Tumor microenvironment immune-related lncRNA signature for patients with melanoma
Introduction
The incidence of malignant melanoma only accounts for approximately 5% of skin malignant tumors, but accounts for 75% of its mortality (1,2). Melanoma can easily metastasize to the brain, liver, lungs, and other important organs (3-6). Benefiting from the immune checkpoint inhibitors, the survival time of patients with advanced melanoma over 5 years has increased from 10% to more than 30%, but many immune checkpoint inhibitors still fail (7-9). Therefore, more and more researches are committed to finding novel mechanisms and treatment strategies. Arasu found that IHH present in the HAS3-EVs from melanoma cells activates the hedgehog signaling pathway in the recipient cells, leads to an increase in proliferation and EMT (10). Cabrita indicated that tertiary lymphoid structures improve immunotherapy and survival in melanoma (11). Tseng reported that co-targeting BET (bromodomain and extra-terminal proteins) and MCL1 induces synergistic cell death, and appears to be a promising therapeutic approach for metastatic melanoma (12). Sharma reported that bempegaldesleukin (NKTR-214), an engineered IL-2 cytokine prodrug, selectively depletes intratumoral Tregs and potentiates T cell-mediated cancer therapy (13). In fact, the detection methods or models with good predictive value for the melanoma progression and therapeutic efficiency are also important. If the patient’s risk can be predicted before therapy, it may increase the effectiveness of the treatment. Aya-Bonilla CA found that despite the high phenotypic and molecular heterogeneity of melanoma CTCs, multimarker derived CTC scores could serve as viable tools for prognostication and treatment response monitoring in patients with metastatic melanoma (14). Pruessmann pointed out the prognostic value of T cell fraction (TCFr) in primary melanoma at risk for metastatic recurrence (15).
Long-chain non-coding RNAs (lncRNAs) have wide-ranging functional activities. Disorders of lncRNAs may lead to the occurrence and development of melanoma, and may also be related to immunotherapy. LncRNA SLNCR1 mediates melanoma invasion via its conserved SRA1-like region (16). The role of lncRNA SAMMSON in driving the mitochondrial function of melanoma had been reported (17,18). Targeting SAMMSON (whose gene is often co-amplified with MITF) highlights the vulnerability of new cell type specific therapy in melanoma, which is not related to BRAF, NRAS, or p53 status (19). LncRNA CCAT1 promotes the proliferation and invasion of melanoma cells by targeting miRNA-33a (20). LncRNA ILF3-AS1 up-regulates the proliferation, migration, and invasion of melanoma cells by inhibiting miRNA-200b/a/429 (21). Tumor progression is not only based on the evolution of the tumor cells themselves, but also the inhibition of the tumor microenvironment in the immune state (22). LncRNAs are not only involved in the progression of melanoma, but may also affect the patient’s anti-tumor immunity. LncRNAs exhibited widespread expression patterns in CD4+, CD8+, and CD14+ peripheral blood cells of patients with stage IV melanoma (22). Recently, immune-related lncRNAs signature has been used as predictors for prognosis, survival and immunotherapeutic efficiency in various cancers, such as breast cancer, lung adenocarcinoma, hepatocellular carcinoma and bladder cancer (23-26). Exploring melanoma immune-related lncRNAs may predict melanoma patients’ responsiveness to immunotherapy and may also be used as potential therapeutic targets. Zhou JG identified a 15 predictive lncRNAs signature for prognosis in advanced melanoma patients treated with anti-PD-1 monotherapy. And the different genes between two consensus clusters were mainly related to the immune process (27). Additionally, 8 immune-related lncRNAs could divide melanoma patients into high- and low-risk groups, and effectively predict the prognosis (28).
In our study, we used the transcriptome data of cutaneous melanoma patients in The Cancer Genome Atlas (TCGA) database to establish the signature of immune-related lncRNAs for patients with cutaneous melanoma. This provides a basis for predicting patient responsiveness to immunotherapy, and also provides targets for further exploring the regulation of lncRNAs to change the responsiveness of patients to immunotherapy.
We present the following article in accordance with the MDAR reporting checklist (available at http://dx.doi.org/10.21037/atm-21-1794).
Methods
Patients and datasets
TCGA- SKCM (skin cutaneous melanoma) is available on the TCGA Data Portal (https://tcga-data.nci.nih.gov/tcga/). The data downloaded from the TCGA Data Portal included age, gender, clinical stage, survival status and survival time. Since the data was obtained from the public databases, approval from the Ethics committee or written informed consent from patients was not required. The study was conducted in accordance with the Declaration of Helsinki (as revised in 2013).
LncRNA profile mining
A total of 14,142 lncRNAs were obtained from the TCGA-SKCM transcriptome dataset. The immune-related genes (immune system process M13664, immune response M19817) were extracted from the molecular signature database v4.0, and a total of 331 genes were obtained. Finally, 460 immune-related lncRNAs were identified by constructing an immuno-lncRNAs co-expression network.
