SMOX expression predicts the prognosis of non-small cell lung cancer
Introduction
Non-small cell lung cancer (NSCLC) accounts for a large proportion of lung cancer. Because the diagnosis is late, the time window for surgical treatment is lost. There are several treatment options for advanced NSCLC, including targeted therapy, chemoradiotherapy, immunotherapy, chemoimmunotherapy, and radiofrequency ablation. However, the prognosis of advanced NSCLC is not ideal due to local and distant metastasis. Therefore, finding a therapeutic target for NSCLC can help realize the diagnosis and treatment of NSCLC at an early stage, thereby greatly improving the prognosis of NSCLC (1).
SMOX, which metabolizes polyamine spermine to spermidine and hydrogen peroxide (HO), is associated with an increased risk of gastric cancer in humans (2). There is evidence that reactive oxygen species (ROS) mediate tissue homeostasis, cell signaling, differentiation, and survival, and ROS are the basis of angiogenesis and tumor growth. In cancer, angiogenesis is a key phenomenon in developing new blood vessels to promote the supply of oxygen and nutrients for tumor progression. Abnormally increased ROS is closely related to the development and metastasis of cancer. Since HO is the main component of ROS, molecular imaging and selective treatment of high HO levels are very attractive for cancer treatment.
Furthermore, SMOX is a highly inducible enzyme whose dysregulation alters the homeostasis of polyamines. Oxidation products of polyamine metabolism can induce cell damage and death. This is also one of the reasons why we chose SMOX for research.
There is a complex and inevitable connection between tumors and immunity (3). At present, immune responses and immune escape have become more widely recognized mechanisms in tumors. Immune responses mainly include humoral immunity and cellular immunity, and cellular immunity is the main tumor immune response. As a specific immune response, it mainly produces an immune response to solid tumor cells with strong antigenicity, and humoral immunity plays a synergistic effect. As a non-specific immune response, circulating antibodies and the like mainly produce immune responses against tumor cells that are weakly antigenic and in a free state.
In contrast, the mechanism of tumor immune escape involves immune selection, antigen modulation, tumor-induced immune suppression, and blocking factors. Immune selection means that tumor cells with strong antigenicity can cause an effective immune response and be eliminated, and tumor cells with relatively weak antigenicity can escape the immune system’s surveillance and proliferate. Antigen modulation refers to the reduction or loss of antigenicity on the surface of tumor cells to avoid the recognition and attack of the host immune system. Tumor-induced immunosuppression means that when tumor cells first appear, the amount of antigens is small and weak, and continuous stimulation of the host can induce immune tolerance and make the host lose the ability to respond to the tumor. Finally, blocking factors refer to factors that cause immune promotion, including blocking antibodies, excessive free antigens, and antigen-antibody complexes. Immune responses have been considered major components of tumor elimination in radiotherapy, and radiation-induced tumor injury promotes radiotherapy vaccination, enhances cytotoxic immune responses, and enhances immunotherapy. The efficacy of immunotherapy depends on the degree of infiltration of immune cells in the immune microenvironment, a complex ecosystem formed by unique and interacting cell populations. Its composition is related to the prognosis of cancer and the response to clinical treatment. The tumor microenvironment (TME) interacts with tumor cells through various cytokines and cytokine receptors to regulate their stemness. The main obstacle of immunotherapy is that effector immune cells cannot fully penetrate the TME. Therefore, exploring the mechanism of the TME of NSCLC is beneficial to improve immunotherapy further. The discovery of prognostic markers is helpful for early diagnosis and treatment, and the combination of the 2 greatly improves the predictive performance for NSCLC. We present the following article following the REMARK reporting checklist (available at http://dx.doi.org/10.21037/atm-21-998).
Methods
Source and collation of data
The data involved in our research came from The Cancer Genome Atlas (TCGA) and Gene Expression Omnibus (GEO) databases, including genetic transcriptome analysis and clinical information. The survival prognosis information of patients was an important basis for our selection of TCGA and GEO datasets. Among them, GSE41271 contained 275 tumor samples, GSE42127 contained 176 tumor samples, and GSE68465 contained 443 samples. The TCGA datasets contained 999 tumor samples. Downloaded transcriptome data were standardized and corrected before analysis, and samples with incomplete information were deleted when the clinical information was integrated.
Establishment and verification of independent prognostic factors
Univariate and multivariate Cox regression analyses were used to calculate 95% confidence interval (CI) and hazard ratio (HR), aiming to determine independent prognostic factors for lung cancer. Univariate survival analysis was used to analyze clinical factors and survival rates, while multivariate survival analysis comprehensively evaluated the relationship between multiple clinical factors and overall survival rates. P value <0.05 was generally considered to be statistically significant. The significance of the Kaplan-Meier survival curve was to show the relationship between the expression of SMOX and the survival of lung cancer. We divided various clinical factors into different subgroups, showed the effect size of the test factors in each subgroup through forest plots, and even visually displayed the difference between each subgroup (4).
