Dipetidyl peptidase-4 and transferrin receptor serve as prognostic biomarkers for acute myeloid leukemia
Introduction
Acute myeloid leukemia (AML) is characterized by a loss of control of myeloid precursor cell proliferation and undifferentiation (1). If AML patients do not undergo appropriate treatment, death can rapidly occur. Anthracycline and cytarabine have remained the standard therapy regimens for AML patients since the 1970s (2). Despite advances in diagnostic and therapeutic methods, the overall survival (OS) of AML patients has not significantly improved. Over the past decade, with the introduction of targeted therapy agents combined with traditional chemotherapy, the rates of complete remission (CR) have been improved, but the rate of relapse is still unchanged. Relapse of disease remains an obstacle for lengthening the OS of AML patients. For high-risk patients, the rate of disease relapse is more than 60% and results in a short median disease-free survival (DFS) of less than 1 year (range, 4 to 11 months) (3). To date, several driver mutations have been observed in AML patients, and these mutations have deep influence on the prognosis of AML patients. Kishtagari et al. study summarizes the driver mutations in AML, based on the functions of driver genes, they are divided into signal transduction (FLT3, NRAS, KRAS, and KIT), splicing mutations (SF3B1, ZRSR2, U2AF1, and SRSF2), tumor suppressors (TP53, WT1, and TET2), AML licensing mutations (NMP1), epigenetic modifiers (IDH1, IDH2, TET2, SRSF2, BCOR, BCORL, TET2, ASXL1, and EHZ2), transcription factors (RUNX1, CEBPA, and GATA2), and chromatin modifiers (Cohesin, ASXL1, and EHZ2) (4). Patients with the mutated NMP1, RUNX1, and TP53 lead to poor prognosis, but biallelic mutated CEBPA indicate favorable prognosis (5). Several studies have also demonstrated the occurrence of targeted therapy resistance (6,7). The target regimens enasidenib and ivosidenib have been used to treat IDH mutated AML patients (8). Sorafenib was used to therapy the with FLT3-ITD mutated AML patients (9). However, the resistance of these target therapy has been found (6,10). These indicated that some unique mutation can be sever as the diagnostic and prognostic biomarkers for AML patients, as well as assessing the drug resistance, relapse risk, and therapy targets markers. Drug resistance and disease relapse may be the main reasons leading to the poor outcomes of AML patients, but the underlying mechanisms are still unclear. It is therefore important to find novel biomarkers for diagnosis, assessing prognosis, monitoring drug resistance, and even supplementary therapy methods for AML patients.
Iron is a fundamental inorganic nutrient which has a critical role in multiple biological processes such as DNA and RNA synthesis, cellular respiration, immune responses, and detoxification processes, among others (11). Ferroptosis was introduced in 2012 and is defined as a unique iron-dependent form of cell death. The features of ferroptosis include smaller mitochondria with increased membrane density, and decreased mitochondrial cristae (12). Ferroptosis strike the death balance in common cells and tissues (13). Several studies have demonstrated that ferroptosis is a significant regulator of tumor progression (14-16). Ferroptosis is regulated via several factors, and ferroptosis-related genes (FRGs) may be the most significant regulators among them. FRGs have been observed to be differentially expressed and play key roles in the prognosis of various cancer types such as pancreatic cancer, glioma, and hepatocellular carcinoma (17-20). From these findings, it is clear that FRGs have been well investigated in solid tumors. In regards to AML, several studies have explored the mechanism of drug-induced ferroptosis (21-23). Du et al.’s study indicated that DHA can inhibit leukemia cell proliferation via inducing ferroptosis (21). Furthermore, Du et al. revealed that inhibition of ferroptosis can promote ATPR-induced AML cell differentiation by regulating the ROS-autophagy-lysosomal pathway (22). Zhu et al. showed that typhaneoside inhibited leukemia cell proliferation via inducing ferroptosis-related autophagy (23). These findings indicate that inducing ferroptosis may be a novel potential anticancer method for AML. However, there have been no studies that have investigated FRG expression levels, their prognostic role, and their association with the tumor microenvironment (TME) and drug resistance in AML patients. In the present study, we used bioinformatics to analyze FRG expression levels, their prognostic role, and their association with immune infiltration and drug sensitivity. Furthermore, we collected normal samples and AML patient samples to validate the gene expression levels via immunohistochemistry, polymerase chain reaction (PCR), and next-generation sequencing (NGS). We present the following article in accordance with the REMARK reporting checklist (https://dx.doi.org/10.21037/atm-21-3368).