Signature development
Based on the 460 immune-related lncRNAs, prognostic genes were screened using univariate Cox regression analysis. According to the P values, the immune-related lncRNAs were sorted in ascending order. P<0.001 was selected as the critical value, and 23 genes were selected for marker development. To determine the predictive survival characteristics, multivariate Cox analysis was performed. High-risk and low-risk groups were classified using a median risk score, and Exprgenen was defined as the expression of lncRNA.
Overall survival curve and receiver operating characteristic (ROC) analysis
According to their risk scores obtained through the prognostic signature, all samples were divided into 2 subgroups, and Kaplan-Meier analysis was used for the comparison of the 2 groups’ survival events. ROC analysis was performed to evaluate the robustness of the prognosis.
Univariate Cox regression and multivariate Cox regression analyses
The univariate Cox regression analysis was performed with the survival package of R version 3.1. A log-rank test P<0.05 was considered significant. Multivariate Cox regression analysis estimated regression coefficients were used to identify the prognostic signature of the risk scores.
Principal component analysis (PCA) and the immune and stromal scores
PCA was performed using R software (version 3.2.3). The immune scores were calculated using the ESTIMATE package of R version 4.0.2.
Gene set enrichment analysis (GSEA) and Venn diagrams
GSEA (http://www.broadinstitute.org/gsea/index.jsp) was used for functional annotation between the 2 groups. The Venn diagrams of overlapping genes among immune process and response groups were plotted using the VennDiagram package version 1.6.20.
Construction of the protein-protein interaction (PPI) network
The PPI was derived from the Retrieval of Interacting Genes (STRING) database (https://string-db.org/, version 11.0) and was reconstructed via Cytoscape version 3.7.1. The interaction score >0.9 was set as the criteria. The Molecular COmplex DEtection (MCODE) plugin of Cytoscape was utilized to identify the most significant clusters.
Comparing the 22 immune cell subtypes between the high and low risk score groups
The CIBERSORT package was used to assess the proportions of 22 immune cell subtypes in two groups based on the expression profiles. The number of permutations was set at 1,000. Samples with P<0.05 in the CIBERSORT analysis results were used in further analysis. The differences in immune cell subtypes between the high and low risk score groups were evaluated by Mann-Whitney U test.
Statistical analysis
The data was processed using the PERL programming language (Version 5.32.0 http://www.perl.org). All statistical analyses were performed using R software (version 4.0.2, https://www.r-project.org/). P<0.05 was regarded as statistically significant.
Results
Identification of the 7 immune-related lncRNA signature in patients with melanoma
The transcriptome data of SKCM in the TCGA database were downloaded and the mRNA and lncRNA expression data were extracted. A total of 331 immune-related genes were extracted from the molecular marker database v4.0 (Immune System Process M13664, immune response M19817). The immune-related lncRNAs were identified by constructing a co-expression network of immune lncRNAs, and finally 460 immune-related lncRNAs were identified. Combined with survival status and time in the TCGA-SKCM data, 20 low-risk and 3 high-risk immune-related lncRNAs were screened at P<0.001 (Figure 1A). Next, multivariate risk-immunity-related lncRNAs developed 7 immune-related lncRNA signatures (WAC-AS1, USP30-AS1, LINC01138, SPRY4-AS1, ZNF667-AS1, AC018553.1, and AC008060.3) and it was observed that with the increase of risk score, the number of patient deaths increased and the survival time of surviving patients shortened (Figure 1B,C). The expression levels of the 7 immune-related lncRNAs were also different when the risk score was elevated. ZNF667-AS1, AC008060.3, and AC018553.1 were gradually increased with increasing risk scores, while WAC-AS1, USP30-AS1, LINC01138, and SPRY4-AS1 were gradually reduced (Figure 1D). This suggests that the 7 immune-related lncRNA signature was related to SKCM risk.
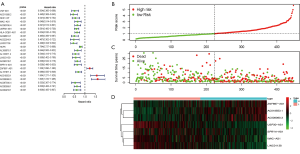
Prediction of patient risk by the 7 immune-related lncRNA signature
The survival curve analysis of the 7 immune-related lncRNAs showed that the survival time of patients was significantly shorter in the ZNF667-AS1, AC008060.3, and AC018553.1 high expression group than in the low expression group (Figure 2A,B,C). Conversely, the survival time of patients in the WAC-AS1, USP30-AS1, LINC01138, and SPRY4-AS1 high expression group was extended (Figure 2D,E,F,G). Subsequently, we compared the sensitivity and specificity of the 7 immune-related lncRNA signature and the individual lncRNAs. In the ROC curve, the order of the areas under the ROC curve (AUC) of the lncRNAs and their combined signature was as follows: Risk Score (AUC =0.737), AC018553.1 (AUC =0.642), AC008060.3 (AUC =0.639), ZNF667-AS1 (AUC =0.597), WAC-AS1 (AUC =0.431), LINC01138 (AUC =0.425), SPRY4-AS1 (AUC =0.425), and USP30-AS1 (AUC =0.328) (Figure 2H). This data suggested that the 7 immune-related lncRNA signature could predict patient risk with more specificity and sensitivity than single immune-related risk lncRNAs.
