The predictive prognostic values of CBFA2T3, STX3, DENR, EGLN1, FUT4, and PCDH7 in lung cancer
Introduction
Lung cancer is one of the most common cancers, especially in developed countries. However, diagnosing lung cancer is a challenge. Despite recent progress in diagnosis, classification and treatment, the overall survival rate is still very low (1). Most patients are diagnosed at the advanced stage, and have a poor prognosis. Indeed, the overall 5-year survival rate is 10–15% (2). In all stages of lung cancer, less than 7% of patients survived for 10 years after diagnosis. Late diagnosis and a lack of effective and personalized drugs reflect the need to better understand the mechanism of lung cancer progression (3). Using predictive biomarkers to identify tumors that respond to targeted therapy means a change in the diagnostic mode of lung cancer (4,5). At present, the potential molecular mechanism of lung cancer is unclear, which hinders the development of its prognosis and treatment strategy. Thus, new biomarkers or biological targets for lung cancer need to be identified urgently.
Tumor-related genes come from circulating cancer cells or directly from patients’ primary tumors via a process called ‘gene shedding’ (6). In recent years, the role of different lung cancer-related genes in lung cancer has not been widely explored. However, experimental evidence has shown that many lung cancer-related genes are involved in the pathogenesis and development of tumors (7). For example, in acute myeloid leukemia (AML), core-binding factor subunit alpha 2 to translocation 3 can inhibit retinoic acid receptors in many AML subtypes and patient samples. Thus, it is necessary and sufficient to downregulate CBFA2T3 to improve the expression and differentiation of myeloid genes induced by all-trans retinoic acid. CBFA2T3 can be used as a potential target to improve the responsiveness of AML to ATRA differentiation therapy (8). In bladder cancer, fucosyltransferase 4 (FUT4) is the target mRNA of microRNA (miR)-125a-5p. FUT4 can reverse the effect of miR-125a-5p on the progression of bladder cancer. Thus, miR-125a-5p inhibits the progression of bladder cancer by targeting FUT4 (9).
Research has shown that density-regulated protein (DENR) is highly expressed in many cancer types, and is related to the low survival rate of patients with hepatocellular carcinoma, gastric cancer, renal cancer, laryngeal cancer, and lung cancer. The high expression of DENR may contribute to the occurrence of tumors, and affect clinical prognoses (10). EglN1 is the main hypoxia-inducible factor α (HIF-α) prolyl hydroxylase in triple negative breast cancer (TNBC), which undergoes oxidative self-inactivation in biochemical analysis and cells in the absence of cysteine, which results in hypoxia-inducible factor 1α accumulation. HIF is a transcription factor that can promote the adaptation to hypoxia and stimulate the growth of TNBC (11). In oral squamous cell carcinoma (OSCC), gene-environment interactions of FUT2 polymorphisms with smoking and betel quid chewing habits may alter oral cancer susceptibility. A correlation has been found between FUT2 gene variation and OSCC risk (12). In castration resistant prostate cancer (CRPC), protocadherin 7 (PCDH7) is overexpressed in a large number of CRPC patients, and PCDH7 knockout reduces phosphorylation of extracellular receptor kinase (ERK), Akt kinase (AKT) and retinoblastoma, colony formation, cell invasion, and cell migration. It has been suggested that PCDH7 may be an attractive target in subgroups of CRPC patients (13). In gastric cancer, the levels of phosphorylated AKT and phosphorylated ERK1/2 were decreased by the silence of plant homeodomain (PHD) finger protein 14 (PHF14). The downregulation of PHF14 in gastric cancer cells inhibited colony formation in vitro and tumorigenesis in vivo. Thus, PHF14 could be used as a potential target for the treatment of gastric cancer (14). In humans, syntaxin 3 (STX3) is an important apical targeting protein in epithelial cell membrane and exocytosis, and is also a vesicle transporter of neutrophil receptor that plays a key role in protein transport. The residue exposed by crustacean cardioactive peptide (Ccap) should be subject to further mutation research, especially the mutation of Val286 of STX3 in humans (15). This method could be applied to STX3 drug design, which is a valuable target for cancer treatment.
This study used lung cancer data from The Cancer Genome Atlas (TCGA) and Gene Expression Omnibus (GEO) dataset to systematically analyze 8 lung cancer-related genes that have been widely reported. A bioinformatics analysis was undertaken to examine the role of lung cancer-related genes in the occurrence and development of lung cancer. Additionally, a univariate analysis, a multivariate regression analysis, and a ROC curve analysis were undertaken to explore their clinical prognostic value. More importantly, a gene set enrichment analysis (GSEA) and immune-infiltration analysis were used to determine the corresponding biological functions of high- and low-risk patients, which confirmed the significance of these markers as new biomarkers for lung cancer patients.
We present the following article in accordance with the MDAR reporting checklist (available at http://dx.doi.org/10.21037/atm-21-1392).
Methods
Data acquisition
Clinical information related to lung cancer and the RNA sequencing data of gene expression [for lung adenocarcinoma (LUAD)] were downloaded from the TCGA (https://gdc-portal.nci.nih.gov/), which includes 526 tumor specimens and 59 adjacent cancers. GSE31210 data were downloaded from the GEO (http://www.ncbi.nlm.nih.gov/geo/). The study was conducted in accordance with the Declaration of Helsinki (as revised in 2013).
