Ideal cardiovascular health metrics and epicardial adipose tissue volume in a Northern Chinese population: a cross-sectional study
Introduction
On a global scale, cardiovascular disease is the leading cause of death accounting for nearly 30% of the annual global death toll (1). The American Heart Association (AHA) formulated the ideal cardiovascular health (ICVH) metrics, which used ideal levels of seven cardiovascular risk factors and healthy behaviors as indicators, to effectively assess the cardiovascular health of the population. These seven indicators included nonsmoking, a healthy diet, active physical activity, low body mass index, and low blood pressure, fasting blood sugar, and total cholesterol (2). Unlike the concept of “primary prevention”, the AHA ICVH underlines the potential concept of “primordial prevention”, which means it aims to encourage the population to practice a healthier lifestyle to avoid risk factors, rather than to prevent disease (3). Since being established, ICVH metrics have been used worldwide to monitor population cardiovascular health and assess the risk of CVD as a simplified 7-item tool which has a positive correlation with a lower incidence of cardiovascular disease and lower cardiovascular and all-cause mortality (4-7).
Epicardial adipose tissue (EAT) is the fat depot between the myocardium and the pericardial visceral layer. It is located next to the myocardium within the right ventricular sidewall and the left ventricular anterior wall, surrounding the right coronary artery and the anterior descending branch of the left coronary artery (8,9). With many local and systemic effects, EAT is a distinctive and multifaceted fat tissue. Research concerning epicardial fat tissue has developed very rapidly with the discovery of its key position, its unique metabolic characteristics, and clinical measurability (9). EAT is anatomically and functionally contiguous with the myocardium (8), with which it shares an unobstructed microcirculation, and has many unique and complex physiological functions, grossly distinguished by metabolic, thermogenic, secretory and mechanical properties (8,10). However, in obese and type 2 diabetes patients, the EAT becomes thicker or bulkier, and this dysfunctional EAT may lead to the occurrence of cardiovascular diseases including coronary heart disease (CAD) (11-13). Increased amounts of EAT are associated with coronary artery disease, metabolic syndrome, insulin resistance, fatty liver, and heart abnormalities. While epicardial fat can be observed and measured using standard non-invasive imaging methods including echocardiography, multi-slice spiral CT, and MRI (14-16), some of these methods lack sensitivity and specificity, and no recognized cut-off value of EAT thickness or volume has yet been established.
In this context, the hypothesis that ICVH factors and behaviours would produce an effect on EAT amounts arose, and on this basis, we carried out this cross-sectional analysis to probe into the relevance between ideal cardiovascular metrics and EAT volume (EATV) in a northern Chinese population. In addition, we provide a further discussion on EAT quantification and cardiovascular health to provide evidence of the availability of EAT quantification in clinical practice.
We present the following article in accordance with the STROBE reporting checklist (available at http://dx.doi.org/10.21037/atm-21-1798).
Methods
Study design and population
Data was collected from a prospective, community-based study of Chinese adults conducted in Jidong, a community located in the large-scale and modern industrial city of Tangshan, situated in the central zone of the Bohai Rim region in Hebei Province in northern China. Some related research from this study has been reported in detail previously (17,18). From July 2013 to August 2014, 9,078 residents of the Jidong community were recruited for the study including 3,590 subjects aged 45 years or older who had complete information on the results of an examination using computed tomography (CT) for EATV. Of these, 238 participants were excluded because of a history of cancer, stroke, and heart-related diseases such as atrial fibrillation, myocardial infarction, or heart failure. We also excluded 870 subjects with incomplete information on ideal cardiovascular health and other baseline data including education level, income, and alcohol consumption. Finally, 2,482 subjects (including 1,243 males and 1,239 females) remained in the study (Figure 1).
The research was performed following guidelines of the Helsinki Declaration (as revised in 2013) and was authorized by the local Ethics Committee of Jidong Oil- field, Inc. Medical Centers. All participants provided signed informed consent.
