Nomograms for predicting cancer-specific survival in patients with primary central nervous system lymphoma: a population-based analysis
Introduction
Primary central nervous system lymphoma (PCNSL) is a rare and lethal lymphoma confined to the central nervous system (CNS). It accounts for 4–5% of all primary CNS tumors and 4–6% of all non-Hodgkin’s lymphomas (1-3). The annual incidence of PCNSL is 0.5 per 100,000, and there are approximately 1,500 newly diagnosed cases in the United States each year (3). Unfortunately, the incidence of PCNSL continues to increase gradually (4). PCNSL exhibits an aggressive course and poor prognosis, with a 5-year survival of only 15–30% (5). However, there is still no ideal tumor staging or prognostic model that can satisfactorily predict the prognosis of PCNSL, with most clinicians relying on their clinical experience alone.
PCNSL mainly occurs in people over the age of 60 years (1). However, many patients in this age group also present with a high rate of comorbidities (6,7). With the prolonged survival of patients with PCNSL, the morbidity and mortality related to these other comorbidities also increase (8,9). Cancer-specific deaths reflect the progress of cancer itself and the impact on prognosis, while non-cancer-specific deaths are competing factors that affect the survival of cancer patients. Therefore, it is essential to consider such competing risks when evaluating the prognosis of patients with PCNSL. The ability to evaluate censored information enables competitive risk analysis to better predict prognosis (10).
In this study, big data from the Surveillance, Epidemiology, and End Results (SEER) database, in conjunction with data from our hospital, were analyzed to determine the prognostic risk factors and develop nomograms to predict overall survival (OS) and cancer-specific survival (CSS) in patients with PCNSL. We present the following article in accordance with the TRIPOD reporting checklist (available at https://dx.doi.org/10.21037/atm-21-753).
Methods
Patient data collection
PCNSL patients were identified from the SEER database according to the International Classification of Diseases for Oncology Third Edition (ICD-O-3) histology codes (9590–9599, 9670–9699, 9700–9719, 9720–9729) and anatomic location (C71.0–C71.9) (Figure S1). The analysis of PCNSLs was restricted to primary cancers, and secondary CNS lymphomas were excluded. Patients diagnosed without histological confirmation or diagnosed by autopsy were excluded. Patients with unknown survival information were also excluded. Human immunodeficiency virus (HIV) infection status and coexistence of other malignancies were included, which allowed a real-world study to be conducted using a large cohort. The same inclusion and exclusion criteria were applied to patients from the Beijing Tiantan Hospital. This study was conducted following the Declaration of Helsinki (as revised in 2013) and was approved by the Ethics Committee of the Beijing Tiantan Hospital (No.: YW2019-016-11). Individual patient consent was waived.
Definition of variables
The patient demographics obtained included age at diagnosis (0–59, 60–74, ≥75 years), gender (male or female), race (white, black, other/unknown), marital status (married, divorced, single, widowed, other/unknown), coexisting malignancies (no, yes), and HIV infection (negative, positive). Tumor variables (tumor site and histological subtype), treatment variables (surgery, radiotherapy, and chemotherapy), and survival information were also collated. The tumor site was divided into a supratentorial, infratentorial, overlapping lesion of the brain, and not-otherwise-specified (NOS) regions of the brain. Tumor histological subtypes were grouped as diffuse large B cell lymphoma (DLBCL), non-diffuse large B-cell lymphoma (non-DLBCL), or not-otherwise-specified lymphoma (lymphoma, NOS). According to the surgery codes for the brain in the SEER database, the surgical treatments were categorized as biopsy, subtotal resection (STR), gross total resection (GTR), partial lobectomy, lobectomy, and surgery of unknown type. Patients who died of HIV infection or Kaposi sarcoma were considered positive for HIV infection. OS was defined as the time from diagnosis to last follow-up or death from any cause. CSS was defined as the time from the date of diagnosis to death due to PCNSL.