Methods
Raw data
The transcriptome data and clinical data of 151 AML samples from The Cancer Genome Atlas (TCGA) database and 70 bone marrow normal samples from the Genotype-Tissue Expression (GTEx) database were collected from the University of California Santa Cruz database (UCSC Xena, https://xenabrowser.net/datapages/). Subsequently, log2 (FPKM+1) normalization was performed on the transcriptome data. We searched and extracted 23 FRGs from PubMed (24-26).
Screening differentially expressed FRGs
We screened differentially expressed FRGs between the TCGA-LAML cohort (tumor) and the GTEx cohort (normal) for further analysis. Differential analysis was carried out with the Wilcoxon test in R software. A heatmap plot of differentially expressed genes was generated via the ggplot2 package. P<0.05 was considered statistically significant.
Construction of the ferroptosis-related prognostic signature
We obtained prognostic FRGs via univariate cox regression based on differential expression of FRGs, then used lasso regression to obtain a more refined signature by constructing a penalty function. Multivariate cox regression (stepwise) was used to construct the final prognostic signature. KM survival analysis was used to generate the survival curves based on median values, and log-rank P<0.05 was considered statistically significant. The receiver operating characteristic (ROC) curves, nomogram, and calibration curve of the prognostic signature were generated via the R packages survivalROC, survminer, and rms, respectively. P<0.05 was considered as statistically significant.
Tumor immune infiltration analysis
We used the CIBERSORT algorithm of tumor immune cell infiltration to calculate the abundance of 22 immune cells in the TCGA-LAML cohort. The correlation analysis of immune cells was carried out via the Spearman method.
Immunohistochemistry
Bone marrow smears of AML and normal cases were collected, fixed with 10% neutral formalin, dehydrated with gradient alcohol, and stained with hematoxylin and eosin (HE). The following antibodies were used for immunostaining: dipetidyl peptidase-4 (DPP4) (Abcam, ab187048), GPX4 (Proteintech, 14432-1-AP), LPCAT3 (Abcam, ab239585), SLC7A11 (Proteintech, 26864-1-AP), and transferrin receptor (TFRC) (Proteintech, 10084-2-AP).
PCR
EDTA anticoagulant tubes were used to collect the peripheral blood of healthy adults and AML patients, and Trizol (Invitrogen, China) was used to extract total RNA. Then, the concentration of total RNA was detected by a nucleic acid analyzer. GeneRuler DNA Ladder Mix and Maxima Reverse Transcriptase were used to reverse transcribe RNA into cDNA, and gene expression levels were detected according to the 2X SG Fast qPCR Master Mix (High Rox, B639273, BBI, ABI) kit instructions. GAPDH was used as an internal reference, and the results were calculated using the 2−∆∆Ct method.
RNA-sequence (RNA-seq)
EDTA anticoagulant tubes were used to collect the peripheral blood of healthy adults and AML patients, and Trizol (Invitrogen, China) was used to extract total RNA. RNA samples were used to perform NGS. The library construction and transcriptome sequencing were completed by Shenggong Bioengineering (Shanghai) Co., Ltd.
Drug sensitivity analysis based on risk score
The R package pRRophetic was used to perform the drug sensitivity analysis.
Statistical analysis
The differential FRGs were screened through the Wilcoxon method. Kaplan-Meier (KM) plots were used to analyze the differential survival between groups, and log-rank P<0.05 was considered statistically significant. Univariate cox regression, lasso regression, and multivariate (stepwise) cox regression were used to construct the prognostic signature. Wilcoxon and Spearman tests were used for difference analysis and correlation analysis, respectively. P<0.05 was considered statistically significant.
Ethical statement
The study was conducted in accordance with the Declaration of Helsinki (as revised in 2013).
Results
Differential expression of FRGs in AML patients
We retrieved 23 FRGs from PubMed and analyzed the differential expression of FRGs between AML (n=151) and normal bone marrow (n=70). The heatmap plot showed that there were 22 differentially expressed FRGs (Figure 1).
