Complication and lung function impairment prediction using perfusion and computed tomography air trapping (CLIPPCAIR): protocol for the development and validation of a novel multivariable model for the prediction of post-resection lung function
Introduction
Lung cancer is the leading cause of cancer death in men (1) and incidence is increasing in women (2). The standard treatment currently offering the best survival is surgical resection. However, the vast majority of lung cancer patients have lung disability that can limit surgical indications. Predicting the effect of a hypothetical resection on lung function plays an essential role in determining surgical candidates by assessing the risk of postoperative complications and long-term respiratory failure. Current prediction algorithms can involve performing additional specific examinations (such as ventilation perfusion scintigraphy or stress testing) for patients in the “gray zone” and deemed high risk. Perfusion scintigraphy enters the decision algorithm to predict postoperative forced expiratory volume at 1 second (FEV1ppo). Though currently necessary for many patients, such FEV1ppo estimates are not only obviously imperfect (3), they can also delay treatment, thus increasing patient anxiety and loss of opportunity (4,5).
Though certain studies have suggested that computed tomography (CT), in particular dual energy CT, can provide more-precise or at least complementary data during pre-surgical evaluations (6-8), the gains when predicting FEV1ppo (9-12) remained modest compared to scintigraphy. However, recent advancements in CT scanning and post-image processing have provided new means of assessing factors affecting postoperative lung function that until now have remained under-studied: (I) disease severity and especially chronic obstructive pulmonary disease (COPD) heterogeneity (7,13,14); (II) the capacity of residual parenchyma for postoperative compensation or functional expansion, which varies according to the underlying parenchyma and the resected lobe (6); (III) postoperative changes in thoracic perfusion (12).
Micro-scanning studies (15-19) have demonstrated that distal airway rarefaction precedes emphysema and functional limitation due to COPD and starts in the early phase of the disease before symptoms appear. Approximately 80% of the distal airways are destroyed in the final stage of the disease (GOLD 4). The degree of distal obstruction may thus play a role in the genesis of postoperative respiratory disability. Obstruction of the distal airways (<2 mm) is not directly visible via CT, but can be indirectly quantified by measuring expiratory air-trapping (20-22).
A vascular origin for COPD was also mentioned as early as the 1950s and illustrated by vascular rarefaction on pulmonary angiographies. The latter was recently updated by advanced processing of CT images (23). Additionally, CT perfusion measurements were more accurate than lung scintigraphy in predicting postoperative lung function (24). However, the latter study did not include an appropriate validation population and their true predictive value or generalizability therefore remains unknown. Given the diverse ways in which CT data may now improve FEV1ppo estimates (via new methods for describing air-trapping and perfusion variables), our study aims to fill this gap. If the CT scan currently often required for assessing the extent of pulmonary cancer can simultaneously provide precise values for FEV1ppo, recourse to pre-surgical pulmonary scintigraphy would no longer be necessary and the preoperative planning pathway thus simplified.
Objectives
The primary objective of the CLIPPCAIR study is to construct and validate a new algorithm for predicting post-operative FEV1 values (FEV1ppo) for lung resection candidates using dual energy chest CT scan data. Secondarily, the predictions made using traditional scintigraphic data will be compared with those from the new algorithm, and cumulative contrast media and irradiation doses associated with imaging will be presented. How other measures of pulmonary function [e.g., transfer factor of the lung for carbon monoxide (TLCO)] and the presence/absence of operative complications might be predicted will also be investigated. Additionally, we will also take the opportunity to explore the potential links between (I) pre-surgical imaging data, (II) post-surgical changes in respiratory function, and (III) changes in health related quality of life. Finally, the creation of a tissue sample collection will provide the means for an ancillary micro CT study.
Methods
Study design
The CLIPPCAIR study is an open, prospective study designed for the development and validation of an algorithm based on novel CT data for the prediction of residual lung function 6 months after lung resection surgery. This study will be conducted according to the principles of the Declaration of Helsinki (as revised in 2013) and was approved by a randomly assigned, independent, ethics committee (Comité de Protection des Personnes Sud-Est III, reference number: 2018- 050 B) as per French law on 28 December 2018. The study was registered on clinicaltrials.gov (NCT03885765) and reporting will be performed according to TRIPOD guidelines (available at https://dx.doi.org/10.21037/atm-21-214) (25,26). Two study groups will be recruited, consisting of the first 60 patients (the “test” group) for algorithm development and the subsequent 100 patients for external validation (the “validation” group) (Figure 1). Predicted values for post-surgical FEV1 (% predicted, determined according to novel CT data (primary objective) or classic methods (a segment-counting algorithm (27), the British Thoracic Society algorithm (28), and lung perfusion scintigraphy)) will be confronted with real values using correlation statistics, the goal being to improve said correlation.