Statistical analysis
The cumulative incidence function (CIF) was used to describe the probability of cancer-specific death and competing death (11). The Gray’s test was used to compare differences in CIF between groups (12). OS was analyzed using the Kaplan-Meier method and compared by log-rank tests. Univariate analysis was conducted to obtain factors significantly related to OS, which were then analyzed by multivariate analyses. The Fine and Gray model was utilized to determine factors significantly related to CSS (13,14). The backward method was then used to select the independent prognostic factors used to establish the nomograms (15). Harrell’s concordance indexes (C-indexes), receiver operating characteristic curve (ROC curves), and calibration curves were used to measure the performance of the nomograms. All statistical analyses were performed using R version 3.6.3 software. Two-tailed distribution with a P value <0.05 was considered statistically significant.
Results
Patient characteristics
From 6,042 patients with intracranial PCNSL who were identified from the SEER database between 1975 and 2016, a total of 5,900 patients were enrolled in this study. The patients were allocated into the training set (n=4,131) and the validation set (n=1,769) at a ratio of 7:3. A consecutive cohort of 163 patients with PCNSL admitted to the Beijing Tiantan Hospital between 2004 and 2018 was included as an external validation set. Baseline patient characteristics are shown in Table 1. All clinicopathological characteristics were comparable between patients in the training set and patients in the validation set (Table S1). Overall, 57.4% of the patients were male, and Caucasians accounted for 79.7% of the total study population. Most of the patients (54.1%) were aged 60 years and older, 45.9% were younger than 59 years. More than half of the patients were married, 25.4% were single, 10.1% were widowed, 7.7% were divorced, and 4.5% were in other relationships, for example, separated or domestic partnership. More than half of the tumors were supratentorial, and DLBCL (n=4,247, 72.0%) was the most common subtype. A total of 4,394 (74.5%) patients received biopsies, 17.0% received surgical resection, and 8.5% received surgery of unknown type, most of which could be biopsied. More than half (54.1%) of the patients received chemotherapy, while less than half (45.1%) received radiotherapy. The vast majority of patients had only one primary malignant tumor (96.4%) and were HIV negative (84.6%).
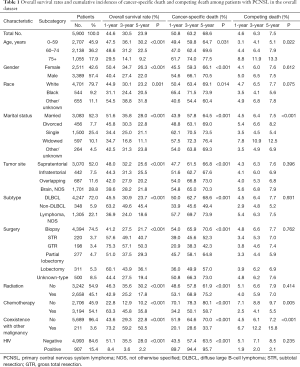
Full table
In total, 4,589 deaths occurred during the follow-up period of 27.7 months (range, 0–469 months), of which 4,030 were cancer-specific deaths, and 559 were competing deaths. The corresponding CIF curves are shown in Figure S2. The 1-, 3-, and 5-year cancer-specific mortalities were 50.8%, 63.2%, and 68.6%, respectively, while the competing mortalities were 4.6%, 6.3%, and 7.5%, respectively. The cumulative probability of death from PCNSL increased with increasing age at diagnosis. The cumulative incidences of competing death also increased with increasing age. The following risk factors significantly increased the probability of PCNSL-specific death: males, black people, single or widowed, overlapping tumor site, DLBCL, biopsy, no radiation, no chemotherapy, HIV positive, and only one primary malignant tumor. Patients with divorced or widowed families who did not undergo chemotherapy and presented with coexisting malignancies were more likely to die of competing causes (Table 1).
Kaplan-Meier analysis and correlation analysis
Kaplan-Meier analysis showed significant differences in OS rates when stratified by these characteristics above (Figure 1). Patients with the following characteristics showed significantly better OS: younger age, female, other race, married, supratentorial site, non-DLBCL, GTR, no radiation, chemotherapy, coexisting malignancies, and HIV negative. The risk coefficients of patients were scored by the Cox regression analysis, and patients were divided into a high-risk group and a low-risk group according to the median value. The survival difference between the high- and low-risk groups was statistically significant (Figure 1). The correlation between these different risk factors is shown in Figure S3. With the increase in time, patients diagnosed with PCNSL were older at the time of diagnosis. The number of patients receiving surgery and radiotherapy decreased, and the number of patients receiving chemotherapy increased. More unmarried younger men were HIV-positive and had shorter survival times.
