Selection of high-producing clones by a relative titer predictive model using image analysis
Introduction
The commercial success of monoclonal antibodies (Mabs) has made biological therapeutics attractive to pharmaceutical companies (1). Biopharmaceutical companies prioritize the acquisition and development of cell lines that enable them to manufacture biologics quickly, consistently, and economically (2-8). The improvement of cell line development techniques have been focused on dilution based plating, robotic high throughput methods, fluorescence-activated cell sorting (FACS), and so on, and clone selection methods followed by detailed characterization of subclones (3,7,9-13). Clone selection is a critical process for cell line development (14); however, the traditional clone selection process needs to evaluate large numbers of clones by using cell growth rate, cell densities and antibody titer, and product quality (6,8,11,15,16). Due to the large number of clones that require evaluation, clones selection of cell line development is a lengthy, labor-intensive screening process (6,8,11,15,16). Clone selection strategies, omic-profiling, such as genomic, proteomic, metabolic studies, and so on, have been developed to evaluate the molecular phenotypes underlying productivity in Chinese hamster ovaries (CHO) in attempts to improve the efficiency of the clone selection process by predicting the cellular attributes of productivity (16-19). Omic-profiling in CHO for clone selection has been not widely adopted mainly on account of omic studies generally being time consuming and labor intensive (19,20). Moreover, these omic-profiling studies usually require additional equipment and experiments (3,16,17,19,21) that further hinder their use in facilitating clone selection.
Recent studies have shown that change of metabolic profile manifests in change of morphology of the CHO cells such as size, circularity, solidity, and so on (22-24). Moreover, the average size of CHO cells was different during the cell culture media selection process (22), and the circuity was used as one of the parameters for clone selection (12). Confocal Raman microscopy has recently been used as a potentially viable and non-invasive method to identify high producing cells during clone selection (25). Therefore, we hypothesized that microscope images obtained during clone selection experiments could contain valuable information to facilitate the clone selection process during cell line development.
To test this hypothesis, we extracted quantitative information as predictors from microscope images during the cell line development process and developed a prediction model using these predictors. The prediction of the relative titer (RT) of a clone during the clone selection process was used as a proof-of-concept study. This RT prediction model was based on the soft independent modeling of class analogy (SIMCA) method. The SIMCA modeling provides a useful classification of variables in graphical data by reducing dimensions of graphical variables by principal component analysis (PCA) (26-29). A SIMCA model consists of a collection of independent PCA models and datasets extracted from images that were able to be used for pattern recognition and other statistical analyses. The SIMCA method has previously been utilized in numerous CHO cell culture applications (9,30-32). It is an excellent modeling method to extract information from a set of numeric variables and interpret these variables into a meaningful and understandable predictive model (28). Here, we present the first RT predictive model of CHO clone selection based on the image analysis during clone selection process. We present the following article in accordance with the MDAR reporting checklist (available at https://dx.doi.org/10.21037/atm-21-2822).
Methods
Passage of host CHO cell culture
Suspension culture CHO-K1 cell line [European Collection of Authenticated Cell Culture (ECACC, UK)] was purchased from Public Health England (Harlow, UK). Cells were directly suspended in QUACELL CD04 media. The CHO-K1Q (Quacell, CHINA) cell line was purchased from Quacell Biotech, and the CHO-S (Gibco, Grand Island, NY, USA) cell line was purchased from Thermo Scientific (Waltham, MA, USA). All 3 host CHO cell lines were adapted by passaging the cells to a viable cell density (VCD) of 0.5×106 cells/mL, when the VCD reached 3×106 cells/mL. All cells were only selected if there is no aggregate in the suspended cell culture.
Electroporation
As previously described (33), the vector containing the appropriate expression promoter and the gene of interest were transformed into Esherichia coli (Takara, Mountain View, CA, USA) for expansion and then the plasmid DNA were isolated using endotoxin-free kits (Axygen, Union City, CA, USA). High-quality DNA was characterized as having an optical density (OD)260/280 ratio between 1.88 and 1.92, an OD260/230 ratio of 2.1–2.2, and a concentration above 0.5 mg·mL−1. Cell growth CD04 media (Quacell, Zhongshan, Guangdong, China) was used to perform at least 5 passages from thawing. As soon as cell density reached 3×106 cells/mL (log phase) before electroporation, the cell suspension was centrifuged at 200 ×g for 5 min at room temperature, followed by washing cells using cell pellet with electroporation solution (Bio-Rad, Hercules, CA, USA). The cells were then resuspended to final cell concentration of 20×106 cells·mL−1 before electroporation by an electroporator (Bio-Rad, USA). Cells were incubated for 7 days before single cell cloning (SCC) experiments.