Setting and participant eligibility
This study will take place at the Arnaud de Villeneuve Hospital, Montpellier University Hospitals, Montpellier, France. The targeted study population corresponds to non-small cell lung cancer patients requiring pulmonary resection or lobectomy or pneumonectomy and who need to update their CT scan prior to surgery. Detailed eligibility criteria are given in Table 1. With the exception of those that directly affect lung function, subjects may participate in other cancer treatment studies as well, given that this represents a real-life situation for most cancer patients, and we do not wish to deprive patients of novel treatment opportunities. Written, informed consent for study participation is required of all study candidates prior to enrolment. The single payer national health insurance program in France provides health care to all residents/citizens; the recruited patients should therefore represent a large range of socioeconomic and urban-versus-rural backgrounds.
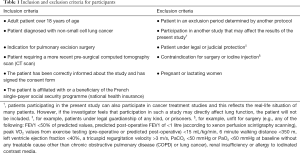
Full table
Predicted and explanatory variables
Outcomes to be predicted
The primary outcome to be predicted is the FEV1 (% predicted and then in litres) from routine spirometry at 6 months following lung resection surgery (Table 2). The correlation between predicted and real values for post-surgical FEV1% predicted will also be considered as a main outcome. Secondarily, we will also develop predictive models for TLCO and the presence/absence of complications and their severity grade according to the Seely classification (29).
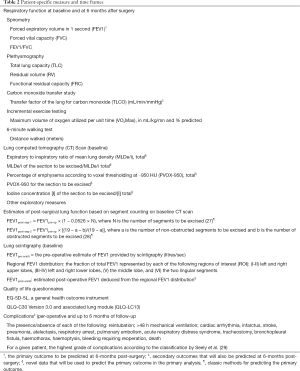
Full table
Explanatory variables
The novel predictive data in this study includes 5 predefined variables derived from baseline thoracic CT scans as indicated in Table 2. The latter cover expiratory to inspiratory ratios of mean lung density (MLDe/i), the percentage of voxels attenuating at less than ‒950 HU (PVOX‒950) and iodine concentrations for both the total lung and for resected sections. MLDe/i estimates the extent of air-trapping experienced by the patient, while PVOX‒950 represents the extent of emphysema as explained in Figure 2. The performance of any new predictive models developed for the primary outcome will be compared with analogous previously published algorithms as presented in Table 2. We will also be exploring CT data for further potential variables with predictive capabilities that are currently unknown.
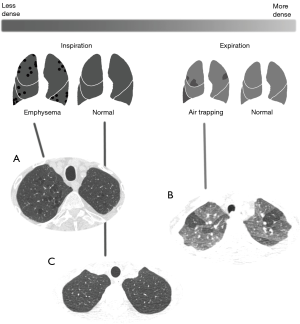
Blinding
Given that this study is based on routine practices with assessments performed by separate teams, and furthermore that modelling occurs after completed data collection for a given subsequent population, the blinding of predicted and explanatory variables when performing assessments was deemed unnecessary.
Describing the study population: baseline and treatments
Basic demographics (age, sex, weight, height and body mass index), comorbidities (specifically, the starting dates for renal insufficiency, diabetes, diffuse interstitial pneumonia, or ischemic heart disease, if present), whether or not the patient is participating in another interventional study, as well as commonly used scores (Charlson score, WHO score, ASA score and Thoracoscore) will be used to describe the population at baseline. Baseline pulmonary function will also be recorded. A listing of any concomitant treatments will be maintained for each patient throughout the study, including adjuvant radio- or chemotherapy. Surgical resection will be characterised by type (pneumonectomy, bi-lobectomy, lobectomy, or lobectomy with anastomosis resection), whether or not lymph node dissection was performed, and whether or not the resection was atypical. Cancer staging using the TNM system will be described once at baseline based on imaging data (cTNM), and again following surgery and incorporating pathology results from excised tissue (pTNM).
Assessments
Spirometry and plethysmography
Spirometry/plethysmography will be carried out as recommended by the European Respiratory Society (30) using Body Box equipment (Medisoft Bodybox 5500, Sorinnes, Belgium). With the exception of the FEV1/FVC ratio, which is calculated as litres/litres, all outcomes listed in Table 2 will be calculated as both % predicted values and as litres.
Single-breath carbon monoxide uptake in the lung
The uptake of carbon monoxide by the lung will be described by the TLCO (mL/min/mmHg) according to current recommendations (31).
Incremental exercise testing
The maximal oxygen consumption (VO2max) during incremental exercise testing will be determined. The latter will be performed according to the American Thoracic Society/American College of Chest Physicians statement (32) using a stationary bicycle (Ergoselect, Sorinnes, Belgium). Briefly, theoretical maximum power is first calculated according to age, sex and body mass index (BMI). The test then starts with a 3-minute warm-up period at 20% of maximum power. Subsequently, power is incremented every minute with increments chosen according to the physician’s discretion. The test ends with volitional fatigue.