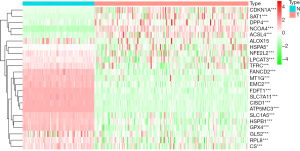
Establishment of the FRG prognostic signature for AML
We obtained 7 FRGs that affected the OS of AML patients via univariate cox regression of differentially expressed FRGs. The results of lasso regression indicated that λ=−4.4 was the optimal value, then 6 FRGs were obtained for further analysis (Figure 2A,2B). Finally, a 5-FRG prognostic signature was established for AML (Figure 2C).
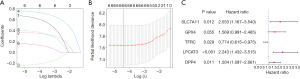
A heatmap was generated showing the FRG signature’s gene expression in low-risk and high-risk samples (Figure 3A). The risk score curve and survival status plot indicated that low and high-risk could well distinguish between surviving and dead patients (Figure 3B,3C). The nomogram and calibration curves demonstrated that the FRG prognostic signature had perfect predictive ability (Figure 3D-3G).
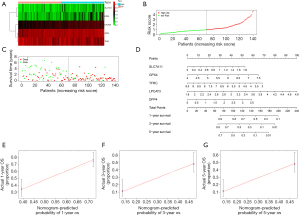
KM survival analysis and ROC curve of the FRG signature
The area under curve (AUC) values of the 1-, 3-, and 5-year ROC curves of the FRG signature were 0.804, 0.785, and 0.930, respectively (Figure 4A). KM survival analysis indicated that patients with low risk had a better OS for AML (log-rank P<0.001) (Figure 4B).
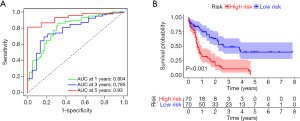
FRG expression levels and their association with prognosis
There were 5 genes in the FRG signature, namely DPP4, LPCAT3, TFRC, GPX4, and SLC7A11. DPP4 was highly expressed in tumors compared with normal samples (Figure 5A), while LPCAT3, TFRC, GPX4, and SLC7A11 were lowly expressed in tumor samples (Figure 5B-5E). In terms of prognosis, high expression of DPP4, LPCAT3, GPX4, and SLC7A11 resulted in a shorter OS, while high expression of TFRC resulted in a better OS in AML patients (Figure 5F-5J).
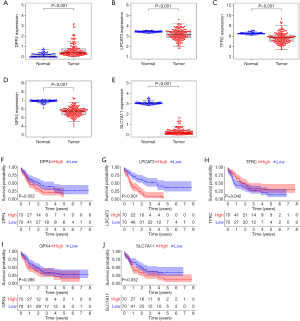
The relative abundance and correlation of 22 immune cells in the TCGA-LAML cohort
The histogram shows the relative abundance of 22 immune cells in the TCGA-LAML cohort (Figure 6A). The heatmap of correlations between the 22 immune cells indicated that M2 macrophages were negatively correlated with other immune cells, and resting mast cells were positively correlated with other immune cells (Figure 6B).
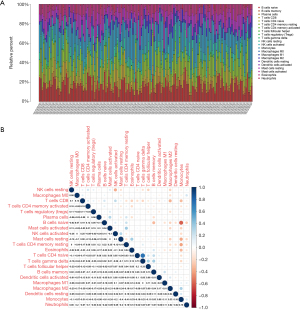
Prognostic immune cells in AML patients
KM survival analysis indicated that high infiltration of resting mast cells resulted in a better OS in AML patients (Figure 7A). Nevertheless, high infiltration of M2 macrophages resulted in a poor prognosis (Figure 7B).
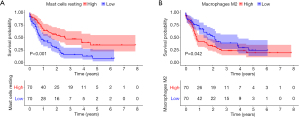
Correlation between the FRG signature biomarker and the abundance of resting mast cells and M2 macrophages
Spearman correlation analysis demonstrated that DPP4 was negatively correlated with resting mast cells and M2 macrophages (Figure 8A). GPX4 was positively correlated with resting mast cells but negatively correlated with M2 macrophages (Figure 8B). LPCAT3 was positively correlated with resting mast cells but negatively correlated with M2 macrophages (Figure 8C). SLC7A11 was positively correlated with resting mast cells but negatively correlated with M2 macrophages (Figure 8D). TFRC was negatively correlated with resting mast cells but positively correlated with M2 macrophages (Figure 8E).