Screening of research objects
In the TCGA dataset, the RDEseq2 and edge R package were used to calculate the p-value difference (16,17). The results of screening by any method are considered as differential genes (Padjust<0.05), that is, differential genes. In the GEO dataset, using the R ‘limma’ package, the P value was calculated using a moderated t-test method and student t-test method. In this study, the common differences between TCGA and GEO dataset were chosen as the follow-up research objects.
Survival analysis
To further determine which genes were significantly related to the survival of lung cancer, we first analyzed survival related genes in the TCGA tumor dataset by undertaking univariable Cox proportional hazards regression analysis with default parameter of R “survival” package, version 3.2-7 (18). The threshold was set to FDR (False Discovery Rate) <0.1 (the P value correction mode was Benjamini and Hochberg procedure) (19), and 491 genes were identified. The least absolute shrinkage and selection operator (LASSO) regression analysis was then used to undertake 300 calculations (20). Genes with a frequency ≥150 were selected as potential genes that were significantly related to survival. Finally, the above genes were verified by a univariate Cox proportional hazards regression analysis using the GEO data set. The threshold was set to FDR <0.1 (the P value correction mode was Benjamini and Hochberg procedure). Ultimately, 13 genes were identified. These 13 genes are considered to be significantly related to survival.
Risk model construction
The 13 selected genes were analyzed by a multivariate Cox regression model and a stepwise regression analysis was undertaken based on akaike information criterion (AIC) information statistics. The genes were eliminated by selecting the smallest AIC information statistics, and finally 8 molecules were selected for risk model construction. Then, Choose a model by AIC in a Stepwise Algorithm (21), the mode of stepwise search is “both”, and a risk formula was constructed according to Cox regression results. The following risk formula was constructed: –0.13 × CBFA2T3 + 0.5 × DENR + 0.28 × EGLN1 – 0.17 × FUT2 + 0.54 × FUT4 + 0.14 × PCDH7 + 0.26 × PHF14 – 0.77 × STX3.
Using this formula, the risk coefficient of each patient was calculated, and the patients were classified into to one of two groups; patients with a score lower than the median risk fell into the low-risk group, and those with a score higher than the median risk fell into the high-risk group.
GSEA
Next, the log2 difference multiples (high versus low) of the genes detected in the data was analyzed. The genes were sorted according to the multiple of log2 difference (from large to small). The database used for the GSEA was the Kyoto Encyclopedia of Genes and Genomes data set (the data was obtained from https://www.gsea-msigdb.org/gsea/msigdb). A pathway with a Padj (p-adjusted) <0.05 and a normalized enrichment scores (NES) absolute value ≥1 in the screening results was considered to be an enriched pathway (22,23). The first 6 of pathways were selected (and sorted from small to large based on the P value) to make the distribution curve of the enrich score.
Immuno-infiltration analysis
To analyze the difference of the immune cell ratio between high- and low-expression risk status samples, the immune cell infiltration of the TCGA-LUAD data set was analyzed by both Timer (tumor immune estimation resource; https://cistrome.shinyapps.io/timer) (24) and Cibersort (https://cibersort.stanford.edu/) (25), respectively. Timer was used to analyze the tumor-infiltrating immune cells, and the correlations between the infiltrating level of different subsets of immune cells and high- and low- risk status samples. Cibersort was used for estimating the abundance of different immune cell types in tumor microenvironment by FPKM data (26). The Wilcox test was used to test the cell-ratio difference between the two groups.
Statistical analysis
To evaluate the prediction accuracy of the risk scoring model, the receiver operating characteristics (ROC) curve was drawn, and the area under the curve (AUC) was calculated. A univariate analysis and a multivariate Cox regression analysis were used to analyze the survival of potential prognostic factors, such as age, fender, risk status in the TCGA and GEO data sets.
Results
Screening genes related to lung cancer survival
To explore the relationship between lung cancer-related genes and survival, based on the common differential genes in the two datasets, a univariable Cox proportional hazards regression analysis and a Least Absolute Shrinkage and Selection Operator (LASSO) analysis were used to further screen them. The following 28 genes with a frequency ≥150 were selected as potential survival significantly related genes: ASB1, FAM189A2, FUT1, FUT2, FUT4, HGSNAT, HOXA7, MS4A1, MYO6, P2RX1, PAX5, PCDH7, PTX3, RHOQ, SFXN1, SHC1, STX3, TLE1, EGLN1, MYLIP, OR7E47P, CBFA2T3, ASCC1, DARS2, IPO9, PHF14, DENR, and GRPEL1 (see Figure 1A). A univariable Cox proportional hazards regression analysis was then undertaken to verify these 28 genes in the GEO data set. By setting a threshold, the following 13 genes significantly related to survival were finally screened out: ASB1, CBFA2T3, DENR, EGLN1, FAM189A2, FUT1, FUT2, FUT4, MYLIP, PCDH7, PHF14, and STX3 (see Figure 1B). Subsequent research on lung cancer markers was based on these 13 genes.