6-minute walking test
The distance walked during a 6-minute walking test performed according to current recommendations (33) will be recorded.
Thoracic CT scans and image post-processing
CT is performed using “Revolution CT” (General Electric) equipment and with respiratory gating (WinspiroPRO, Medical International Research, version 7.9.0). Dual energy acquisitions employing rapidly changing kilovoltage will be used (the x-ray generator will rapidly commute between 80 and 140 kV during a single acquisition). Inspiratory acquisitions (DLP <30 mGy-cm) are performed at total lung capacity and expiratory acquisitions (DLP <30 mGy-cm)at residual volume according to recommendations set by the French Radiology Society (http://www.sfrnet.org). The injected contrast media used will be Iomeperol at 350 mg iodine per mL (Iomeron, Bracco Imaging, France).
Image post-processing will be performed by a single radiologist (SB) at a General Electric AW Volumeshare 7 station and will generate (I) a pulmonary perfusion map for visualizing zones of hypoperfusion, (II) measures of mean lung density, and (III) the percentages of voxels attenuating at <‒950 HU. For pulmonary perfusion mapping, Gemstone Spectral Imaging software will be used and the concentration of iodine [I] in the chosen zone measured. Myrian software (Intrasense, Montpellier, France) will be used to determine mean lung density of a given zone. The latter software will also be used to segment emphysematous tissues according to an attenuation threshold at ‒950 HU, as in Gevenois et al. (34). Measures (as listed in Table 2) will be made for the total lung acquisition, as well as for future resected zones.
Perfusion lung scintigraphy
Perfusion lung scintigraphy is performed for those patients who are considered at increased risk for post-operative lung insufficiency (i.e., those with COPD and impaired pre-operative FEV1, who are likely to undergo postoperative respiratory insufficiency). The patients are installed in a seated, upright position and 185 MBq of 99mTc-macroaggregated albumin are injected intravenously. Subsequently, scintigraphic data are acquired using a large-field-of-view dual-head gamma-camera as in (35) and according to current recommendations (36). Scintigraphic images are recorded in planar mode using the two detectors of the gamma-camera facing the back of the patient in left and right posterior oblique incidences. The contribution of each lung lobe to the global FEV1 is estimated and a simple subtraction of FEV1 fractions corresponding to regions-to-be-resected provides an estimate of post-surgical FEV1 (see Table 2).
Quality of life questionnaires
Two validated quality of life questionnaires available in French will be administrated. The first is a generic measure of health status (EQ-5D-5L) applicable to any patient population (37,38), while the second (QLQ-C30 Version 3.0) was designed for cancer patients (39-42), with a sub-module (QLQ- LC13) specific to lung cancer (43,44). The EQ-5D-5L results in a single index score describing a general health profile ranging from 0 to 1, as well as a visual analogue scale score for overall health evaluation. The QLQ-C30 Version 3.0 results in three scale scores [(I) level of function, (II) global health status, and (III) symptomatology/problems] with higher scores (ranging from 0 to 100) representing a higher response level (45). The QLQ-LC13 provides an additional lung-cancer-specific symptom scale ranging from 0 to 100 with higher scores indicating worse symptomatology (45).
Patient pathways
Throughout the study, patient pathways, i.e., episodes of hospitalization, intensive care, and mechanical ventilation, will be separately characterised by their beginning and end dates. The duration of the initial hospitalization will be determined, as well as the cumulative number of days of each episode type at the end of the study. In addition, the cumulative days alive and non-hospitalized at the end of the study will be determined for each patient. These episodic data are important descriptors of disease severity.
Harms
The potential burdens associated with imaging will be characterized via the quantities of iodine products administered and x-ray exposition data (in Dose length product (DLP) and mSv).
The risk-adjusted morbidity and mortality for lung resection for lung cancer as endorsed by the Society of Thoracic Surgeons (https://www.sts.org/quality-safety/performance-measures) will be calculated for the study population. Complications (i.e., any deviation from a normal post-operative cursus) will also be recorded on a per-patient basis and will specifically note the presence/absence of elements listed in Table 2. In addition, each complication encountered will be graded according to Seely et al. (29):
- Grade 1: any complication without need for pharmacologic treatment or other intervention;
- Grade 2: any complication that requires pharmacologic treatment or minor intervention only;
- Grade 3: any complication that requires surgical, radiologic, endoscopic intervention or multitherapy;
- Grade 3a: intervention does not require general anaesthesia;
- Grade 3b: intervention requires general anaesthesia;
- Grade 4: any complication requiring intensive care unit management and life support;
- Grade 4a: single organ dysfunction;
- Grade 4b: multiorgan dysfunction;
- Grade 5: any complication leading to the death of the patient.
