Identification of JAK2 and FOXM1 expression as novel candidate biomarkers for predicting the benefit of immunotherapy in lung squamous cell carcinoma
Introduction
Non-small cell lung cancer (NSCLC) is currently the leading cause of cancer-related deaths worldwide and lung squamous cell carcinoma (LUSC) is among the most common histological subtypes of NSCLC (1-3). Unlike lung adenocarcinoma (LUAD) with oncogenic driver variations, treatment for LUSC is limited and conventional chemotherapy has endured as the standard therapy (4-6). Campbell et al. found that NFE2L2, KDM6A, RASA1, NOTCH1 and HRAS were significantly mutated only in LUSC,including over 47% of LUAD and 53% of LUSC samples had more than five predicted neoepitopes, which indicated that immunotherapy strategy had great potential, the change of neoepitopes antigen includes the site p.Glu79Gln of NFE2L2 (7).
Recently, immune checkpoint inhibitors (ICIs) targeting programmed cell death 1 (PD-1), its ligand (PD-L1), and CTLA-4 have revolutionized the treatment of both LUAD and LUSC. Some studies found express of PD-L1 was more frequent in central type, tumor location that could predict expression status of PD-L1, and could potentially serve as clinical response to immunotherapy. To date, several anti-PD-1/PD-L1 or CTLA-4 antibodies including nivolumab, pembrolizumab, atezolizumab, and ipilimumab combination with or without chemotherapy have been approved as first-line treatment for patients with advanced LUSC. However, only a small subset of patients with NSCLC (20–25%) can benefit from ICIs (8). Increasing evidence indicates the prognostic value of immune-related signatures in the tumor microenvironment. Qu et al. found that genes related to prognosis in LUAD include: ICAM, MS4A1 and IL-16. LUAD had more memory B cells and CD8+T cells, while M0 macrophages were less than to early N stage. Meanwhile, for LUSC, only GSTA1 and HAS1 genes are associated with prognostic factors related to immune-related. It was found that the high invasiveness of naive CD4+ T cells was related to the more advanced T stage, while the CD8+T cells and M1 macrophages were higher in the infiltration advanced N stage in LUSC (9). Thus, the identification of predictive biomarkers of response to ICIs is emergent in LUSC. Emerging evidence has suggested that positive PD-L1 expression and high tumor mutation burden (TMB) could predict the response to ICIs in NSCLC (10,11); Tumor can evade immune surveillance by inhibiting the activation of immune cells with up-regulation of PD-L1. Compared with traditional therapies that directly target cancer cells, that anti-PD-1/PD-L1 antibodies reactivate the patient’s immune system to eradicate tumors, to improve patients anti-tumor immunity with different tumor types, including lung cancer Anti-tumor immunity (12). Some studies showed that when atezolizumab is as single-agent for PD-L1 high expression patients, the ORR, PFS and overall survival (OS) in treatment was significantly better than that of PD-L1 negative patients (13). However, these biomarkers still have some limitations. Firstly, not all patients with positive expression of PD-L1 benefit from immunotherapy. Secondly, the immunotherapy can occasionally result in hyperprogressive disease. Thirdly, the use of PD-L1 expression is controversial because of its varied definition, cut-off value, and spatial as well as temporal heterogeneity (14). Finally, TMB alone cannot fully predict response to ICIs; the combination of TMB with other biomarkers may improve the predictive ability. Thus, it is an urgent need to find novel effective biomarkers for precise immunotherapy.
With the progress of next-generation sequencing (NGS) technology, a growing number of high-throughput sequencing data on cancer have been published in public databases of the Gene Expression Omnibus (GEO), providing a novel strategy to solve the abovementioned dilemma (15).
Previous studies have confirmed the use of gene mutational signature as biomarkers for predicting the therapeutic efficacy of immunotherapy. However, the efficacy of immunotherapy in LUSC cannot yet be predicted completely and accurately. Due to the complexity of the immune response and immunotherapy, we hypothesized that in addition to genomic studies, transcriptomic and proteomic investigation might also be essential for accurately predicting the clinical benefit.