Assessment of cardiovascular health metrics
According to the guidelines of the AHA, the seven cardiovascular health metrics were divided into three levels: “poor”, “intermediate”, and “ideal” (2). For the smoking metric, poor represented current smoking, intermediate represented former smoking in less than 1 year, and ideal represented never smoking or quit smoking for more than 1 year. For the BMI index, exceeding 30 kg/m2 was defined as poor, while 25–29.9 kg/m2 was intermediate, and less than 25 kg/m2 was ideal. For physical activity metrics, poor was determined as never doing any exercise, intermediate indicated weekly exercise not more than 150 minutes in moderate intensity or 75 minutes in vigorous intensity, and ideal indicated exercise time per week greater than 150 minutes in moderate intensity or 75 minutes in vigorous intensity. For healthy dietary behavior, poor represented a dietary intake of 0 or 1 component, intermediate represented 2 or 3 components, and ideal represented 4 or 5 components. For total cholesterol status, total cholesterol greater than 240 mg/dL was considered poor, between 200 and 239 mg/dL was considered intermediate, and less than 200 mg/dL or having reached that goal after treatment was considered ideal. For blood pressure values, systolic blood pressure (SBP) ≥140 mmHg or diastolic blood pressure (DBP) ≥90 mmHg was classified as poor, SBP 120–139 mmHg and DBP 80–90 mmHg, and having reached that goal after treatment was classified as intermediate, and SBP less than 120 mmHg and DBP less than 80 mmHg and untreated was classified as ideal. Fasting blood glucose level was classified as poor (0–125 mg/dL), intermediate (100–125 mg/dL and reached that goal after treatment), or ideal (<100 mg/dL while untreated).
Data on smoking, physical activity, and dietary intake was gathered from questionnaires. Based on the information reported by the participants themselves, smoking status was divided into three categories: “never”, “former” and “current” and the status quo of physical activity was based on answers about the type and frequency of physical activity during working hours and leisure time. The collection of dietary data was based on a short semi-quantitative food frequency questionnaire which is based on the AHA’s definition of eating habits (2). A healthy diet requires ≥4.5 servings of fruits and vegetables per day; ≥2 servings of fish or mollusk per week; ≥3 servings of fiber enriched whole grains per day; intake of sugary drinks once a week or less; and salt intake less than 6 g per day. Based on the results collected by our questionnaire, the three indicators of smoking status, physical activity and dietary intake were divided into three levels: poor, intermediate and ideal. As example, for smoking status, a “poor” group represented people who still smoke, an “intermediate” group represented people who smoked before, and an “ideal” group included people who have never smoked.
We conducted accurate measurements of the height and weight of the participants to one decimal place, and calculated body mass index (BMI) based on this, for which the calculation formula is: BMI=weight/height*height. A mercury sphygmomanometer with an appropriate size cuff was used to measure blood pressure with an accuracy of 2 mmHg. After resting on a chair for more than 5 minutes, two measurements of the systolic blood pressure (SBP) and diastolic blood pressure (DBP) at a 5-minute intermission were performed and the average of these results was used as the data for final analysis. If the difference between the first two blood pressure measurements exceeded 5 mmHg, a third measurement was performed, and finally the average value of the three measurement results was used. The criteria for hypertension was as follow: hypertension history, or taking antihypertensive drugs, or a SBP exceeding 140 mmHg, or a DBP exceeding 90 mmHg.
After overnight fasting, blood samples collected by experienced phlebotomists were stored immediately in tubes containing ethylene diamine tetraacetic acid at a temperature of 4 °C. The venous blood samples were then centrifuged at 3,000 rpm for 10 minutes at room temperature (25 °C), and the separated plasma samples were valid for 4 hours. Using an automatic analyzer (AU400, Olympus, Japan) as the measurement tool, biochemical indicators including total cholesterol (measured by the endpoint test method for TC), triglycerides (measured by the GPO method for TG), and fasting blood glucose levels were tested at the testing center of Jidong Oilfield Hospital. Those with a fasting blood glucose over 7.0 mmol/L, people who were currently being treated with insulin or oral hypoglycemic drugs, or those having a history of self-reported diabetes, were collectively referred to as diabetic patients and those currently using cholesterol-lowering drugs, or a total cholesterol level greater than 220 mg/dL, or triglycerides greater than 150 mg/dL, or those with relevant medical history, were collectively referred to as hyperlipidemia patients.
