer: Results From a Phase Ib Study. J Clin Oncol 2020;38:1887-96. [Crossref] [PubMed]
- Modi S, Saura C, Yamashita T, et al. Trastuzumab Deruxtecan in Previously Treated HER2-Positive Breast Cancer. N Engl J Med 2020;382:610-21. [Crossref] [PubMed]
- Palma DA, Olson R, Harrow S, et al. Stereotactic ablative radiotherapy versus standard of care palliative treatment in patients with oligometastatic cancers (SABR-COMET): a randomised, phase 2, open-label trial. Lancet 2019;393:2051-8. [Crossref] [PubMed]
- Andrews DW, Scott CB, Sperduto PW, et al. Whole brain radiation therapy with or without stereotactic radiosurgery boost for patients with one to three brain metastases: phase III results of the RTOG 9508 randomised trial. Lancet 2004;363:1665-72. [Crossref] [PubMed]
- Gradishar WJ, Anderson BO, Abraham J, et al. Breast Cancer, Version 3.2020, NCCN Clinical Practice Guidelines in Oncology. Journal of the National Comprehensive Cancer Network 2020;18:452-78. [Crossref] [PubMed]
- Cardoso F, Senkus E, Costa A, et al. 4th ESO-ESMO International Consensus Guidelines for Advanced Breast Cancer (ABC 4). Ann Oncol 2018;29:1634-57. [Crossref] [PubMed]
- Ramakrishna N, Temin S, Chandarlapaty S, et al. Recommendations on Disease Management for Patients With Advanced Human Epidermal Growth Factor Receptor 2-Positive Breast Cancer and Brain Metastases: ASCO Clinical Practice Guideline Update. J Clin Oncol 2018;36:2804-7. [Crossref] [PubMed]
- Schade B, Rao T, Dourdin N, et al. PTEN deficiency in a luminal ErbB-2 mouse model results in dramatic acceleration of mammary tumorigenesis and metastasis. J Biol Chem 2009;284:19018-26. [Crossref] [PubMed]
- Pestalozzi BC, Zahrieh D, Price KN, et al. Identifying breast cancer patients at risk for Central Nervous System (CNS) metastases in trials of the International Breast Cancer Study Group (IBCSG). Ann Oncol 2006;17:935-44. [Crossref] [PubMed]
- Lin NU, Claus E, Sohl J, et al. Sites of distant recurrence and clinical outcomes in patients with metastatic triple-negative breast cancer: high incidence of central nervous system metastases. Cancer 2008;113:2638-45. [Crossref] [PubMed]
- Yerushalmi R, Woods R, Kennecke H, et al. Patterns of relapse in breast cancer: changes over time. Breast Cancer Res Treat 2010;120:753-9. [Crossref] [PubMed]
- Albiges L, André F, Balleyguier C, et al. Spectrum of breast cancer metastasis in BRCA1 mutation carriers: highly increased incidence of brain metastases. Ann Oncol 2005;16:1846-7. [Crossref] [PubMed]
- Song Y, Barry WT, Seah DS, et al. Patterns of recurrence and metastasis in BRCA1/BRCA2-associated breast cancers. Cancer 2020;126:271-80. [Crossref] [PubMed]
- Evans AJ, James JJ, Cornford EJ, et al. Brain metastases from breast cancer: identification of a high-risk group. Clin Oncol (R Coll Radiol) 2004;16:345-9. [Crossref] [PubMed]
- Slimane K, Andre F, Delaloge S, et al. Risk factors for brain relapse in patients with metastatic breast cancer. Ann Oncol 2004;15:1640-4. [Crossref] [PubMed]
- Tham YL, Sexton K, Kramer R, et al. Primary breast cancer phenotypes associated with propensity for central nervous system metastases. Cancer 2006;107:696-704. [Crossref] [PubMed]
- Aversa C, Rossi V, Geuna E, et al. Metastatic breast cancer subtypes and central nervous system metastases. Breast 2014;23:623-8. [Crossref] [PubMed]
- Hung MH, Liu CY, Shiau CY, et al. Effect of age and biological subtype on the risk and timing of brain metastasis in breast cancer patients. PLoS One 2014;9:e89389 [Crossref] [PubMed]
- Xue J, Peng G, Yang JS, et al. Predictive factors of brain metastasis in patients with breast cancer. Med Oncol 2013;30:337. [Crossref] [PubMed]
- Graesslin O, Abdulkarim BS, Coutant C, et al. Nomogram to predict subsequent brain metastasis in patients with metastatic breast cancer. J Clin Oncol 2010;28:2032-7. [Crossref] [PubMed]
- Fan L, Strasser-Weippl K, Li JJ, et al. Breast cancer in China. Lancet Oncol 2014;15:e279-89. [Crossref] [PubMed]
- Martin AM, Cagney DN, Catalano PJ, et al. Brain Metastases in Newly Diagnosed Breast Cancer: A Population-Based Study. JAMA Oncol 2017;3:1069-77. [Crossref] [PubMed]
- Azim HA, Abdel-Malek R, Kassem L. Predicting Brain Metastasis in Breast Cancer Patients: Stage Versus Biology. Clin Breast Cancer 2018;18:e187-95. [Crossref] [PubMed]
- Kim YJ, Kim JS, Kim IA. Molecular subtype predicts incidence and prognosis of brain metastasis from breast cancer in SEER database. J Cancer Res Clin Oncol 2018;144:1803-16. [Crossref] [PubMed]
- Genre L, Roché H, Varela L, et al. External validation of a published nomogram for prediction of brain metastasis in patients with extra-cerebral metastatic breast cancer and risk regression analysis. Eur J Cancer 2017;72:200-9. [Crossref] [PubMed]
- Saip P, Cicin I, Eralp Y, et al. Identification of patients who may benefit from the prophylactic cranial radiotherapy among breast cancer patients with brain metastasis. J Neurooncol 2009;93:243-51. [Crossref] [PubMed]
- Al Feghali KA, Ballout RA, Khamis AM, et al. Prophylactic Cranial Irradiation in Patients With Non-Small-Cell Lung Cancer: A Systematic Review and Meta-Analysis of Randomized Controlled Trials. Front Oncol 2018;8:115. [Crossref] [PubMed]
- Maeng CH, Song JU, Shim SR, et al. The Role of Prophylactic Cranial Irradiation in Patients With Extensive Stage Small Cell Lung Cancer: A Systematic Review and Meta-Analysis. J Thorac Oncol 2018;13:840-8. [Crossref] [PubMed]
- Yin X, Yan D, Qiu M, et al. Prophylactic cranial irradiation in small cell lung cancer: a systematic review and meta-analysis. BMC Cancer 2019;19:95. [Crossref] [PubMed]
(English Language Editor: J. Jones)