Hemorrhagic stroke outcomes of KApSR patients with co-morbid diabetes and Alzheimer’s disease
Introduction
Kentucky and West Virginia lie within a portion of rural Appalachia, a population with an increased burden of negative health disparities (1-3). According to the United Heath Foundation, as of 2019, Kentucky and West Virginia ranked 43rd and 45th out of 50, respectively, for overall health in the US. Contributing to this overall negative heath disparity are their rankings of 44th and 46th for stroke and 44th and 50th for diabetes, respectively. Using Medicare costs as a measure of overall health (i.e., high Medicare cost indicates poor health), diabetes mellitus (DM; i.e., type 2 diabetes), has been shown to increase cost by a factor of 2.3 (4). DM is associated with an increased risk of Alzheimer’s disease (AD) (5-7) and poorer outcomes following stroke (8). AD is also an additive comorbidity that doubles Medicare cost when associated with stroke and diabetes (9) and has an increased risk of hemorrhagic stroke (10). Stroke, AD, and DM are among the top 7 leading causes of death, not only in Kentucky and West Virginia (11), but globally (12). Many retrospective studies have examined how a single comorbidity influences a neurological disease, such as hemorrhagic stroke, but few have looked at the effect of dual comorbidities or specifically how AD influences hemorrhagic stroke outcomes.
The Kentucky Appalachian Stroke Registry (KApSR) is a database of demographic and clinical data from patients in the rural Kentucky and West Virgina regions with stroke (13). In this study, we used KApSR to retrospectively analyze the contributions of diabetes and AD to outcomes following hemorrhagic stroke such as length of stay, discharge destination, change in National Institute of Health Stroke (NIHSS), and 30-day readmission rates. Our central aim was to examine the relationship between the combination of diabetes and AD and hemorrhagic stroke severity.
We present the following article in accordance with the STROBE reporting checklist (available at https://dx.doi.org/10.21037/atm-21-1451).
Methods
Ethical statement
The study was conducted in accordance with the Declaration of Helsinki (as revised in 2013). The study was approved by Kentucky Appalachian Stroke Registry (KApSR) (de-identified data provided under IRB# 13-08058) and individual consent for this retrospective analysis was waived.
Study sample
Kentucky Appalachian Stroke Registry (KApSR) is a database of demographic and clinical data from patients that live in Appalachia Kentucky (13), a distinct region with increased health disparities and stroke severity. A cross-sectional analysis of inpatients with cardiovascular disease (CVD) containing a principle diagnosis of hemorrhagic stroke were evaluated for DM and/or AD in the KApSR database from 2010–2018. Hemorrhagic stroke patients were identified in KApSR as presenting with intracerebral hemorrhage (ICH) or subarachnoid hemorrhage (SAH), methods previously described (13). Briefly, demographics, comorbidities (defined by International Classification of Disease (ICD) codes), glycated hemoglobin A1 (hbA1c) values, previous diagnosis of AD, and outcome measures were collected from electronic health records (EHR) for inpatients, >18 years old, with an ICH or SAH, ICD9 codes: 431 and 430; ICD10 codes of 161 and 160, respectively. The accuracy of using EHR in KApSR was previously validated and reported by Kitzman et al. (13). We separated patients into four comorbidity categories: no previous diagnosis of DM or AD (DM-/AD-), DM only (DM+), AD only (AD+), or combined DM and AD (DM+/AD+).
Outcomes
Admission and discharge NIHSS were collected, along with length of stay, and discharge destination (favorable or unfavorable). Favorable discharge destinations include: alive/routine, transfer to other institution, hospital swing bed, or home health services, rehabilitation facility/unit, against medical advice, police case, or billin. Unfavorable discharge destinations include: death (≤48 or >48 hrs), hospice, skilled nursing facility, or long-term care hospital. Change in NIHSS from admission to discharge was measured as an indicator of short-term inpatient outcome (14).