Janus kinases (JAKs) are a family of non-receptor tyrosine kinases, which are related to autoimmune diseases and malignant tumors. As a member of the Janus kinase family, JAK2 is an important part of the signal transducer and activator of transcription (JAK-STAT) signaling pathway, and plays an important role in promoting tumor phenotypes such as tumorigenesis, invasion, metastasis, proliferation, survival, angiogenesis, anti-apoptosis, and immune evasion (16-18).
Forkhead box transcription factor M1 (FOXM1) is a well-known proliferation-associated transcription factor which belongs to the forkhead box (FOX) transcription factor family, and is characterized by a conserved DNA-binding domain referred to as the FOX. It is involved in a variety of biological functions, including cell proliferation, cell cycle regulation, angiogenesis, cell migration, tumor invasion, aging, DNA damage repair, stem cell expansion, renewal, and immunoregulation (19). The loss of Cyclin-dependent kinase (CDK) family function can cause the expression of DNA damage repair genes to be silenced, which may increase the sensitivity of tumor cells to platinum-based chemotherapy (20).
In this study, we explored the differences in gene expression profiles between PD-L1 high-expression/TMB-high (TPH) and PD-L1 low-expression/TMB-low (TPL) groups of patients, and identified JAK2 and FOXM1 as novel candidate biomarkers to predict the clinical benefit of ICIs in LUSC. The results of this investigation are of great clinical significance for personalized immunotherapy in LUSC.
We present the following article in accordance with the REMARK reporting checklist (available at https://dx.doi.org/10.21037/atm-21-2186).
Methods
Participants
The study was conducted in accordance with the Declaration of Helsinki (as revised in 2013). Messenger RNA (mRNA) expression profiles and relative clinical information of patients with LUSC were downloaded from The Cancer Genome Atlas (TCGA) data portal. We processed and normalized TCGA level 3 RNA-seq data, and the fragments per kilobase of exon model per million mapped fragments (FPKM) values were used for the gene expression levels. Reverse phase protein array (RPPA) data were also downloaded at the gene level. In total 16,383 expressed genes used from 502 LUSC samples and 237 expressed proteins across 325 LUSC samples.
The clinical follow-up information and gene expression profiles of NSCLC tissue in GSE126044 (21) and GSE136961 (22) were downloaded from the GEO (http://www.ncbi.nlm.nih.gov/geo/) database (23). The microarray data of GSE126044 and GSE136961 were based on GPL16791 and GPL24014 platforms, respectively, and the former included 16 patients (Submission date: Feb 04, 2019), while the latter included 21 patients (Submission date: Sep 05, 2019) with NSCLC receiving anti-PD-1 treatment.
Identification of DEGs
A total of 317 patients with LUSC were divided into 2 groups based on PD-L1 expression and TMB status: the top 25% of patients with high TMB and high level of PD-L1 expression were defined as the TPH group (23 participants), and the bottom 25% of patients with low TMB and low level of PD-L1 expression were defined as the TPL group (21 participants). The differences in clinicopathological characteristics between TPH and TPL groups were compared. The ‘limma’ package (http://bioinf.wehi.edu.au/limma) was used to identify the differentially expressed genes (DEGs) in the TPH and TPL groups and P<0.05 and |logFC|>1 were used as the cut-off criterions to select DEGs for mRNA and protein expressions. Finally, the overlapping DEGs between the 2 omics were detected (Figure 1).
Immune infiltration in LUSC
The relative percent of 22 immune cells in each LUSC sample was calculated using the CIBERSORT algorithm, which included gene expression of 22 leukocyte subtypes (24). At the same time, the gene expression profile data were used to quantify the infiltration of immune cells in tumor tissues by single-sample gene set enrichment analysis (ssGSEA) (25), and the enrichment score of 29 immune cells was obtained. Then, the infiltration level of 22 immune cells and the enrichment score of 29 immune cells were compared between TPH group and TPL group by Wilcoxon ranked-sum test.
Gene Ontology (GO) and Kyoto Encyclopedia of Genes and Genomes (KEGG) pathway enrichment analysis
GO terms analysis of JAK2 and FOXM1 was performed using the R package ‘clusterProfiler’ (https://rdocumentation.org/packages/clusterProfiler/). The bar-chart of GO results for cellular component (CC), biological process (BP), and molecular function (MF) were ranked by P value and exhibited. The KEGG pathways of DEGs at the significant level (P<0.05) were employed.