Functional enrichment analysis
We performed gene set enrichment analysis (GSEA) on RNA-seq data in the TCGA database, which aimed to further understand the biological function of SMOX by analyzing Gene Ontology (GO) terms and Kyoto Encyclopedia of Genes and Genomes (KEGG) pathways (5,6). The number of permutations was set to 1000. Other parameters were set based on their default values. The enrichment pathways in each phenotype were classified based on the nominal P value and the normalized enrichment score (NES). By extending the GSEA, singe sample GSEA (SSGSEA) allows the definition of an enrichment fraction that represents the absolute enrichment of a gene set in each sample within a given dataset.
Immune infiltration analysis
CIBERSORT is a method used to characterize the cell composition of complex tissues from the gene expression profile. When applied to hematopoietic subpopulations in RNA mixtures extracted from fresh, frozen, and fixed tissues (including solid tumors), CIBERSORT outperforms other methods in terms of noise, unknown mixture content, and closely related cell types. CIBERSORT can perform large-scale analysis of RNA mixtures used for cell biomarkers and therapeutic targets (http://cibersort.stanford.edu). CIBERSORT is also a deconvolution algorithm based on gene expression that can be used to evaluate samples. The variation among the 22 tumor-infiltrating immune cells reflects the correlation. Through Monte Carlo sampling, CIBERSORT approximately sets the p value for deconvolution to determine the confidence of the result. Monte Carlo sampling is a random approximate inference method. In essence, it transforms the longitudinal distribution of the sample in space into the equivalent distribution of the sample in the horizontal direction. The screening condition for further analysis was CIBERSORT P<0.05. Tumor mutational burden (TMB) refers to the total number of mutations per megabase in tumor tissues, the mutation density of tumor genes, including the total number of gene coding errors, and splicing and gene insertion or deletion errors. The higher the TMB, the higher the characteristic of the tumor, the more obvious the difference from normal tissues, and the more effective the immunotherapy (7).
Western blotting analysis
Proteins were extracted from tissues using 1× radioimmunoprecipitation. The lysis buffer was assayed with protease inhibitors. The samples were loaded on a 10% SDS polyacrylamide gel for electrophoresis and transferred to polyvinylidene fluoride film. Protein bands were identified by visualized primary antibodies using the Odyssey Infrared Imaging System (LI-COR Biological Sciences), and images were used for optical density analysis (8).
Reverse transcription-polymerase chain reaction (RT-PCR)
Total RNAs were extracted from the patients’ tissues according to the instructions of the product specification. Reverse transcription of 1 µg of total RNA was performed using the M-MLV reverse transcriptase product (Promega). The ABI7500 real-time PCR system (Thermo Fisher Science) was used to perform RT-qPCR. Glyceraldehyde 3-phosphate dehydrogenase (GAPDH) was used as the internal control, and the level of SMOX was detected by RT-qPCR as follows: denatured at 94 °C for 5 s, annealed, and extended at 62 °C for 40 s for PCR amplification. The 1 Ct method was used to calculate the relative expression level of SMOX. Simply put, the difference between the GAPDH Ct value and the SMOX Ct value was defined as the 1Ct value. A high 1Ct value was considered to indicate a relatively low expression of SMOX in each sample. All PCR amplifications were performed in triplicate, and 3 independent experiments were repeated (9). SMOX forward primer was cag tgg tga cag tgc gga tga c. SMOX reverse primer was acc ctg ctc aag tgc ttt gg.
Immunohistochemical analysis
Lung cancer tissue sections were provided by the Affiliated Hospital of Nantong University. After lung cancer tissue was deparaffinized, hydrated, and blocked, SMOX antibody (1:1,000, Proteintech) was added dropwise and incubated overnight at 4 °C. Sections were then washed with phosphate-buffered saline (PBS) 3 times and then incubated with horseradish peroxidase (HRP) goat anti-rabbit IgG polyclonal antibody at room temperature for 30 minutes. DAB and hematoxylin were used to stain tissue slides. SMOX expression in lung cancer tissues was estimated by software (Image-Pro Plus 6.0) and evaluated by 2 independent investigators who did not know clinical information, and consensus resolved the disagreements. When the results of the two investigators diverged, a third investigator was invited to analyze (10).
Statistical analysis
One-way ANOVA and the Kruskal-Wallis test, non-parametric and parametric methods, were used to compare 2 or more groups. Spearman and distance correlation analysis was used to calculate the correlation coefficient. To identify genes in the analysis of differential genes, the Benjamini-Hochberg method converted the P value to false discovery rate (FDR). P values were two-sided, and P values of less than 0.05 were regarded as statistically significant.
Ethics declaration
The study was conducted in accordance with the Declaration of Helsinki (as revised in 2013). The study was approved by ethics board of Affiliated Hospital of Nantong University (No.: 2020-L002).