Finally, any other adverse events will also be recorded and reported in line with French regulations in force.
Logistics
Screening, enrolment and baseline assessments
The visits required during this study correspond to usual, routine care, and are listed in Figure 3 with corresponding interventions and assessments detailed in Table 3.
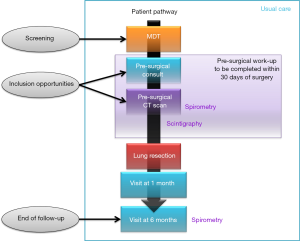
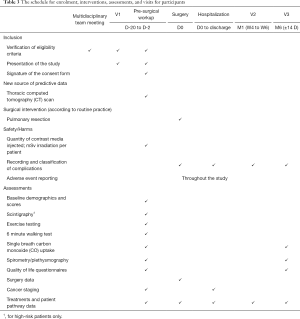
Full table
Preliminary patient screening will take place during weekly multidisciplinary team (MDT) meetings during which lung cancer cases requiring resection will be discussed; confirmation of eligibility and enrolment will be carried out by investigators during visits required for the pre-surgical workup (Figure 3). Care will be taken during the screening and enrolment process to retain sufficient data for the production of a flow chart demonstrating how the study population was constituted.
The study will be presented to eligible patients by investigators during a pre-surgical consult, and the signature of the study consent form collected by the investigator during the visit required for thoracic CT scanning (the timing of the latter two visits may be inversed as long as the patient is correctly informed about the study and has sufficient time to consider study participation). Following enrolment and prior to surgery, the required baseline data will be recorded and the patient will be asked to fill out quality of life questionnaires; outcomes (Table 2) pertaining to baseline spirometry, scintigraphy and CT scan data will be gathered and recorded in the study CRF.
Surgery, hospitalization, and follow-up
Surgery (which defines ‘day 0’ for the study) will be carried out as per usual care with no particular constraints imposed by the present study. Throughout the initial hospitalization, data recording will include treatments, the occurrence and characterization of surgical complications and adverse events, the results from the routine pathological analysis of excised tissues (pTNM characterisation), as well as patient pathway data (episodes of intensive care and/or mechanical ventilation, plus length of initial hospital stay).
The recording of treatments, complications, adverse events, and patient pathway data will continue throughout the 6 months of follow-up required for this study. Medical records and routine visits at 1 month and 6 months will be used to recover these data. In addition, during the 6-month visit, patients will be requested to re-fill out the study questionnaires, and their lung function will be characterized via spirometry, plethysmography and TLCO testing. If required, the latter assessment is postponed in case of intercurrent infection or residual thoracic pain.
Resection samples and tissue library
Resected lung tissues will be primarily dedicated to routine pathological analysis. However, if the eligibility criteria in Table 4 are met, a block of paraffin-embedded lung tissue may be retained for an ancillary micro-scanning study. The specific micro-scanning procedures used will be detailed in the separate ancillary study protocol foreseen for N=40 patients.
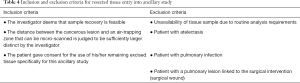
Full table
Sample size
We assume that a model with a maximum of 5 predictive parameters will be developed and have allowed for 10 subjects per parameter. An additional 10 subjects are considered in order to allow for potentially unusable data, resulting in a test-group samples size of 60. This number of subjects is quite similar to that of the study by Chae et al. (24), who developed a similar model with a population of N=51, but performed no validation study. A validation sample of 100 further subjects was chosen based on our recruitment capacity. Though based on a rule that is more empirical than statistical (there is no data in the literature on which we can base a specific hypothesis), the total size (N=160) of the sample is compatible with our inclusion potential and the size of the validation cohort allows a good estimate of the prediction error for the sample.
Potentially missing data
This study is based on routinely available data sources with no experimental changes to patient pathways, and we therefore expect few missing data. Only complete cases (i.e., those with a CT scan at baseline and spirometry at baseline and at 6 months) will be analysed.
Statistical analysis
Statistical analyses will be performed by the Clinical Research and Epidemiology Unit (CREU) at the University Hospitals of Montpellier (Montpellier, France). The programming environments used may include SAS (SAS Institute, Cary, NC, USA), R (46), or Julia (47). The statistical methods presented here will serve as a general guide, and a detailed statistical analysis plan (SAP) will be developed prior to N=50 inclusions (i.e., before the end of the test-population inclusions). Any deviations from the SAP must be justified, approved by the study methodologist (NM) and included in the final study report.
The main parameters studied (demographic and clinical data, predictors and outcomes) will be compiled at each time point for each group. Care will be taken to juxtapose results for the test versus validation groups so that differences in centrality and/or variation can be identified. Before considering the use of statistical tests, the underlying assumptions will be verified with appropriate methods (e.g., Shapiro’s normality test), guiding relevant data transformations. Where appropriate, the Box-Cox transformation is preferred to arbitrary transformations.