Protein-protein interaction (PPI) network construction and analysis
PPI networks were integrated from the Search Tool for the Retrieval of Interacting Genes (STRING) database (https://string-db.org/cgi/input.pl; version: 11.0) (26). Cytoscape software (version: 3.6.1; https://cytoscape.org) (27) was used to establish and visualize the interaction network of DEGs.
Risk score construction and survival analysis
In order to determine an effective prognostic feature, we derived the risk score for survival analysis with JAK2 and FOXM1 gene- and protein-expressions, which was accessed on the basis of the following equation, where .m is the gene expression and .p is the protein expression. The median risk score of participants was used as cut-off value; afterwards, samples were divided into high and low-risk groups. Kaplan-Meier survival analysis was performed to compare the prognosis between 2 groups.
Validation for the performance of risk score
The performance of the risk score was verified through 2 external datasets, which included 16 and 21 patients with NSCLC receiving immune checkpoint blockade (ICB), respectively. Receiver operating characteristic (ROC) curves were applied to assess the sensitivity and specificity of ICB-response based on the risk score, and the R package ‘pROC’ (28) was used to quantify the area under the curve (AUC). The association between clinical outcome and the level of JAK2 or FOXM1 expression was evaluated through Wilcoxon ranked-sum test and shown by boxplot.
Statistical analysis
The ‘limma’ package (http://bioinf.wehi.edu.au/limma) was used to identify the differentially expressed genes (DEGs) in the TPH and TPL groups with P<0.05 and |logFC| >1. The enrichment analysis was performed using the R package ‘clusterProfiler’. PPI networks were visualized by Cytoscape software. Univariate and multivariate Cox regression analyses were used to evaluate the association of variables with OS in the ‘survival’ R package, as well as the log-rank test, was applied to compare the prognostic value. In this study, we used area under the curve (AUC) as the performance measurement method for predictive models, which was plotted using the ‘survivalROC’ R package, and all statistical tests were performed using R-3.6.3.
Results
Different immune infiltration and survival analysis between TPH and TPL
The clinical information of TPH and TPL LUSC patients is listed in Table 1. To investigate the association between PD-L1 expression, TMB, and immune infiltration in LUSC, we firstly calculated the percentages of 22 leukocyte cells of TPH and TPL participants using the CIBERSORT algorithm and then compared immune cell fractions. We found that the TPH group had more abundant macrophages M1 (P<0.01), resting natural killer (NK) cells (P<0.05), activated memory CD4 T cells (P<0.001), and CD8 T cells (P<0.05), but fewer memory B cells (P<0.05), activated dendritic cells (P<0.05), and neutrophils (P<0.05). It manifested the patients with high expression of PD-L1 and high TMB harbored more abundant immune cell infiltration, which might predict good adaptive immune response and survival when receiving immunotherapy (Figure 2A).
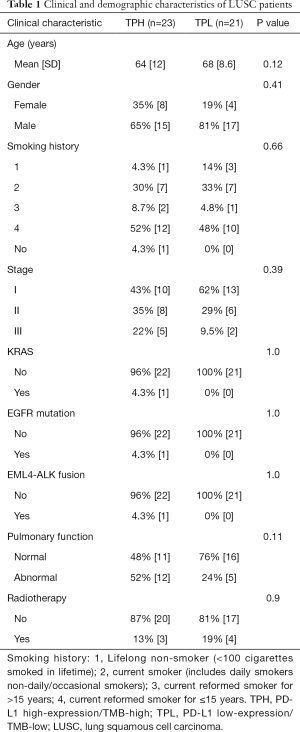
Full table
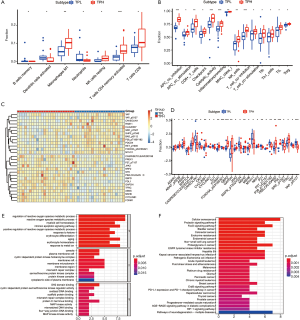
We then quantified the enrichment levels of 29 immune cells, immune-related pathways, and activity of immune-related function in the two groups by ssGSEA score. We discovered that compared with the TPL group, the TPH group harbored more immune cells, immune-related pathways, and activities of immune-related function, which might further predict favorable adaptive immune response and clinical outcome when receiving immunotherapy (Figure 2B). Both median OS (mOS) and median progression-free survival (mPFS) were worse in the TPL group than in the TPH group. The mOS in the TPH group was 61.9 months, while that in the TPL group was 19.6 months. However, TPH and TPL both still have some limitations for prognosis (Figure S1).