Results
Establishment of independent prognostic factors in the GEO Database
We obtained genome-wide expression from multiple datasets (GSE41271, GSE42127, and GSE68465) in the GEO database, aiming to reflect the function of mRNAs in lung cancer. After annotating the expression data and sorting out the clinical data, the dataset could be subjected to survival analysis, independent prognosis, and clinical relevance filtering. After a series of screenings, GSE41271 screened out 466 independent prognostic factors, GSE42127 screened out 300 independent prognostic factors, while GSE68465 screened out 341 independent prognostic factors. A total of 16 common genes were obtained by taking the intersection of the 3 through a Venn diagram (Figure 1A, Table 1). The correlation of these 16 prognostic genes in the TCGA database was shown through a network diagram, and the selected correlation threshold was 0.3 (Figure 1B). Since the functional properties of most of these genes have been thoroughly explored, while the functional properties of SMOX needed further exploration, we chose the SMOX gene and explored its functional role in lung cancer. The survival analysis curves of SMOX in the 3 datasets are shown in Figure 1C. Simultaneously, univariate and multivariate Cox regression analyses found that SMOX was indeed an independent prognostic factor for lung cancer and could be displayed in forest plots (Figure 1D,E).
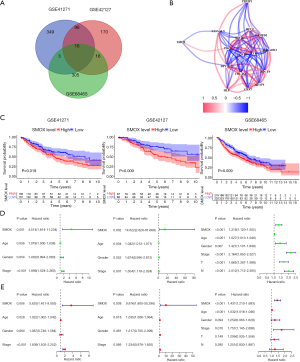

Full table
Clinical correlation analysis
The TNM staging of lung cancer in the clinical staging of the location, size, and spread of lung cancer. T contains T1-T4, N contains N1-N3, and M contains M0-M1. All 3 datasets contained stage information, but GSE42127 and GSE685465 only contained stages I-III, as there were no patients with stage IV. It is worth noting that only patients in GSE68465 had clinical information of T and N. Since no patients had N3 disease, they were classified as N0-N2. Clinical correlation analysis displayed that in the GSE41271 dataset, there were significant differences in the expression of SMOX by gender and stage (stage I compared to stage II, stage II compared to stage III). In the GSE42127 dataset, the only stage made a difference in SMOX expression (stage I compared to stage II). In the GSE68465 dataset, T, N, and stage all made a difference to SMOX expression (T1 compared to T2, N0 compared N1, stage I compared to stage II) (Figure 2) (11).
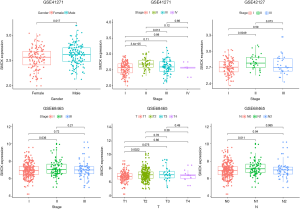
Functional enrichment analysis of differential genes in GSE68465
We obtained 389 differential genes from GSE68465, and the screening criteria were |log2FC| >0.5 and P value <0.05 (Figure 3A,B). We conducted GO enrichment analysis to understand further the location, function, and biological pathways of gene products in cells. The most enriched pathways were organelle fission, nuclear division, chromosome segregation, mitotic nuclear division, nuclear chromosome segregation, sister chromatid segregation, mitotic sister chromatid segregation, and DNA replication (Figure 3C). Also, we learned about related metabolic pathways through KEGG enrichment analysis, such as cell cycle, phagosome, oocyte meiosis, DNA replication, and homologous recombination (Figure 3D). The most significant 60 differential genes were used to construct the protein interaction network and were visualized by Cytoscape7.2 software, including 27 up-regulated genes and 33 down-regulated genes (Figure 3E) (12).

Validation of SMOX in the TCGA Dataset
A total of 102 pairs of lung cancer and adjacent tissues in the TCGA database had significant differences (Figure 4A). Simultaneously, in the TCGA database, the expression of SMOX in 103 normal tissues and 999 lung cancer tissues was significantly different (Figure 4B). The overall survival rate of SMOX in the low expression group was better than that of the high expression group (P=0.004) (Figure 4C). After univariate and multivariate Cox regression analyses, we determined that SMOX was indeed an independent prognostic factor for lung cancer in the TCGA dataset (Figure 4D). Also, it was worth noting that the expression of SMOX differed significantly in terms of gender and T stage (Figure 4E). GSEA contributed to showing the GO and KEGG pathways related to SMOX expression. In GO term annotation, 5 categories were positively related to the low expression group: regulation of presynapse organization, glucose catabolic process, wide pore channel activity, negative regulation of epidermis development, and glycolytic process through fructose 6 phosphate. Furthermore, 5 categories were negatively associated with the low expression group: branched-chain amino acid metabolic process, fatty acid beta-oxidation using acyl CoA oxidase, fatty acid catabolic process, immunoglobulin binding, and response to the tumor cell. Additionally, in the KEGG pathways, the 5 pathways that were positively correlated with the low expression group were small cell lung cancer, WNT signaling pathway, P53 signaling pathway, ERBB signaling pathway, and pathways in cancer. In comparison, the 5 pathways that were negatively related to the low expression group were FC epsilon RI signaling pathway, autoimmune thyroid disease, PPAR signaling pathway, GNRH signaling pathway, and vascular smooth muscle contraction (Figure 4F) (13-15).