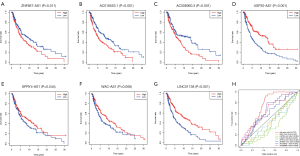
Evaluation of the immune-related lncRNA signature risk scores
The age, gender, staging, T, M, N, and immune-related lncRNA signature risk scores of patients in the TCGA-SKCM dataset were analyzed by univariate risk analysis. The forest plot of the univariate risk analysis showed that the risk score of the immune-related lncRNA signature was significant, as was age, stage, T, and N (Figure 3A). Multivariate risk analysis was used to analyze the age, gender, staging, T, M, N, and immune-related lncRNA signature risk scores of patients in the TCGA-SKCM dataset. The results showed that only T, N, and immune-related lncRNA signature risk scores were significant (Figure 3B). To assess the sensitivity and specificity of the 7 immune-related lncRNA signature risk scores, tumor stage, T, N, and M clinical parameters in the survival of melanoma patients, ROC analysis was performed which showed that the AUCs were 0.749, 0.675, 0.703, 0.510, and 0.688, respectively (Figure 3C). This indicated that the 7 immune-related lncRNA signature risk scores have higher sensitivity and specificity than the tumor stage, T, N, and M clinical parameters. Seven immune-related lncRNA signature risk scores divided TCGA-SKCM tumor patients into high-risk and low-risk groups. Survival analysis showed that OS was significantly shorter in the high-risk group than in the low-risk group (Figure 3D).
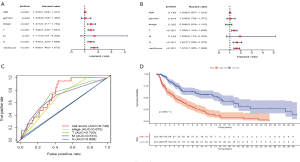
Low and high-risk groups displayed different immune statuses
All 7 lncRNAs in the signature were immune-related, so we investigated the immune status differences between the high and low-risk groups through PCA. The whole gene expression profiles and immune lncRNAs showed that there was no significant difference in the direction of distribution between the high and low-risk groups (Figure 4A,B). A total of 331 immune-related genes were used to divide SKCM into 2 sections better than the whole gene expression profiles and immune lncRNAs, indicating that the 331 immune-related genes were involved in the risk of SKCM to varying degrees between the 2 groups (Figure 4C). The 7 immune-related lncRNA signature almost completely separated the low-risk and high-risk patients with SKCM (Figure 4D), suggesting that the immune status with a certain lncRNA signature indicates immune status in SKCM. Then, we identified the relationship between the 7 immune-related lncRNA signature risk score and immune score in the SKCM microenvironment, which were significantly reversely correlated (r=−0.5904) (Figure 4E). The tumor microenvironment immune score was decreased in the high-risk group compared to the low-risk group (Figure 4F). The above results demonstrated that the 7 immune-related lncRNA signature risk score could reflect the immune microenvironment in SKCM.
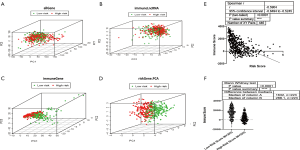
The enrichment of different immune-related key genes in low and high-risk groups
Functional annotation was further performed by GSEA, and the results displayed that different genes were enriched in immune system process and immune response pathways between the 2 groups (Figure 5A,B). GSEA indicated significant enrichment of immune phenotype in the low-risk group. There were 124 overlapping genes in immune system process and immune response pathways (Figure 5C). Co-expression network analysis was used to search the different key genes, and 17 genes were obtained, including CCL4, CCL5, CCL19, CCL25, CXCL12, CXCL13, CCR1, CCR2, CCR4, CCR5, CCR8, CCR9, CXCR4, C5AR1, CNR2, GPR183, and SIPR4 (Figure 5D,E). Subsequently, we analyzed the correlations between the risk score and the 17 key genes in 446 patients with primary and metastatic melanoma, and found that the 17 genes were significantly negatively correlated with the risk score (Figure 5F). The results demonstrated that the 7 immune-related lncRNA signature risk score could indicate the expression of immune-related genes in the SKCM microenvironment.
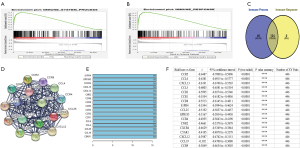
Immune cell infiltration in the low and high-risk groups
In order to further analyze the immune components affected by the 7 immune-related lncRNA signature risk score, we measured the infiltration of 22 kinds of immune cells in the low and high-risk groups (Figure 6). Nine cell types, including T cells CD8, T cells CD4 memory activated, T cells gamma delta, T cells follicular helper (Tfh), NK cells resting, macrophages M0/M1/M2, and mast cells resting were significantly different in the low and high-risk groups. The numbers of macrophages M0/M2, NK cells resting, and mast cells resting were increased, while T cells CD8, T cells CD4 memory activated, T cells gamma delta, Tfh cells and macrophages M1 were decreased in the high-risk group. The results suggested that the 7 immune-related lncRNA signature risk score affected immune cell infiltration in the SKCM microenvironment.
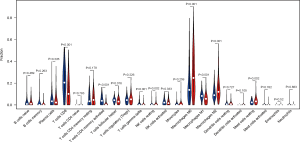
Discussion
The TCGA-SKCM dataset was collected to investigate the prognostic value of immune-related lncRNAs in SKCM patients. The 7 immune-related lncRNAs were identified in a risk prediction model to judge the prognosis of patients. Importantly, it was found that this risk prediction model can better reflect the patient’s immune status.