Descriptive statistics will be presented as numbers and percentages for qualitative variables, means ± standard deviations for quantitative variables whose distribution is Gaussian, and medians with interquartiles for other variables. Sample sizes demonstrating missing data will be presented for each variable.
The general strategy of the primary analysis is to use data from the test population to create a predictive model for post-operative outcomes at six months after resection surgery. Primary (FEV1) and secondary (TLCO, complications) outcomes will be predicted using (A) five a priori-specified variables (expiratory to inspiratory ratios of mean lung density (MLDe/i for the total lung and resected volume), the percentage of voxels attenuating at less than ‒950 HU (PVOX‒950 for the total lung and resected volume) and the ratio of iodine concentrations for the resected volume over that of the total lung), (B) previously published variable combinations, and (C) exploratory combinations of variables.
The preferred method for predicting post-resection FEV1 or TLCO is multivariable linear regression, while logistic regression will be used for predicting post-resection complications. In case of non-linearity between continuous variables, the following may be used: variable transformation, restricted cubic splines or fractional polynomial functions (the model with the best fit statistics will be retained). The categorization of continuous variables is not foreseen and discouraged, and if used must be thoroughly justified and approved by the study methodologist. For each model, an automated backward-stepwise process minimizing the Aikake Information Criterion (AIC) will be used to select the most appropriate combination of explanatory variables.
Predictive scores generated using the test population will be externally validated using data from the subsequently recruited validation population (see Figure 1). Least-square errors for predicted versus real values will be calculated, and the prediction error calculated with a 95% confidence interval. Given the small samples size of this first study, risk-stratified sub-groups will not be addressed. Following validation of the primary models, model updating using the combined test and validation sets will be performed on an exploratory basis, with internal validation via bootstrapping.
Data entry and quality verifications
Individual patient data will be entered in a password-protected, web-accessible eCRF (Ennov Clinical; https://en.ennov.com) by participating investigators or their approved delegates. A paper-version of the eCRF will be made available at https://osf.io/2m7z4/, and can be used to facilitate field requirements for speed or provide a back-up data collection tool in case of temporary electronic system unavailability. In as much as possible, eCRF data entry will occur in real-time, thus taking advantage of specifically designed data format, range and coherence rules. The Clinical Research and Epidemiology Unit (CREU) at the University Hospitals of Montpellier (Montpellier, France) will oversee coherence rules, database maintenance and data management procedures. eCRF content will be audited against source documents by sponsor-designated data-monitoring personnel throughout the study, and corrections made via a well-documented (traceable) system of queries and responses.
De-identifying data
In the eCRF and subsequent study database, participants will be de-identified and represented by a study number. Publicly available deliverables listing individual data will be completely de-identified, with any data suspected of facilitating patient re-identification removed. Public availability will be subject to specific foreseen usage criteria, as specified in the study data sharing plan (available at https://osf.io/2m7z4/).
Monitoring study conduct
The project will be monitored by sponsor CRAs via regular on-site inspections, the frequency of which will be adapted to the rhythm of inclusions. The following visits are planned at the time of this publication: study initiation, end of inclusions for the test cohort, end of follow-up for the test cohort, end of inclusions for the validation cohort, study closure. All monitoring visits will be the subject of a written report and will cover consent procedures, primary endpoint data quality, and protocol adherence.
Study steering committee and communication activities
(I) The CLIPPCAIR steering committee (SB, AB, IV, NM, CMS) will supervise study implementation and execution, (II) the creation and management of the associated tissue resection collection, and (III) oversee communication activities in accordance with the data-sharing plan. Access to key study documents (participant information materials, statistical analysis plan, analytic code, data sharing plan) will be centralized via the study’s Open Science Framework website: https://osf.io/2m7z4/.
Aggregated study results will be made available on clinicaltrials.gov and via publication in a peer reviewed journal. Authorship will be attributed according to the criteria stipulated by the ICMJE. In accordance with French regulations, study participants will be provided with results upon request. Finally, the study data-sharing plan stipulates that in accordance with French regulations, data will be made available to persons having received approval from the National Commission for Informatics and Liberties (CNIL, France) for a re-analysis protocol.
Study time frames and status
The initial planning for the CLIPPCAIR study calls for eighteen months of inclusions, followed by 6 months of follow-up. At the time of publication of this work, the study is in the early phases of inclusion. The first inclusion occurred on 21 September 2020.
Discussion
This study will produce a novel prediction algorithm for estimating post- resection lung function using novel CT scan data. This algorithm will be presented with all required coefficients and explanations for implementation by the wider scientific community for individual prediction. Should the resulting predictions be comparable or superior to those provided by lung perfusion scintigraphy, patient pathways may be simplified by eliminating the need for the scintigraphy (thus economising time and opportunity for cancer patients, and health care resources for the state).