Cloning selection experiments
The SCC experiments were performed as previously described (11,16) with some modifications. Briefly, 100 µL of different compositions of SCC medium were added to each well in a 96-well plate except for the A1 well. A total of 200 µL of cell suspension was added to the A1 well. Then, 100 µL of cell suspension from A1 was quickly transferred into B1. This process was repeated to dilute the cells in the first column from A1 to the H1 well. After this dilution, 100 µL of culture medium was added into each well in the first column to bring the final volume to 200 µL. A total of 100 µL of cell suspension from first column was transferred into the second column. The process was repeated to dilute column by column until the volume of the twelfth column had increased to 200 µL. The 96 well plates were incubated at 37 °C with 5% CO2 in a humidified incubator (Thermo Scientific, USA). The plates were scanned with a Cell-Metric Imager (Solentim, Dorset, UK) to identify single cell clones at 0, 24, 48, and 96 h. Proliferated single cells were identified by Cell-Metric Imager at 96 h. Selected clones were expanded sequentially to 24-well, 6-well plates and TubeSpin bioreactors (TPP, Trasadingen, Switzerland) in the CD004 Media (Quacell, China). The clones were selected for cryopreservation and further evaluation. Selection of the CHO clones with the phenotye of high productivity and fast growth rate were used as the directed evolution method.
Clone expansion and model evaluation
Fed-batch evaluations were performed in TubeSpin bioreactors (TPP, Switzerland). Individual tubes were set up with a working volume of 30 mL of production media, incubated at 37 °C, 5% CO2, 85% relative humidity, and shaken at 225 rpm at 50 mm orbital diameter in a ISF4-X incubator (Kuhner, Birsfelden, Switzerland). Cultures were inoculated at a target cell density ranging from 8×105 to 1×106 cells/mL and fed a single bolus Feed02 (Quacell, China) at days 3, 6, and 9. In-process samples were taken from cultures on days 3, 5, 7, 9, 11, 13, and 14 for cell image analysis and cell count by Bio-Rad TC-1000 (Bio-Rad, USA). Antibody titers were measured by affinity Protein A high-performance liquid chromatography (Agilent Technologies, Inc., Santa Clara, CA, USA).
Titer and protein quality assessment
Antibody titers were measured by the affinity Protein A chromatography in a high performance liquid chromatography (HPLC) (Agilent, USA) system equipped with a quaternary pump, solvent degasser, column oven, and variable-wavelength UV detector. A POROS A/20 of 100 mm × 4.6 mm (Thermo Scientific, USA) column was used with the column temperature set at 25 °C. There were 3 mobile phases (A: 0.05 M phosphate buffer, 0.02% sodium azide, pH 7.5 for equilibration of the column; B: 0.25 M glycine, pH 2.5 for product elution; C: 0.25 M glycine, pH 6.1) used with the flow rate at 2 mL/min for washing of impurities before product elution. The injection volume of the samples and curve calibration was 50 µL. The final profile was obtained after subtracting the profile of a blank to assist in the integration of the baseline. Ion exchange chromatography (IEC) of the protein was analyzed on an HPLC system (Agilent, USA) after protein A purification. A MAbPac SCX-10 4 mm × 250 mm column (Thermo, USA) was equilibrated at 25 °C. The mobile phases consisting of 20 mM Na2HPO4 (Buffer A) and 20 mM of Na2HPO4 with 250 mM of NaCl (Buffer B) at pH 7 were operated at 0.7 mL/min. After an isocratic elution at 20% of Buffer B for 5 min, a linear gradient was applied from 20% to 50% of B in 40 min. The column was then washed for 5 min at 70% of B and further equilibrated for 25 min at 20% of B. Elution was monitored by UV absorbance at 280 nm. The acidic peaks, basic peaks, and main IEC peaks were obtained after subtraction of a blank to assist in the integration of the baseline. The main peak percentage was calculated by the main IEC peak divided by all the peaks.
The same HPLC system (Agilent, USA) was used for all size exclusion chromatography (SEC) measurements. An injection volume of 20 µL into A TSKgel G2000SWXL column (Tosoh Bioscience, Griesheim, Germany; 7.8 mm i.d. ×30 cm, 5 µm particle size, 125 Å pore size) with a flow rate of 0.8 mL/min at a column temperature of 25 °C. For the mobile phase, stock solutions of 0.05 M of ammonium salt were prepared using ultrapure water and filtered over Whatman Ltd. (Maidstone, United Kingdom) regenerated cellulose membrane filters (0.45 µm). Adjustment of the solution’s pH was performed after filtration. Equilibration of the column with the respective mobile phase was performed for at least 5 column volumes prior to protein injection. The low molecular weight peaks, high molecular weight peaks, and main SEC peaks were obtained after subtraction of a blank to assist in the integration of the baseline. The main SEC peak percentage was calculated by the main SEC peak divided by all the peaks.
Image acquisition
Images of cells were obtained on a TC-1000 Cell Counter (Bio-Rad, USA) and quantified using the Fiji software package in ImageJ (https://imagej.net/Fiji) (34).