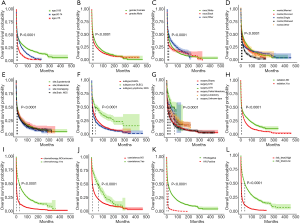
Univariate and multivariate analysis of factors for OS and CSS
As shown in Table 2, univariate analysis for OS revealed that age, gender, race, marital status, tumor site, subtype, surgery, radiation, chemotherapy, coexisting malignancies, and HIV infection status were all significantly associated with survival. The univariate competing risk analysis also showed that all the characteristics were significantly associated with CSS. These risk factors were further incorporated into the multivariate analysis, which demonstrated that the independent prognostic factors of OS were age, gender, marital status, tumor site, subtype, surgery, radiation, chemotherapy, coexisting malignancies, and HIV infection status. In contrast to OS, the independent prognostic factors of CSS were age, tumor site, subtype, surgery, chemotherapy, coexisting malignancies, and HIV infection status (Table 2).
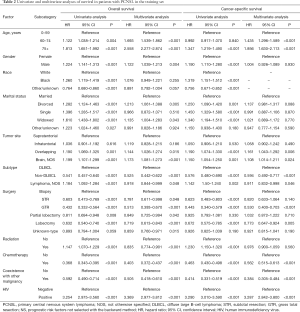
Full table
Construction and internal validation of nomograms for OS and CSS
The backward method was used to select the independent prognostic factors of the final models to construct the nomograms. The forest plots showing the prognostic factors’ hazard ratios (HRs) are shown in Figure S4. The nomogram for predicting OS was established with a C-index of 0.728 [95% confidence interval (CI): 0.703–0.753], and the nomogram for predicting CSS was established with a C-index of 0.726 (95% CI: 0.696–0.756) in the training cohort (Figure 2). The red points in the nomograms represent the scores and the 1-, 3-, and 5-year survival probability of the same patients in the OS and CSS nomogram. To facilitate individualized survival prediction, formulae for survival probability calculations were established (Table S2). Free software is available online (http://www.pcnsl-survivalprediction.cn), the friendly interface of which is shown in Figure S5.
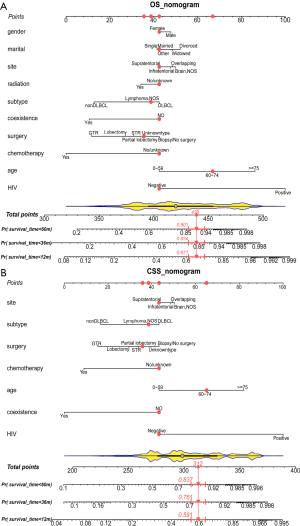
During the validation of the nomograms, the risk scores of each patient in the validation cohort were firstly calculated according to the established Cox regression model by which the nomograms were constructed. Cox regression was then performed using the risk score as a factor. The ROC curves and calibration curves were derived based on the regression analysis. The calibration curves for OS prediction showed high consistency between the predicted survival and actual survival in both the training and internal validation sets. The calibration curves for CSS prediction also confirmed ideal consistency in the training set and internal validation set (Figure 3). The ROC curves of the nomograms for the 1-, 3-, and 5-year OS prediction and CSS prediction are shown in Figure 4. For the training set, the area under ROC curve (AUC) values for predicting the 1-, 3-, and 5-year OS rates were 0.799, 0.791, and 0.778, respectively, and the AUC values for predicting the 1-, 3-, and 5-year CSS rate were 0.801, 0.792, and 0.781, respectively. Similar results were obtained for the internal validation set and external validation set (Figure 4).