Among the 7 immune-related lncRNAs, ZNF667-AS1, AC008060.3, and AC018553.1 gradually raised with the increase in risk score, but only ZNF667-AS1 has been reported in related literature (29-32). ZNF667-AS1 inhibits cervical cancer proliferation, invasion and metastasis by counteracting miRNA-93-3p-dependent PEG3 down-regulation (31). ZNF667-AS1 also decreases the inflammatory response and promotes the recovery of spinal cord injury by inhibiting the JAK-STAT pathway (32). Aberrant methylation of ZNF667-AS1 and down-regulation promotes malignant progression of laryngeal squamous cell carcinoma (33). On the other hand, among the 7 immune-related lncRNAs, WAC-AS1, USP30-AS1, LINC01138, and SPRY4-AS1 were gradually down-regulated with the increase in risk scores, but only LINC01138 has been reported in related literature. LINC01138 drives malignant tumors by activating arginine methyltransferase 5 in hepatocellular carcinoma (34). LINC01138 promotes clear cell renal cell carcinoma cell growth by enhancing SREBP1-mediated lipid desaturation by interacting with PRMT5 (35). LINC01138 can also accelerate tumor growth and invasion by targeting miR-1273e in gastric cancer (36). These evidences suggest that ZNF667-AS1 and LINC01138 acts as an oncogene or tumor suppressor, but there is no report about their roles in the regulation of immune genes. We speculate that the 7 immune-related lncRNAs are directly or indirectly involved in the down- or up-regulation of tumor related immune genes. Their abnormal expressions affect the SKCM immune microenvironment and the patient risk scores.
Although the univariate risk analysis showed that these 7 immune-related lncRNAs could be used as low-risk or high-risk factors for SKCM, their AUCs were below 0.7, indicating that their sensitivity and specificity as risk factors alone were not high enough. When they were analyzed as a whole, the 7 immune-related lncRNA signature was found to be an independent risk factor. Its sensitivity and specificity even exceeded the clinical parameters of SKCM tumor staging, tumor size, and tumor lymph node infiltration. The 7 lncRNA signature was screened for its correlation with tumor immune genes and should reflect the immune microenvironment of tumors in SKCM patients. This view was validated with PCA and GSEA analysis.
How do immune-related lncRNAs affect SKCM’s immune microenvironment through core immune genes? We analyzed the 124 genes shared by the immune process and immune response dataset. PPI function analysis showed that 17 genes constituted a core network that affects the SKCM immune microenvironment. The 17 genes were mainly composed of 11 G protein coupled receptors (CCR1, CCR2, CCR4, CCR5, CCR8, CCR9, CXCR4, C5AR1, CNR2, GPR183, and SIPR4) and 6 corresponding ligands (CCL4, CCL5, CCL19, CCL25, CXCL12, and CXCL13).
CCR (CC chemokine receptor) 1 and its ligands, including RANTES (regulated on activation, normal T cell expressed and secreted), MPIF-1 (myeloid progenitor inhibitory factor 1), MIP-1 alpha (macrophage inflammatory protein 1 alpha) and MCP-3 (monocyte chemotactic protein 3), mediates signal transduction are essential for the recruitment of effector immune cells to the site of inflammation (37). CCR2, combination with CCL2, which expressed on monocytes and macrophages, mediates chemotaxis and migration inducted by activating the PI3K cascade, the small G protein Rac, and lamellipodia protrusions (38,39). CCR2 also acts as a receptor for β-defensins DEFB106A/DEFB106B, regulates the expression of T cell inflammatory cytokines and T cell differentiation, and promotes T cell differentiation into T helper 17 cells (Th17) during inflammation (40). CCR4 is the receptor of MIP-1, RANTES, thymus- and activation-regulated chemokine (TARC), and MCP-1 (41), it can regulate the cell transportation of various types of white blood cells, and also plays a basic role in the development, homeostasis, and function of the immune system (42). CCR8 can help activate the correct positioning of T cells in antigenic attack sites and specific areas of lymphatic tissue (43). The specific ligand of CC chemokine receptor 9 (CCR9) is CCL25, which is a key regulator of thymocyte migration and maturation under normal and inflammatory conditions (44). CXC motif chemokine receptor 4 (CXCR4) is a CXC chemokine receptor specific for stromal cell-derived factor 1. It transduces signals by increasing intracellular calcium levels and enhancing MAPK1/MAPK3 activation, and regulates cell migration (45-47). Complement C5a receptor 1 (C5AR1), a biochemical pathway involved in innate and adaptive immune responses, can lyse microorganisms, promote phagocytosis, and trigger inflammation and immune clearance (48). G protein-coupled receptor 183 (GPR183) acts as a chemotactic receptor for T cells, B cells, monocytes/macrophages, splenic dendritic cells, and astrocytes by binding to oxysterol 7-α,25-dihydroxycholesterol (7-α, 25-OHC) and other related oxysterol receptors, forming a chemotactic gradient of 7-α, 25-OHC ligands to mediate the cellular positioning and movement of many cells (49). GPR183 guides B cells to move along the boundary of the B cell area-T cell area, and then reach the intravesicular and extrafollicular area (50-52). The sphingosine-1-phosphate receptor 4 (S1PR4) is a member of the endothelial cell differentiation receptor gene family (53). EDG receptors bind lysophospholipids or lysosphingolipids and participate in cell signal transduction of many different cell types, and may be involved in the process of cell migration specific to lymphocytes (54).