Statistical analysis
Image analysis, data analysis, data visualization, and predictive modeling were performed using SIMCA P14 Trial Version (Sartorious, Goettingen, Germany) and Python (3.7.3) (35) with libraries Numpy (v1.16.5), Pandas (v0.25.1), Matplotlib (v3.1.1), Seaborn (v0.9.0), and Scipy (v1.3.1).
Results
Database generation
A total of 45 K1Q Clones expressing Mab A (K1Q-Mab A clones) were successfully expanded and cryopreserved. These clones were evaluated by their growth curves (Figure 1). The maximum cell densities were between 5.98×106 and 15.89×106 cells per mL. Most of the clones had titer between 4 and 7 g/L, indicating that these clones are relevant to the titer in most industrial cases (3,7,36,37). Since the volumetric productivities were different among different molecules, RT was used to generate the predictive model for clone selection. The RT were calculated by the ratio of the clonal volumetric productivity to the average volumetric productivity of all 45 clones. The RT were found between 0.35 and 1.97 for K1Q-Mab A expressing clones with an average RT of 1.09, and standard deviation (SD) at 0.35 (Figure 2). A total of 5 clones with RT above 1.66 were considered extremely high producers as they were 3 SD away from the average. The RT range of clones below 0.43, and between 1.44 and 1.66 were considered low producers and high producers, respectively, since they were both 2 SD away from the average. The classification of the 45 K1Q Mab A expressing clones are shown in Figure 3.
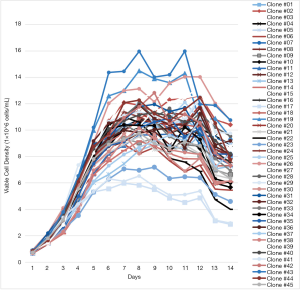
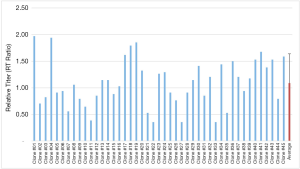
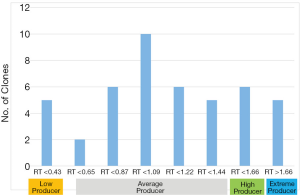
Predictors identification
Day 3 cell counting images by TC1000 were selected for image analysis as they were in the early stage of culture while enough generations had passaged for statistical significance. The representative images from day 3 cell counting images of a low producer (Figure 4A), medium producer (Figure 4B), high producer (Figure 4C), and an extreme producer (Figure 4D) are shown in Figure 4. The quantified data extracted from the image analysis is displayed in Table 1. Quantitative image analysis (QIA) is a range of techniques for extracting objective, quantitative information from microscopy, spectroscopy, and chromatography images (38-40). The main steps of QIA are image capturing, image storage, correcting imaging defects, image enhancement, segmentation of objects in the image, and image quantification (41-43). It has long been used by medical professionals and researchers for omics and pathological studies (19,21,44,45). The QIA of microscopy have been recently been applied to extract information from microscope-generated images (28,38,40,46). Here, we generated the images (Figure 5) using TC-1000 cell counter. The qualification of these images was performed by converting the image signal into numerical information using the Fiji software package. The numerical information consisted of the grey scale values that described the brightness of every pixel within the image of the cell population. The background noise of the images was optimized by discrimination between different cells in an image and the uniformity of illumination in the whole image. Image enhancement, reduction of background noise, extraction of edges, identification of points, strengthening texture elements, and improving contrast were used to ensure more accurate quantification of cell density, cell shape, and cell size. The summary of the imaging quantitative information (IQI) extracted from QIA by Fiji software is presented in Table 1.
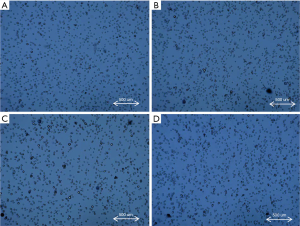
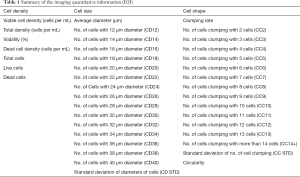
Full table
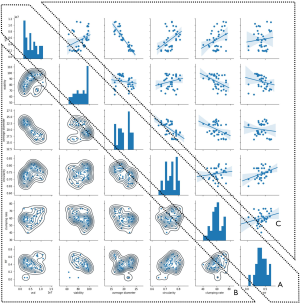
The IQI was then qualified as a predictor for RT using Python software (35). The IQIs, such as VCD, viability, average diameter, circularity, clumping rate, and RT were calculated to generate a pair-plot (Figure 5) with the pair-plot function in the Seaborn library in Python. The diagonal axis represents the distribution of the IQIs (Figure 5A); it should be noted that the data for VCD and viability was poorly distributed. This was due to the use of day 3 data cells which were still in log phase, where most of the cell density was close to 2−4×106, and viability was mostly above 95% (Figure 5A). The lower triangle represents the kernel density estimation (KDE) (kdeplot with default parameters, Seaborn) of the respective IQIs on the x-axis and y-axis (Figure 5B). The KDE plot showed the estimated probability of IQIs on the y-axis as the density function of the variables on the x-axis. For example, when the average VCD was at 0.5×107, the highest probability for RT was 0.4 (Figure 5B). However, it should be noted that KDE is limited to estimation of the density function for homogenous cells (47). Therefore, KDE probability function needed to be coupled with regression model estimation to ensure the data was accurately correlated (47,48). The upper triangle represented the regression model estimation (regplot with default parameters, Seaborn) of the respective variables on x-axis and y-axis (Figure 5C). The deep slope of the regression plot indicated that there was a correlation among the IQIs (Figure 5A). The regression model indicated that RT showed little correlation with the IQIs (Figure 5C, last column). However, correlations were identified with some of the IQIs, such as the correlation of average diameter and VCD (Figure 5B). These correlations indicated that interactions existed among these variables.