This study created a dichotomy variable for the components of each health indicator, with 1 representing “ideal”, and 0 representing “intermediate” and “poor” (both regarded as “non-ideal”). The overall effect of each health indicator has been tested.
Assessment of potential covariates
We used questionnaires to collect demographic information including age, gender, education level, income, and alcohol consumption and divided participants into two categories, namely 45–59 years old and ≥60 years old. The average salary of every family member per month was classified as “<¥3,000”, “¥3,000–5,000” or “>¥5,000”, and participants’ educational attainment was categorized as “primary school or below”, “middle or high school”, and “college or above”. Alcohol intake was defined as “none”, “<a standard quantity”, and “≥a standard quantity”, where a standard quantity amount was 120 mL wine, 360 mL beer, or 45 mL liquor daily.
Assessment of EATV
Compared with echocardiography, cardiac CT has the advantage of being more sensitive and accurate in measuring the region-specific thickness and volume of deep epicardial fat layers such as fat layers around the coronary artery (19,20).
We evaluated EATV using a high-pitch dual-source CT (Siemens, Germany). Two radiologists who had extensive clinical experience but had no knowledge of the purpose of the study and the patient’s personal information measured the total EATV through the same images sets collected by CT. Using a cursor pointer to manually trace the pericardial contour with 0.75-mm-thick reconstructed axial slices, the radiologists traced the pericardium contour starting from the lower visible level of the pulmonary artery bifurcation until the last slice where the pericardium is still visible for every 10 mm (21).
Software (syngo volume, Siemens Medical Solutions) was then applied to extrapolate the pericardial contour for the non-traced slices, and the results were checked again by the operator. The determination of EAT depended on the attenuation references of adipose tissue between −190 and −30 Hounsfield units (22). This study did not analyze pericardial adipose tissue, which is located outside the visceral pericardium and on the external surface of the parietal pericardium.
The two radiologists were blinded to each other’s measurements for the assessment of inter-observer consistency in 50 randomly selected patients and assessed the total EATV independently. The intra-observer correlation coefficient for EATV measurements was 0.96, while the inter-observer correlation coefficient was 0.92.
Based on previous reports (23), patients were divided into a low EATV group and a high EATV group according to a cut-off value for EATV of 100 mL.
Statistical analysis
SAS software version 9.4 (SAS Institute, Cary, North Carolina, USA) was used as the statistical analysis tool. The value of mean ± SD represents continuous data on condition that the distribution was normal, and categorical data was described as frequencies and percentages. Normally distributed parameters were compared by using ANOVA test and if the variables were categorical, the chi-squared test was applied for comparison.
This study calculated odds ratios (ORs) and 95% confidence intervals (95% CI) to evaluate the correlation between ideal cardiovascular health metrics and EATV by logistic regression analysis. Considering there were only a few participants in the 0 or 7 ideal CVH metrics groups, the 0/1 ideal metric groups and 6/7 ideal metrics groups were combined respectively. Further, the cardiovascular health metrics model was adjusted because age, gender, income, education level, and alcohol consumption may affect EATV, and were related to exposure (i.e., ideal health metrics) according to Table 1. Finally, all statistical analyses were two-sided, and P<0.05 was regarded as statistically significant.