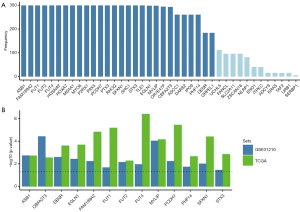
Expression level of 13 survival-related genes in the tumor and normal groups
In view of the role of these 13 survival-related genes in the occurrence and development of lung cancer, their expression levels in tumor and normal groups were analyzed in the TCGA and GEO data sets (see Figure 2). The results obtained from the two data sets exhibited the same trend, and the upregulated genes in lung cancer were mainly FUT2, SFXN1, DENR, PCDH7, PHF14, EGLN1, and FUT4, while the downregulated genes were mainly FAM189A2, CBFA2T3, MYLIP, FUT1, STX3, and ASB1. Notably, the difference between FUT2 and FAM189A2 was the most significant.
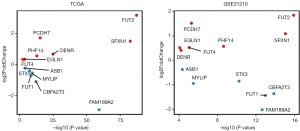
Prognostic value of lung cancer markers
To study the prognostic value of lung cancer markers, the aforementioned 13 genes were first analyzed by a multivariate Cox regression model. 8 risk genes were then screened out by a stepwise regression analysis (see Table 1). Next, we divided patients into either the low- or high-risk group according to the median risk in the TCGA and GEO data sets, and drew survival curves (see Figure 3A,B). As Figure 3A,B shows, low-risk patients had high survival rates. We then conducted univariate and multivariate regression analyses using the TCGA and GEO data sets to analyze survival in relation to age, gender, and risk status (see Tables 2-5). The analysis showed that whether due to a single factor or multiple factors, the risk situation was related to survival. The results also indicated that risk status may be an independent prognostic factor. To analyze the predictive value of the risk score for lung cancer prognosis, we made time-dependent ROC curves based on risk scores of 8 genes in the TCGA and GEO data sets and calculated the AUC (see Figure 3C,D). In the TCGA data set, we found that the AUC of ROC curves of the prognosis model at 1, 3, and 5 years were 0.756, 0.756, and 0.761, respectively. In the GEO data set, we found that AUC of ROC curves of the prognosis model at 1, 3 and 5 years were 0.802, 0.74, and 0.817, respectively. Thus, in the two data sets, the 1-, 3- and 5-year risk scoring models had high predictive power. Generally speaking, if the AUC value was more than 0.75, then the predicted value was relatively high.
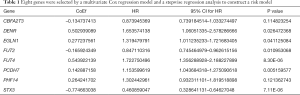
Full table
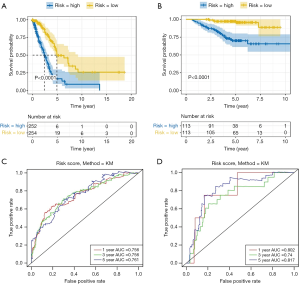
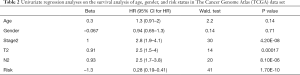
Full table
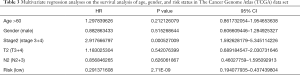
Full table

Full table

Full table
Identification and analysis of the risk genes related to lung cancer prognoses
To explore the significance of each risk gene in relation to the survival time of the lung cancer patients from the TCGA and GEO data sets, the samples were divided into high- and low-risk groups according to whether the median value of gene expression was lower than or higher than the median value of overall gene expression (see Figure 4A,B). As Figure 4A,B shows, in the TCGA data set, patients with a high expression of CBFA2T3 and STX3 had high survival rates, while patients with a high expression of DENR, EGLN1, FUT4, and PCDH7 had low survival rates. Similarly, in the GEO data set, patients with a high expression of CBFA2T3 and STX3 had high survival rates, while patients with high expression of DENR, EGLN1, FUT4, and PCDH7 had low survival rates. Thus, CBFA2T3 and STX3 appear be protective lung cancer genes, while DENR, EGLN1, FUT4 and PCDH7 appear to be dangerous genes. These 6 genes can be used as lung cancer markers to evaluate the prognosis of lung cancer.
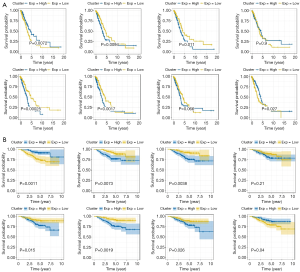
Exploring the involved signal pathways through a GSEA
A GSEA was undertaken to gain a better understanding of the corresponding biological functions of high- and low-risk patients. The results showed that linoleic acid metabolism and metabolism of xenobiotics by the cytochrome P450 pathway were downregulated in high-risk samples, while the p53 signaling pathway, oocyte meiosis, deoxyribonucleic acid (DNA) replication, homologous recombination, and the cell cycle pathway were upregulated in high-risk samples (see Figure 5A). We also selected the first 6 pathways (sorted from small to large based on the P values) to make the distribution curve of the enrich score (see Figure 5B). As Figure 5B shows, genes in the pathway of linoleic acid metabolism and xenobiotic metabolism by cytochrome P450 tended to be more concentrated in the low-expression region, while genes in the p53 signaling pathway, Oocyte meiosis, DNA replication, and homologous recombination tended to be more concentrated in the high expression region. Thus, the results indicated that the corresponding biological functions of high-risk patients are mostly related to tumor proliferation.