DEGs and differentially expressed proteins (DEPs) between the 2 groups
The DEGs and DEPs between the TPH and TPL groups are shown in Figure 2C,D, respectively.
GO and KEGG enrichment analysis of signaling pathways between the 2 groups
Compared with the TPL group, GO analysis indicated that TPH mainly enriched signaling pathways such as regulation of reactive oxygen species (ROS) metabolic process, intrinsic apoptotic signaling pathway, aging, and response to metal ion (BP) (Figure 2E). The KEGG analysis indicated that TPH mainly enriched signaling pathways such as cellular senescence, FoxO signaling, and proteoglycans in cancer (Figure 2F).
Identification of JAK2 and FOXM1 as differentially expressed genes and proteins between the two groups
The ‘Limma’ package with multiple testing correction methods was used to identify DEGs between TPH and TPL groups (Figure 2C,D), respectively. Finally, JAK2 and FOXM1 were detected as the overlapping DEGs between the 2 omics.
Enrichment-pathway and PPI network analysis of JAK2 and FOXM1
The GO and KEGG biological pathway enrichment analyses demonstrated that signaling pathways JAK2 was involved in included ErbB, VEGF, Rap1, TNF, MAPK, JAK-STAT, and the AGE-RAGE signaling pathway in diabetic complications, EGFR tyrosine kinase inhibitor (TKI) resistance, platinum drug resistance, PI3K-AKT, PD-L1 expression and PD-1 checkpoint pathway in cancer, and FoxO and HIF-1 signaling pathway. In addition to some of the same signaling pathways of JAK2 such as JAK-STAT, AGE-RAGE signaling pathway in diabetic complications, EGFR TKI resistance, platinum drug resistance, PI3K-AKT, PD-L1 expression and PD-1 checkpoint pathway in cancer, and FoxO and HIF-1 signaling pathway, FOXM participation also included the p53 and cell cycle signaling pathway (Figure 3A). Based on the STRING database, about 20 proteins were predicted to directly interact with JAK2 or FOXM1 (Figure 3B,C). The JAK2 gene is associated with many genes in the STAT family, such as STAT3 and STAT2. Specifically, STAT3 increases tumor cell proliferation (29), survival and invasion and activates tumor-promoting inflammation, but also suppresses anti-tumor immune responses. Therefore, STAT3 is a promising target for cancer therapy. The FOXM1 gene is related to cell-cycle pathways (Figure 3A), which control cell proliferation, and cancer is a disease of inappropriate cell proliferation (30).
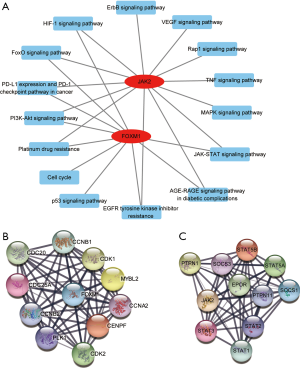
Validation the robustness of risk score as prognostic factors for immunotherapy through internal and external datasets
To verify the robustness of JAK2 and FOXM1 gene expression, we proposed a new risk score by integrating the gene and protein expression of JAK2 and FOXM1 through LUSC patients. Through univariate and multivariate Cox regression analysis it was revealed that the risk score was significantly associated with OS (Table 2). The Kaplan-Meier curve demonstrated the survival rate of the high-risk group was significantly lower than that of the low-risk group. Thus, JAK2 and FOXM1 expression might serve as potential prognostic factors to distinguish the OS of patients with LUSC (Figure 4A,B). The risk score prognosis model achieved AUC =0.709 and showed better prediction of survival than PD-L1 and TMB in regard to overall survival (Figure 4C). The distribution of patient risk scores and survival status were shown in Figure 4D.