To further investigate interactions among IQI and their relation to RT, all IQIs were used as input to calculate a correlation matrix with the corr function (default parameters) in Pandas of Python. The resulting heatmap based on this correlation matrix is displayed in Figure 6 with the heatmap function (default parameters) of Seaborn. The red color in the correlation matrix represents positive correlation coefficient and blue color represents negative ones. Correlation matrix data visualization with the right tools facilitates data interpretation from multiple dimensions. For example, Figure 5 shows that diameter had 2 clusters centering at about CD17 and CD23, which could potentially reveal characteristics of this cell line and possible predictor for RT. The circularity was negatively correlated with average diameter, which could potentially indicate that these 45 clones were actively undergoing cell division. The correlation matrix in Figure 4 shows that RT had the strongest correlations with IQIs that were related to cell shape (CC04, Pearson Correlation Coefficient, PCC =0.30) and cell size (CD12, PCC =0.32). Moreover, these IQIs showed a strong correlation data in the heat maps, indicating that the IQIs relevant to cell shape and cell size should be considered as predictors for our predictive models. Consistent with the KDE estimation and distribution observation in Figure 4, the cell density related IQIs such cell density and viability were not able to reveal a strong correlation cluster in the correlation matrix. Therefore, these cell density-related IQIs were not used as predictors to construct our predictive model.
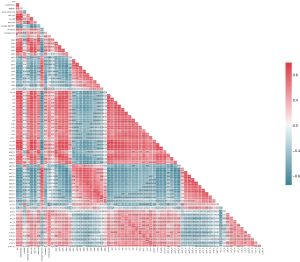
Model construction
The SIMCA method was used to build an RT predictive model that can associate the titer with the predictors and interactions of the predictors. It is a supervised statistical classification technique that is based on PCA (26,30), and it is particularly powerful for building multiple class models for prediction (49). Using SIMCA for image analysis data has previously been described and elaborated on in several papers (50-52). Here, we used our image data from the 45 clones as the training data set to build a predictive model with R2=0.75, and Q2=0.48 (Figure 7). This RT predictive model was based on an orthogonal projection to latent structures (OPLS) modeling. The OPLS model was an extension of the PLS model that separated the predictors in Table 1 into 2 categories of X variables: the first category was linearly related to titer while the second category was orthogonal to titer. Hence, the linearly related X variables are modeled by the predictive components, while orthogonal X variables are modeled by the orthogonal components. This partitioning of the X data provides improved model transparency and interpretability (53). The visualization of the predictive components was labelled as t1, t2, and so on, and the orthogonal components had a subscript o, for example, to 1 for the first orthogonal component in X variables (Figure 8). The scores of the scatter plot of the RT predictive model are shown in Figure 8, where the predictors listed in Table 1 were summarized as scores t[1] and t0[1]. This RT model scatter plot of scores is a visual representation for the model space of the predictors in Table 1, and it illustrates how these predictors are situated with respect to each other. The scatter plot shows the presence of 3 outliers (clone #04, #07, and #17), and no specific groups or patterns were presented in the data from the 45 clones. The Hotelling’s T2 plot (Figure 9) summarizes the clone from all the predictors listed in Table 1. The T2 range in the y-axis represents how far away the combinations of all the predictors of a clone are from the OPLS model (Figure 9). The Hotelling’s T2 plot showed that the T2 range of 3 clones (clone #04, #07, and #17) were greater than the 95% critical value (8.89), indicating that these clones were far away from the RT predictive model. Hence, the model was unable to accurately predict the RT of these clones. Nevertheless, the outliers were not eliminated during adjustment of the RT prediction model for calibration purposes when more data was added. Together with scattering score plots, this data indicated that the outliers emerged during construction of the predictive model and thus a limitation of the predictive models became apparent, even though the RT predictive model had a goodness of fit for most of the clones.
