Nomograms-based prediction of overall and cancer-specific survivals for patients diagnosed with major salivary gland carcinoma
Introduction
Major salivary glands carcinoma (MSGC) is a rare malignant tumor, which accounts for 11% of oropharyngeal neoplasms in the United States (U.S.) (1,2). MSGC comprises over 20 different histological subtypes with different biological behavior and therapy responses. Due to the low number of patients in each subtype, there are no randomized phase III studies (3). The management of MSGC is still challenging. It there are no randomized phase III studies is reported that the 5-year overall survival (OS) is only about 50% (4). The rarity of MSGC, combined with complex classification schema and non-standard therapy, makes the prediction of the survival of MSGC patients difficult. Therefore, developing an effective prognostic tool to improve clinical treatment strategies for MSGC is of great clinical importance.
Although there are many prognosis factors for cancers such as sex, race, age, and other characteristics, tumor staging systems are most commonly applied for the assessment of risk and the decision-making of cancer management in clinical practice, especially American Joint Committee on Cancer (AJCC) classification system and TNM staging system (5,6). Still, these systems have limitations: only tumor size, lymph nodes, and distant metastasis were considered. Moreover, MSGC patients classified as in the same stage may have completely different survival outcomes (7). The nomogram is an effective and convenient tool based on a statistical model for quantitative prediction of clinical events using multiple selected prognostic factors. It has been widely applied for predicting survival in patients with malignancies, such as lung cancer, breast cancer, and colon cancer (8-10). In their recent study, Lukovic et al. developed a predictive model for the prediction of distant metastasis (11), thus revealing the broad application prospect of predictive models in clinical practice for MSGC management. Ali et al. created a nomogram for predicting survival and recurrence of major salivary gland carcinoma based on the characteristics of 301 patients recruited at Memorial Sloan Kettering Cancer Centre between 1985 and 2009; however, the sample size of the development cohort was limited (12,13). Furthermore, histological type, which is a key factor in determining the biological behavior of the tumor, is not considered in the nomogram. Li et al. constructed a nomogram for survival prediction of MSGC (14). Nonetheless, improper study design and non-transparent reporting of results significantly damaged the reliability of the study. Therefore, an updated and reliable nomogram for predicting the survival of MSGC patients is urgently needed.
In the present study, we used data of MSGC patients from the Surveillance, Epidemiology, and End Results (SEER) database to develop a predictive model, which was presented as a nomogram, and to test the performance for OS and cancer-specific survival (CSS). We present the following article in accordance with the TRIPOD reporting checklist (available at https://dx.doi.org/10.21037/atm-21-1725) (15).
Methods
Data source and selection criteria
Data for this study were obtained from the SEER database (approval number: 21476-Nov2019), which is an authoritative source for cancer statistics in the United States (https://seer.cancer.gov/). The study was conducted in accordance with the Declaration of Helsinki (as revised in 2013). The ethical approval was waived since the study was based on secondary data analysis.
The inclusion criteria were the following: (I) patients diagnosed with major salivary gland carcinoma since 2004; (II) the survival time of patients is known. Patients were excluded if they met the following conditions: (I) survival time <1 month; (II) patients with missing data; (III) patients only diagnosed by autopsy or death certificate.
Fourteen predictor variables included in the present study were: sex, age at diagnosis, race, marital status, location of the tumor, grade of differentiation, T/N/M stage, AJCC stage, size of the tumor, histological type of the tumor, surgery, and previously having/not having surgery of lymph nodes. Vital status and cancer-specific death, which were primary outcomes in this study, were also extracted. For radiotherapy and chemotherapy, patients were divided into two groups—“Yes” and “No/Unknown” in the SEER. Combining “No” and “Unknown” as a group would probably reduce the effect of the predictor and lead to confusion in the clinical practice. Radiotherapy and chemotherapy were not extracted in this study. All data were extracted using SEER*Stat 8.3.5 software.