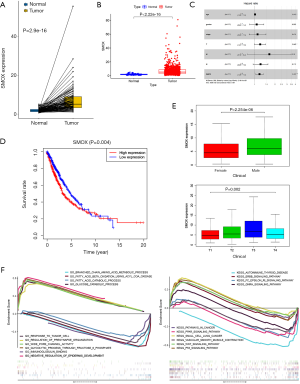
Immune infiltration analysis
We analyzed the prognostic efficacy of radiotherapy and chemotherapy patients in the TCGA database and found that there were significant differences between the drug efficacy indicators (Figure 5A). Since GSEA showed that SMOX was involved in some immune-related pathways, to explore the role of SMOX in the immune microenvironment, we conducted immune infiltration analysis in GSE68465, TCGA, respectively. In GSE68465, resting mast cells and resting dendritic cells showed the highest positive correlation, while CD4 memory resting T cells and CD8 T cells displayed the highest negative correlation (Figure 5B). In the TCGA data set, CD4 memory activated T cells and CD8 T cells displayed the highest positive correlation, while CD8 T cells and M0 macrophages showed the highest negative correlation (Figure 5C). Various immune cells showed a certain correlation with SMOX in the immune microenvironment, such as regulatory T cells, resting CD4 memory T cells, activated CD4 memory T cells, resting NK cells, monocytes, M0 Macrophages, M2 macrophages, resting mast cells, resting dendritic cells, and activated dendritic cells (Figure 5D). Furthermore, the distribution of the two groups in term of some immune cells was different. In GSE68465, naive B cells, memory B cells, plasma cells, CD4 memory resting T cells, CD4 memory activated T cells, follicular helper T cells, resting NK cells, monocytes, M0 macrophages, M2 macrophages, resting dendritic cells, resting mast cells, activated mast cells, and neutrophils showed significant differences between two groups (Figure 5E). CD4 memory resting T cells, regulatory T cells, resting NK cells, activated NK cells, Monocytes, M0 macrophages, M2 macrophages, resting dendritic cells, resting mast cells, and activated mast cells displayed differences between the two groups in the TCGA cohort (Figure 5F). It was worth noting that most of the immune cells with differences in GSE68465 and TCGA were the same (16,17). To further analyze the immune microenvironment of lung cancer, we calculated the immune and matrix scores of the patients and divided the patients into two groups according to the median value of the expression of SMOX. Significant differences were found in the immune and stromal scores between the two groups (Figure 6A,B). According to the median values of the immune score and matrix score, patients were divided into high and low-risk groups, and the top 50 differential genes with the most significant differences were shown in a heat map (Figure 6C,D). Secondly, the differential genes obtained through immune scores and matrix scores were crossed, and it was found that there were 1,222 differential up-regulated genes and 442 differential down-regulated genes (Figure 6E). The enrichment analysis of differential genes found that GO annotation was mainly enriched in immune response-activating cell surface receptor signal transduction and lymphocyte-mediated immunity, among others (Figure 6F). KEGG pathway enrichment analysis showed that the main enrichment was in cytokine-cytokine receptor interaction, staphylococcus aureus infection, and hematopoietic cell lineage, among others (Figure 6G). Costimulatory molecules are components involved in transmitting costimulatory signals, affecting the activation of T lymphocytes and B lymphocytes, which was fully verified in a violin plot. The expression level of costimulatory molecules was extracted from the GEO dataset and TCGA dataset, respectively. By dividing the costimulatory molecules into two groups according to the level of SMOX expression and comparing the differences between the two groups, in these 4 datasets, 9 costimulatory molecules were different between the two groups. They were CD40LG, HLA-DMA, HLA-DMB, HLA-DPA1, HLA-DPB1, HLA-DQB1, HLA-DQB2, HLA-DRA, and HLA-DRB6 (Figure 7A,B,C,D). Immune checkpoints refer to a series of molecules expressed on immune cells regulating the degree of immune activation. They play an important role in preventing autoimmunity (abnormal immune function, attacking normal cells). We analyzed the differences in genes related to immune checkpoints in the SMOX high and low expression groups and reflected them in heat maps (Figure 7E). It is worth noting that there were significant differences between the high and low groups of SMOX expression in terms of TMB (Figure 7F). Eventually, the scores of immune cells and immune-related functions in each sample were obtained through SSGSEA analysis (18). In the correlation analysis of the immune score, SMOX expression in the high and low groups showed significant differences in terms of the correlation of immune cells (Figure 7G) and immune functions (Figure 7H).