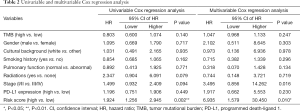
Full table
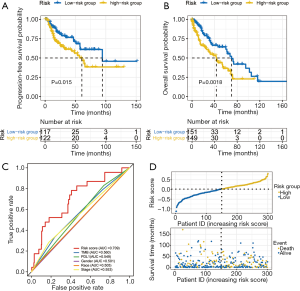
Validation of the association of risk score with immunotherapeutic efficacy through external datasets GSE126044 and GSE136961
We used the external datasets GSE126044 and GSE136961 to validate the robustness of risk score in participants who received immunotherapy. The risk score prognosis model achieved AUCs of 0.727 and 0.67 for predicting the response of NSCLC patients treated with anti-PD-1 and anti-PDL1 antibodies, respectively (Figure 5A). In addition, we used the external dataset GSE136961 to validate the robustness of FOXM1 gene expression in participants who received immunotherapy. As shown in Figure 5B, the level of FOXM1 expression was much higher in participants who had durable clinical benefit than those who had not received such benefits with immunotherapy (P=0.043). In GSE126044, the results revealed that the level of both JAK2 and FOXM1 expression was significantly higher in participants who responded to immunotherapy than in those who did not (P=0.038 and P=0.009, respectively) (Figure 5C,D).
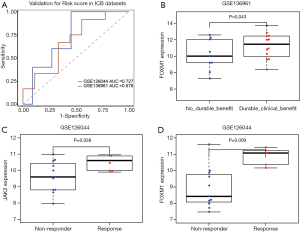
Discussion
LUSC accounts for approximately 45% of primary lung cancers in men and 25% in women, which can be divided into central type and peripheral type. Some studies found that PD-L1 expression was more frequent in central type, tumor location that could predict expression status of PD-L1, and could potentially serve as clinical response to immunotherapy. With the advance of ICIs in LUSC, it is urgent to explore effective biomarkers for LUSC patients. PD-1 can be used as a predictor of the efficacy of multiple cancers treated in tumor tissues by immunotherapy, The proportion of PD-L1 antigen-presenting cells had relationship with Clinical response (31). Although PD-L1 and TMB are the most common predictors for the patients with LUSC receiving immunotherapy, they could not meet patient needs due to their individual limitations. We recommend that a preoperative biopsy to detect driver gene mutations is necessary to guide the decision of neoadjuvant therapy.
Studies have revealed that patients with NSCLC who harbor both positive expression of PD-L1 and high TMB demonstrate significantly favorable objective response rate (ORR) and PFS than those with only 1 of or no such variables (14). Thus, we divided the LUSC patients into two participant groups (TPH and TPL) according to their expression of PD-L1 and TMB status. Through the DEG profiles, we identified JAK2 and FOXM1 as the DEGs between these two groups.
Results of GO and KEGG pathway analyses revealed the signaling pathways in which JAK2 or FOXM1 are involved, which was consistent with the role that JAK2 or FOXM1 played in the development of cancer, such as proliferation [JAK-STAT (32-34) and ErbB/PI3K-AKT signaling pathway (35), PI3K-AKT/FoxO (36)], anti-apoptosis (p53 signaling pathway), angiogenesis (VEGF signaling pathway), and immune response [JAK-STAT (37)]. The JAK2 component is an important part of JAK-STAT signaling pathway. Prior studies reported that JAK-STAT signaling mediates almost all immune regulatory processes (38). It has also been shown that FOXM1 could promote the activation, proliferation, and survival of immune cells (39,40). We discovered that levels of JAK2 and FOXM1 expression were correlated with immune infiltration in LUSC, which indicated that JAK2 and FOXM1 not only played a key role in the tumorigenesis, but were also involved in the regulation of immune response. Thus, we speculated that patients with higher levels of JAK2 or FOXM1 expression might gain durable clinical benefits when receiving treatment with ICIs. Further analysis with external datasets GSE126044 and GSE136961 supported our hypothesis. Some of our findings were consistent with those of previous investigations (41,42). For example, Liu et al. (41) revealed that a higher level of JAK2 expression was correlated with the tumor infiltrating lymphocytes, especially infiltrating T cells, in breast cancer. The difference was that the study by Liu et al. showed that expression of JAK2 was an independent indicator of favorable prognosis in patients with breast cancer. Further investigation is required to clarify that particular finding.