Statistical analysis
The whole population extracted from the SEER database was randomly divided into training dataset and validation dataset at ratio 7:3. Categorical variables were analyzed by Chi-square test, and continuous variables (survival months) were analyzed by rank-sum test. Univariate and multivariate Cox proportional hazards regression were performed to assess the relationship between predictor variables and survival outcomes. Hazard ratio (HR) was applied as the effect size. To select predictor variables, the least absolute shrinkage and selection operator (LASSO) regression was adopted (16).
The performance of the predictive model was evaluated in both the training dataset and validation data set. The discriminative performance of the predictive model was evaluated by Harrell C-index (C-index) at a given time point and over time C-index (17). The C-index estimates the probability that the model can correctly sort the sequence of events in a randomly selected pair of cases. The value of the C-index ranges from 0.5 to 1. A higher C-index means better predict performance (18). The receiver operating characteristic (ROC) curves were drawn, and the areas under the curves (AUC) were also calculated. AUC is another parameter for measuring the discriminative power of the model. Calibration curves were drawn to further evaluate the concordance of the predicted survival probability and the observed probability. Brier score, which is squared prediction error, was calculated. A lower Brier score means a lower prediction error (19). The decision curve analysis (DCA) was conducted for clinical significance assessment by calculating net benefit (20). All statistical analyses were performed using R version 4.0.3 (https://www.r-project.org/). An α level was set as 0.05.
Results
Characteristics of patients
A total of 14,753 eligible patients recorded in the SEER databases were initially recruited. Patients whose survival time was less than 1 month were excluded (n=718). Since the design of this study implied complete case analysis, patients with missing values were also excluded (n=2,673). Finally, 11,362 patients were included. Patients were randomly assigned into training dataset (n=7,953) and validation dataset (n=3,409) with a ratio 7:3. The flowchart of patient selection is shown in Figure 1. There was no statistical difference in patients’ distribution between the training and validation dataset (P>0.05). The median survival time for the whole population was 48 months. The majority of the patients were male (n=6,400, 56%), white (n=9,214, 81%), married when diagnosed with MSGC, with an age between 60 and 80 (n=4,592, 40%). Most of lesions were located at parotid gland (n=9,191, 81%) and were classified as AJCC IV stage (n=3,139, 28%). For TNM stage, T1 accounted for 29% (n=3,305), N0 accounted for 67% (n=7,568), and M0 accounted for 89% (n=10,082). The size of most tumors was 0–1 cm (n=9,651, 85%). Mucoepidermoid carcinoma was the most common histological type (n=2,637, 23%). There were 86% patients (n=9,758, 86%) who underwent surgery, while 61% of total population (n=6,896) underwent removal of lymph nodes. A total of 3,616 death cases were reported in this cohort, including 2,338 cancer-related death cases and 1,278 competing death cases. The detailed characteristics of patients are shown in Table 1.
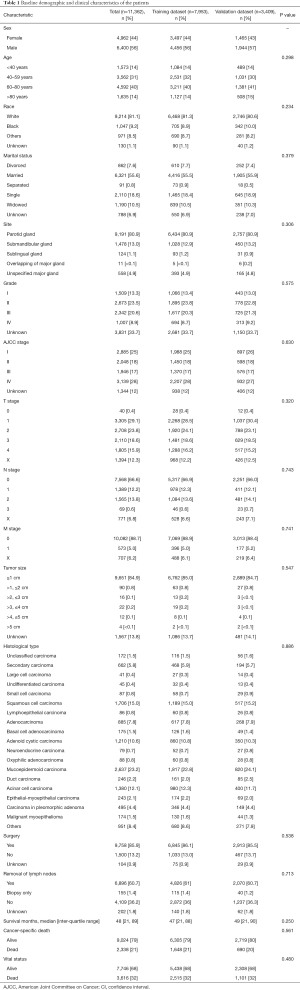
Full table
Establishment of predict model
First, univariate Cox regression was constructed. With reference to OS, all variables were significantly correlated with outcome (P<0.001). The results of multivariate Cox regression showed that sex, age, race, grade, AJCC stage, T/N stage, tumor size, surgery, and histological type were significantly correlated with OS (P<0.001) (Table 2). Both M stage and site also showed a significant correlation (P<0.01), except for marital status and removal of lymph nodes (P>0.05). As for CSS, similar results were obtained in the univariate Cox regression, while marital status and M stage were not significantly associated with CSS in the multivariate Cox regression (P>0.05) (Table 3). Then the LASSO model was applied for variable selection (Figure S1). For OS, only one variable (removal of lymph nodes) was not incorporated in the model. A total of 12 predictors, except for marital status and site, were selected for CSS prediction. The regression coefficients are provided in Table S1.