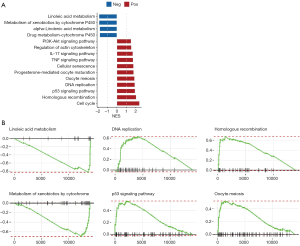
An analysis of immune cell infiltration with Timer and Cibersort
To analyze the immune cell ratio between high- and low-expression samples of risk status, we used Timer and Cibersort to analyze immune cell infiltration (see Figure 6A,B). Together, the Timer and Cibersort results showed that the high-risk status sample was biased toward the high abundance of most immune cells. The expressions of B cell, B plasma, and Mast-activated cells were low in the high-risk status samples, whereas Neutrophil, CD8+ T, Macrophage M0, Macrophage M1, and mDC-activated cells showed opposite results.
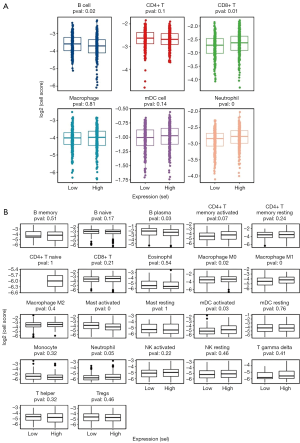
Discussion
Lung cancer has one of the highest tumor morbidity and mortality rates in the world; however, its pathogenesis is very complex and remains unclear (27,28). Previous studies have shown that the upregulation or downregulation of many genes is related to the occurrence and development of lung cancer. For example, the methylation of RASSF1A, CDKN2A, and DLEC1 is only found in lung cancer patients, while the methylation of CACA, CDH13, PITX2, HOXA9, and WT1 is found in both lung cancer and non-lung cancer patients (29). Nine genes (i.e., HMMR, B4GALT1, SLC16A3, ANGPTL4, EXT1, GPC1, RBCK1, SOD1, and AGRN) are considered to be related to poor prognosis and metastasis among LUAD patients (30). Further, some studies have found that there are differences in the expression of m6A-related genes in LUAD, and that the m6A-related genes of METTL3, YTHDF1 and YTHDF2 can be used as new biomarkers for the prognosis of LUAD (31). In this study, we focused on the relationship between 8 lung cancer-related genes and the occurrence and development of lung cancer. We found that they are closely related to the malignant degree and prognosis of lung cancer. Thus, our research has further improved understandings of the mechanism of the occurrence and development of lung cancer.
To explore the relationship between lung cancer-related genes and survival, we screened genes related to lung cancer survival. A follow-up study was then conducted of the 13 genes that we identified as being related to lung cancer survival. First, we analyzed their expression levels in tumor and normal groups using the TCGA and GEO data sets. Next, we selected 8 risk genes by a multivariate Cox regression model analysis and stepwise regression analysis to construct a risk model. Finally, we examined whether risk status was an independent prognostic factor by undertaking univariate and multivariate regression analyses. In addition, we used a ROC curve to analyze the predictive value of risk scores on the prognosis of lung cancer. We calculated the AUC, and found that the predictive value is still relatively high. We then identified and analyzed the risk genes related to prognosis in lung cancer. According to the results, it appears that CBFA2T3 and STX3 are the protective genes of lung cancer, while DENR, EGLN1, FUT4, and PCDH7 are the risk genes. Finally, we analyzed the biological function by a GSEA and immune-infiltration analysis, and confirmed their significance as new biomarkers for lung cancer patients.
CBFA2T3 and STX3 can promote the occurrence and development of tumors in many cancers. For example, the CBFA2T3/ACSF3 locus is a new recurrent carcinogenic target of immunoglobulin heavy chain locus (IGH) translocation that may be involved in the pathogenesis of GC-derived b-cell lymphoma in children (32). The expression of CBFA2T3-GLIS2 in drosophila and mouse hematopoietic cells induced bone morphogenetic protein signal transduction, and led to a significant improvement in the self-renewal ability of hematopoietic progenitor cells, which indicated that the expression of CBFA2T3-GLIS2 was directly involved in the occurrence of AMKL (33). In addition, STX3 promotes the growth of breast cancer cells by regulating the PTEN-PI3K-Akt-mTOR signaling pathway. The upregulation of STX3 is related to the malignant stage of breast cancer patients, and can predict the overall survival rate and disease-free survival rate of breast cancer patients (34). The formation of lateral basal areas and inclusion bodies in intestinal cells by microvilli dislocation is a pathological feature of congenital bowel disease, which is related to the mutation of apical plasma membrane receptor binding protein 3 (STX3) (35).