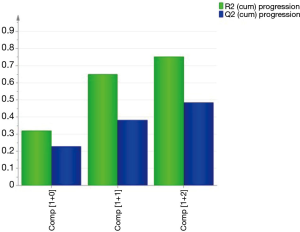
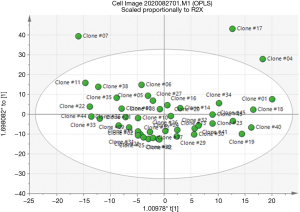
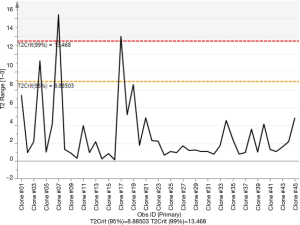
Model performance evaluation and model validation
The overall model performance of our RT predictive model was evaluated by the difference between the predicted RT and the actual RT. These differences are related to the concept of “goodness of fit” of a model, with better models having higher correlation between predicted and observed outcomes (54,55). Therefore, new datasets were used to evaluate the “goodness of fit” of our RT predictive model. To assess the goodness of fit of our RT predictive model, 5 clones were used to test the predicted RT against the actual RT (Figure 10). The predictive data were generated by inserting the predictors into the RT predictive model, and the predicted RT and actual RT are listed in Table 2. The growth curve and the correlation plot between the predictive and actual RT are shown in Figure 10.
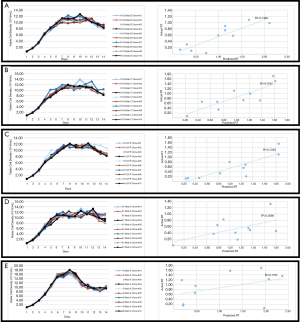
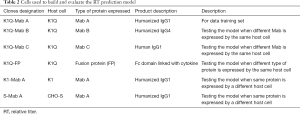
Full table
Using the RT predictive model to predict relative predictivity of CHO-K1Q clones that were expressing Mab B (Figure10A) and Mab C (Figure 10B) provided a good accuracy (r2=0.74, 0.70 for K1Q-Mab B and K1Q-Mab C, respectively). Since the RT predictive model was based on data from K1Q host cells clones that were expressing Mab A, a K1Q clone expressing a fusion protein was used to test the predictivity when a different type of molecule was being expressed (Figure 10C). The correlation of the predicted RT to the actual RT for K1Q FP expressing clones was 0.72. The r2 was similar to that of the K1Q Mab expressing clone. Therefore, our OPLS model was shown to have the ability to distinguish a low producer from high producer (Figure 10) when CHO-K1Q was used as host cell.
To evaluate the goodness of fit of the RT predictive model among other host cells, we correlated the predicted RT obtained from predictive model to the actual RT of the CHO-K1 Mab A expressing clones, as well as that of the CHO-S Mab A expressing clones. The correlation of CHO-K1 Mab A clones and the CHO-S Mab A clones to their predictive titer was 0.31 and 0.17, respectively (Figure 10D,E). The K1Q host cell was a sub-clone of a K1 host cell line. Therefore, it is surprising that the current RT predictive model was not able to provide a good prediction of the RT of CHO-K1 Mab A clones (r2=31) (Figure 10D). It has been reported that sub-cloning of CHO cells can lead to diversity of size, shape, and cell surface glycan content (56); therefore, it is reasonable to speculate that the changes in size and shape of the CHO-K1 cells affected the predictors, and thus led to a poor prediction of RT. Similarly, CHO-S also showed a poor goodness of fit for the actual RT when compared to the predicted RT generated by the RT predictive model (r2=0.17). The RT predictive model were not able to distinguish a high producer from a low producer (Figure 10E). The poor prediction of the model on CHO-S cells was expected because of the significant genomic and metabolic difference among different host cells (20). Moreover, the current model was used to test its application for protein quality prediction. The main SEC and IEC peaks were measured and reported in Figure 11A,B, respectively. A model with statistical significance was not able to be generated. The SEC predictive model had R2=0.13 and Q2=−0.17 (Figure 11C). The IEC predictive model had R2=0.22 and Q2=−0.09 (Figure 11D). Both of these predictive models will need more training data to build a predictive model for protein quality. These studies are currently in progress.