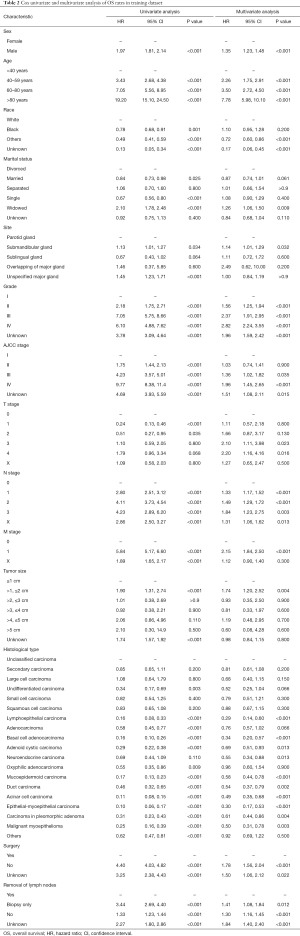
Full table
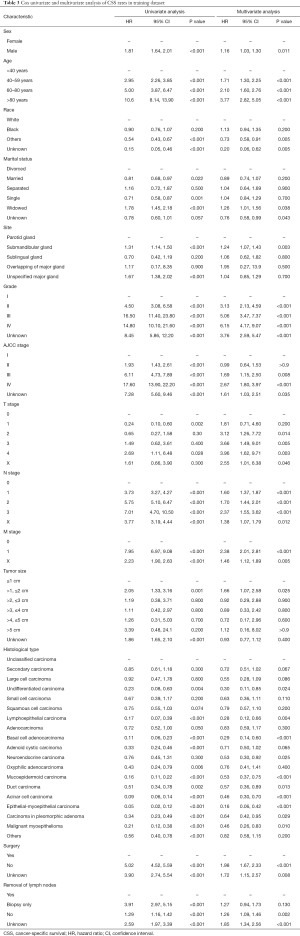
Full table
The nomogram was drawn using the “rms” package (Figure 2). When using the nomogram to predict the survival of patients, a perpendicular line was drawn towards the point line from each predictor to identify the specific value of each variable. The total points were the sum of values for each variable. Then, the survival likelihood was determined by drawing a line from the total points’ line down along the survival axis.
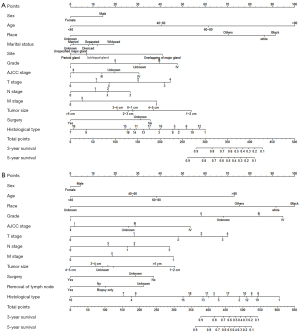
Evaluation of model performance
The performance of the predictive model was compared with the AJCC stage and TNM stage system. The values of AUC, C-index, and Brier score are presented in Table 4 and Table S2. For OS, AUC of nomogram in the training dataset was 83.5 (82.4–84.7), while the AUC of TNM stage and AJCC stage was 72.7 (71.3–74.1) and 71.6 (70.2–73.0), respectively. In the validation dataset, the AUC of the nomogram was 82.7 (81.0–84.3), while the AUC of the TNM stage and AJCC stage were 71.0 (68.9–73.2) and 69.8 (67.7–71.9), respectively. Similar results were found for CSS: nomogram yielded the highest value of AUC of 83.9 (82.6–85.2), followed by TNM stage (75.9, 74.4–77.3) and AJCC stage (73.9, 72.4–75.3) in the training cohort. The AUC values of the nomogram, TNM stage, AJCC stage were 82.8 (80.9–84.7), 74.5 (72.2–76.7), 72.6 (70.4–74.8), respectively, in the validation cohort. As shown in Figure 3 and Figure S2, the AUC values of the nomogram were the best across all settings.