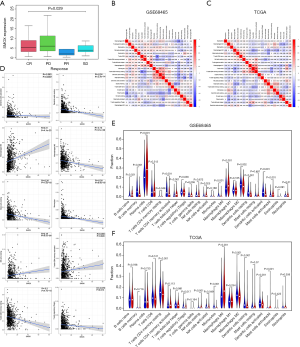
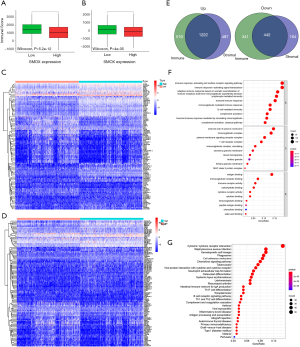
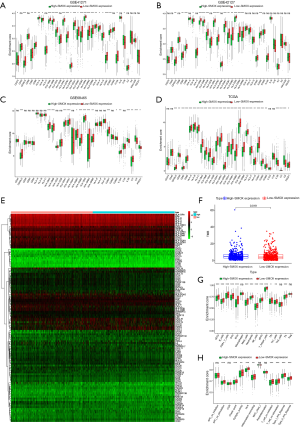
External experimental verification
Predictions from the GEO and TCGA databases found that patients with low SMOX expression survive longer. It was speculated that the expression of SMOX in adjacent tissues was lower than that in tumor tissues. To further verify the expression of SMOX in NSCLC, western blot analysis was performed. It was found that SMOX had high-level expression in most of the 24 pairs of NSCLC tumor and paracancerous tissues, while it had low-level expression in paracancerous tissues (Figure 8A,B) (19). Notably, in 24 pairs of NSCLC tumor tissues and paracancerous tissues, SMOX had high-level expression in tumor tissues and low-level expression in normal tissues determined by RT-PCR analysis (Figure 8C) (20,21). Immunohistochemical analysis showed that SMOX was indeed highly expressed in 155 NSCLC patients (Figure 8D) (22). Furthermore, analysis of survival data from 155 NSCLC patients showed that there was a significant difference in the survival prognosis in the low-SMOX expression group, and patients with low SMOX expression had a better survival prognosis (Figure 8E). The median value of SMOX expression divided patients into high and low expression groups (Table S1). Finally, the forest plot showed that SMOX was identified as an independent prognostic factor for 155 NSCLC patients (Figure 8F,G).
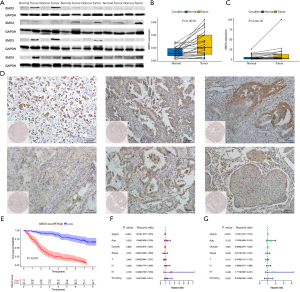
In conclusion, SMOX can be used as a predictive target for NSCLC.
Discussion
In recent years, the incidence and mortality of lung cancer have increased rapidly. The 5-year survival rate of advanced lung cancer is less than 10%. Therefore, the early detection of lung cancer is extremely important. Although there are different research directions, the ultimate goal is to diagnose and treat lung cancer. Basal cells are stem/progenitor cells that maintain airway homeostasis and repair after epithelial injury and are candidate source genes for lung squamous cell carcinoma (23), while alveolar type 2 (AT2) cells are the origin cells for lung adenocarcinoma (24). Circulating tumor cells (CTCs) are considered a valuable marker for early diagnosis, prognosis, and risk stratification in cancer patients, and they have been found to predict survival in patients with various types of cancer, including lung cancer (25). These studies aimed to understand the precursors of lung cancer and identify molecular changes during its development to facilitate early detection and better molecular targeting in the early stages of the disease.
The TME is mainly composed of many stromal cells, including immune cells, which are now considered key regulators of tumor development. According to the theory of cancer immunoediting, immune cells can kill tumor cells, especially in the early stages of tumor development, thereby reducing tumor incidence (26). Tumor evolution often leads to many heterogeneous cancer cell types in a single tumor, and heterogeneity includes epigenetic and gene expression changes, which cannot be identified by histological characteristics alone. Cancer cells invade nearby healthy tissues and the infiltration of immune cells, resulting in more complex structures of tumor and non-tumor cells (27). The extensive deletion of immunomodulatory genes is the reason for the low immune infiltration in cold immune tumors. The harmonious up-regulation of multiple immune checkpoint proteins may be due to the resistance of anti-programmed cell death protein 1 monotherapy on immune-hot tumors (28). Inflammatory cytokines in the TME promote immune cell infiltration. Infiltration of immune and peritumor stromal cells supports tumor growth, angiogenesis, metastasis, and immunosuppression through communication with inflammatory cytokines and cell adhesion molecules. Notably, the infiltrating immune cells and tumor cells have immunosuppressive molecules, such as programmed death ligand 1 (PD-L1) and CD80/CD86. Inhibition of cytotoxic T cells promotes tumor avoidance of immune surveillance and larger malignancies. Also, glycosylation and sialylation of proteins overexpressed on the surface of cancer cells have been shown to enhance immune escape and metastasis (29). The intricate interactions between malignant cells, host cells, and non-cellular components play key roles in different stages of tumor development, progression, and metastasis. Tumor cells and stromal cells communicate through the secretion of signaling molecules such as integrins and other receptors and growth factors, cytokines, chemokines, and inflammatory mediators.