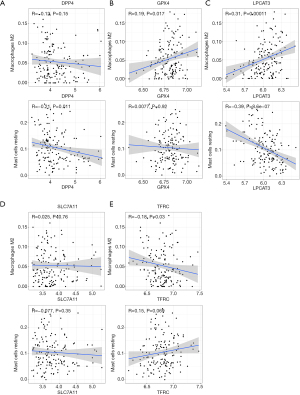
The results of validation experiments
The results of immunohistochemistry indicated that DPP4, GPX4, LPCAT3, SLC7A11, and TFRC had higher expression in AML bone marrow samples (Figure 9). Furthermore, PCR results showed that TFRC (P<0.01) was significantly overexpressed, but DPP4 (P <0.01), GPX4 (P<0.01), LPCAT3 (P<0.01), and SLC7A11 (P<0.01) were significantly downregulated in AML samples (Figure 10). To further validate these selected gene expression levels between normal and AML samples, RNA-seq was performed, and the results showed that TFRC was significantly overexpressed in AML samples (P=2.13E-6), while DPP4 (P=0.016) was significantly downregulated in AML samples (https://cdn.amegroups.cn/static/public/atm-21-3368-1.xls).
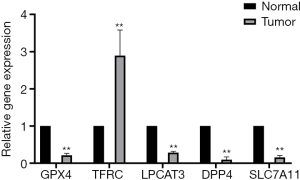
Drug sensitivity
The ultimate goal of cancer research is finding novel or complementary therapy regimens for cancer patients. We used TFRC and DPP4 to divide AML patients into high- and low-risk score groups, and explored the association between risk score and drug sensitivity. The results showed that patients with downregulation of TFRC were resistant to many drugs such as ATRA, axitinib, and vinorelbine, among others, but sensitive to dasatinib, bryostatin.1, and so on (Figure 11). According to DPP4, the sensitivity analysis revealed that patients with scores based on the DPP4 group were resistant to CMK and cytarabine, and among others, but sensitive to dasatinib Figure 12).
Discussion
With the better learning the critical role of ferroptosis in tumorigenesis, therapy response, drug resistance in various cancer types. FRGs have been shown to be important factors that significantly influence tumor progression in multiple cancer types such as hepatocellular carcinoma, clear cell renal cell carcinoma, and breast cancer (27-29). AML, as the most common hematological malignancy in adult patients, is still an incurable disease and poses a big challenge for public health. A number of studies have shown that ferroptosis-related signatures take part in several important processes in solid cancer, but no study has revealed the underlying mechanism and role of FRGs in AML. We therefore attempted to investigate their expression levels, prognostic role, influence on the TME, and the effect of drug resistance in AML.
The transcriptome data of AML patients was downloaded from TCGA and the transcriptome data of normal samples was obtained from the GTEx database, and FRGs were selected via public articles. We analyzed the expression levels of FRGs between AML and normal samples. A prognostic model based on FRGs was constructed via lasso regression. Among the genes, SLC7A11, GPX4, TFRC, LPCAT3, and DPP4 were further investigated in terms of their expression levels and prognostic role in AML. We performed validation experiments to verify the final selected gene expression levels via immunohistochemistry, PCR, and RNA-seq. Finally, we explored whether there was an association between immune infiltration and drug sensitivity, and finally selected FRGs.
Recently, more and more studies have revealed the significant role of ferroptosis in cancer. Apart from being a unique form of cell death, ferroptosis has been shown to play important roles in cancer stem cells and the TME (30-32). As the most important regulators in the ferroptosis process, FRGs have been confirmed to play critical roles in the prognosis and resistance of glioma (33,34). In our study, DPP4 was overexpressed, while LPCAT3, TFRC, GPX4, and SLC7A11 were downregulated in AML samples compared to normal samples. Interestingly, several gene expression levels were inconsistent in the public dataset analysis. DPP4, GPX4, LPCAT3, SLC7A11, and TFRC all had higher expression in AML bone marrow samples. TFRC was significantly overexpressed, but DPP4, GPX4, LPCAT3, and SLC7A11 were significantly downregulated in AML samples via PCR analysis. RNA-seq results showed that TFRC was significantly overexpressed while DPP4 was significantly downregulated in AML samples. The prognostic model showed that SLC7A11, GPX4, TFRC, LPCAT3, and DPP4 significantly influenced the prognosis of AML patients. DPP4, LPCAT3, GPX4, and SLC7A11 may act as adverse biomarkers, while controversially, TFRC may act as a protective factor for AML patients. DPP4 acts as an adverse signature for breast, prostate, and pancreatic cancer, and inhibition of DPP4 can improve the prognosis of these patients (35). Zhang et al.’s study indicated that overexpression of glutathione peroxidase 4 (GPX4) could enhance cisplatin resistance in vitro (36). Guerriero et al. revealed that GPX4 was significantly overexpressed in human hepatocellular carcinoma, further indicating that expression levels may be impacted by cancer status (37). Ma et al. revealed that SLC7A11 was overexpressed in laryngeal squamous cell carcinoma, and the upregulation of SLC7A11 promoted tumor progression (38). From these findings, we can conclude that DPP4, LPCAT3, GPX4, and SLC7A11 have essential biological functions in multiple cancer types, and most of them act as tumor promoters. In regards to AML, only GPX4 has been investigated in terms of its expression and prognostic role. Wei et al. showed that GPX4 was significantly downregulated in AML patient samples, and overexpression of GPX4 indicated a better outcome (39). In regards to TFRC, Huang et al. revealed that TFRC accelerated the progression of epithelial ovarian cancer via upregulating AXIN2 expression (40). In another study, TFRC also acted as a promoter of liver cancer cells, and inhibition of TFRC could suppress cancer cell growth and survival (41). From these findings, TFRC may be an oncogene for liver cancer and epithelial ovarian cancer, which is inconsistent with its prognostic role in AML patients. There has been no study that has explored the role of TFRC in AML.