There are certain limitations to this protocol. First, sample size was determined in quite a practical fashion. There is also a risk that the test and validation samples may differ in some unforeseen way, perhaps due to seasonality. Nevertheless, the planned size of this study is comparable to a previous, similar, influential study that lacked external validation (24). Our perspectives therefore include the construction of a novel prediction algorithm based on more recent imaging post-treatment techniques, accompanied by an improved evidence base via external validation.
Acknowledgments
Funding: This work was supported by the 2017 Montpellier University Hospitals Young Researcher Clinical Research Internal Call-for-tender (AOI Montpellier 2017 – RC JC: RECHMPL17_0370).
Footnote
Reporting Checklist: The authors have completed the TRIPOD reporting checklist. Available at https://dx.doi.org/10.21037/atm-21-214
Conflicts of Interest: All authors have completed the ICMJE uniform disclosure form (available at https://dx.doi.org/10.21037/atm-21-214). Dr. Suehs reports grants from Astra Zeneca, outside the submitted work. Dr. Molinari reports personal fees from Astra Zeneca, grants from GSK, outside the submitted work. Dr. Bourdin reports grants, personal fees, non-financial support and other (Ad Board; participation in congress; investigator) from Astra Zeneca, grants, personal fees and other (Ad Board; participation in congress; investigator) from GSK, grants, personal fees, non-financial support and other (Ad Board; participation in congress; investigator) from Boeringher Ingelheim, personal fees, non-financial support and other (Ad Board; participation in congress; investigator) from Novartis, personal fees and other (Ad Board; investigator) from Teva, personal fees and other (Ad Board; investigator) from Regeneron, personal fees, non-financial support and other (Ad Board; participation in congress; investigator) from Chiesi Farmaceuticals, personal fees, non-financial support and other (Ad Board; participation in congress; investigator) from Actelion, other (Investigator) from Gilead, personal fees, non-financial support and other (Ad Board; investigator) from Roche, outside the submitted work.
Ethical Statement: The authors are accountable for all aspects of the work in ensuring that questions related to the accuracy or integrity of any part of the work are appropriately investigated and resolved. This study will be conducted according to the principles of the Declaration of Helsinki (as revised in 2013) and was approved by a randomly-assigned, independent, ethics committee (Comité de Protection des Personnes Sud-Est III, reference number: 2018- 050 B) as per French law on 28 December 2018. Informed, written consent for study participation is required of all study candidates prior to enrolment.
Open Access Statement: This is an Open Access article distributed in accordance with the Creative Commons Attribution-NonCommercial-NoDerivs 4.0 International License (CC BY-NC-ND 4.0), which permits the non-commercial replication and distribution of the article with the strict proviso that no changes or edits are made and the original work is properly cited (including links to both the formal publication through the relevant DOI and the license). See: https://creativecommons.org/licenses/by-nc-nd/4.0/.
References
- Bray F, Ferlay J, Soerjomataram I, et al. Global cancer statistics 2018: GLOBOCAN estimates of incidence and mortality worldwide for 36 cancers in 185 countries. CA Cancer J Clin 2018;68:394-424. [Crossref] [PubMed]
- Fidler-Benaoudia MM, Torre LA, Bray F, et al. Lung cancer incidence in young women vs. young men: A systematic analysis in 40 countries. Int J Cancer 2020;147:811-9. [Crossref] [PubMed]
- Mathew B, Nag S, Agrawal A, et al. Comparison of predicted postoperative forced expiratory volume in the first second (FEV1) using lung perfusion scintigraphy with observed forced expiratory volume in the first second (FEV1) post lung resection. World J Nucl Med 2020;19:131-6. [Crossref] [PubMed]
- Pujol JL, Quantin X. Time to diagnosis of lung cancer: technical and pyschological factors that slow down diagnostic and treatment timelines. J Thorac Oncol 2009;4:1192-4. [Crossref] [PubMed]
- Nadpara P, Madhavan SS, Tworek C. Guideline-concordant timely lung cancer care and prognosis among elderly patients in the United States: A population-based study. Cancer Epidemiol 2015;39:1136-44. [Crossref] [PubMed]
- Jamadar DA, Kazerooni EA, Martinez FJ, et al. Semi-quantitative ventilation/perfusion scintigraphy and single-photon emission tomography for evaluation of lung volume reduction surgery candidates: description and prediction of clinical outcome. Eur J Nucl Med 1999;26:734-42. [Crossref] [PubMed]
- Thurnheer R, Engel H, Weder W, et al. Role of lung perfusion scintigraphy in relation to chest computed tomography and pulmonary function in the evaluation of candidates for lung volume reduction surgery. Am J Respir Crit Care Med 1999;159:301-10. [Crossref] [PubMed]