C-C motif chemokine ligand (CCL) 4 is a mitogen-induced single factor produced by CD8 + T cells (55). CCL5 can act as a chemokine for blood monocytes, memory T helper cells, and eosinophils, and may activate several chemokine receptors, including CCR1, CCR3, CCR4, and CCR5 (56-59). CCL19 shows effective chemotactic activity on T cells and B cells, and may play a role in normal lymphocyte recirculation and homing, and T cells and B cells to secondary lymphoid organs in the migration (60,61). CCL25 binds to the chemokine receptor CCR9, shows chemotactic activity on dendritic cells, thymocytes, and activated macrophages, and potentially participates in the development of T cells (62,63). CXCL12 is a ligand for CXCR4 and plays a role in many different cellular functions, including embryogenesis, immune surveillance, inflammation, tissue stability, tumor growth, and metastasis (64,65). CXCL13 is a B lymphocyte chemokine that stimulates calcium influx and expresses Burkitt’s lymphoma receptor 1 (BLR-1) cell chemotaxis, thereby preferentially promoting B migration of lymphocytes and may play a role in homing B lymphocytes into follicles (66,67).
In order to verify how immune genes affect the infiltration of immune cells in SKCM, the CIBERSORT algorithm was used to calculate the immune cell subtypes in the tumor microenvironment (68). T cells CD8, T cells CD4 memory activated, Tfh cells, T cells gamma delta, and macrophages M1 had lower levels in the high-risk group, while NK cells resting, macrophages M0/M2, and mast cells remained at a higher level. We speculated that the 7 immune-related lncRNAs affect the tumor infiltration of those immune cells through changing the expression and secretion of the 17 core genes. Of course, the roles of these 7 immune-related lncRNAs and their related tumor immune genes in the regulation of the SKCM immune microenvironment needs to be supported by experimental evidence, which is worthy of further investigation.
Based on these efforts, we constructed a 7 immune-related lncRNA signature to identify not only SKCM patients at low risk or high risk, but also to differentiate patients’ tumor immune microenvironment. Specifically, the tumor immune microenvironment is in a state of inhibition in high-risk patients, while for low-risk patients, it is in a state of activation. In conclusion, we identified 7 immune-related lncRNAs from the TCGA-SKCM as a risk prediction model to judge the prognosis of patients. More importantly, it was found that this risk prediction model could better reflect the tumor microenvironment immune status of patients and predict the efficacy of immunotherapy for patients.
Acknowledgments
Thank you to The Cancer Genome Atlas (TCGA) Program for providing the data required for this study.
Funding: This work was supported in part by the National Natural Science Foundation of China (81172322, 81302006 and 81572796), Science and Technology Committee of Shanghai (14401901500).
Footnote
Reporting Checklist: The authors have completed the MDAR reporting checklist. Available at http://dx.doi.org/10.21037/atm-21-1794
Conflicts of Interest: All authors have completed the ICMJE uniform disclosure form (available at http://dx.doi.org/10.21037/atm-21-1794). The authors have no conflicts of interest to declare.
Ethical Statement: The authors are accountable for all aspects of the work in ensuring that questions related to the accuracy or integrity of any part of the work are appropriately investigated and resolved. The study was conducted in accordance with the Declaration of Helsinki (as revised in 2013).
Open Access Statement: This is an Open Access article distributed in accordance with the Creative Commons Attribution-NonCommercial-NoDerivs 4.0 International License (CC BY-NC-ND 4.0), which permits the non-commercial replication and distribution of the article with the strict proviso that no changes or edits are made and the original work is properly cited (including links to both the formal publication through the relevant DOI and the license). See: https://creativecommons.org/licenses/by-nc-nd/4.0/.