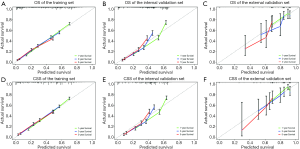
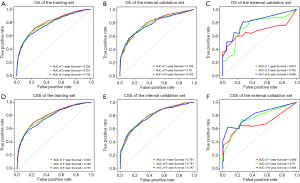
External validation of nomograms
The external validation using the Asian patient cohort from our hospital showed that the established OS and CSS nomograms demonstrated good consistency between predicted survival and actual survival (Figure 3). The AUC values for predicting the 1-, 3-, and 5-year OS rate were 0.674, 0.742, and 0.805, respectively, and the values for predicting the 1-, 3-, and 5-year CSS rate were 0.689, 0.760, and 0.806, respectively (Figure 4). The deviation of the calibration curves and the irregular ROC curves in the external validation may be due to several reasons, including the relatively small sample size, the patients were all HIV-negative, most of the pathological subtypes were DLBCL, and there were no patients with partial lobectomy or lobectomy (Table S3).
Comparison of the OS nomogram
Comparing the discriminatory capacity between the present OS nomogram and the published Deng nomogram revealed that the former performed better than the latter (Figure 5). The AUC values of Deng’s nomogram for predicting the 1-, 3-, and 5-year OS rates were 0.762, 0.758, and 0.744, respectively.
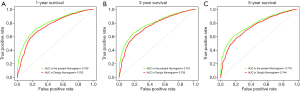
Subgroup analysis
To further explore the stability and validity of the nomograms, a subgroup analysis was conducted. Univariate and multivariate Cox analyses were performed in a cohort of patients with the only PCNSL and HIV negative. The prognostic factors of OS and CSS were the same as those in the training set. However, the C-index of the OS and CSS prognostic model constructed by the subgroup analysis were 0.697 and 0.685, respectively, which were inferior to those of the present nomograms (Figure 6). Other subgroup analyses of HIV infection (negative/positive) or coexistence of other malignancies (yes/no) yielded similar results (Figure S6).
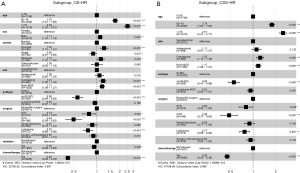
Discussion
PCNSL is an aggressive brain tumor that has been evaluated in previous studies (1,16). However, such studies were either single-center studies with small sample sizes or failed to consider competing risks, resulting in misleading conclusions. Commonly used prognostic models for PCNSL, such as the International Prognostic Index (IPI), the International Extra-nodal Lymphoma Study Group prognostic score scale (IELSG score), and the New York Memorial Sloan Kettering Cancer Center score scale (MSKCC score), cannot satisfactorily determine the prognosis of PCNSL patients (17). An ideal prediction model should include patient characteristics and tumor-related characteristics, and treatment-related characteristics (18). This current study considered all these factors in establishing well-calibrated prognostic nomograms to predict OS and CSS in patients with PCNSL. In addition, these prognostic models were based on the largest cohort of PCNSL patients, and the superior discriminative power of the nomograms was confirmed by the high C-index and AUC values, which were superior to that of other published OS nomogram of PCNSL (16). The present nomograms are the first prognostic nomograms for PCNSL that have been externally validated using a PCNSL database and in the Chinese population to the best of our knowledge.
The present nomograms developed in this report were superior to the models constructed in the subgroup analyses, as demonstrated by the better C-index and AUC values. However, when the present nomogram was applied to the Chinese cohort, its predictive performance was relatively reduced. This may be due to the heterogeneity of different data sets. In the OS nomogram, predictors of long-term survival for patients with PCNSL were younger age, female gender, married status, supratentorial, non-DLBCL, GTR, radiation, chemotherapy, coexisting malignancies, and HIV negative status. In the CSS nomogram, predictors of long-term survival for patients with PCNSL included younger age, supratentorial, non-DLBCL, GTR, chemotherapy, coexisting malignancies, and HIV negative status. Previous reports have suggested that age has a significant impact on survival (19). The present study suggested that age-related non-cancer-specific death was a competing risk, and the most common competing risks for PCNSL were cardiac diseases, cerebrovascular diseases, pneumonia and influenza, HIV, and accidents and adverse events. Therefore, it is important to evaluate treatment tolerance, such as surgery and radiotherapy, among older patients and to consider age when prognostic models are developed for patients with PCNSL.