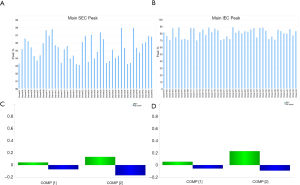
Discussion
In our current study, an RT prediction model was generated by the quantitative information extracted from microscopic images during a cell line development process. The performance of this RT prediction model was further evaluated by 50 clones engineered from 5 different host cells. While there is a caveat of the current RT prediction model, that is, inaccurate prediction when different host cell prediction is used, this RT prediction model served as a proof of concept study that quantitative information from cell line development images provides valuable information to facilitate the cell line development process. Moreover, it is speculative that the current RT model can be adapted to directly to evaluate the early productivity and product quality of complex multi-subunit vaccine antigens during the development of Chinese hamster ovary cell lines by using HPLC and protein interactions data to build the RT model specifically. Similarly, improved viability and capacity of single cell clones could also be selected using such predictive model. As big data, deep learning, artificial intelligence, and other computational techniques have recently been applied to bioprocess development and biological data analysis (44,57-61), using data science to facilitate the efficiency of bioprocess development seems inevitable. Here, we have presented the first predictive model that can be used to estimate the relative productivity of CHO clones during cell line development. This current RT prediction model requires more clonal data and images to improve its prediction accuracy. Moreover, images from SCC experiments for prediction will also provide earlier prediction during cell line engineering. The application of imaging flow cytometry to characterize recombinant cell lines at the single-cell level can potentially predict even earlier. These experiments are currently in process. Nevertheless, our current study will serve as a foundation for more predication models for cell line developments to facilitate the selection of the clones using quantitative image analysis. By predicting the high producers, this prediction model provide investigator a higher probability to select high producing clones, and the earlier indication by the model can minimize the duration of the selection process.
Acknowledgments
Funding: This work was financially supported by the National Key Research and Development Program of China (2017YFC0909002).
Footnote
Reporting Checklist: The authors have completed the MDAR reporting checklist. Available at https://dx.doi.org/10.21037/atm-21-2822
Data Sharing Statement: Available at https://dx.doi.org/10.21037/atm-21-2822
Conflicts of Interest: All authors have completed the ICMJE uniform disclosure form (available at https://dx.doi.org/10.21037/atm-21-2822). The authors have no conflicts of interest to declare.
Ethical Statement: The authors are accountable for all aspects of the work in ensuring that questions related to the accuracy or integrity of any part of the work are appropriately investigated and resolved. This article does not contain any studies with human participants performed by any of the authors.
Open Access Statement: This is an Open Access article distributed in accordance with the Creative Commons Attribution-NonCommercial-NoDerivs 4.0 International License (CC BY-NC-ND 4.0), which permits the non-commercial replication and distribution of the article with the strict proviso that no changes or edits are made and the original work is properly cited (including links to both the formal publication through the relevant DOI and the license). See: https://creativecommons.org/licenses/by-nc-nd/4.0/.
References
- Ledford H. 'Biosimilar' drugs poised to penetrate market. Nature 2010;468:18-9. [Crossref] [PubMed]
- Orlova NA, Kovnir SV, Vorobiev II, et al. Stable Expression of Recombinant Factor VIII in CHO Cells Using Methotrexate-Driven Transgene Amplification. Acta Naturae 2012;4:93-100. [Crossref] [PubMed]
- Le H, Vishwanathan N, Jacob NM, et al. Cell line development for biomanufacturing processes: recent advances and an outlook. Biotechnol Lett 2015;37:1553-64. [Crossref] [PubMed]
- Hurst S, Ryan AM, Ng CK, et al. Comparative nonclinical assessments of the proposed biosimilar PF-05280014 and trastuzumab (Herceptin BioDrugs 2014;28:451-9. [Crossref] [PubMed]
- Tirelli U, Carbone A, Di Francia R, et al. A new peg-filgrastim biosimilar, mecapegfilgrastim for primary prophylaxis of chemotherapy-related neutropenia is now available. Ann Transl Med 2020;8:166. [Crossref] [PubMed]
- Hou JJ, Hughes BS, Smede M, et al. High-throughput ClonePix FL analysis of mAb-expressing clones using the UCOE expression system. N Biotechnol 2014;31:214-20. [Crossref] [PubMed]
- Yuan J, Xu W, Yu W, et al. Cell Culture Technologies in Successful Biosimilar Development. Bioequivalence Bioavailab Int J 2018;2:1-7.
- Xu W, Yu X, Zhang J, et al. Soy hydrolysate mimic autocrine growth factors effect of conditioned media to promote single CHO-K1 cell proliferation. Tissue Cell 2019;58:130-3. [Crossref] [PubMed]
- Le H, Kabbur S, Pollastrini L, et al. Multivariate analysis of cell culture bioprocess data--lactate consumption as process indicator. J Biotechnol 2012;162:210-23. [Crossref] [PubMed]
- Min Z, Lawshe A, Koskie K, et al. Rapid Development and Optimization of Cell Culture Media. Biopharm Int 2008;21:60-8.
- Dhulipala P, Liu M, Beatty S, et al. Differential cell culture media for single-cell cloning. Bioprocess Int 2011;9:44-51.