Statistical analysis
Demographic characteristics are presented without adjustment for covariates as mean ± standard deviation or sample number (percentages). A1Cs were categorized as normal (<5.7%) or as abnormal (≥5.7%) (15,16) that included diabetics (A1C ≥6.5%) and prediabetics (5.7≤ A1C <6.5) (17). Between-group differences in demographic characteristics were investigated using a series of one-way ANOVAs for continuous variables and Chi-square or Fisher’s exact tests for categorical variables. Differences in non-normal continuous variables were assessed using a Kruskal-Wallis test. Significant between group differences were used as control variables in model 3 of the main outcome analysis (described below). To investigate between group differences in the main outcome variables, three models were employed. Model 1 was unadjusted, model 2 was adjusted for age and sex, and model 3 was adjusted for age, sex, type of hemorrhage, race, and all significant comorbidities (other than DM and AD). The DM/AD was the reference group for all analyses. Depending on the distribution of the outcome, these models were analyzed using linear regression, logistic regression, or ordinal logistic regression. Due to its non-normal distribution and inability to achieve normality under any data transformation, NIHSS was recoded into an ordinal variable defined as: 0–5= mild, 6–13= moderate, and >13= severe stroke (18-20). The change (Admission-Discharge) in NIHSS was transformed into an ordinal variable based on whether the patient was: declining, staying the same, or improving. This change was based on the continuous NIHSS value. Discharge destination, a binary variable, was classified as favorable or unfavorable using the rules outlined above. Length of stay positively skewed and was log transformed to approximate normality. For all models, odds ratios and 95% confidence intervals (CI) were calculated for all outcome measures. For each outcome, Dunnett’s procedure was used to correct for multiple comparisons, since each category was being compared against the DM/AD condition (21). Graphical and statistical analysis was performed using Graphpad Prism and SAS version 9.4 (SAS Institute Inc.).
Missing data and statistical assumption
NIHSS, the main outcome, was missing for 40.2% (n=833) of the sample. Our hospital institution transitioned from the Primary Stroke Center to a Comprehensive Stroke Center in 2013, and our standards and mechanisms for documentation in the EMR changed. These evolutions could account for missing data points in our study, which, unfortunately, could not be recovered. A missing data analysis was performed using demographic and comorbidity variables as predictors of missingness in a multivariable logistic regression. Table S1 (see supplemental materials) contains the results of this analysis. The following characteristics and comorbidities were associated with lower odds of having missing NIHSS: older age, current hypertension, tobacco use, dyslipidemia and carotid stenosis. Females and those with SAH stroke type had higher levels of missing data. There were no significant differences between AD or DM conditions. These variables were included in the models where NIHSS were the outcome of interest. No covariates contained missing data.
As a result of missing NIHSS data and a small sample for the main comparison condition (DM+/AD+), a there is a concern for the violation of statistical assumptions due to the small expected cell sizes for the contingency tables of Model 1 (described above). To address this concern, Fisher’s exact tests, a nonparametric version of a chi-square test of independence, was performed on NIHSS outcomes for all pairwise comparisons where DM+/AD+ was the reference group. The substantive results of these tests aligned with the parametric versions reported in the results. Despite certain statistical assumptions of our model not being met, the agreement between these two sets of analyses lends credence to our interpretations.
Results
Demographic characteristics
A total of 2,071 patients with hemorrhagic stroke were included: 1,459 (70.4%) were without DM and AD (DM-/AD-), 567 (27.4%) had DM alone (DM+), 29 (1.4%) had AD alone (AD+), and 16 had both DM and AD (DM+/AD+). Table 1 presents descriptive statistics for the demographic and comorbidity variables with between group comparisons. There were significant (P<0.0001) differences in the age of the groups (Figure 1A): 61.3±16.5 yrs (DM-/AD-) to 66.01±12.5 yrs (DM+), 80.9±6.6 yrs (AD+), and 77.4±10.0 yrs (DM+/AD+). Patients with an AD diagnosis (AD+ or DM+/AD+) were significantly (P<0.0001, P<0.05, respectively) older than DM alone. Sex was significantly (P<0.05) different among the groups, with ~50% female for DM-/AD- and DM+ to ~76% female in AD+ and ~31% female in DM+/ AD+ (Figure 1B). A significantly higher percentage of DM+ (P<0.0001) and AD+ (P<0.01), compared to the DM-/AD- (Figure 1C), had a prior stroke. DM+/AD+ followed this trend but did not reach significance (P=0.08). No statistical difference among race in the four groups was observed (Table 1). Across the four groups, ICH was more common than SAH (Figure 1D), with DM+ and AD+ having significantly more ICH than SAH compared to DM-/AD-.