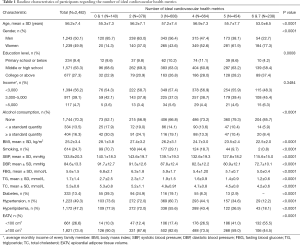
Full table
Results
Table 1 shows the basic characteristics of participants concerning the number of ideal CVH metrics. Those with a relatively large number of ideal CVH metrics were inclined to be women, younger, and had higher education, as well as having less smoking and daily alcohol consumption. Parameters such as BMI, SBP, DBP, FBG, TG, and TC were higher in the participants with lower ICVH metrics, who also had a higher prevalence of diabetes, hypertension, and hyperlipidemia compared to those with a comparatively larger number of ICVH metrics. In addition, the EATV tended to be smaller in those who had more ICVH factors and behaviours. Figure 2 shows the percentage of participants with normal and high EAT volume by the number of ideal cardiovascular health metrics. We did not observe any significant differences in income between different numbers of ideal CVH metrics in this analysis.
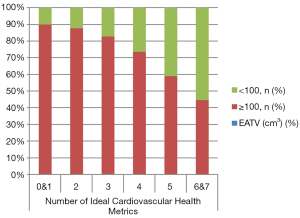
Table 2 shows the relationship between each element of the CVH metrics and the prevalence of high EATV. Based on the adjustment of gender, age, education, income, alcohol consumption, and the other six indicators, the results showed that there was a significant correlation between ideal BMI with the low prevalence of high EATV in all gender and age groups, when respectively compared with the non-ideal group. There was also a significant correlation between ideal total cholesterol and a lower prevalence of high EATV in the general population, but in further subgroup analysis, only younger groups (<60 years of age) and male groups met a significant level, respectively. In addition, there was a significant correlation between ideal fasting blood glucose and a low prevalence of high EATV in the total population, although this correlation was only reflected in men, and no significant correlation between smoking, physical activity, diet, or blood pressure and the prevalence of high EATV in any group of participants.
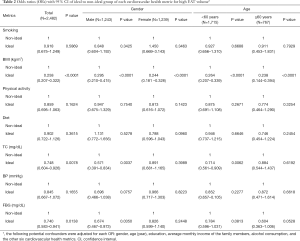
Full table
Table 3 depicts the ORs of high EAT volume due to ideal CVH metrics numbers and shows the group with more ideal CVH metrics numbers had lower crude OR values of prevalence of high EATV. This pattern (P trend less than 0.0001) did not change after adjustment for age, gender, education level, income, and daily alcohol consumption. In addition, significant negative correlations were discovered in both male (P trend less than 0.0001) and female (P trend less than 0.0001), and both age groups (P trend less than 0.0001).
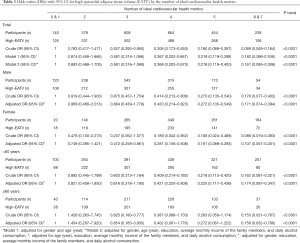
Full table
Discussion
The ideal cardiovascular health metrics defined by the American Heart Association make it possible to measure progress toward the goal of ideal population-based cardiovascular health. However, according to several studies, there was an exceedingly low prevalence of ideal cardiovascular health in American adults (6,24) and studies from China also showed that only few adults achieved ideal cardiovascular health (25-27). Similarly, the results of our study showed that of the 2,482 participants, only 48 people (1.93%) reached ideal levels of all seven metrics in the northern Chinese population. Compared to participants who met only one level of metric or did not meet any metrics, those who met six or more ideal CVH metrics were less likely to have a high EATV and similar inverse relationships were found in every gender and age subgroups. To the best of our knowledge, this study is the first to demonstrate the relationship between AHA ideal CVH metrics and EATV.