- Riba J, Schoendube J, Zimmermann S, et al. Single-cell dispensing and 'real-time' cell classification using convolutional neural networks for higher efficiency in single-cell cloning. Sci Rep 2020;10:1193. [Crossref] [PubMed]
- Mattanovich D, Borth N. Applications of cell sorting in biotechnology. Microb Cell Fact 2006;5:12. [Crossref] [PubMed]
- Yuan J, Xu W, Jing W, et al. Drugability Studies are Keys to the Successful Commercialization of Biotherapeutics. Biomed Pharmacol J 2017;10:1593-601. [Crossref]
- Pak SC, Hunt SM, Bridges MW, et al. Super-CHO-A cell line capable of autocrine growth under fully defined protein-free conditions. Cytotechnology 1996;22:139-46. [Crossref] [PubMed]
- Lim UM, Yap MG, Lim YP, et al. Identification of autocrine growth factors secreted by CHO cells for applications in single-cell cloning media. J Proteome Res 2013;12:3496-510. [Crossref] [PubMed]
- Griffin TJ, Seth G, Xie H, et al. Advancing mammalian cell culture engineering using genome-scale technologies. Trends Biotechnol 2007;25:401-8. [Crossref] [PubMed]
- Lattenmayer C, Trummer E, Schriebl K, et al. Characterisation of recombinant CHO cell lines by investigation of protein productivities and genetic parameters. J Biotechnol 2007;128:716-25. [Crossref] [PubMed]
- Stolfa G, Smonskey MT, Boniface R, et al. CHO-Omics Review: The Impact of Current and Emerging Technologies on Chinese Hamster Ovary Based Bioproduction. Biotechnol J 2018;13:e1700227 [Crossref] [PubMed]
- Lakshmanan M, Kok YJ, Lee AP, et al. Multi-omics profiling of CHO parental hosts reveals cell line-specific variations in bioprocessing traits. Biotechnol Bioeng 2019;116:2117-29. [Crossref] [PubMed]
- Poon F, Mathura VS. Introduction to proteomics. Bioinformatics: A Concept-Based Introduction. 2009:107-13.
- Kiehl TR, Shen D, Khattak SF, et al. Observations of cell size dynamics under osmotic stress. Cytometry A 2011;79:560-9. [Crossref] [PubMed]
- Pan X, Dalm C, Wijffels RH, et al. Metabolic characterization of a CHO cell size increase phase in fed-batch cultures. Appl Microbiol Biotechnol 2017;101:8101-13. [Crossref] [PubMed]
- Fomina-Yadlin D, Du Z, McGrew JT. Gene expression measurements normalized to cell number reveal large scale differences due to cell size changes, transcriptional amplification and transcriptional repression in CHO cells. J Biotechnol 2014;189:58-69. [Crossref] [PubMed]
- Klein K, Gigler AM, Aschenbrenner T, et al. Label-free live-cell imaging with confocal Raman microscopy. Biophys J 2012;102:360-8. [Crossref] [PubMed]
- Vanden Branden K. 1 Karlien, Hubert, M. Robust classification in high dimensions based on the SIMCA Method. Chemometrics and Intelligent Laboratory Systems 2005;79:10-21. [Crossref]
- Yang IC, Tsai CY. Integration of SIMCA and near-infrared spectroscopy for rapid and precise identification of herbal medicines. J Food Drug Anal 2013;21:268-278. [Crossref]
- Bonnet N. Multivariate statistical methods for the analysis of microscope image series: Applications in materials science. Journal of Microscopy 1998;190:2. [Crossref]
- Wold S. Pattern recognition by means of disjoint principal components models. Pattern Recognit 1976;8:127-39. [Crossref]
- Mercier SM, Diepenbroek B, Dalm MC, et al. Multivariate data analysis as a PAT tool for early bioprocess development data. J Biotechnol 2013;167:262-70. [Crossref] [PubMed]
- Yuan J, Gang H, Wang J, et al. Scientific Strategy in China: Starting with Biosimilar Platforms. Advances in Biopharmaceutical Technology in China, 2nd ed 2018;51–64.
- Poon HF, Wu F, Shen L, et al. Quality by Design (QbD) Concept: A Potential Solution to Chinese Current Biomanufacturing Challenges. Biomedical and Pharmacology Journal 2019;12:499-502. [Crossref]
- A Longo P, Kavran JM, Kim MS, et al. Generating mammalian stable cell lines by electroporation. Methods Enzymol 2013;529:209-26.
- Schindelin J, Arganda-Carreras I, Frise E, et al. Fiji: an open-source platform for biological-image analysis. Nat Methods 2012;9:676-82. [Crossref] [PubMed]
- Pilnenskiy N, Smetannikov I. Feature Selection Algorithms as One of the Python Data Analytical Tools. Future Internet 2020;12:54. [Crossref]
- Lu F, Toh PC, Burnett I, et al. Automated dynamic fed-batch process and media optimization for high productivity cell culture process development. Biotechnol Bioeng 2013;110:191-205. [Crossref] [PubMed]
- Wurm FM. Production of recombinant protein therapeutics in cultivated mammalian cells. Nat Biotechnol 2004;22:1393-8. [Crossref] [PubMed]
- Zeitvogel F, Schmid G, Hao L, et al. ScatterJ: An ImageJ plugin for the evaluation of analytical microscopy datasets. J Microsc 2016;261:148-56. [Crossref] [PubMed]
- Randall NX. The current state-of-the-art in scratch testing of coated systems. Surface and Coatings Technology 2019;380:125092 [Crossref]
- Zhou W, Wang ZL. Scanning Microscopy for Nanotechnology. Scanning Transmission Electron Microscopy for Nanostructure Characterization 2007:152-91.