Based on the fundamental function of ferroptosis in immune responses, we also performed an analysis of the relationship between final selected FRGs and immune cell infiltration. Based on the validation experiment results, we finally selected DPP4 and TFRC for this analysis. The results showed that TFRC and DPP4 were negatively correlated with resting mast cells but positively correlated with M2 macrophages. The TME is one of the critical regulators of immunotherapy, chemotherapy response, and tumor progression (42-44). Research on the TME in solid tumors has been prosperous, but the underlying mechanisms of the TME in therapy response, prognosis, and tumor progression are still unclear. Based on the complexity of the microenvironment of AML, only a few studies have preliminarily investigated the TME of AML (45-48). Carter et al. revealed that the TME can significantly influence the drug sensitivity of AML (45). Furthermore, our results showed that resting mast cell infiltration resulted in a better OS, but high infiltration of M2 macrophages resulted in a poor prognosis for AML patients. Lan et al. revealed that M2 macrophage-derived exosomes promoted the invasion and migration ability of colon cancer cells (49). M2 macrophages also served as promoters of multiple cancer types such as breast, gastric, and bladder cancer (50,51). The fundamental biological function of resting mast cells in cancer still remains to be elucidated, but several studies have shown that they may have a strong influence on cancer (52-54). Xu et al. indicated that M2 macrophages were enriched in AML, and led to poor outcomes (55). The other type of macrophages, M1 macrophages, may serve as protective factors in AML (56). These results also highlight the important role of the TME in AML, but there is still a long way to go.
The ultimate goal of the present study was to find a reasonable novel or complimentary therapy regimen for AML patients. We analyzed the association between DPP4, TFRC, and drug sensitivity in AML patients. The results showed that patients with downregulation of TFRC lead to resistant to ATRA, AZD.2281, CMK, and metformin, and upregulated TFRC induce resistant to bexarotene, bicalutamide, and dasatinib. According to DPP4, patients with high-risk scores were resistant to CMK and cytarabine, and among others, but sensitive to dasatinib. The dysregulated expression of DPP4 can influence the sensitivity to cytarabine, and cytarabine is one of the first-line therapy regimens in AML. Therefore, more reasonable chemotherapy regimens can be selected via this analysis.
Conclusions
In our study, we found that FRGs can serve as diagnostic and prognostic biomarkers for AML patients. FRGs not only have a strong influence on the TME of AML, but also drug resistance. The findings of this study provide useful information for clinicians to select therapy regimens based on FRG expression levels, and pave the way for future fundamental research to understand the underlying mechanisms of ferroptosis in AML.
Acknowledgments
Funding: None.
Footnote
Reporting Checklist: The authors have completed the REMARK reporting checklist. Available at https://dx.doi.org/10.21037/atm-21-3368
Conflicts of Interest: All authors have completed the ICMJE uniform disclosure form (available at https://dx.doi.org/10.21037/atm-21-3368). The authors have no conflicts of interest to declare.
Ethical Statement: The authors are accountable for all aspects of the work in ensuring that questions related to the accuracy or integrity of any part of the work are appropriately investigated and resolved. The study was conducted in accordance with the Declaration of Helsinki (as revised in 2013).