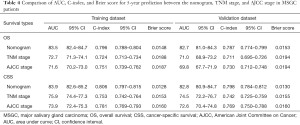
Full table
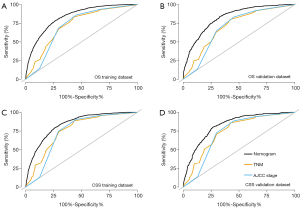
The C-index of the nomogram in predicting OS was 0.796 (0.788–0.804) and 0.787 (0.774–0.799), showing a greater predictive performance compared with TNM stage (0.724, 0.713–0.734; 0.711, 0.695–0.726) and AJCC stage (0.751, 0.739–0.762; 0.730, 0.712–0.748) in the training group and validation group. For CSS, the C-index of nomogram was 0.806 (0.797–0.815) in training group and 0.798 (0.78–0.81) in validation group. The C-index of TNM stage was 0.75 (0.74–0.76) and 0.74 (0.784–0.812), while the C-index of AJCC stage was 0.781 (0.769–0.793) and 0.769 (0.750–0.788) in training set and validation set, respectively. The over-time C-indexes of nomogram were consistently better than TNM stage and AJCC stage throughout the investigated period, as shown in Figure 4.
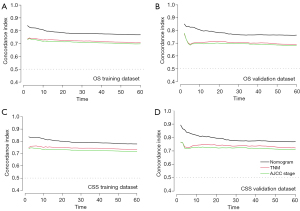
The Brier scores of the nomogram in predicting OS, which was 0.0148 and 0.0153 in the training set and validation set, respectively, were better than the TNM stage and AJCC stage. Concerning the TNM stage and AJCC stage, the Brier scores were 0.0188, 0.0187 in the training group, and 0.0194, 0.0194 in the validation group. As for CSS, the Brier score of the nomogram, TNM stage, AJCC stage in the training dataset were 0.0128, 0.0153, 0.0160, and 0.0130, 0.0155, 0.0160 in the validation set, respectively. The calibration curves displayed by the nomogram were closer to the reference line, which indicated that the nomogram exhibited a higher accuracy than other systems (Figure 5, Figure S3).
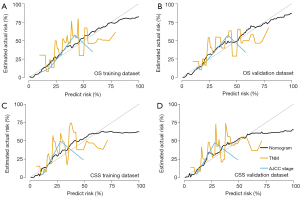
The DCA was adopted for the clinical significance assessment of the nomogram. The performance of the nomogram was also compared with the TNM stage and AJCC stage. As shown in Figure 6 and Figure S4, the net benefits of nomogram were higher compared to the other two systems, no matter in training dataset or validation dataset, for OS or CSS. The results of DCA indicated that the nomogram established by the present study was applicable for predicting the 3- and 5-year CSS and OS.
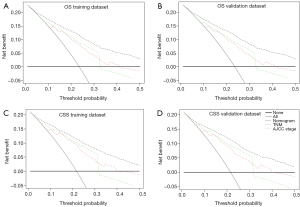
Discussion
The incidence rate of MSGC ranges from 1.1 to 1.3/100,000/year, which is quite low (21,22). The limited sample size greatly impacts the related research, as it increases the research error and may lead to a biased result. SEER database is an authoritative source of information on cancer incidence, diagnosis, therapy, and survival in the United States. This database collects and publishes cancer incidence and survival data from eighteen cancer registries covering approximately 35% of the U.S. population. The SEER database has been widely adopted in cancer-related research (23-25). In this study, a large cohort of patients (n=14,753) diagnosed with MSGC was recruited from SEER. To the best of our knowledge, this is the largest sample size used so far in the research on the prognosis of MSGC.