- Oberholzer M, Ostreicher M, Christen H, et al. Methods in quantitative image analysis. Histochem Cell Biol 1996;105:333-55. [Crossref] [PubMed]
- Abràmoff MD, Garvin MK, Sonka M. Retinal imaging and image analysis. IEEE Rev Biomed Eng 2010;3:169-208. [Crossref] [PubMed]
- Poon HF, Vaishnav RA, Getchell TV, et al. Quantitative proteomics analysis of differential protein expression and oxidative modification of specific proteins in the brains of old mice. Neurobiol Aging 2006;27:1010-9. [Crossref] [PubMed]
- Madabhushi A, Lee G. Image analysis and machine learning in digital pathology: Challenges and opportunities. Med Image Anal 2016;33:170-5. [Crossref] [PubMed]
- Hamilton PW, Bankhead P, Wang Y, et al. Digital pathology and image analysis in tissue biomarker research. Methods 2014;70:59-73. [Crossref] [PubMed]
- Passot S, Fonseca F, Alarcon-Lorca M, et al. Physical characterisation of formulations for the development of two stable freeze-dried proteins during both dried and liquid storage. Eur J Pharm Biopharm 2005;60:335-48. [Crossref] [PubMed]
- Ruan D. Kernel density estimation-based real-time prediction for respiratory motion. Phys Med Biol 2010;55:1311-26. [Crossref] [PubMed]
- Chwialkowski K, Strathmann H, Gretton A. A Kernel Test of Goodness of Fit. International Conference on Machine Learning; 2016.
- Tsugawa H, Tsujimoto Y, Arita M, et al. GC/MS based metabolomics: development of a data mining system for metabolite identification by using soft independent modeling of class analogy (SIMCA). BMC Bioinformatics 2011;12:131. [Crossref] [PubMed]
- Wold JP, Westad F, Heia K. Detection of parasites in cod fillets by using SIMCA classification in multispectral images in the visible and NIR region. Appl Spectrosc 2001;55:1025-34. [Crossref]
- Esbensen KH, Geladi P. Principal Component Analysis: Concept, Geometrical Interpretation, Mathematical Background, Algorithms, History, Practice. Comprehensive Chemometrics 2009;2:211-26. [Crossref]
- Adams F, Butaye L, Janssens G, et al. Materials characterization with 3-Dimensional ion microscopy/microprobe analysis. Anal Sci 1991;7:383-8. [Crossref]
- Sanchez DH, Schwabe F, Erban A, et al. Comparative metabolomics of drought acclimation in model and forage legumes. Plant Cell Environ 2012;35:136-49. [Crossref] [PubMed]
- Steyerberg EW, Vickers AJ, Cook NR, et al. Assessing the performance of prediction models: a framework for traditional and novel measures. Epidemiology 2010;21:128-38. [Crossref] [PubMed]
- Shipe ME, Deppen SA, Farjah F, et al. Developing prediction models for clinical use using logistic regression: an overview. J Thorac Dis 2019;11:S574-84. [Crossref] [PubMed]
- Davies SL, Lovelady CS, Grainger RK, et al. Functional heterogeneity and heritability in CHO cell populations. Biotechnol Bioeng 2013;110:260-74. [Crossref] [PubMed]
- Aynsley M, Hofland A, Morris AJ, et al. Artificial intelligence and the supervision of bioprocesses (real-time knowledge-based systems and neural networks). Adv Biochem Eng Biotechnol 1993;48:1-27. [Crossref] [PubMed]
- Mahmud M, Kaiser MS, Hussain A, et al. Applications of Deep Learning and Reinforcement Learning to Biological Data. IEEE Trans Neural Netw Learn Syst 2018;29:2063-79. [Crossref] [PubMed]
- Chen CL, Mahjoubfar A, Tai LC, et al. Deep Learning in Label-free Cell Classification. Sci Rep 2016;6:21471. [Crossref] [PubMed]
- Kennedy PJ, Oliveira C, Granja PL, et al. Monoclonal antibodies: technologies for early discovery and engineering. Crit Rev Biotechnol 2018;38:394-408. [Crossref] [PubMed]
- Swan M. The Quantified Self: Fundamental Disruption in Big Data Science and Biological Discovery. Big Data 2013;1:85-99. [Crossref] [PubMed]
(English Language Editor: J. Jones)