Some studies have reported that the marital status of patients with PCNSL was significantly associated with survival and suggested that married status was a good prognosis (20). However, the present study demonstrated that marital status was only related to OS. When competing risks were included in the analysis, there was no significant correlation between marital status and prognosis. In patients with PCNSL presenting with comorbidities, the care and support from the spouse may improve their ability to fight multiple diseases. In the competing risks analysis, gender and radiotherapy were also not significantly related to prognosis. Radiotherapy only prolonged the progression-free survival (PFS) time, but not the OS time due to the delayed brain damage (21). In the current study, PCNSL patients benefited most from chemotherapy, but radiotherapy had little effect. Tumors at the supratentorial site and non-DLBCL indicated a better prognosis, suggesting that DLBCL was the most malignant pathological subtype.
Surgical treatment has always been a controversial topic for PCNSL. Single-center clinical studies conducted before the 20th century with few cases suggested that PCNSL patients did not benefit from surgery (22). In the latest 2020 National Comprehensive Cancer Network (NCCN) Central Nervous System Cancer Guidelines, a biopsy is regarded as the “gold standard” for PCNSL, and surgical excision is recommended only in cases of rapid neurological deterioration resulting from an acute increase in intracranial pressure or when the cerebral hernia is imminent. However, the present study revealed that, compared with biopsies, patients with PCNSL could benefit from surgery, and the benefits of patients increased gradually as the scope of surgical resection expanded. Furthermore, patients receiving GTR benefited the most. However, the advantages from partial lobectomy and lobectomy were less obvious than that observed with STR, which may be due to the increased incidence of postoperative complications after major surgery, affecting a patient’s prognosis. In a large randomized controlled trial in Germany in 2012, the PFS and OS of 526 PCNSL patients in the biopsy group were significantly shorter than in the STR and GTR groups, even after the patient’s age and performance status (KPS score) were adjusted (23). Moreover, in a previous study, we found that PCNSL patients benefited the most from GTR and chemotherapy treatment regimen, even after the patient’s age, gender, and pathological subtype were adjusted (24). Therefore, it is imperative to carry out prospective studies to determine whether patients with PCNSL can benefit from surgery.
In this study, HIV was considered a prognostic factor. The results showed that the risk of death in HIV-positive patients was 3.63 times greater than that of HIV-negative patients, indicating that HIV-positive PCNSL patients had unique biological characteristics. Continuous advancements in treatment methods have continued to extend the patient’s survival period. Nowadays, adequate antiretroviral therapy has significantly reduced the prevalence of HIV-associated PCNSL, and as shown in Figure S3, the number of patients with HIV-positive PCNSL has decreased over time. Since the year of diagnosis does not have much significance in predicting the survival of patients, this was excluded from the nomogram as reported (16).
Additionally, it was interesting to note that the probability of long-term survival in patients with coexisting malignancies was higher than that in patients with PCNSL alone. This protective effect was still observed in the subgroup analyses (Figure S6). It is possible that treatment for other malignant tumors, such as immunotherapy and autologous stem cell transplantation, also exerted an inhibitory effect on PCNSL. Subgroup analysis of PCNSL patients with coexisting malignancies showed that age ≥75 years, radiation, and HIV positive status were all risk factors for prognostic (Figure S6). Further research into this complex situation is warranted in the future.
In recent years, competing risk nomograms have been developed for many tumors, such as lung cancer (11), prostate cancer (25), and gallbladder carcinoma (26). However, there has been no specific competing risk analysis for PCNSL. Therefore, the present study was the first to evaluate prognostic factors based on a competing risk analysis model for patients with PCNSL. The published Deng’s nomogram only considered OS. When Deng’s nomogram was applied to our data, the accuracy of the predictions was significantly lower than the present nomogram. Nowadays, increasingly, patients with PCNSL participate in clinical trials of new therapies, such as immune checkpoint inhibitor PD-1 monoclonal antibody treatment. However, clinical trials have strict inclusion criteria and are only suitable for a small number of patients. Most patients still receive traditional radiotherapy and chemotherapy, and thus, the present nomogram has significant clinical value. The inclusion of competing risks in the analysis makes assessing the long-term survival of patients with PCNSL more accurate. Future research should investigate the association of OS and CSS with primary tumors and comorbidities. Since the SEER database does not provide detailed information on comorbidities, we cannot clarify which comorbidity can significantly affect the survival of patients with PCNSL.