Given the morphologic diversity and rarity of MSGC, there are no high-quality randomized data defining predictive factors. In a relatively large cohort, site, histological type, race, sex, and age at diagnosis were identified as predictors for MSGC OS (26). Li et al. failed to find a prognostic, predictive factor for lymphoepithelial carcinoma in the major salivary gland in a cohort containing 37 patients (27). In a study comprising 95 patients recruited from a single institution, male sex and >10 pack-year smoking history, grade, N stage, squamous cell carcinoma, perineural invasion, and neck dissection significantly reduced OS (28). In the present study, multivariate Cox analysis identified sex, age, race, grade, AJCC stage, T/N/M stage, tumor size, surgery, histological type, and site as significant factors predicting OS. All predictors, except for marital status and M stage, were significant in multivariate Cox analysis for CSS.
Sex was proven to be an independent risk factor of MSGC survival. Females have been reported to have better survival outcomes compared with males, which is consistent with our result (26,29). Not surprisingly, the risk increases with the patients’ age. As for the race, black people were at the highest risk, followed by white, and then other races, which was consistent with some previous studies (30-32). It is important to note that this may be due to the socioeconomic differences between black people and other races in some areas (33). Poor differentiation grade and high AJCC stage are two independent indicators for worse survival outcomes. T stage and tumor size reflected the condition of the primary site. Interestingly, the patient with a larger primary lesion might have better survival compared to a smaller lesion, which was contradictory to the results of other studies (29). On the one hand, the larger tumor was often associated with better differentiation grade and histological type, which led to better survival outcomes. Patients who suffered from highly malignant tumors usually died before their tumor grew larger. On the other hand, this may be due to the small sample size with large tumors (>1 cm) in this cohort (n=1,171, 15.1%). Whether in univariate, multivariate Cox regression, or LASSO model, tumor size was significantly related to the OS and CSS; thus, it was incorporated in the final model. M stage, which reflects distant tumor metastasis, was one of the most important predictors for cancer survival (34). In the present study, the M stage showed a significant association with OS but not CSS (P>0.05). The important role of distant metastasis in MGSC has been well-recognized (35,36). Considering the clinical significance, the M stage was finally included. Surgery was the primary approach for MSGC management. Patients who underwent surgery had better survival compared to those who did not. In the 2017 World Health Organization classification, there were over 20 different histological subtypes of MSGC, with specific features and outcomes. Consequently, it is important to consider the histological type for prediction survival of MSGC. In the present study, when the number of patients diagnosed as a specific subtype was less than 10, it was put into the category of “Other”. Epithelial-myoepithelial carcinoma and lymphoepithelial carcinoma had better survival, while patients diagnosed with unclassified carcinoma had worse OS and CSS. Radiotherapy and chemotherapy were not incorporated in the model. For radiotherapy and chemotherapy, patients were divided into two groups—“Yes” and “No/Unknown” in the SEER, while “Unknown” was set as a separated group for other variables, such as race, material status, grade, AJCC stage. After discussion, we thought that combining “No” and “Unknown” as a group would reduce the effect of these two variables and might lead to confusion in the clinical practice.
The key strengths in the present study are the following: first, the SEER database provided a total of 11,362 MSGC patients. Compared with a study by Li et al., the sample size in the present study was larger (4,218 vs. 14,753). This cohort, which to the best of our knowledge is the largest one used thus far, is large enough to ensure the reliability and effectiveness of the nomogram. Second, the histological type is considered in the nomogram. The Memorial Sloan Kettering Cancer Center (MSKCC) a salivary carcinoma nomogram, is a common tool used for MSGC survival prediction (12,13,37). Still, unlike the histological type, the only histological grade was considered for the development of the MSKCC nomogram. As an important factor, the survival outcomes in different histological types greatly vary. Third, treatment planning and prognosis prediction are currently dependent on the AJCC stage and TNM stage systems to a great extent. To evaluate the value of using the nomogram, the performance of the nomogram was compared with the AJCC stage and TNM stage. C-index, AUC, Brier score was calculated. Calibration curves, ROC curves, and DCA were also drawn. The result showed that the performance of the nomogram was consistently better compared to the AJCC stage and TNM stage. Finally, even though Li et al. and our nomograms were constructed based on the SEER database, only the present study was conducted according to the TRIPOD statement (30). Moreover, the performance of the nomogram was evaluated all-around to ensure the reliability of the prediction tool.