References
- Muchmore JH, Mizuguchi RS, Lee C. Malignant melanoma in American black females: an unusual distribution of primary sites. J Am Coll Surg 1996;183:457-65. [PubMed]
- Richards MA, Stockton D, Babb P, et al. How many deaths have been avoided through improvements in cancer survival? BMJ 2000;320:895-8. [Crossref] [PubMed]
- Tanaka R, Kashiwagi S, Ishihara S, et al. A case of malignant melanoma metastasis to the mammary gland. Gan To Kagaku Ryoho 2014;41:1939-41. [PubMed]
- Braeuer RR, Watson IR, Wu CJ, et al. Why is melanoma so metastatic? Pigment Cell Melanoma Res 2014;27:19-36. [Crossref] [PubMed]
- Bates JE, Youn P, Usuki KY, et al. Brain metastasis from melanoma: the prognostic value of varying sites of extracranial disease. J Neurooncol 2015;125:411-8. [Crossref] [PubMed]
- Alqahtani S, Alhefdhi AY, Almalik O, et al. Primary oral malignant melanoma metastasis to the brain and breast: A case report and literature review. Oncol Lett 2017;14:1275-80. [Crossref] [PubMed]
- Stucci LS, D’Oronzo S, Tucci M, et al. Vitamin D in melanoma: Controversies and potential role in combination with immune check-point inhibitors. Cancer Treat Rev 2018;69:21-8. [Crossref] [PubMed]
- Okiyama N, Tanaka R. Varied immuno-related adverse events induced by immune-check point inhibitors - Nivolumab-associated psoriasiform dermatitis related with increased serum level of interleukin-6. Nihon Rinsho Meneki Gakkai Kaishi 2017;40:95-101. [Crossref] [PubMed]
- Esmaeli B, Sagiv O. Targeted Biological Drugs and Immune Check Point Inhibitors for Locally Advanced or Metastatic Cancers of the Conjunctiva, Eyelid, and Orbit. Int Ophthalmol Clin 2019;59:13-26. [Crossref] [PubMed]
- Arasu UT, Deen AJ, Pasonen-Seppänen S, et al. HAS3-induced extracellular vesicles from melanoma cells stimulate IHH mediated c-Myc upregulation via the hedgehog signaling pathway in target cells. Cell Mol Life Sci 2020;77:4093-115. [Crossref] [PubMed]
- Cabrita R, Lauss M, Sanna A, et al. Tertiary lymphoid structures improve immunotherapy and survival in melanoma. Nature 2020;577:561-5. [Crossref] [PubMed]
- Tseng HY, Dreyer J, Emran AA, et al. Co-targeting bromodomain and extra-terminal proteins and MCL1 induces synergistic cell death in melanoma. Int J Cancer 2020;147:2176-89. [Crossref] [PubMed]
- Sharma M, Khong H, Fa'ak F, et al. Bempegaldesleukin selectively depletes intratumoral Tregs and potentiates T cell-mediated cancer therapy. Nat Commun 2020;11:661. [Crossref] [PubMed]
- Aya-Bonilla CA, Morici M, Hong X, et al. Detection and prognostic role of heterogeneous populations of melanoma circulating tumour cells. Br J Cancer 2020;122:1059-67. [Crossref] [PubMed]
- Pruessmann W, Rytlewski J, Wilmott J, et al. Molecular analysis of primary melanoma T cells identifies patients at risk for metastatic recurrence. Nat Cancer 2020;1:197-209. [Crossref] [PubMed]
- Schmidt K, Joyce CE, Buquicchio F, et al. The lncRNA SLNCR1 Mediates Melanoma Invasion through a Conserved SRA1-like Region. Cell Rep 2016;15:2025-37. [Crossref] [PubMed]
- Fogal V, Zhang L, Krajewski S, et al. Mitochondrial/cell-surface protein p32/gC1qR as a molecular target in tumor cells and tumor stroma. Cancer Res 2008;68:7210-8. [Crossref] [PubMed]
- Fogal V, Richardson AD, Karmali PP, et al. Mitochondrial p32 protein is a critical regulator of tumor metabolism via maintenance of oxidative phosphorylation. Mol Cell Biol 2010;30:1303-18. [Crossref] [PubMed]
- Goding CR. Targeting the lncRNA SAMMSON Reveals Metabolic Vulnerability in Melanoma. Cancer Cell 2016;29:619-21. [Crossref] [PubMed]
- Lv L, Jia JQ, Chen J. The lncRNA CCAT1 Upregulates Proliferation and Invasion in Melanoma Cells via Suppressing miR-33a. Oncol Res 2018;26:201-8. [Crossref] [PubMed]
- Chen X, Liu S, Zhao X, et al. Long noncoding RNA ILF3-AS1 promotes cell proliferation, migration, and invasion via negatively regulating miR-200b/a/429 in melanoma. Biosci Rep 2017;37:BSR20171031 [Crossref] [PubMed]
- Best MG, Wesseling P, Wurdinger T. Tumor-Educated Platelets as a Noninvasive Biomarker Source for Cancer Detection and Progression Monitoring. Cancer Res 2018;78:3407-12. [Crossref] [PubMed]
- Ma W, Zhao F, Yu X, et al. Immune-related lncRNAs as predictors of survival in breast cancer: a prognostic signature. J Transl Med 2020;18:442. [Crossref] [PubMed]
- Li JP, Li R, Liu X, et al. A Seven Immune-Related lncRNAs Model to Increase the Predicted Value of Lung Adenocarcinoma. Front Oncol 2020;10:560779 [Crossref] [PubMed]