The protective effect of epicardial fat on the heart is mainly dependent on paracrine or vascular secretion of anti-atherosclerotic cytokines under normal physiological conditions. However, in obesity and T2DM, if EAT becomes thicker and dysfunctional, the production and secretion of protective adipokines are down-regulated, leading to an imbalance of epicardial fat secretosome causing EAT to establish local and systemic harmful functions (8). It is plausible that these processes are reciprocal and bidirectional, some cross-sectional clinical and translational correlative studies have established to prove that. Besides, in the pathobiology of atrial fibrillation and its recurrence after ablation, EAT also plays an important role. We analyzed the relationship between each CVH metric and the prevalence of high EATV respectively, and found there was a positive correlation between ideal BMI and a lower risk of high EATV, which conforms with research in other populations, although not the Chinese population. De Vos et al. found that EAT was positively related to body mass index (P<0.001) (28) and a study consisting of 215 individuals without known CAD (the mean age was 58±11 years) found that EAT volume was directly associated with BMI (21). In some previous studies (28,29), a significant negative association between ideal fasting blood glucose and the prevalence of high EATV was reported. However, Nakazatoa et al. concluded the correlation of EATV with fasting blood glucose did not remain significant after adjustment (30). We detected a significant association between ideal fasting blood glucose and the prevalence of high EATV in the overall population, but only in male participants, which was probably due to women in this study having relatively more ideal serum, a lower prevalence of diabetes, and a lower EATV, as has been previously reported (31). In accordance with the above-mentioned outcome, it was only in younger groups (<60 years of age) and the male group that the association between ideal total cholesterol and the lower prevalence of high EATV reached a significant level respectively, although this was significant in the whole population. That the statistical significance was lost in the elder group may be because these participants were more likely to have an unhealthy level of total cholesterol. While existing studies reported that EAT correlated significantly with total cholesterol, TGs, and HDL-C in respective participants (29,30,32-34), the relationship between the EAT and serum biomarkers needs to be investigated further.
The results confirmed the correlation between health behaviors/factors and the prevalence of high EATV, which is of great significance for the general population. However, while the sample volume of our study was large and various potential confounding factors had been adjusted, there are still several limitations demanding careful consideration. First, we used a modified questionnaire survey which relied on an established food frequency questionnaire to define information on dietary intake, which may explain why our study did not find a significant relationship between diet and high EATV. Second, this study was cross-sectional, which limited our observations of the impact of AHA CVH metrics on the increase of EATV. We were unable to determine whether the existence of concerning risk factors was earlier or later than the development of EATV and whether there was a causal relationship between them. However, the research results did show there was a negative correlation between the number of ideal CVH metrics and high EATV, and further research to justify the temporal direction of the observed correlation between AHA CVH metrics and high EATV risk is required. A third limitation to this study concerns the lack of threshold values of EATV for high-risk CT measures as referred to in a previous report (23). In this study, we used 100 cm3 as the cut off value of EATV, which may be inappropriate for the northern Chinese population. Further prospective studies will involve more information in the analysis and attempt to define a reference value for the diagnosis of high EATV.
Conclusions
The results of the study confirmed there is an obvious inverse gradient relationship between the number of ideal CVH metrics and the prevalence of high EATV in the northern Chinese population, which further reflects the significance of ideal health behaviors and factors in maintaining a normal volume of EAT. The quantification of EAT by computed tomography may allow greater access to this measurement in clinical practice.
Acknowledgments
Funding: Beijing Natural Science Foundation (7192193) & Military Healthcare Grant (16BJZ15).
Footnote
Reporting Checklist: The authors have completed the STROBE reporting checklist. Available at http://dx.doi.org/10.21037/atm-21-1798
Data Sharing Statement: Available at http://dx.doi.org/10.21037/atm-21-1798
Conflicts of Interest: All authors have completed the ICMJE uniform disclosure form (available at http://dx.doi.org/10.21037/atm-21-1798). The authors have no conflicts of interest to declare.
Ethical Statement: The authors are accountable for all aspects of the work in ensuring that questions related to the accuracy or integrity of any part of the work are appropriately investigated and resolved. The research was performed following guidelines of the Helsinki Declaration (as revised in 2013) and was authorized by the local Ethics Committee of Jidong Oil-field, Inc. Medical Centers. All participants provided signed informed consent.
Open Access Statement: This is an Open Access article distributed in accordance with the Creative Commons Attribution-NonCommercial-NoDerivs 4.0 International License (CC BY-NC-ND 4.0), which permits the non-commercial replication and distribution of the article with the strict proviso that no changes or edits are made and the original work is properly cited (including links to both the formal publication through the relevant DOI and the license). See: https://creativecommons.org/licenses/by-nc-nd/4.0/.