Open Access Statement: This is an Open Access article distributed in accordance with the Creative Commons Attribution-NonCommercial-NoDerivs 4.0 International License (CC BY-NC-ND 4.0), which permits the non-commercial replication and distribution of the article with the strict proviso that no changes or edits are made and the original work is properly cited (including links to both the formal publication through the relevant DOI and the license). See: https://creativecommons.org/licenses/by-nc-nd/4.0/.
References
- Callens C, Coulon S, Naudin J, et al. Targeting iron homeostasis induces cellular differentiation and synergizes with differentiating agents in acute myeloid leukemia. J Exp Med 2010;207:731-50. [Crossref] [PubMed]
- Roboz GJ. Novel approaches to the treatment of acute myeloid leukemia. Hematology Am Soc Hematol Educ Program 2011;2011:43-50. [Crossref] [PubMed]
- Rashidi A, Weisdorf DJ, Bejanyan N. Treatment of relapsed/refractory acute myeloid leukaemia in adults. Br J Haematol 2018;181:27-37. [Crossref] [PubMed]
- Kishtagari A, Levine RL, Viny AD. Driver mutations in acute myeloid leukemia. Curr Opin Hematol 2020;27:49-57. [Crossref] [PubMed]
- Döhner H, Estey E, Grimwade D, et al. Diagnosis and management of AML in adults: 2017 ELN recommendations from an international expert panel. Blood 2017;129:424-47. [Crossref] [PubMed]
- Eguchi M, Minami Y, Kuzume A, et al. Mechanisms Underlying Resistance to FLT3 Inhibitors in Acute Myeloid Leukemia. Biomedicines 2020;8:245. [Crossref] [PubMed]
- Weisberg E, Sattler M, Ray A, et al. Drug resistance in mutant FLT3-positive AML. Oncogene 2010;29:5120-34. [Crossref] [PubMed]
- Martelli MP, Martino G, Cardinali V, et al. Enasidenib and ivosidenib in AML. Minerva Med 2020;111:411-26. [Crossref] [PubMed]
- Antar A, Otrock ZK, El-Cheikh J, et al. Inhibition of FLT3 in AML: a focus on sorafenib. Bone Marrow Transplant 2017;52:344-51. [Crossref] [PubMed]
- Intlekofer AM, Shih AH, Wang B, et al. Acquired resistance to IDH inhibition through trans or cis dimer-interface mutations. Nature 2018;559:125-9. [Crossref] [PubMed]
- Kerins MJ, Ooi A. The Roles of NRF2 in Modulating Cellular Iron Homeostasis. Antioxid Redox Signal 2018;29:1756-73. [Crossref] [PubMed]
- Dixon SJ, Lemberg KM, Lamprecht MR, et al. Ferroptosis: an iron-dependent form of nonapoptotic cell death. Cell 2012;149:1060-72. [Crossref] [PubMed]
- Stockwell BR, Friedmann Angeli JP, Bayir H, et al. Ferroptosis: A Regulated Cell Death Nexus Linking Metabolism, Redox Biology, and Disease. Cell 2017;171:273-85. [Crossref] [PubMed]
- Friedmann Angeli JP, Krysko DV, Conrad M. Ferroptosis at the crossroads of cancer-acquired drug resistance and immune evasion. Nat Rev Cancer 2019;19:405-14. [Crossref] [PubMed]
- Shi L, Huang Y, Huang X, et al. Analyzing the key gene expression and prognostics values for acute myeloid leukemia. Transl Cancer Res 2020;9:7284-98. [Crossref]
- Viswanathan VS, Ryan MJ, Dhruv HD, et al. Dependency of a therapy-resistant state of cancer cells on a lipid peroxidase pathway. Nature 2017;547:453-7. [Crossref] [PubMed]
- Liu Y, Xu Z, Jin T, et al. Ferroptosis in Low-Grade Glioma: A New Marker for Diagnosis and Prognosis. Med Sci Monit 2020;26:e921947 [PubMed]
- Tang B, Zhu J, Li J, et al. The ferroptosis and iron-metabolism signature robustly predicts clinical diagnosis, prognosis and immune microenvironment for hepatocellular carcinoma. Cell Commun Signal 2020;18:174. [Crossref] [PubMed]