The signaling pathway mediated by chemokines has become the main mechanism for host cells to play multiple roles in tumor development. In response to tumor stimulation, chemokines derived from host cells further activate the signaling cascade, supporting the ability of tumor cells to invade the surrounding basement membrane and extracellular matrix. Host-derived chemokines act on endothelial cells to increase their permeability and promote the infiltration and extravasation of tumor cells. The interaction between tumor cells and host neutrophils in the vascular system initiates chemokines to drive the recruitment of inflammatory cells, protecting CTCs from immune attack (30). Also, by stimulating the body’s immune system, cancer immunotherapy has emerged as a promising strategy for treating many types of cancer. This therapy not only eradicates tumor cells by inducing a strong anti-tumor immune response but also prevents tumor recurrence (31). The metabolism of cancer cells is usually very different from that of normal cells. However, in tumor masses, the continuous interference and competition between different cells for nutrition and oxygen lead to metabolic changes in cancer cells and different stromal cells and immune cells in the TME. This is highly correlated with tumor progression (32).Human leukocyte antigen-DM (HLA-DM) is a component of the major histocompatibility complex class II (MHCII) antigen processing and presentation pathway.
HLA-DM shapes the immune system by differentially catalyzing peptide exchange on MHCII molecules to edit the peptide-MHCII (pMHCII) repertoire by imposing a bias on the foreign and self-derived peptide cargos which are presented on the cell surface for immune surveillance and tolerance induction by CD4+ T cells (33). The HLA complex contains the most polymorphic genes in the human genome. HLA class II genes define the specificity of the adaptive immune response. Hereditary variations in the HLA gene are associated with autoimmunity and susceptibility to infectious diseases (34). HLA-DPA1/DPB1 plays a key role in antigen presentation to CD4(+) helper T cells. Furthermore, inflammation can provide biologically active molecules for the TME, and the products of the inflammatory process can be considered as potential biomarkers. Studies have shown that neutrophil-to-lymphocyte ratio (NLR) and systemic immune-inflammation index (SII) are prognostic factor for NSCLC.
Corresponding studies have shown the ability of SMOX to produce oxidative cell stress, thereby driving cells into the initial stage of repair, which means SMOX induces cellular stress via an imbalance in ROS generation, but not apoptosis (35). Also, several studies have shown that SMOX plays an integral role in various diseases, but its role in NSCLC has not been reported. SMOX is associated with gastric inflammation and carcinogenesis and can be up-regulated in patients with inflammatory bowel disease. SMOX has an immunomodulatory role in experimental colitis polyamine flux (36). Simultaneously, SMOX promotes Helicobacter pylori induced cancer by causing inflammation, DNA damage, and activation of beta-catenin signaling (37). In naive mice, SMOX knockdown leads to airway hyperresponsiveness, remodeling, and apoptosis of bronchial epithelial cells (38). Also, SMOX is involved in diabetic retinal neurodegeneration and visual dysfunction (39). Related studies have shown that molecules with a high SMOX correlation have an important association with NSCLC. Polycomb group genes (PCGs) are epigenetic effectors, and PCGs are overexpressed in many cancers. They are a hot topic in cancer research and are essential for stem cell self-renewal and multi-performance (40). Dihydropyrimidinase-like proteins (DPYSLs) are a family of proteins that are developmentally regulated during nervous system maturation. Members of the DPYSL family are involved in cancer. Low expression of DPYSL1 in NSCLC is associated with adverse clinical outcomes and has a metastatic inhibitory effect (41).
Acknowledgments
Funding: This work was supported by the National Natural Science Foundation of China (81770266) and “Six-one” Project for High-level Health Talents (LGY2016037) in Jiangsu, China.
Footnote
Reporting Checklist: The authors have completed the REMARK reporting checklist. Available at http://dx.doi.org/10.21037/atm-21-998
Conflicts of Interest: All authors have completed the ICMJE uniform disclosure form (available at http://dx.doi.org/10.21037/atm-21-998). The authors have no conflicts of interest to declare.
Ethical Statement: The authors are accountable for all aspects of the work in ensuring that questions related to the accuracy or integrity of any part of the work are appropriate investigated and resolved. The study was conducted in accordance with the Declaration of Helsinki (as revised in 2013). The study was approved by ethics board of Affiliated Hospital of Nantong University (No.: 2020-L002).
Open Access Statement: This is an Open Access article distributed in accordance with the Creative Commons Attribution-NonCommercial-NoDerivs 4.0 International License (CC BY-NC-ND 4.0), which permits the non-commercial replication and distribution of the article with the strict proviso that no changes or edits are made and the original work is properly cited (including links to both the formal publication through the relevant DOI and the license). See: https://creativecommons.org/licenses/by-nc-nd/4.0/.