References
- World Health Organization. Cardiovascular Diseases. World Health Organization Fact Sheet No. 317 [Online]. 2015.
- Lloyd-Jones DM, Hong Y, Labarthe D, et al. Defining and setting national goals for cardiovascular health promotion and disease reduction: the American Heart Association's strategic Impact Goal through 2020 and beyond. Circulation 2010;121:586-613. [Crossref] [PubMed]
- Bambs C, Reis SE. Embracing primordial prevention for ideal cardiovascular health. Future Cardiol 2011;7:447-50. [Crossref] [PubMed]
- Ford ES, Greenlund KJ, Hong Y. Ideal cardiovascular health and mortality from all causes and diseases of the circulatory system among adults in the United States. Circulation 2012;125:987-95. [Crossref] [PubMed]
- Bi Y, Jiang Y, He J, et al. Status of cardiovascular health in Chinese adults. J Am Coll Cardiol 2015;65:1013-25. [Crossref] [PubMed]
- Folsom AR, Yatsuya H, Nettleton JA, et al. Community prevalence of ideal cardiovascular health, by the American Heart Association definition, and relationship with cardiovascular disease incidence. J Am Coll Cardiol 2011;57:1690-6. [Crossref] [PubMed]
- Maclagan LC, Tu JV. Using the concept of ideal cardiovascular health to measure population health: a review. Curr Opin Cardiol 2015;30:518-24. [Crossref] [PubMed]
- Iacobellis G. Local and systemic effects of the multifaceted epicardial adipose tissue depot. Nat Rev Endocrinol 2015;11:363-71. [Crossref] [PubMed]
- Iacobellis G. Epicardial and pericardial fat: close, but very different. Obesity 2009;17:625. [Crossref] [PubMed]
- Iacobellis G, Malavazos AE, Corsi MM. Epicardial fat: from the biomolecular aspects to the clinical practice. Int J Biochem Cell Biol 2011;43:1651-4. [Crossref] [PubMed]
- McAninch EA, Fonseca TL, Poggioli R, et al. Epicardial adipose tissue has a unique transcriptome modified in severe coronary artery disease. Obesity 2015;23:1267-78. [Crossref] [PubMed]
- Rabkin SW. The relationship between epicardial fat and indices of obesity and the metabolic syndrome: a systematic review and meta-analysis. Metab Syndr Relat Disord 2014;12:31-42. [Crossref] [PubMed]
- Shimabukuro M, Hirata Y, Tabata M, et al. Epicardial adipose tissue volume and adipocytokine imbalance are strongly linked to human coronary atherosclerosis. Arterioscler Thromb Vasc Biol 2013;33:1077-84. [Crossref] [PubMed]
- Iacobellis G, Willens HJ. Echocardiographic epicardial fat: a review of research and clinical applications. J Am Soc Echocardiogr 2009;22:1311-9; quiz 1417-8. [Crossref] [PubMed]
- Nelson AJ, Worthley MI, Psaltis PJ, et al. Validation of cardiovascular magnetic resonance assessment of pericardial adipose tissue volume. J Cardiovasc Magn Reson 2009;11:15. [Crossref] [PubMed]
- Sarin S, Wenger C, Marwaha A, et al. Clinical significance of epicardial fat measured using cardiac multislice computed tomography. Am J Cardiol 2008;102:767-71. [Crossref] [PubMed]
- Song Q, Liu X, Wang A, et al. Associations between non-traditional lipid measures and risk for type 2 diabetes mellitus in a Chinese community population: a cross-sectional study. Lipids Health Dis 2016;15:70. [Crossref] [PubMed]
- Hao Z, Zhang Y, Li Y, et al. The Association between Ideal Cardiovascular Health Metrics and Extracranial Carotid Artery Stenosis in a Northern Chinese Population: A Cross-Sectional Study. Sci Rep 2016;6:31720. [Crossref] [PubMed]