- Tang R, Hua J, Xu J, et al. The role of ferroptosis regulators in the prognosis, immune activity and gemcitabine resistance of pancreatic cancer. Ann Transl Med 2020;8:1347. [Crossref] [PubMed]
- Zhuo S, Chen Z, Yang Y, et al. Clinical and Biological Significances of a Ferroptosis-Related Gene Signature in Glioma. Front Oncol 2020;10:590861 [Crossref] [PubMed]
- Du J, Wang T, Li Y, et al. DHA inhibits proliferation and induces ferroptosis of leukemia cells through autophagy dependent degradation of ferritin. Free Radic Biol Med 2019;131:356-69. [Crossref] [PubMed]
- Du Y, Bao J, Zhang MJ, et al. Targeting ferroptosis contributes to ATPR-induced AML differentiation via ROS-autophagy-lysosomal pathway. Gene 2020;755:144889 [Crossref] [PubMed]
- Zhu HY, Huang ZX, Chen GQ, et al. Typhaneoside prevents acute myeloid leukemia (AML) through suppressing proliferation and inducing ferroptosis associated with autophagy. Biochem Biophys Res Commun 2019;516:1265-71. [Crossref] [PubMed]
- Hassannia B, Vandenabeele P, Vanden Berghe T. Targeting Ferroptosis to Iron Out Cancer. Cancer Cell 2019;35:830-49. [Crossref] [PubMed]
- Li J, Cao F, Yin HL, et al. Ferroptosis: past, present and future. Cell Death Dis 2020;11:88. [Crossref] [PubMed]
- Liang C, Zhang X, Yang M, et al. Recent Progress in Ferroptosis Inducers for Cancer Therapy. Adv Mater 2019;31:e1904197 [Crossref] [PubMed]
- Li Z, Chen L, Chen C, et al. Targeting ferroptosis in breast cancer. Biomark Res 2020;8:58. [Crossref] [PubMed]
- Liang JY, Wang DS, Lin HC, et al. A Novel Ferroptosis-related Gene Signature for Overall Survival Prediction in Patients with Hepatocellular Carcinoma. Int J Biol Sci 2020;16:2430-41. [Crossref] [PubMed]
- Wu G, Wang Q, Xu Y, et al. A new survival model based on ferroptosis-related genes for prognostic prediction in clear cell renal cell carcinoma. Aging (Albany NY) 2020;12:14933-48. [Crossref] [PubMed]
- Bisaro B, Mandili G, Poli A, et al. Proteomic analysis of extracellular vesicles from medullospheres reveals a role for iron in the cancer progression of medulloblastoma. Mol Cell Ther 2015;3:8. [Crossref] [PubMed]
- Schonberg DL, Miller TE, Wu Q, et al. Preferential Iron Trafficking Characterizes Glioblastoma Stem-like Cells. Cancer Cell 2015;28:441-55. [Crossref] [PubMed]
- Zhu T, Shi L, Yu C, et al. Ferroptosis Promotes Photodynamic Therapy: Supramolecular Photosensitizer-Inducer Nanodrug for Enhanced Cancer Treatment. Theranostics 2019;9:3293-307. [Crossref] [PubMed]
- Liu HJ, Hu HM, Li GZ, et al. Ferroptosis-Related Gene Signature Predicts Glioma Cell Death and Glioma Patient Progression. Front Cell Dev Biol 2020;8:538. [Crossref] [PubMed]
- Wan RJ, Peng W, Xia QX, et al. Ferroptosis-related gene signature predicts prognosis and immunotherapy in glioma. CNS Neurosci Ther 2021;27:973-86. [Crossref] [PubMed]
- Shah C, Hong YR, Bishnoi R, et al. Impact of DPP4 Inhibitors in Survival of Patients With Prostate, Pancreas, and Breast Cancer. Front Oncol 2020;10:405. [Crossref] [PubMed]
- Zhang X, Sui S, Wang L, et al. Inhibition of tumor propellant glutathione peroxidase 4 induces ferroptosis in cancer cells and enhances anticancer effect of cisplatin. J Cell Physiol 2020;235:3425-37. [Crossref] [PubMed]
- Guerriero E, Capone F, Accardo M, et al. GPX4 and GPX7 over-expression in human hepatocellular carcinoma tissues. Eur J Histochem 2015;59:2540. [Crossref] [PubMed]
- Ma Z, Zhang H, Lian M, et al. SLC7A11, a component of cysteine/glutamate transporter, is a novel biomarker for the diagnosis and prognosis in laryngeal squamous cell carcinoma. Oncol Rep 2017;38:3019-29. [Crossref] [PubMed]