Survival analysis was conducted to validate the potential clinical benefits of assessing the risk score to guide ICI strategies, which demonstrated that patients with high risk scores were associated with worse OS and PFS (P=0.0018 and P=0.015, respectively). Some previous studies explained that JAK2 and FOXM1 were involved in promoting the pathogenesis of malignancy (32-39). In addition, the predictive performance of the risk score model on ICB-response indicated that it may be a potential immune-related biomarker for LUSC. Although the risk score can effectively aid in predicting the prognosis of LUSC patients, there is still a lack of clinical trials. If more comprehensive LUSC immunotherapy data emerges in the future, we believe it will be possible to better verify the performance of the risk score. Overall, this was the first study to identify that JAK2 and FOXM1 expression could independently predict the clinical outcome of LUSC patients receiving ICIs. Wu et al. found tumor profile of advanced NSCLC in May 2021, comprehensively characterize the disease characteristics of patients with advanced non-small cell lung cancer from multiple aspects. The results of innovative drug camrelizumab combined with chemotherapy for the first-line treatment of stage III Chinese LUSC patients, which confirmed the excellent efficacy of camrelizumab in the first-line treatment of lung squamous cell carcinoma (43). With the discovery of new drug targets and the continuous emergence of new combination treatment options, our investigation may provide insight into identifying the correct candidates for precision immunotherapy.
Acknowledgments
We would like to thank to ChosenMed Technology (Beijing) Co. Ltd for providing guidance for this study.
Funding: None.
Footnote
Reporting Checklist: The authors have completed the REMARK reporting checklist. Available at https://dx.doi.org/10.21037/atm-21-2186
Conflicts of Interest: All authors have completed the ICMJE uniform disclosure form (available at https://dx.doi.org/10.21037/atm-21-2186). The authors thank to ChosenMed Technology (Beijing) Co. Ltd for providing guidance for this study. There is no payment support between them. The authors have no other conflicts of interest to declare.
Ethical Statement: The authors are accountable for all aspects of the work in ensuring that questions related to the accuracy or integrity of any part of the work are appropriately investigated and resolved. The study was conducted in accordance with the Declaration of Helsinki (as revised in 2013). The data released by the TCGA database was publicly available and therefore did not require informed patient consent.
Open Access Statement: This is an Open Access article distributed in accordance with the Creative Commons Attribution-NonCommercial-NoDerivs 4.0 International License (CC BY-NC-ND 4.0), which permits the non-commercial replication and distribution of the article with the strict proviso that no changes or edits are made and the original work is properly cited (including links to both the formal publication through the relevant DOI and the license). See: https://creativecommons.org/licenses/by-nc-nd/4.0/.
References
- Perez-Moreno P, Brambilla E, Thomas R, et al. Squamous cell carcinoma of the lung: molecular subtypes and therapeutic opportunities. Clin Cancer Res 2012;18:2443-51. [Crossref] [PubMed]
- Kinoshita T. Ideal prognostic model in lung squamous cell carcinoma. Transl Lung Cancer Res 2020;9:430-1. [Crossref] [PubMed]
- Gandara DR, Hammerman PS, Sos ML, et al. Squamous cell lung cancer: from tumor genomics to cancer therapeutics. Clin Cancer Res 2015;21:2236-43. [Crossref] [PubMed]
- Reck M, Rabe KF. Precision Diagnosis and Treatment for Advanced Non-Small-Cell Lung Cancer. N Engl J Med 2017;377:849-61. [Crossref] [PubMed]