However, in lung cancer, we found that CBFA2T3 and STX3 were both protective genes, and their high expression promotes the survival of lung cancer patients. According to reports, CBFA2T3 and STX3 play inhibitory roles in many cancers. For example, CBFA2T3 was proved to be a transcription inhibitor when it was linked to GAL4-DNA binding domain, which indicates that CBFA2T3 may be a candidate gene of the breast cancer tumor suppressor gene, which is a common target gene of 16q24 LOH in breast cancer (36). RUNX1-CBFA2T3 has a good prognosis in childhood acute myeloid leukemia, and patients with RUNX1-CBFA2T3-rearrangement AML may benefit from the risk stratification of standard intensive treatment (37). In addition, the expression of BDNF, STXBP2, STX3, TGFB1, and CHAT was downregulated in a human neuroblastoma SH-SY5Y cell model (38).
Conversely, we found that DENR, EGLN1, FUT4, and PCDH7 were risk genes in lung cancer. Their high expression inhibits the survival of lung cancer patients. It has been shown that DENR gene encodes density regulatory protein, which acts on translation initiation together with multiple copies of oncogene (39). Many key ribosome binding proteins are important in cancer and participate in re-initiation, including the eukaryotic translation initiation factor 2D (eIF2D) or MCT-1/DENR homologous complex (40). Cancer cells are exposed to many pressures and need ATF4 to survive and proliferate. We found that there is a strong correlation between DENR•MCTS1 expression and ATF4 activity in cancer (41).
The mechanism of EGLN1 in different cancers differs. For example, in clear cell ovarian cancers, the knockout of EGLN1 encoding prolyl hydroxylase domain protein 2 (PHD2) was found to reduce the proliferation of some clear cell ovarian cancer cell lines. EGLN1 is a potential therapeutic target for patients with clear cell ovarian cancers (42). EGLN1 can be used as a prognostic biomarker in gynecological cancer, and, the imbalance of EGLN1 in cervical squamous cell carcinoma (CESC) is related to OS (Overall survival)time, which has been identified as the central gene of cancer progression (43). However, in clear cell renal cell carcinomas (ccRCCs), EGLN1 can mediate the degradation of SFMBT1, and the deletion of SFMBT1 inhibits the proliferation of CCRCC cells in vitro and the growth of tumor in situ in vivo (44). EGLN1 is a member of prolyl hydroxylase that can promote the degradation of HIF-1 by hydroxylation and ubiquitination. Introducing wild-type EGLN1 into endometrial cancer cell lines (e.g., HHUA, Ishikawa, and HWCA) with EGLN1 gene mutation can induce aging. EGLN1 can be used as a candidate tumor suppressor gene of chr. 1q (45).
The role of FUT4 in the occurrence and development of cancer has also been reported in the literature. For example, in AML, the miR-29b/Sp1/FUT4 axis regulates fucosylated CD44 through Wnt/β-catenin pathway, which promotes the malignant behavior of LSCs. Identifying LSCs surface markers and targeting LSCs are of great significance to the development of potential treatment methods for AML (46). In colorectal cancer, MALAT1 increases the expression of FUT4 through miR-26a/26b, and the MALAT1/miR-26a/26b/FUT4 axis plays an important role in the development of colorectal cancer mediated by exosomes (47).
PCDH7 is a transmembrane receptor and belongs to the cadherin superfamily (48). It plays different roles in different tumors. For example, in breast cancer, the overexpression of PCDH7 promotes the proliferation and invasion of breast cancer cells in vitro and the formation of bone metastasis in vivo (49). In colon cancer, LNAPPCC plays a carcinogenic role by forming a positive feedback loop with PCDH7. Targeting LNAPPCC/EZH2/PCDH7/ERK/c-FOS signal axis is a potential treatment strategy for colon cancer (50). However, in gastric cancer, the knockout of tumor suppressor gene PCDH7 in PRMT6-KO-GC cells promotes cell migration and invasion. Gastric cancer cells overexpressing PRMT6 may increase invasiveness by directly repressing PCDH7 by increasing the H3R2me2as level (51). In androgen-independent prostate cancer (AIPC), androgen receptor targets PCDH7, and its hypermethylation may inhibit the growth and invasion of AIPC cells and promote apoptosis. This study provides a new target for the treatment of AIPC (52).
FUT2 does not appear to have a significant relationship with the survival of lung cancer patients; however, it has been reported that FUT2 induces fucosylation in airway epithelium of asthma patients, which partly aggravates airway inflammation through the production of C3a and the aggregation of monocyte-derived dendritic cells in lung (53). The FUT2 genotype was significantly correlated with the prognosis of patients with non-cystic fibrosis bronchiectasis, and the homozygous secretor showed low lung function, increased aggravation times, and increased infection frequency of Pseudomonas aeruginosa (54). In addition, in breast cancer, the overexpression of FUT2 increases the migration and invasion of cells in vitro and the metastasis of breast cancer in vivo. FUT2 plays an important role in regulating growth, adhesion and migration, and has the characteristics of cancer stem cells, which may be a therapeutic target for breast cancer (55). The relationship between FUT2 and lung cancer should be further examined.