There were several limitations in this study. First, some potential prognostic factors, such as KPS score, serum lactic dehydrogenase (LDH) levels, and lesion size, were not available in the SEER database and were therefore not included in the nomograms in this study. Second, as a retrospective study, treatment selection bias could not be avoided. For example, patients with a single supratentorial site, low surgical risk, and tumors misdiagnosed as gliomas were more likely to receive surgery.
Conclusions
In conclusion, this study reports the development of novel prognostic nomograms based on a large population cohort to estimate the OS and CSS for patients with PCNSL. The well-calibrated nomograms may promote patient management and patient counseling in clinical practice, better predict individualized survival, and promote the stratification of patients in subsequent clinical trials in the future. To facilitate the clinical use of this nomogram, free software for individualized survival prediction is provided (http://www.pcnsl-survivalprediction.cn).
Acknowledgments
We would like to thank J. Teoh and J. Chapnick, editors of AME Editing Service, for their medical writing service.
Funding: None.
Footnote
Reporting Checklist: The authors have completed the TRIPOD reporting checklist. Available at https://dx.doi.org/10.21037/atm-21-753
Peer Review File: Available at https://dx.doi.org/10.21037/atm-21-753
Conflicts of Interest: All authors have completed the ICMJE uniform disclosure form (available at https://dx.doi.org/10.21037/atm-21-753). The authors have no conflicts of interest to declare.
Ethical Statement: The authors are accountable for all aspects of the work in ensuring that questions related to the accuracy or integrity of any part of the work are appropriately investigated and resolved. This study was conducted following the Declaration of Helsinki (as revised in 2013) and was approved by the Ethics Committee of the Beijing Tiantan Hospital (No.: YW2019-016-11). Individual patient consent was waived.
Open Access Statement: This is an Open Access article distributed in accordance with the Creative Commons Attribution-NonCommercial-NoDerivs 4.0 International License (CC BY-NC-ND 4.0), which permits the non-commercial replication and distribution of the article with the strict proviso that no changes or edits are made and the original work is properly cited (including links to both the formal publication through the relevant DOI and the license). See: https://creativecommons.org/licenses/by-nc-nd/4.0/.
References
- Rae AI, Mehta A, Cloney M, et al. Craniotomy and Survival for Primary Central Nervous System Lymphoma. Neurosurgery 2019;84:935-44. [Crossref] [PubMed]
- Villano J L, Koshy M, Shaikh H, et al. Age, gender, and racial differences in incidence and survival in primary CNS lymphoma. Br J Cancer 2011;105:1414-8. [Crossref] [PubMed]
- Grommes C, DeAngelis LM. Primary CNS Lymphoma. J Clin Oncol 2017;35:2410-8. [Crossref] [PubMed]
- Mahale P, Herr MM, Engels EA, et al. Autoimmune conditions and primary central nervous system lymphoma risk among older adults. Br J Haematol 2020;188:516-21. [Crossref] [PubMed]
- Kasenda B, Ferreri A J M, Marturano E, et al. First-line treatment and outcome of elderly patients with primary central nervous system lymphoma (PCNSL)--a systematic review and individual patient data meta-analysis. Ann Oncol 2015;26:1305-13. [Crossref] [PubMed]
- Han KR, Kim HL, Pantuck AJ, et al. Use of American Society of Anesthesiologists physical status classification to assess perioperative risk in patients undergoing radical nephrectomy for renal cell carcinoma. Urology 2004;63:841-6. [Crossref] [PubMed]