The present study also has some limitations. The variables extracted from the SEER database were limited. Records related to prognosis, such as lymphovascular invasion, perineural invasion, tobacco/alcohol use, were inaccessible. In this cohort, the seventh edition of the AJCC stage was adopted as only a small portion of patients had information about the eighth edition of the AJCC stage. As a result, the extranodal extension of lymph nodes was not considered in the present study, which is a common limitation in research collecting information from SEER. Moreover, target therapy has an increasingly important role in MSGC treatment (38). Once the patient accepts target therapy, the survival will certainly change. Furthermore, different drugs can also have different roles. Due to the limitation of SEER, the information on target therapy remains unknown. Although the nomogram performed well in internal validation, external validation is needed in future studies.
Conclusions
A reliable nomogram for OS and CSS prediction in MSGC patients based on the Cox regression model was developed using the SEER database. The nomogram showed good predictive discrimination and accuracy compared with the AJCC stage and TNM stage. Consequently, the nomogram can become a practical tool to help clinicians to make a treatment plan and prognosis predictions.
Acknowledgments
The authors would like to thank the SEER program for open access to the database.
Funding: This work was supported by grants from the Natural Science Fund of China (grant No. 81901049), Outstanding Young Scholars of Shanghai Health Commission (2018YQ34), and Young Scholars and Innovative Team of Shanghai Ninth People’s Hospital (QC201901).
Footnote
Reporting Checklist: The authors have completed the TRIPOD reporting checklist. Available at https://dx.doi.org/10.21037/atm-21-1725
Peer Review File: Available at https://dx.doi.org/10.21037/atm-21-1725
Conflicts of Interest: All authors have completed the ICMJE uniform disclosure form (available at https://dx.doi.org/10.21037/atm-21-1725). The authors have no conflicts of interest to declare.
Ethical Statement: The authors are accountable for all aspects of the work in ensuring that questions related to the accuracy or integrity of any part of the work are appropriately investigated and resolved. The study was conducted in accordance with the Declaration of Helsinki (as revised in 2013). Since individual information has been removed from all the SEER databases, informed consent from patients and approval by the institutional review board were exempted.
Open Access Statement: This is an Open Access article distributed in accordance with the Creative Commons Attribution-NonCommercial-NoDerivs 4.0 International License (CC BY-NC-ND 4.0), which permits the non-commercial replication and distribution of the article with the strict proviso that no changes or edits are made and the original work is properly cited (including links to both the formal publication through the relevant DOI and the license). See: https://creativecommons.org/licenses/by-nc-nd/4.0/.