References
- Dong RF, Zhu ML, Liu MM, et al. EGFR mutation mediates resistance to EGFR tyrosine kinase inhibitors in NSCLC: From molecular mechanisms to clinical research. Pharmacol Res 2021;167:105583 [Crossref] [PubMed]
- van Veen S, Martin S, Van den Haute C, et al. ATP13A2 deficiency disrupts lysosomal polyamine export. Nature 2020;578:419-24. [Crossref] [PubMed]
- Giraud J, Chalopin D, Blanc JF, et al. Hepatocellular Carcinoma Immune Landscape and the Potential of Immunotherapies. Front Immunol 2021;12:655697 [Crossref] [PubMed]
- Zhang Y, Xu M, Sun Y, et al. Identification of LncRNAs Associated With FOLFOX Chemoresistance in mCRC and Construction of a Predictive Model. Front Cell Dev Biol 2021;8:609832 [Crossref] [PubMed]
- Chen HQ, Chen DJ, Li Y, et al. DNA methylation and hydroxymethylation associated with gene expression regulatory network during 3-methylcholanthrene induced lung cell malignant transformation. Sci Total Environ 2021;771:144839 [Crossref] [PubMed]
- Liu J, Hou S, Wang J, et al. A Prognostic 14-Gene Expression Signature for Lung Adenocarcinoma: A Study Based on TCGA Data Mining. Oxid Med Cell Longev 2020;2020:8847226 [Crossref] [PubMed]
- Hu K, Li J, Wu G, et al. The novel roles of virus infection-associated gene CDKN1A in chemoresistance and immune infiltration of glioblastoma. Aging (Albany NY) 2021;13:6662-80. [Crossref] [PubMed]
- Ni S, Li J, Qiu S, et al. KIF21B Expression in Osteosarcoma and Its Regulatory Effect on Osteosarcoma Cell Proliferation and Apoptosis Through the PI3K/AKT Pathway. Front Oncol 2021;10:606765 [Crossref] [PubMed]
- Liang H, Xu M, Xiong Z, et al. Identification of miRNAs as diagnostic and prognostic markers in hepatocellular carcinoma. Aging (Albany NY) 2021;13:6115-33. [Crossref] [PubMed]
- Song Y, Wang J, Sun J, et al. Screening of Potential Biomarkers for Gastric Cancer with Diagnostic Value Using Label-free Global Proteome Analysis. Genomics Proteomics Bioinformatics 2021; [Crossref] [PubMed]
- Cai X, Qiu W, Qian M, et al. A Candidate Prognostic Biomarker Complement Factor I Promotes Malignant Progression in Glioma. Front Cell Dev Biol 2021;8:615970 [Crossref] [PubMed]
- Yu K, Li D, Xu F, et al. IDO1 as a new immune biomarker for diabetic nephropathy and its correlation with immune cell infiltration. Int Immunopharmacol 2021;94:107446 [Crossref] [PubMed]
- Yu F, Liang M, Wu W, et al. Upregulation of Long Non-Coding RNA GCC2-AS1 Facilitates Malignant Phenotypes and Correlated With Unfavorable Prognosis for Lung Adenocarcinoma. Front Oncol 2021;10:628608 [Crossref] [PubMed]
- Wu WL, Li S, Zhao GJ, et al. Identification of circular RNAs as novel biomarkers and potentially functional competing endogenous RNA network for myelodysplastic syndrome patients. Cancer Sci 2021;112:1888-98. [Crossref] [PubMed]
- Zhu P, Feng R, Lu X, et al. Diagnostic and prognostic values of AKR1C3 and AKR1D1 in hepatocellular carcinoma. Aging (Albany NY) 2021;13:4138-56. [Crossref] [PubMed]
- Kim A, Wu X, Allende DS, et al. Gene deconvolution reveals aberrant liver regeneration and immune cell infiltration in alcohol-associated hepatitis. Hepatology 2021; [Crossref] [PubMed]
- Zeng H, Liu X, Zhang Y. Identification of Potential Biomarkers and Immune Infiltration Characteristics in Idiopathic Pulmonary Arterial Hypertension Using Bioinformatics Analysis. Front Cardiovasc Med 2021;8:624714 [Crossref] [PubMed]
- Yan H, Chen Y, Yang Z, et al. An Immune Cell Signature Is Associated With Disease-Free Survival and Adjuvant Chemosensitivity of Patients With Resectable Gastric Cancer. Front Immunol 2021;11:621623 [Crossref] [PubMed]
- Wang X, Xia X, Xu E, et al. Estrogen Receptor Beta Prevents Signet Ring Cell Gastric Carcinoma Progression in Young Patients by Inhibiting Pseudopodia Formation via the mTOR-Arpc1b/EVL Signaling Pathway. Front Cell Dev Biol 2021;8:592919 [Crossref] [PubMed]
- Zhang C, Wang X, Fang D, et al. STK39 is a novel kinase contributing to the progression of hepatocellular carcinoma by the PLK1/ERK signaling pathway. Theranostics 2021;11:2108-22. [Crossref] [PubMed]