- Janssen-Heijnen ML, Houterman S, Lemmens VE, et al. Prognostic impact of increasing age and co-morbidity in cancer patients: a population-based approach. Crit Rev Oncol Hematol 2005;55:231-40. [Crossref] [PubMed]
- Zhang W, Hong HJ, Chen YL. Establishment of a gallbladder cancer-specific survival model to predict prognosis in non-metastatic gallbladder cancer patients after surgical resection. Dig Dis Sci 2018;63:2251-8. [Crossref] [PubMed]
- Eguchi T, Bains S, Lee MC, et al. Impact of increasing age on cause-specific mortality and morbidity in patients with stage I non-small-cell lung cancer: a competing risks analysis. J Clin Oncol 2017;35:281-90. [Crossref] [PubMed]
- Putter H, Fiocco M, Geskus RB. Tutorial in biostatistics: competing risks and multi-state models. Stat Med 2007;26:2389-430. [Crossref] [PubMed]
- Zhang MJ, Zhang X, Scheike TH. Modeling cumulative incidence function for competing risks data. Expert Rev Clin Pharmacol 2008;1:391-400. [Crossref] [PubMed]
- Gray RJ. A class of K-sample tests for comparing the cumulative incidence of a competing risk. Ann Stat 1988;16:1141-54. [Crossref]
- Kuk D, Varadhan R. Model selection in competing risks regression. Stat Med 2013;32:3077-88. [Crossref] [PubMed]
- Fine JP, Gray RJ. A proportional hazards model for the subdistribution of a competing risk. J Am Stat Assoc 1999;94:496-509. [Crossref]
- Mogensen UB, Ishwaran H, Gerds TA. Evaluating random forests for survival analysis using prediction error curves. J Stat Softw 2012;50:1-23. [Crossref] [PubMed]
- Deng X, Xu X, Lin D, et al. Real-World Impact of Surgical Excision on Overall Survival in Primary Central Nervous System Lymphoma. Front Oncol 2020;10:131. [Crossref] [PubMed]
- Liu CJ, Lin SY, Yang CF, et al. A new prognostic score for disease progression and mortality in patients with newly diagnosed primary CNS lymphoma. Cancer Med 2020;9:2134-45. [Crossref] [PubMed]
- Montero PH, Yu C, Palmer FL, et al. Nomograms for preoperative prediction of prognosis in patients with oral cavity squamous cell carcinoma. Cancer 2014;120:214-21. [Crossref] [PubMed]
- He C, Zhang Y, Cai Z, et al. Nomogram to predict cancer-specific survival in patients with pancreatic acinar cell carcinoma: a competing risk analysis. J Cancer 2018;9:4117-27. [Crossref] [PubMed]
- Norden AD, Drappatz J, Wen PY, et al. Survival among patients with primary central nervous system lymphoma, 1973-2004. J Neurooncol 2011;101:487-93. [Crossref] [PubMed]
- Schlegel U, Korfel A. Is whole-brain radiotherapy still a standard treatment for primary central nervous system lymphomas? Curr Opin Neurol 2018;31:733-9. [Crossref] [PubMed]
- Hayakawa T, Takakura K, Abe H, et al. Primary central nervous system lymphoma in Japan--a retrospective, co-operative study by CNS-Lymphoma Study Group in Japan. J Neurooncol 1994;19:197-215. [Crossref] [PubMed]
- Kreher S, Strehlow F, Martus P, et al. Prognostic impact of intraocular involvement in primary CNS lymphoma: experience from the G-PCNSL-SG1 trial. Ann Hematol 2015;94:409-414. [Crossref] [PubMed]
- Yang CW, Ren XH, Jiang HH, et al. Different treatment regimens for primary central nervous system lymphoma:based on SEER database. Zhonghua Wai Ke Za Zhi 2021;59:52-8. [PubMed]
- Castro E, Goh C, Olmos D, et al. Germline BRCA mutations are associated with higher risk of nodal involvement, distant metastasis, and poor survival outcomes in prostate cancer. J Clin Oncol 2013;31:1748-57. [Crossref] [PubMed]
- He C, Cai Z, Zhang Y, et al. Prognostic Model to Predict Cancer-Specific Survival for Patients With Gallbladder Carcinoma After Surgery: A Population-Based Analysis. Front Oncol 2019;9:1329. [Crossref] [PubMed]
(English Language Editors: J. Teoh and J. Chapnick)