In sum, this study sought to identify the expression, potential function, and prognostic value of lung cancer-related genes in lung cancer. Risk genes may contribute to the personalized prediction of lung cancer prognosis. Further, as potential biomarkers, risk genes reflect the response of lung cancer patients to specific targeted therapies of lung cancer markers. The further study of these genes could fully reveal the potential association between lung cancer-related genes and the prognosis of lung cancer. This study also highlighted the important role of lung cancer-related genes in the occurrence and development of lung cancer, and provided potential guidance in relation to biomarkers for the selection of treatment methods.
Conclusions
This study comprehensively analyzed the relationship between the expression of lung cancer-related genes and the occurrence, development, and prognosis of lung cancer. In lung cancer-related genes, the abnormal expression of CBFA2T3, STX3, DENR, EGLN1, FUT4, and PCDH7 was found to be significantly related to the progression of lung cancer. Of these genes, CBFA2T3 and STX3 were identified as protective genes, while DENR, EGLN1, FUT4 and PCDH7 were identified as dangerous genes. These 6 genes can be used as independent lung cancer markers to evaluate the prognosis of lung cancer, and whether there is serious inflammatory infiltration in the tumors of high-risk patients. This study not only provided insights into the mechanism of occurrence and the development of lung cancer, it also provided potential targets for targeted lung cancer therapy. However, our study still needs further clinical verification.
Acknowledgments
Funding: This study was supported by the Nantong Science and Technology Project (no. MS12020029), the National Natural Science Foundation of China (grant no. 81501967), and the Postgraduate Research and Practice Innovation Program of Jiangsu Province (no. SJCX20_1156).
Footnote
Reporting Checklist: The authors have completed the MDAR reporting checklist. Available at http://dx.doi.org/10.21037/atm-21-1392
Conflicts of Interest: All authors have completed the ICMJE uniform disclosure form (available at http://dx.doi.org/10.21037/atm-21-1392). The authors have no conflicts of interest to declare.
Ethical Statement: The authors are accountable for all aspects of the work, including ensuring that questions related to the accuracy or integrity of any part of the work have been appropriately investigated and resolved. The study was conducted in accordance with the Declaration of Helsinki (as revised in 2013).
Open Access Statement: This is an Open Access article distributed in accordance with the Creative Commons Attribution-NonCommercial-NoDerivs 4.0 International License (CC BY-NC-ND 4.0), which permits the non-commercial replication and distribution of the article with the strict proviso that no changes or edits are made and the original work is properly cited (including links to both the formal publication through the relevant DOI and the license). See: https://creativecommons.org/licenses/by-nc-nd/4.0/.
References
- de Sousa VML, Carvalho L. Heterogeneity in Lung Cancer. Pathobiology 2018;85:96-107. [Crossref] [PubMed]
- Cagle PT, Allen TC, Olsen RJ. Lung cancer biomarkers: present status and future developments. Arch Pathol Lab Med 2013;137:1191-8. [Crossref] [PubMed]
- Cheung CHY, Juan HF. Quantitative proteomics in lung cancer. J Biomed Sci 2017;24:37. [Crossref] [PubMed]
- Kerr KM, Bubendorf L, Edelman MJ, et al. Second ESMO consensus conference on lung cancer: pathology and molecular biomarkers for non-small-cell lung cancer. Ann Oncol 2014;25:1681-90. [Crossref] [PubMed]
- Jacob JR, Chakravarti A, Palanichamy K. Nicotinamide N -methyltransferase epigenetic and metabolic rewiring promotes metastatic progression. Biotarget 2019;3. [Crossref]
- Sonobe M, Tanaka F, Wada H. Lung cancer-related genes in the blood. Ann Thorac Cardiovasc Surg 2004;10:213-7. [PubMed]
- Zhao Y, Brasier AR. Uronic acid pathway metabolites regulate mesenchymal transition and invasiveness in lung adenocarcinoma. Biotarget 2019;3:19. [Crossref] [PubMed]
- Steinauer N, Guo C, Zhang J. The transcriptional corepressor CBFA2T3 inhibits all-trans-retinoic acid-induced myeloid gene expression and differentiation in acute myeloid leukemia. J Biol Chem 2020;295:8887-900. [Crossref] [PubMed]
- Zhang Y, Zhang D, Lv J, et al. MiR-125a-5p suppresses bladder cancer progression through targeting FUT4. Biomed Pharmacother 2018;108:1039-47. [Crossref] [PubMed]