References
- Boukheris H, Curtis RE, Land CE, et al. Incidence of carcinoma of the major salivary glands according to the WHO classification, 1992 to 2006: a population-based study in the United States. Cancer Epidemiol Biomarkers Prev 2009;18:2899-906. [Crossref] [PubMed]
- McKenna RJ. Tumors of the major and minor salivary glands. CA Cancer J Clin 1984;34:24-39. [Crossref] [PubMed]
- Andry G, Hamoir M, Locati LD, et al. Management of salivary gland tumors. Expert Rev Anticancer Ther 2012;12:1161-8. [Crossref] [PubMed]
- Cruz A, Magalhães H, Pereira FF, et al. A 10-year review of primary major salivary gland cancers. Ecancermedicalscience 2020;14:1055. [Crossref] [PubMed]
- Egger EK, Stope MB, Recker F, et al. Lower Genital Tract Melanomas: Staging, Predictors of Outcome, and New Therapeutic Options. Anticancer Res 2021;41:999-1004. [Crossref] [PubMed]
- Pu N, Li J, Xu Y, et al. Comparison of prognostic prediction between nomogram based on lymph node ratio and AJCC 8th staging system for patients with resected pancreatic head carcinoma: a SEER analysis. Cancer Manag Res 2018;10:227-38. [Crossref] [PubMed]
- Lombardi D, Tomasoni M, Paderno A, et al. The impact of nodal status in major salivary gland carcinoma: A multicenter experience and proposal of a novel N-classification. Oral Oncol 2021;112:105076 [Crossref] [PubMed]
- Peng F, Li J, Mu S, et al. Epidemiological features of primary breast lymphoma patients and development of a nomogram to predict survival. Breast 2021;57:49-61. [Crossref] [PubMed]
- Liu C, Hu C, Huang J, et al. A Prognostic Nomogram of Colon Cancer With Liver Metastasis: A Study of the US SEER Database and a Chinese Cohort. Front Oncol 2021;11:591009 [Crossref] [PubMed]
- Lin G, Qi K, Liu B, et al. A nomogram prognostic model for large cell lung cancer: analysis from the Surveillance, Epidemiology and End Results Database. Transl Lung Cancer Res 2021;10:622-35. [Crossref] [PubMed]
- Lukovic J, Alfaraj FA, Mierzwa ML, et al. Development and validation of a clinical prediction-score model for distant metastases in major salivary gland carcinoma. Ann Oncol 2020;31:295-301. [Crossref] [PubMed]
- Ali S, Palmer FL, Yu C, et al. Postoperative nomograms predictive of survival after surgical management of malignant tumors of the major salivary glands. Ann Surg Oncol 2014;21:637-42. [Crossref] [PubMed]
- Ali S, Palmer FL, Yu C, et al. A predictive nomogram for recurrence of carcinoma of the major salivary glands. JAMA Otolaryngol Head Neck Surg 2013;139:698-705. [PubMed]
- Li Y, Ju J, Liu X, et al. Nomograms for predicting long-term overall survival and cancer-specific survival in patients with major salivary gland cancer: a population-based study. Oncotarget 2017;8:24469-82. [Crossref] [PubMed]
- Collins GS, Reitsma JB, Altman DG, et al. Transparent Reporting of a multivariable prediction model for Individual Prognosis or Diagnosis (TRIPOD): the TRIPOD statement. Ann Intern Med 2015;162:55-63. [Crossref] [PubMed]
- Tibshirani R. The lasso method for variable selection in the Cox model. Stat Med 1997;16:385-95. [Crossref] [PubMed]
- Pencina MJ, D'Agostino RB. Overall C as a measure of discrimination in survival analysis: model specific population value and confidence interval estimation. Stat Med 2004;23:2109-23. [Crossref] [PubMed]
- Huitzil-Melendez FD, Capanu M, O'Reilly EM, et al. Advanced hepatocellular carcinoma: which staging systems best predict prognosis? J Clin Oncol 2010;28:2889-95. [Crossref] [PubMed]
- Graf E, Schmoor C, Sauerbrei W, et al. Assessment and comparison of prognostic classification schemes for survival data. Stat Med 1999;18:2529-45. [Crossref] [PubMed]
- Vickers AJ, Cronin AM, Elkin EB, et al. Extensions to decision curve analysis, a novel method for evaluating diagnostic tests, prediction models and molecular markers. BMC Med Inform Decis Mak 2008;8:53. [Crossref] [PubMed]