- Zhang Y, Zhang L, Xu Y, et al. Immune-related long noncoding RNA signature for predicting survival and immune checkpoint blockade in hepatocellular carcinoma. J Cell Physiol 2020;235:9304-16. [Crossref] [PubMed]
- Cao R, Yuan L, Ma B, et al. Immune-related long non-coding RNA signature identified prognosis and immunotherapeutic efficiency in bladder cancer (BLCA). Cancer Cell Int 2020;20:276. [Crossref] [PubMed]
- Zhou JG, Liang B, Liu JG, et al. Identification of 15 lncRNAs Signature for Predicting Survival Benefit of Advanced Melanoma Patients Treated with Anti-PD-1 Monotherapy. Cells 2021;10:977. [Crossref] [PubMed]
- Wang Y, Ba HJ, Wen XZ, et al. A prognostic model for melanoma patients on the basis of immune-related lncRNAs. Aging (Albany NY) 2021;13:6554-64. [Crossref] [PubMed]
- Zhao LP, Li RH, Han DM, et al. Independent prognostic Factor of low-expressed LncRNA ZNF667-AS1 for cervical cancer and inhibitory function on the proliferation of cervical cancer. Eur Rev Med Pharmacol Sci 2017;21:5353-60. [PubMed]
- Li JW, Kuang Y, Chen L, et al. LncRNA ZNF667-AS1 inhibits inflammatory response and promotes recovery of spinal cord injury via suppressing JAK-STAT pathway. Eur Rev Med Pharmacol Sci 2018;22:7614-20. [PubMed]
- Meng W, Cui W, Zhao L, et al. Aberrant methylation and downregulation of ZNF667-AS1 and ZNF667 promote the malignant progression of laryngeal squamous cell carcinoma. J Biomed Sci 2019;26:13. [Crossref] [PubMed]
- Li YJ, Yang Z, Wang YY, et al. Long noncoding RNA ZNF667-AS1 reduces tumor invasion and metastasis in cervical cancer by counteracting microRNA-93-3p-dependent PEG3 downregulation. Mol Oncol 2019;13:2375-92. [Crossref] [PubMed]
- Dong Z, Li S, Wu X, et al. Aberrant hypermethylation-mediated downregulation of antisense lncRNA ZNF667-AS1 and its sense gene ZNF667 correlate with progression and prognosis of esophageal squamous cell carcinoma. Cell Death Dis 2019;10:930. [Crossref] [PubMed]
- Li Z, Zhang J, Liu X, et al. The LINC01138 drives malignancies via activating arginine methyltransferase 5 in hepatocellular carcinoma. Nat Commun 2018;9:1572. [Crossref] [PubMed]
- Zhang X, Wu J, Wu C, et al. The LINC01138 interacts with PRMT5 to promote SREBP1-mediated lipid desaturation and cell growth in clear cell renal cell carcinoma. Biochem Biophys Res Commun 2018;507:337-42. [Crossref] [PubMed]
- Dou GX, Zhang JN, Wang P, et al. Long Intergenic Non-Protein-Coding RNA 01138 Accelerates Tumor Growth and Invasion in Gastric Cancer by Regulating miR-1273e. Med Sci Monit 2019;25:2141-50. [Crossref] [PubMed]
- Morteau O, Castagliuolo I, Mykoniatis A, et al. Genetic deficiency in the chemokine receptor CCR1 protects against acute Clostridium difficile toxin A enteritis in mice. Gastroenterology 2002;122:725-33. [Crossref] [PubMed]
- Nomiyama H, Osada N, Yoshie O. A family tree of vertebrate chemokine receptors for a unified nomenclature. Dev Comp Immunol 2011;35:705-15. [Crossref] [PubMed]
- Terashima Y, Onai N, Murai M, et al. Pivotal function for cytoplasmic protein FROUNT in CCR2-mediated monocyte chemotaxis. Nat Immunol 2005;6:827-35. [Crossref] [PubMed]
- Trivedi NR, Gilliland KL, Zhao W, et al. Gene array expression profiling in acne lesions reveals marked upregulation of genes involved in inflammation and matrix remodeling. J Invest Dermatol 2006;126:1071-9. [Crossref] [PubMed]
- Imai T, Baba M, Nishimura M, et al. The T cell-directed CC chemokine TARC is a highly specific biological ligand for CC chemokine receptor 4. J Biol Chem 1997;272:15036-42. [Crossref] [PubMed]
- Andrews G, Jones C, Wreggett KA. An intracellular allosteric site for a specific class of antagonists of the CC chemokine G protein-coupled receptors CCR4 and CCR5. Mol Pharmacol 2008;73:855-67. [Crossref] [PubMed]
- Bernardini G, Hedrick J, Sozzani S, et al. Identification of the CC chemokines TARC and macrophage inflammatory protein-1 beta as novel functional ligands for the CCR8 receptor. Eur J Immunol 1998;28:582-8. [Crossref] [PubMed]
- Li J, Xiong T, Xiao R, et al. Anti-CCL25 antibody prolongs skin allograft survival by blocking CCR9 expression and impairing splenic T-cell function. Arch Immunol Ther Exp (Warsz) 2013;61:237-44. [Crossref] [PubMed]
- Nagasawa T, Hirota S, Tachibana K, et al. Defects of B-cell lymphopoiesis and bone-marrow myelopoiesis in mice lacking the CXC chemokine PBSF/SDF-1. Nature 1996;382:635-8. [Crossref] [PubMed]