- Wang TD, Lee WJ, Shih FY, et al. Association of epicardial adipose tissue with coronary atherosclerosis is region-specific and independent of conventional risk factors and intra-abdominal adiposity. Atherosclerosis 2010;213:279-87. [Crossref] [PubMed]
- Maurovich-Horvat P, Kallianos K, Engel LC, et al. Influence of pericoronary adipose tissue on local coronary atherosclerosis as assessed by a novel MDCT volumetric method. Atherosclerosis 2011;219:151-7. [Crossref] [PubMed]
- Bettencourt N, Toschke AM, Leite D, et al. Epicardial adipose tissue is an independent predictor of coronary atherosclerotic burden. Int J Cardiol 2012;158:26-32. [Crossref] [PubMed]
- Yoshizumi T, Nakamura T, Yamane M, et al. Abdominal fat: standardized technique for measurement at CT. Radiology 1999;211:283-6. [Crossref] [PubMed]
- Oka T, Yamamoto H, Ohashi N, et al. Association between epicardial adipose tissue volume and characteristics of non-calcified plaques assessed by coronary computed tomographic angiography. Int J Cardiol 2012;161:45-9. [Crossref] [PubMed]
- Bambs C, Kip KE, Dinga A, et al. Low prevalence of "ideal cardiovascular health" in a community-based population: the heart strategies concentrating on risk evaluation (Heart SCORE) study. Circulation 2011;123:850-7. [Crossref] [PubMed]
- Wu HY, Sun ZH, Cao DP, et al. Cardiovascular health status in Chinese adults in urban areas: analysis of the Chinese Health Examination Database 2010. Int J Cardiol 2013;168:760-4. [Crossref] [PubMed]
- Zeng Q, Dong SY, Song ZY, et al. Ideal cardiovascular health in Chinese urban population. Int J Cardiol 2013;167:2311-7. [Crossref] [PubMed]
- Wu S, Huang Z, Yang X, et al. Prevalence of ideal cardiovascular health and its relationship with the 4-year cardiovascular events in a northern Chinese industrial city. Circ Cardiovasc Qual Outcomes 2012;5:487-93. [Crossref] [PubMed]
- de Vos AM, Prokop M, Roos CJ, et al. Peri-coronary epicardial adipose tissue is related to cardiovascular risk factors and coronary artery calcification in post-menopausal women. Eur Heart J 2008;29:777-83. [Crossref] [PubMed]
- Kerr JD, Holden RM, Morton AR, et al. Associations of epicardial fat with coronary calcification, insulin resistance, inflammation, and fibroblast growth factor-23 in stage 3-5 chronic kidney disease. BMC Nephrol 2013;14:26. [Crossref] [PubMed]
- Nakazato R, Rajani R, Cheng VY, et al. Weight change modulates epicardial fat burden: a 4-year serial study with non-contrast computed tomography. Atherosclerosis 2012;220:139-44. [Crossref] [PubMed]
- Iacobellis G, Singh N, Wharton S, et al. Substantial changes in epicardial fat thickness after weight loss in severely obese subjects. Obesity 2008;16:1693-7. [Crossref] [PubMed]
- Wang CP, Hsu HL, Hung WC, et al. Increased epicardial adipose tissue (EAT) volume in type 2 diabetes mellitus and association with metabolic syndrome and severity of coronary atherosclerosis. Clin Endocrinol (Oxf) 2009;70:876-82. [Crossref] [PubMed]
- Yerramasu A, Dey D, Venuraju S, et al. Increased volume of epicardial fat is an independent risk factor for accelerated progression of sub-clinical coronary atherosclerosis. Atherosclerosis 2012;220:223-30. [Crossref] [PubMed]
- Wang TD, Lee WJ, Shih FY, et al. Relations of epicardial adipose tissue measured by multidetector computed tomography to components of the metabolic syndrome are region-specific and independent of anthropometric indexes and intraabdominal visceral fat. J Clin Endocrinol Metab 2009;94:662-9. [Crossref] [PubMed]
(English Language Editor: B. Draper)