- Bolliger CT, Gückel C, Engel H, et al. Prediction of functional reserves after lung resection: comparison between quantitative computed tomography, scintigraphy, and anatomy. Respiration 2002;69:482-9. [Crossref] [PubMed]
- Wu MT, Chang JM, Chiang AA, et al. Use of quantitative CT to predict postoperative lung function in patients with lung cancer. Radiology 1994;191:257-62. [Crossref] [PubMed]
- Wu MT, Pan HB, Chiang AA, et al. Prediction of postoperative lung function in patients with lung cancer: comparison of quantitative CT with perfusion scintigraphy. AJR Am J Roentgenol 2002;178:667-72. [Crossref] [PubMed]
- Yoshimoto K, Nomori H, Mori T, et al. Prediction of pulmonary function after lung lobectomy by subsegments counting, computed tomography, single photon emission computed tomography and computed tomography: a comparative study. Eur J Cardiothorac Surg 2009;35:408-13. [Crossref] [PubMed]
- Choe J, Lee SM, Chae EJ, et al. Evaluation of postoperative lung volume and perfusion changes by dual-energy computed tomography in patients with lung cancer. Eur J Radiol 2017;90:166-73. [Crossref] [PubMed]
- Yoshimoto K, Nomori H, Mori T, et al. Quantification of the impact of segmentectomy on pulmonary function by perfusion single-photon-emission computed tomography and multidetector computed tomography. J Thorac Cardiovasc Surg 2009;137:1200-5. [Crossref] [PubMed]
- Yoshimoto K, Nomori H, Mori T, et al. A segmentectomy of the right upper lobe has an advantage over a right upper lobectomy regarding the preservation of the functional volume of the right middle lobe: analysis by perfusion single-photon emission computed tomography/computed tomography. Surg Today 2010;40:614-9. [Crossref] [PubMed]
- McDonough JE, Yuan R, Suzuki M, et al. Small-airway obstruction and emphysema in chronic obstructive pulmonary disease. N Engl J Med 2011;365:1567-75. [Crossref] [PubMed]
- Hogg JC, McDonough JE, Suzuki M. Small airway obstruction in COPD: new insights based on micro-CT imaging and MRI imaging. Chest 2013;143:1436-43. [Crossref] [PubMed]
- Scott AE, Vasilescu DM, Seal KA, et al. Three dimensional imaging of paraffin embedded human lung tissue samples by micro-computed tomography. PLoS One 2015;10:e0126230 [Crossref] [PubMed]
- Mai C, Verleden SE, McDonough JE, et al. Thin-Section CT Features of Idiopathic Pulmonary Fibrosis Correlated with Micro-CT and Histologic Analysis. Radiology 2017;283:252-63. [Crossref] [PubMed]
- Vasilescu DM, Phillion AB, Tanabe N, et al. Nondestructive cryomicro-CT imaging enables structural and molecular analysis of human lung tissue. J Appl Physiol (1985) 2017;122:161-9. [Crossref] [PubMed]
- Bommart S, Marin G, Bourdin A, et al. Relationship between CT air trapping criteria and lung function in small airway impairment quantification. BMC Pulm Med 2014;14:29. [Crossref] [PubMed]
- Bommart S, Marin G, Bourdin A, et al. Computed tomography quantification of airway remodelling in normal ageing subjects: a cross-sectional study. Eur Respir J 2015;45:1167-70. [Crossref] [PubMed]
- Bommart S, Kovacsik H, Vachier I, et al. Software-assisted Computed Tomography Quantification of Airway Remodeling in the Normal Aging Process. Am J Respir Crit Care Med 2017;195:540-1. [Crossref] [PubMed]
- Coste F, Dournes G, Dromer C, et al. CT evaluation of small pulmonary vessels area in patients with COPD with severe pulmonary hypertension. Thorax 2016;71:830-7. [Crossref] [PubMed]
- Chae EJ, Kim N, Seo JB, et al. Prediction of postoperative lung function in patients undergoing lung resection: dual-energy perfusion computed tomography versus perfusion scintigraphy. Invest Radiol 2013;48:622-7. [Crossref] [PubMed]
- Moons KG, Altman DG, Reitsma JB, et al. Transparent Reporting of a multivariable prediction model for Individual Prognosis or Diagnosis (TRIPOD): explanation and elaboration. Ann Intern Med 2015;162:W1-73. [Crossref] [PubMed]
- Moons KG, Altman DG, Reitsma JB, et al. New Guideline for the Reporting of Studies Developing, Validating, or Updating a Multivariable Clinical Prediction Model: The TRIPOD Statement. Adv Anat Pathol 2015;22:303-5. [Crossref] [PubMed]
- Juhl B, Frost N. A comparison between measured and calculated changes in the lung function after operation for pulmonary cancer. Acta Anaesthesiol Scand Suppl 1975;57:39-45. [Crossref] [PubMed]
- British Thoracic Society. BTS guidelines: guidelines on the selection of patients with lung cancer for surgery. Thorax 2001;56:89-108. [Crossref] [PubMed]
- Seely AJ, Ivanovic J, Threader J, et al. Systematic classification of morbidity and mortality after thoracic surgery. Ann Thorac Surg 2010;90:936-42; discussion 942. [Crossref] [PubMed]
- Quanjer PH, Stanojevic S, Cole TJ, et al. Multi-ethnic reference values for spirometry for the 3-95-yr age range: the global lung function 2012 equations. Eur Respir J 2012;40:1324-43. [Crossref] [PubMed]
- Graham BL, Brusasco V, Burgos F, et al. ERS/ATS standards for single-breath carbon monoxide uptake in the lung. Eur Respir J 2017;49:1600016 Erratum in Eur Respir J 2017;2018:52.