- Zou YR, Kottmann AH, Kuroda M, et al. Function of the chemokine receptor CXCR4 in haematopoiesis and in cerebellar development. Nature 1998;393:595-9. [Crossref] [PubMed]
- Ma Q, Jones D, Borghesani PR, et al. Impaired B-lymphopoiesis, myelopoiesis, and derailed cerebellar neuron migration in CXCR4- and SDF-1-deficient mice. Proc Natl Acad Sci U S A 1998;95:9448-53. [Crossref] [PubMed]
- Atkinson SM, Nansen A, Usher PA, et al. Treatment with anti-C5aR mAb leads to early-onset clinical and mechanistic effects in the murine delayed-type hypersensitivity arthritis model. Autoimmunity 2015;48:460-70. [Crossref] [PubMed]
- Sun S, Liu C. 7α, 25-dihydroxycholesterol-mediated activation of EBI2 in immune regulation and diseases. Front Pharmacol 2015;6:60. [Crossref] [PubMed]
- Kelly LM, Pereira JP, Yi T, et al. EBI2 guides serial movements of activated B cells and ligand activity is detectable in lymphoid and nonlymphoid tissues. J Immunol 2011;187:3026-32. [Crossref] [PubMed]
- Pereira JP, Kelly LM, Cyster JG. Finding the right niche: B-cell migration in the early phases of T-dependent antibody responses. Int Immunol 2010;22:413-9. [Crossref] [PubMed]
- Li J, Lu E, Yi T, et al. EBI2 augments Tfh cell fate by promoting interaction with IL-2-quenching dendritic cells. Nature 2016;533:110-4. [Crossref] [PubMed]
- Cartier A, Hla T. Sphingosine 1-phosphate: Lipid signaling in pathology and therapy. Science 2019;366:eaar5551 [Crossref] [PubMed]
- Takuwa Y, Takuwa N, Sugimoto N. The Edg family G protein-coupled receptors for lysophospholipids: their signaling properties and biological activities. J Biochem 2002;131:767-71. [Crossref] [PubMed]
- Joosten SA, van Meijgaarden KE, Savage ND, et al. Identification of a human CD8+ regulatory T cell subset that mediates suppression through the chemokine CC chemokine ligand 4. Proc Natl Acad Sci U S A 2007;104:8029-34. [Crossref] [PubMed]
- Soria G, Ben-Baruch A. The inflammatory chemokines CCL2 and CCL5 in breast cancer. Cancer Lett 2008;267:271-85. [Crossref] [PubMed]
- Nelson PJ, Kim HT, Manning WC, et al. Genomic organization and transcriptional regulation of the RANTES chemokine gene. J Immunol 1993;151:2601-12. [PubMed]
- Devergne O, Marfaing-Koka A, Schall TJ, et al. Production of the RANTES chemokine in delayed-type hypersensitivity reactions: involvement of macrophages and endothelial cells. J Exp Med 1994;179:1689-94. [Crossref] [PubMed]
- Wang JH, Devalia JL, Xia C, et al. Expression of RANTES by human bronchial epithelial cells in vitro and in vivo and the effect of corticosteroids. Am J Respir Cell Mol Biol 1996;14:27-35. [Crossref] [PubMed]
- Ngo VN, Tang HL, Cyster JG. Epstein-Barr virus-induced molecule 1 ligand chemokine is expressed by dendritic cells in lymphoid tissues and strongly attracts naive T cells and activated B cells. J Exp Med 1998;188:181-91. [Crossref] [PubMed]
- Dieu MC, Vanbervliet B, Vicari A, et al. Selective recruitment of immature and mature dendritic cells by distinct chemokines expressed in different anatomic sites. J Exp Med 1998;188:373-86. [Crossref] [PubMed]
- Carramolino L, Zaballos A, Kremer L, et al. Expression of CCR9 beta-chemokine receptor is modulated in thymocyte differentiation and is selectively maintained in CD8(+) T cells from secondary lymphoid organs. Blood 2001;97:850-7. [Crossref] [PubMed]
- Youn BS, Kim CH, Smith FO, et al. TECK, an efficacious chemoattractant for human thymocytes, uses GPR-9-6/CCR9 as a specific receptor. Blood 1999;94:2533-6. [Crossref] [PubMed]
- Janssens R, Mortier A, Boff D, et al. Truncation of CXCL12 by CD26 reduces its CXC chemokine receptor 4- and atypical chemokine receptor 3-dependent activity on endothelial cells and lymphocytes. Biochem Pharmacol 2017;132:92-101. [Crossref] [PubMed]
- Karin N. The multiple faces of CXCL12 (SDF-1alpha) in the regulation of immunity during health and disease. J Leukoc Biol 2010;88:463-73. [Crossref] [PubMed]
- Förster R, Mattis AE, Kremmer E, et al. A putative chemokine receptor, BLR1, directs B cell migration to defined lymphoid organs and specific anatomic compartments of the spleen. Cell 1996;87:1037-47. [Crossref] [PubMed]
- Jenh CH, Cox MA, Hipkin W, et al. Human B cell-attracting chemokine 1 (BCA-1; CXCL13) is an agonist for the human CXCR3 receptor. Cytokine 2001;15:113-21. [Crossref] [PubMed]
- Newman AM, Liu CL, Green MR, et al. Robust enumeration of cell subsets from tissue expression profiles. Nat Methods 2015;12:453-7. [Crossref] [PubMed]
(English Language Editor: C. Betlazar-Maseh)