- Wei J, Xie Q, Liu X, et al. Identification the prognostic value of glutathione peroxidases expression levels in acute myeloid leukemia. Ann Transl Med 2020;8:678. [Crossref] [PubMed]
- Huang Y, Huang J, Huang Y, et al. TFRC promotes epithelial ovarian cancer cell proliferation and metastasis via up-regulation of AXIN2 expression. Am J Cancer Res 2020;10:131-47. [PubMed]
- Muhammad JS, Bajbouj K, Shafarin J, et al. Estrogen-induced epigenetic silencing of FTH1 and TFRC genes reduces liver cancer cell growth and survival. Epigenetics 2020;15:1302-18. [Crossref] [PubMed]
- Farhood B, Najafi M, Mortezaee K. CD8(+) cytotoxic T lymphocytes in cancer immunotherapy: A review. J Cell Physiol 2019;234:8509-21. [Crossref] [PubMed]
- Hinshaw DC, Shevde LA. The Tumor Microenvironment Innately Modulates Cancer Progression. Cancer Res 2019;79:4557-66. [Crossref] [PubMed]
- Junttila MR, de Sauvage FJ. Influence of tumour micro-environment heterogeneity on therapeutic response. Nature 2013;501:346-54. [Crossref] [PubMed]
- Carter BZ, Mak PY, Wang X, et al. An ARC-Regulated IL1beta/Cox-2/PGE2/beta-Catenin/ARC Circuit Controls Leukemia-Microenvironment Interactions and Confers Drug Resistance in AML. Cancer Res 2019;79:1165-77. [Crossref] [PubMed]
- Nahas MR, Stroopinsky D, Rosenblatt J, et al. Hypomethylating agent alters the immune microenvironment in acute myeloid leukaemia (AML) and enhances the immunogenicity of a dendritic cell/AML vaccine. Br J Haematol 2019;185:679-90. [Crossref] [PubMed]
- Shafat MS, Gnaneswaran B, Bowles KM, et al. The bone marrow microenvironment - Home of the leukemic blasts. Blood Rev 2017;31:277-86. [Crossref] [PubMed]
- van Galen P, Hovestadt V, Wadsworth Ii MH, et al. Single-Cell RNA-Seq Reveals AML Hierarchies Relevant to Disease Progression and Immunity. Cell 2019;176:1265-81.e24. [Crossref] [PubMed]
- Lan J, Sun L, Xu F, et al. M2 Macrophage-Derived Exosomes Promote Cell Migration and Invasion in Colon Cancer. Cancer Res 2019;79:146-58. [Crossref] [PubMed]
- Chen Y, Zhang S, Wang Q, et al. Tumor-recruited M2 macrophages promote gastric and breast cancer metastasis via M2 macrophage-secreted CHI3L1 protein. J Hematol Oncol 2017;10:36. [Crossref] [PubMed]
- Martínez VG, Rubio C, Martinez-Fernandez M, et al. BMP4 Induces M2 Macrophage Polarization and Favors Tumor Progression in Bladder Cancer. Clin Cancer Res 2017;23:7388-99. [Crossref] [PubMed]
- Li J, Li X, Zhang C, et al. A signature of tumor immune microenvironment genes associated with the prognosis of nonsmall cell lung cancer. Oncol Rep 2020;43:795-806. [PubMed]
- Pan Q, Wang L, Chai S, et al. The immune infiltration in clear cell Renal Cell Carcinoma and their clinical implications: A study based on TCGA and GEO databases. J Cancer 2020;11:3207-15. [Crossref] [PubMed]
- Tang X, Shu Z, Zhang W, et al. Clinical significance of the immune cell landscape in hepatocellular carcinoma patients with different degrees of fibrosis. Ann Transl Med 2019;7:528. [Crossref] [PubMed]
- Xu ZJ, Gu Y, Wang CZ, et al. The M2 macrophage marker CD206: a novel prognostic indicator for acute myeloid leukemia. Oncoimmunology 2020;9:1683347 [Crossref] [PubMed]
- Yang X, Feng W, Wang R, et al. Repolarizing heterogeneous leukemia-associated macrophages with more M1 characteristics eliminates their pro-leukemic effects. Oncoimmunology 2018;7:e1412910 [Crossref] [PubMed]
(English Language Editor: C. Betlazar-Maseh)