- Wang D, Wang L, Ren C, et al. High expression of density-regulated re-initiation and release factor drives tumourigenesis and affects clinical outcome. Oncol Lett 2019;17:141-8. [PubMed]
- Briggs KJ, Koivunen P, Cao S, et al. Paracrine Induction of HIF by Glutamate in Breast Cancer: EglN1 Senses Cysteine. Cell 2016;166:126-39. [Crossref] [PubMed]
- Su KJ, Ho CC, Lin CW, et al. Combinations of FUT2 gene polymorphisms and environmental factors are associated with oral cancer risk. Tumour Biol 2016;37:6647-52. [Crossref] [PubMed]
- Shishodia G, Koul S, Koul HK. Protocadherin 7 is overexpressed in castration resistant prostate cancer and promotes aberrant MEK and AKT signaling. Prostate 2019;79:1739-51. [Crossref] [PubMed]
- Zhao Y, He J, Li Y, et al. PHF14 Promotes Cell Proliferation and Migration through the AKT and ERK1/2 Pathways in Gastric Cancer Cells. Biomed Res Int 2020;2020:6507510 [Crossref] [PubMed]
- Maheshwari AS, Rajesh D, Padmanabhan P, et al. Effect of mutation on aggregation propensity in homology model structures of syntaxin-3 from Homo sapiens. Indian J Biochem Biophys 2014;51:335-42. [PubMed]
- Ho XD, Phung P, Le VQ, et al. Whole transcriptome analysis identifies differentially regulated networks between osteosarcoma and normal bone samples. Exp Biol Med (Maywood) 2017;242:1802-11. [Crossref] [PubMed]
- Oghabian A, Greco D, Frilander MJ. IntEREst: intron-exon retention estimator. BMC Bioinformatics 2018;19:130. [Crossref] [PubMed]
- Wang Z, Chen X. Establishment and validation of an immune-associated signature in lung adenocarcinoma. Int Immunopharmacol 2020;88:106867 [Crossref] [PubMed]
- Cho MJ, Jang SH. Relationship between post-traumatic amnesia and white matter integrity in traumatic brain injury using tract-based spatial statistics. Sci Rep 2021;11:6898. [Crossref] [PubMed]
- Liu Z, Zhang H, Hu H, et al. A Novel Six-mRNA Signature Predicts Survival of Patients With Glioblastoma Multiforme. Front Genet 2021;12:634116 [Crossref] [PubMed]
- van der Tuuk K, Tajik P, Koopmans CM, et al. Blood pressure patterns in women with gestational hypertension or mild preeclampsia at term. Eur J Obstet Gynecol Reprod Biol 2017;210:360-5. [Crossref] [PubMed]
- Subramanian A, Tamayo P, Mootha VK, et al. Gene set enrichment analysis: a knowledge-based approach for interpreting genome-wide expression profiles. Proc Natl Acad Sci U S A 2005;102:15545-50. [Crossref] [PubMed]
- Maimaiti A, Jiang L, Wang X, et al. Identification and validation of an individualized prognostic signature of lower-grade glioma based on nine immune related long non-coding RNA. Clin Neurol Neurosurg 2021;201:106464 [Crossref] [PubMed]
- Li T, Fan J, Wang B, et al. TIMER: A Web Server for Comprehensive Analysis of Tumor-Infiltrating Immune Cells. Cancer Res 2017;77:e108-e10. [Crossref] [PubMed]
- Chen B, Khodadoust MS, Liu CL, et al. Profiling Tumor Infiltrating Immune Cells with CIBERSORT. Methods Mol Biol 2018;1711:243-59. [Crossref] [PubMed]
- Wang Z, Wang Y, Peng M, et al. UBASH3B Is a Novel Prognostic Biomarker and Correlated With Immune Infiltrates in Prostate Cancer. Front Oncol 2020;9:1517. [Crossref] [PubMed]
- Kim J. Long noncoding RNA MALAT1 and cancer metastasis. Biotarget 2019;3. [Crossref]
- Wu Y, Jiang M. The revolution of lung cancer treatment: from vaccines, to immune checkpoint inhibitors, to chimeric antigen receptor T therapy. Biotarget 2017;1. [Crossref]
- Yang Z, Qi W, Sun L, et al. DNA methylation analysis of selected genes for the detection of early-stage lung cancer using circulating cell-free DNA. Adv Clin Exp Med 2019;28:355-60. [Crossref] [PubMed]
- Zhang L, Zhang Z, Yu Z. Identification of a novel glycolysis-related gene signature for predicting metastasis and survival in patients with lung adenocarcinoma. J Transl Med 2019;17:423. [Crossref] [PubMed]
- Zhang Y, Liu X, Liu L, et al. Expression and Prognostic Significance of m6A-Related Genes in Lung Adenocarcinoma. Med Sci Monit 2020;26:e919644 [Crossref] [PubMed]
- Salaverria I, Akasaka T, Gesk S, et al. The CBFA2T3/ACSF3 locus is recurrently involved in IGH chromosomal translocation t(14;16)(q32;q24) in pediatric B-cell lymphoma with germinal center phenotype. Genes Chromosomes Cancer 2012;51:338-43. [Crossref] [PubMed]
- Gruber TA, Larson Gedman A, Zhang J, et al. An Inv(16)(p13.3q24.3)-encoded CBFA2T3-GLIS2 fusion protein defines an aggressive subtype of pediatric acute megakaryoblastic leukemia. Cancer Cell 2012;22:683-97. [Crossref] [PubMed]