- Herbst RS, Morgensztern D, Boshoff C. The biology and management of non-small cell lung cancer. Nature 2018;553:446-54. [Crossref] [PubMed]
- Langer CJ, Obasaju C, Bunn P, et al. Incremental Innovation and Progress in Advanced Squamous Cell Lung Cancer: Current Status and Future Impact of Treatment. J Thorac Oncol 2016;11:2066-81. [Crossref] [PubMed]
- Campbell JD, Alexandrov A, Kim J, et al. Distinct patterns of somatic genome alterations in lung adenocarcinomas and squamous cell carcinomas. Nat Genet 2016;48:607-16. [Crossref] [PubMed]
- Miura Y, Kasahara N, Sunaga N. Immune checkpoint inhibitors and driver oncogenes in non-small cell lung cancer. Transl Cancer Res 2019;8:S628-32. [Crossref]
- Qu Y, Cheng B, Shao N, et al. Prognostic value of immune-related genes in the tumor microenvironment of lung adenocarcinoma and lung squamous cell carcinoma. Aging (Albany NY) 2020;12:4757-77. [Crossref] [PubMed]
- Mok TSK, Wu YL, Kudaba I, et al. Pembrolizumab versus chemotherapy for previously untreated, PD-L1-expressing, locally advanced or metastatic non-small-cell lung cancer (KEYNOTE-042): a randomised, open-label, controlled, phase 3 trial. Lancet 2019;393:1819-30. [Crossref] [PubMed]
- Tomasini P, Greillier L. Targeted next-generation sequencing to assess tumor mutation burden: ready for prime-time in non-small cell lung cancer? Transl Lung Cancer Res 2019;8:S323-6. [Crossref] [PubMed]
- Sharpe AH, Pauken KE. The diverse functions of the PD1 inhibitory pathway. Nat Rev Immunol 2018;18:153-67. [Crossref] [PubMed]
- Kowanetz M, Zou W, Gettinger SN, et al. Differential regulation of PD-L1 expression by immune and tumor cells in NSCLC and the response to treatment with atezolizumab (anti-PD-L1). Proc Natl Acad Sci U S A 2018;115:E10119-26. [Crossref] [PubMed]
- Otoshi T, Nagano T, Tachihara M, et al. Possible Biomarkers for Cancer Immunotherapy. Cancers (Basel) 2019;11:935. [Crossref] [PubMed]
- Qi X, Qi C, Wu T, et al. CSF1R and HCST: Novel Candidate Biomarkers Predicting the Response to Immunotherapy in Non-Small Cell Lung Cancer. Technol Cancer Res Treat 2020;19:1533033820970663 [Crossref] [PubMed]
- Kleppe M, Kwak M, Koppikar P, et al. JAK-STAT pathway activation in malignant and nonmalignant cells contributes to MPN pathogenesis and therapeutic response. Cancer Discov 2015;5:316-31. [Crossref] [PubMed]
- Schwartz DM, Bonelli M, Gadina M, et al. Type I/II cytokines, JAKs, and new strategies for treating autoimmune diseases. Nat Rev Rheumatol 2016;12:25-36. [Crossref] [PubMed]
- Balko JM, Schwarz LJ, Luo N, et al. Triple-negative breast cancers with amplification of JAK2 at the 9p24 locus demonstrate JAK2-specific dependence. Sci Transl Med 2016;8:334ra53 [Crossref] [PubMed]
- Zhou Z, Chen H, Xie R, et al. Epigenetically modulated FOXM1 suppresses dendritic cell maturation in pancreatic cancer and colon cancer. Mol Oncol 2019;13:873-93. [Crossref] [PubMed]
- Quereda V, Bayle S, Vena F, et al. Therapeutic Targeting of CDK12/CDK13 in Triple-Negative Breast Cancer. Cancer Cell 2019;36:545-558.e7. [Crossref] [PubMed]
- Cho JW, Hong MH, Ha SJ, et al. Genome-wide identification of differentially methylated promoters and enhancers associated with response to anti-PD-1 therapy in non-small cell lung cancer. Exp Mol Med 2020;52:1550-63. [Crossref] [PubMed]
- Hwang S, Kwon AY, Jeong JY, et al. Immune gene signatures for predicting durable clinical benefit of anti-PD-1 immunotherapy in patients with non-small cell lung cancer. Sci Rep 2020;10:643. [Crossref] [PubMed]
- Edgar R, Domrachev M, Lash AE. Gene Expression Omnibus: NCBI gene expression and hybridization array data repository. Nucleic Acids Res 2002;30:207-10. [Crossref] [PubMed]