- Vandierendonck A, Degroote H, Vanderborght B, et al. NOX1 inhibition attenuates the development of a pro-tumorigenic environment in experimental hepatocellular carcinoma. J Exp Clin Cancer Res 2021;40:40. [Crossref] [PubMed]
- Fassan M, Milione M, Maddalena G, et al. Synaptophysin expression in (V600EBRAF-)mutated advanced colorectal cancers identifies a new subgroup of tumours with worse prognosis. Eur J Cancer 2021;146:145-54. [Crossref] [PubMed]
- Hynds RE, Janes SM. Airway Basal Cell Heterogeneity and Lung Squamous Cell Carcinoma. Cancer Prev Res (Phila) 2017;10:491-3. [Crossref] [PubMed]
- Sainz de Aja J, Dost AFM, Kim CF. Alveolar progenitor cells and the origin of lung cancer. J Intern Med 2021;289:629-35. [Crossref] [PubMed]
- Yousefi M, Ghaffari P, Nosrati R, et al. Prognostic and therapeutic significance of circulating tumor cells in patients with lung cancer. Cell Oncol (Dordr) 2020;43:31-49. [Crossref] [PubMed]
- Strack E, Rolfe PA, Fink AF, et al. Identification of tumor-associated macrophage subsets that are associated with breast cancer prognosis. Clin Transl Med 2020;10:e239 [Crossref] [PubMed]
- Pierce SE, Kim SH, Greenleaf WJ. Finding needles in a haystack: dissecting tumor heterogeneity with single-cell transcriptomic and chromatin accessibility profiling. Curr Opin Genet Dev 2021;66:36-40. [Crossref] [PubMed]
- Huang C, Chen L, Savage SR, et al. Proteogenomic insights into the biology and treatment of HPV-negative head and neck squamous cell carcinoma. Cancer Cell 2021;39:361-79.e16. [Crossref] [PubMed]
- Huang HW, Chang CC, Wang CS, et al. Association between Inflammation and Function of Cell Adhesion Molecules Influence on Gastrointestinal Cancer Development. Cells 2021;10:67. [Crossref] [PubMed]
- Hussain S, Peng B, Cherian M, et al. The Roles of Stroma-Derived Chemokine in Different Stages of Cancer Metastases. Front Immunol 2020;11:598532 [Crossref] [PubMed]
- Thakur N, Thakur S, Chatterjee S, et al. Nanoparticles as Smart Carriers for Enhanced Cancer Immunotherapy. Front Chem 2020;8:597806 [Crossref] [PubMed]
- Virga F, Quirico L, Cucinelli S, et al. MicroRNA-Mediated Metabolic Shaping of the Tumor Microenvironment. Cancers (Basel) 2021;13:127. [Crossref] [PubMed]
- Reyes-Vargas E, Barker AP, Zhou Z, et al. HLA-DM catalytically enhances peptide dissociation by sensing peptide-MHC class II interactions throughout the peptide-binding cleft. J Biol Chem 2020;295:2959-73. [Crossref] [PubMed]
- Wittig M, Anmarkrud JA, Kassens JC, et al. Development of a high-resolution NGS-based HLA-typing and analysis pipeline. Nucleic Acids Res 2015;43:e70 [Crossref] [PubMed]
- Fratini E, Cervelli M, Mariottini P, et al. Link between spermine oxidase and apoptosis antagonizing transcription factor: A new pathway in neuroblastoma. Int J Oncol 2019;55:1149-56. [Crossref] [PubMed]
- Gobert AP, Al-Greene NT, Singh K, et al. Distinct Immunomodulatory Effects of Spermine Oxidase in Colitis Induced by Epithelial Injury or Infection. Front Immunol 2018;9:1242. [Crossref] [PubMed]
- Sierra JC, Piazuelo MB, Luis PB, et al. Spermine oxidase mediates Helicobacter pylori-induced gastric inflammation, DNA damage, and carcinogenic signaling. Oncogene 2020;39:4465-74. [Crossref] [PubMed]
- Jain V, Raina S, Gheware AP, et al. Reduction in polyamine catabolism leads to spermine-mediated airway epithelial injury and induces asthma features. Allergy 2018;73:2033-45. [Crossref] [PubMed]
- Liu F, Saul AB, Pichavaram P, et al. Pharmacological Inhibition of Spermine Oxidase Reduces Neurodegeneration and Improves Retinal Function in Diabetic Mice. J Clin Med 2020;9:340. [Crossref] [PubMed]
- Crea F, Paolicchi E, Marquez VE, et al. Polycomb genes and cancer: time for clinical application? Crit Rev Oncol Hematol 2012;83:184-93. [Crossref] [PubMed]
- Tan F, Wahdan-Alaswad R, Yan S, et al. Dihydropyrimidinase-like protein 3 expression is negatively regulated by MYCN and associated with clinical outcome in neuroblastoma. Cancer Sci 2013;104:1586-92. [Crossref] [PubMed]
(English Language Editors: R. Scott and J. Gray)