- Nan H, Han L, Ma J, et al. STX3 represses the stability of the tumor suppressor PTEN to activate the PI3K-Akt-mTOR signaling and promotes the growth of breast cancer cells. Biochim Biophys Acta Mol Basis Dis 2018;1864:1684-92. [Crossref] [PubMed]
- Feng Q, Bonder EM, Engevik AC, et al. Correction: Disruption of Rab8a and Rab11a causes formation of basolateral microvilli in neonatal enteropathy (doi:
10.1242/jcs.201897 ). J Cell Sci 2018;131.10.1242/jcs.201897 - Kochetkova M, McKenzie OL, Bais AJ, et al. CBFA2T3 (MTG16) is a putative breast tumor suppressor gene from the breast cancer loss of heterozygosity region at 16q24.3. Cancer Res 2002;62:4599-604. [PubMed]
- Noort S, Zimmermann M, Reinhardt D, et al. Prognostic impact of t(16;21)(p11;q22) and t(16;21)(q24;q22) in pediatric AML: a retrospective study by the I-BFM Study Group. Blood 2018;132:1584-92. [Crossref] [PubMed]
- Attoff K, Johansson Y, Cediel-Ulloa A, et al. Acrylamide alters CREB and retinoic acid signalling pathways during differentiation of the human neuroblastoma SH-SY5Y cell line. Sci Rep 2020;10:16714. [Crossref] [PubMed]
- Mazan-Mamczarz K, Gartenhaus RB. Post-transcriptional control of the MCT-1-associated protein DENR/DRP by RNA-binding protein AUF1. Cancer Genomics Proteomics 2007;4:233-9. [PubMed]
- Weisser M, Schafer T, Leibundgut M, et al. Structural and Functional Insights into Human Re-initiation Complexes. Mol Cell 2017;67:447-56 e7. [Crossref] [PubMed]
- Bohlen J, Harbrecht L, Blanco S, et al. DENR promotes translation reinitiation via ribosome recycling to drive expression of oncogenes including ATF4. Nat Commun 2020;11:4676. [Crossref] [PubMed]
- Price C, Gill S, Ho ZV, et al. Genome-Wide Interrogation of Human Cancers Identifies EGLN1 Dependency in Clear Cell Ovarian Cancers. Cancer Res 2019;79:2564-79. [Crossref] [PubMed]
- Zhang X, Wang Y. Identification of hub genes and key pathways associated with the progression of gynecological cancer. Oncol Lett 2019;18:6516-24. [Crossref] [PubMed]
- Liu X, Simon JM, Xie H, et al. Genome-wide Screening Identifies SFMBT1 as an Oncogenic Driver in Cancer with VHL Loss. Mol Cell 2020;77:1294-306 e5. [Crossref] [PubMed]
- Kato H, Inoue T, Asanoma K, et al. Induction of human endometrial cancer cell senescence through modulation of HIF-1alpha activity by EGLN1. Int J Cancer 2006;118:1144-53. [Crossref] [PubMed]
- Liu B, Ma H, Liu Q, et al. MiR-29b/Sp1/FUT4 axis modulates the malignancy of leukemia stem cells by regulating fucosylation via Wnt/beta-catenin pathway in acute myeloid leukemia. J Exp Clin Cancer Res 2019;38:200. [Crossref] [PubMed]
- Xu J, Xiao Y, Liu B, et al. Exosomal MALAT1 sponges miR-26a/26b to promote the invasion and metastasis of colorectal cancer via FUT4 enhanced fucosylation and PI3K/Akt pathway. J Exp Clin Cancer Res 2020;39:54. [Crossref] [PubMed]
- Zhou X, Padanad MS, Evers BM, et al. Modulation of Mutant Kras(G12D) -Driven Lung Tumorigenesis In Vivo by Gain or Loss of PCDH7 Function. Mol Cancer Res 2019;17:594-603. [Crossref] [PubMed]
- Li AM, Tian AX, Zhang RX, et al. Protocadherin-7 induces bone metastasis of breast cancer. Biochem Biophys Res Commun 2013;436:486-90. [Crossref] [PubMed]
- Li T, Li Z, Wan H, et al. Recurrence-Associated Long Non-coding RNA LNAPPCC Facilitates Colon Cancer Progression via Forming a Positive Feedback Loop with PCDH7. Mol Ther Nucleic Acids 2020;20:545-57. [Crossref] [PubMed]
- Okuno K, Akiyama Y, Shimada S, et al. Asymmetric dimethylation at histone H3 arginine 2 by PRMT6 in gastric cancer progression. Carcinogenesis 2019;40:15-26. [Crossref] [PubMed]
- Xu S, Wu X, Tao Z, et al. Effect of aberrantly methylated androgen receptor target gene PCDH7 on the development of androgen-independent prostate cancer cells. Genes Genomics 2020;42:299-307. [Crossref] [PubMed]
- Saku A, Hirose K, Ito T, et al. Fucosyltransferase 2 induces lung epithelial fucosylation and exacerbates house dust mite-induced airway inflammation. J Allergy Clin Immunol 2019;144:698-709 e9. [Crossref] [PubMed]
- Taylor SL, Woodman RJ, Chen AC, et al. FUT2 genotype influences lung function, exacerbation frequency and airway microbiota in non-CF bronchiectasis. Thorax 2017;72:304-10. [Crossref] [PubMed]
- Lai TY, Chen IJ, Lin RJ, et al. Fucosyltransferase 1 and 2 play pivotal roles in breast cancer cells. Cell Death Discov 2019;5:74. [Crossref] [PubMed]
(English Language Editor: L. Huleatt)