- Pinkston JA, Cole P. Incidence rates of salivary gland tumors: results from a population-based study. Otolaryngol Head Neck Surg 1999;120:834-40. [Crossref] [PubMed]
- Spiro RH. Salivary neoplasms: overview of a 35-year experience with 2,807 patients. Head Neck Surg 1986;8:177-84. [Crossref] [PubMed]
- Dess RT, Suresh K, Zelefsky MJ, et al. Development and Validation of a Clinical Prognostic Stage Group System for Nonmetastatic Prostate Cancer Using Disease-Specific Mortality Results From the International Staging Collaboration for Cancer of the Prostate. JAMA Oncol 2020;6:1912-20. [Crossref] [PubMed]
- Kurian AW, Ward KC, Abrahamse P, et al. Time Trends in Receipt of Germline Genetic Testing and Results for Women Diagnosed With Breast Cancer or Ovarian Cancer, 2012-2019. J Clin Oncol 2021;39:1631-40. [Crossref] [PubMed]
- Sung H, Hyun N, Leach CR, et al. Association of First Primary Cancer With Risk of Subsequent Primary Cancer Among Survivors of Adult-Onset Cancers in the United States. JAMA 2020;324:2521-35. [Crossref] [PubMed]
- Spitz MR, Batsakis JG. Major salivary gland carcinoma. Descriptive epidemiology and survival of 498 patients. Arch Otolaryngol 1984;110:45-9. [Crossref] [PubMed]
- Li F, Zhu G, Wang Y, et al. A clinical analysis of 37 cases with lymphoepithelial carcinoma of the major salivary gland treated by surgical resection and postoperative radiotherapy: a single institution study. Med Oncol 2014;31:957. [Crossref] [PubMed]
- Gutschenritter T, Machiorlatti M, Vesely S, et al. Outcomes and Prognostic Factors of Resected Salivary Gland Malignancies: Examining a Single Institution's 12-year Experience. Anticancer Res 2017;37:5019-25. [PubMed]
- Taylor ZC, Kaya EA, Bunn JD, et al. Overall and cause-specific survival for mucoepidermoid carcinoma of the major salivary glands: Analysis of 2210 patients. World J Clin Oncol 2020;11:1029-44. [Crossref] [PubMed]
- Li Y, Zhao Z, Liu X, et al. Nomograms to estimate long-term overall survival and tongue cancer-specific survival of patients with tongue squamous cell carcinoma. Cancer Med 2017;6:1002-13. [Crossref] [PubMed]
- Wang F, Zhang H, Wen J, et al. Nomograms forecasting long-term overall and cancer-specific survival of patients with oral squamous cell carcinoma. Cancer Med 2018;7:943-52. [Crossref] [PubMed]
- Booth JR, Unsal AA, Tadros S, et al. Salivary Gland Cancers of the Nasopharynx: A Population-Based Analysis of 383 Cases. Otolaryngol Head Neck Surg 2019;161:442-9. [Crossref] [PubMed]
- Weng Y, Korte JE. Racial disparities in being recommended to surgery for oral and oropharyngeal cancer in the United States. Community Dent Oral Epidemiol 2012;40:80-8. [Crossref] [PubMed]
- Gennari A, Conte P, Rosso R, et al. Survival of metastatic breast carcinoma patients over a 20-year period: a retrospective analysis based on individual patient data from six consecutive studies. Cancer 2005;104:1742-50. [Crossref] [PubMed]
- Fushimi C, Tada Y, Takahashi H, et al. A prospective phase II study of combined androgen blockade in patients with androgen receptor-positive metastatic or locally advanced unresectable salivary gland carcinoma. Ann Oncol 2018;29:979-84. [Crossref] [PubMed]
- Locati LD, Galbiati D, Calareso G, et al. Patients with adenoid cystic carcinomas of the salivary glands treated with lenvatinib: Activity and quality of life. Cancer 2020;126:1888-94. [Crossref] [PubMed]
- Hay A, Migliacci J, Zanoni DK, et al. Validation of nomograms for overall survival, cancer-specific survival, and recurrence in carcinoma of the major salivary glands. Head Neck 2018;40:1008-15. [Crossref] [PubMed]
- Uijen MJM, Lassche G, van Engen-van Grunsven ACH, et al. Systemic therapy in the management of recurrent or metastatic salivary duct carcinoma: A systematic review. Cancer Treat Rev 2020;89:102069 [Crossref] [PubMed]