- American Thoracic Society. ATS/ACCP Statement on cardiopulmonary exercise testing. Am J Respir Crit Care Med 2003;167:211-77. [Crossref] [PubMed]
- ATS Committee on Proficiency Standards for Clinical Pulmonary Function Laboratories. ATS statement: guidelines for the six-minute walk test. Am J Respir Crit Care Med 2002;166:111-7. [Crossref] [PubMed]
- Gevenois PA, de Maertelaer V, De Vuyst P, et al. Comparison of computed density and macroscopic morphometry in pulmonary emphysema. Am J Respir Crit Care Med 1995;152:653-7. [Crossref] [PubMed]
- Mariano-Goulart D, Barbotte E, Basurko C, et al. Accuracy and precision of perfusion lung scintigraphy versus 133Xe-radiospirometry for preoperative pulmonary functional assessment of patients with lung cancer. Eur J Nucl Med Mol Imaging 2006;33:1048-54. [Crossref] [PubMed]
- Parker JA, Coleman RE, Grady E, et al. SNM practice guideline for lung scintigraphy 4.0. J Nucl Med Technol 2012;40:57-65. [Crossref] [PubMed]
- Herdman M, Gudex C, Lloyd A, et al. Development and preliminary testing of the new five-level version of EQ-5D (EQ-5D-5L). Qual Life Res 2011;20:1727-36. [Crossref] [PubMed]
- Schwenkglenks M, Matter-Walstra K. Is the EQ-5D suitable for use in oncology? An overview of the literature and recent developments. Expert Rev Pharmacoecon Outcomes Res 2016;16:207-19. [Crossref] [PubMed]
- Giesinger JM, Kieffer JM, Fayers PM, et al. Replication and validation of higher order models demonstrated that a summary score for the EORTC QLQ-C30 is robust. J Clin Epidemiol 2016;69:79-88. [Crossref] [PubMed]
- Sprangers MA, Cull A, Groenvold M, et al. The European Organization for Research and Treatment of Cancer approach to developing questionnaire modules: an update and overview. EORTC Quality of Life Study Group. Qual Life Res 1998;7:291-300. [Crossref] [PubMed]
- Aaronson NK, Ahmedzai S, Bergman B, et al. The European Organization for Research and Treatment of Cancer QLQ-C30: a quality-of-life instrument for use in international clinical trials in oncology. J Natl Cancer Inst 1993;85:365-76. [Crossref] [PubMed]
- Osoba D, Zee B, Pater J, et al. Psychometric properties and responsiveness of the EORTC quality of Life Questionnaire (QLQ-C30) in patients with breast, ovarian and lung cancer. Qual Life Res 1994;3:353-64. [Crossref] [PubMed]
- Bergman B, Aaronson NK, Ahmedzai S, et al. The EORTC QLQ-LC13: a modular supplement to the EORTC Core Quality of Life Questionnaire (QLQ-C30) for use in lung cancer clinical trials. EORTC Study Group on Quality of Life. Eur J Cancer 1994;30A:635-42. [Crossref] [PubMed]
- Koller M, Warncke S, Hjermstad MJ, et al. Use of the lung cancer-specific Quality of Life Questionnaire EORTC QLQ-LC13 in clinical trials: A systematic review of the literature 20 years after its development. Cancer 2015;121:4300-23. [Crossref] [PubMed]
- Fayers PM, Aaronson NK, Bjordal K, et al. EORTC QLQ-C30 scoring manual. Eortc 1999.
- R Core Team. R: A Language and Environment for Statistical Computing [Internet]. Vienna, Austria: R Foundation for Statistical Computing 2016. Available online: https://www.R-project.org/
- Bezanson J, Edelman A, Karpinski S, et al. Julia: A fresh approach to numerical computing. SIAM Review 2017;59:65-98. [Crossref]