- Newman AM, Liu CL, Green MR, et al. Robust enumeration of cell subsets from tissue expression profiles. Nat Methods 2015;12:453-7. [Crossref] [PubMed]
- Finotello F, Trajanoski Z. Quantifying tumor-infiltrating immune cells from transcriptomics data. Cancer Immunol Immunother 2018;67:1031-40. [Crossref] [PubMed]
- Szklarczyk D, Morris JH, Cook H, et al. The STRING database in 2017: quality-controlled protein-protein association networks, made broadly accessible. Nucleic Acids Res 2017;45:D362-8. [Crossref] [PubMed]
- Shannon P, Markiel A, Ozier O, et al. Cytoscape: a software environment for integrated models of biomolecular interaction networks. Genome Res 2003;13:2498-504. [Crossref] [PubMed]
- Robin X, Turck N, Hainard A, et al. pROC: an open-source package for R and S+ to analyze and compare ROC curves. BMC Bioinformatics 2011;12:77. [Crossref] [PubMed]
- Sun X, Wang J, Huang M, et al. STAT3 promotes tumour progression in glioma by inducing FOXP1 transcription. J Cell Mol Med 2018;22:5629-38. [Crossref] [PubMed]
- Maddika S, Ande SR, Panigrahi S, et al. Cell survival, cell death and cell cycle pathways are interconnected: implications for cancer therapy. Drug Resist Updat 2007;10:13-29. [Crossref] [PubMed]
- Lin H, Wei S, Hurt EM, et al. Host expression of PD-L1 determines efficacy of PD-L1 pathway blockade-mediated tumor regression. J Clin Invest 2018;128:1708. [Crossref] [PubMed]
- Aaronson DS, Horvath CM. A road map for those who don't know JAK- STAT. Science 2002;296:1653-5. [PubMed]
- Chen SL, Cai GX, Ding HG, et al. JAK/STAT signaling pathway-mediated microRNA-181b promoted blood-brain barrier impairment by targeting sphingosine-1-phosphate receptor 1 in septic rats. Ann Transl Med 2020;8:1458. [Crossref] [PubMed]
- O'Shea JJ, Pesu M, Borie DC, et al. A new modality for immunosuppression: targeting the JAK/STAT pathway. Nat Rev Drug Discov 2004;3:555-64. [Crossref] [PubMed]
- Park HK, Kim IH, Kim J, et al. Induction of apoptosis and the regulation of ErbB signaling by laminarin in HT-29 human colon cancer cells. Int J Mol Med 2013;32:291-5. [Crossref] [PubMed]
- Shukla S, Bhaskaran N, Babcook MA, et al. Apigenin inhibits prostate cancer progression in TRAMP mice via targeting PI3K/Akt/FoxO pathway. Carcinogenesis 2014;35:452-60. [Crossref] [PubMed]
- Liongue C, O'Sullivan LA, Trengove MC, et al. Evolution of JAK-STAT pathway components: mechanisms and role in immune system development. PLoS One 2012;7:e32777 [Crossref] [PubMed]
- Owen KL, Brockwell NK, Parker BS. JAK-STAT Signaling: A Double-Edged Sword of Immune Regulation and Cancer Progression. Cancers (Basel) 2019;11:2002. [Crossref] [PubMed]
- Balli D, Ren X, Chou FS, et al. Foxm1 transcription factor is required for macrophage migration during lung inflammation and tumor formation. Oncogene 2012;31:3875-88. [Crossref] [PubMed]
- Gage MC, Bécares N, Louie R, et al. Disrupting LXRα phosphorylation promotes FoxM1 expression and modulates atherosclerosis by inducing macrophage proliferation. Proc Natl Acad Sci U S A 2018;115:E6556-65. [Crossref] [PubMed]
- Liu Q, Ai B, Kong X, et al. JAK2 expression is correlated with the molecular and clinical features of breast cancer as a favorable prognostic factor. Int Immunopharmacol 2021;90:107186 [Crossref] [PubMed]
- Miller CP, Thorpe JD, Kortum AN, et al. JAK2 expression is associated with tumor-infiltrating lymphocytes and improved breast cancer outcomes: implications for evaluating JAK2 inhibitors. Cancer Immunol Res 2014;2:301-6. [Crossref] [PubMed]
- Wu F, Fan J, He Y, et al. Single-cell profiling of tumor heterogeneity and the microenvironment in advanced non-small cell lung cancer. Nat Commun 2021;12:2540. [Crossref] [PubMed]
(English Language Editor: J. Jones)