Table 1
Variable | -DM/-AD | DM | AD | DM/AD | Test statistic | P value |
---|---|---|---|---|---|---|
Total N | 1,459 (70.4%) | 567 (27.4%) | 29 (1.4%) | 16 (0.8%) | ||
Age, yrs (mean ± SD) | 61.3±16.5 | 66.0±12.5 | 80.9±6.6 | 77.4±10.0 | 31.1 | <0.0001 |
Sex, female, N (%) | 745 (51.1%) | 290 (51.1%) | 22 (75.9%) | 5 (31.3%) | 9.615 | 0.0221 |
Stroke type, ICH, n (%) | 974 (66.8%) | 436 (76.9%) | 25 (86.2%) | 13 (81.3%) | 24.69 | <0.0001 |
Race, n (%) | 0.2954 | |||||
White | 1,317 (90.3%) | 565 (99.8%) | 29 (100%) | 12 (75%) | ||
Black | 107 (7.3%) | 0 | 0 | 4 (25%) | ||
Asian | 11 (0.8%) | 0 | 0 | 0 | ||
Hispanic | 3 (0.2%) | 0 | 0 | 0 | ||
Other/non-Hispanic | 8 (0.5%) | 1 (0.2%) | 0 | 0 | ||
Refused/unreported | 13 (0.9%) | 1 (0.2%) | 0 | 0 | ||
Comorbidities, n (%) | ||||||
Prior stroke/transient ischemic attack | 225 (15.4%) | 130 (22.9%) | 10 (34.5%) | 5 (31.3%) | 23.25 | <0.0001 |
Atrial fibrillation | 208 (14%) | 131 (23%) | 9 (31.0%) | 6 (38.0%) | 31.42 | <0.0001 |
Coronary artery disease | 258 (17.7%) | 205 (36.2%) | 11 (37.9%)) | 9 (56.3%) | 91.32 | <0.0001 |
Dyslipidemia | 470 (32.2%) | 324 (57.1%) | 14 (48.3%) | 10 (62.5%) | 110.7 | <0.0001 |
Hypertension | 1,233 (84.6%) | 544 (95.9%) | 22 (75.9%) | 14 (87.5%) | 52.63 | <0.0001 |
Obesity | 177 (12.1%) | 127 (22.4%) | 2 (6.9%) | 1 (6.25%) | 36.52 | <0.0001 |
Carotid stenosis | 42 (2.9%) | 36 (6.3%) | 1 (3.4%) | 0 | 14.06 | 0.0048 |
History of myocardial infarction | 72 (4.9%) | 45 (7.9%) | 1 (3.4%) | 2 (12.5%) | 8.351 | 0.0301 |
Acute myocardial infarction | 78 (5.3%) | 41 (7.2%) | 0 (0) | 2 (12.5%) | 5.729 | 0.1036 |
Tobacco | 600 (41.2%) | 188 (33.2%) | 6 (20.7%) | 6 (37.5%) | 15.14 | 0.0014 |
hbAIc | ||||||
n | 413 | 369 | 12 | 11 | ||
Mean ± SD | 5.6±0.7 | 7.5±1.9 | 5.4±0.4 | 6.6±0.6 | 119.2 | <0.0001 |
≥5.7 AIC (%) | 162 (39.0%) | 326 (88%) | 6 (50%) | 10 (100) | 207.8 | <0.0001 |
Outcomes† | ||||||
Admission NIHSS value, median [IQR] | 7 [1–19] | 7 [2–18] | 9 [2–23] | 19 [15–26] | ||
Ordinal admission NIHSS, n (%) | ||||||
Mild: 0–5 | 389 (46.6%) | 160 (43.4%) | 9 (39.1%) | 1 (9.1%) | ||
Moderate: 6–13 | 166 (19.9%) | 78 (21.1%) | 6 (26.1%) | 1 (9.1%) | ||
Severe: >13 | 280 (33.5%) | 131 (35.5%) | 8 (34.8%) | 9 (81.8%) | ||
Discharge NIHSS value, median [IQR] | 6 [1–18] | 6 [1–19] | 12 [4–23] | 19 [10–22] | ||
Ordinal discharge NIHSS, n (%) | ||||||
Mild: 0–5 | 412 (49.3%) | 179 (48.5%) | 7 (30.4%) | 0 (0%) | ||
Moderate: 6–13 | 149 (17.8%) | 68 (18.4%) | 5 (21.7%) | 4 (36.4%) | ||
Severe: >13 | 274 (32.8%) | 122 (33.1%) | 11 (47.8%) | 7 (63.6%) | ||
NIHSS change value, median [IQR] | 0 [−1 to 1] | 0 [−1 to 2] | 0 [−4 to 1] | 1 [0 to 7] | ||
Ordinal NIHSS change, n (%) | ||||||
Decline | 228 (27.3%) | 108 (29.3%) | 9 (39.1%) | 2 (18.2%) | ||
Stable | 332 (39.8%) | 122 (33.1%) | 7 (30.4%) | 3 (27.3%) | ||
Improve | 275 (32.9%) | 139 (37.7%) | 7 (30.4%) | 6 (54.6%) | ||
Unfavorable discharge, n (%) | 571 (39.1%) | 240 (42.3%) | 18 (62.1%) | 12 (75.0%) | ||
Length of stay (log), mean (SD) | 1.63 (1.16) | 1.71 (1.09) | 1.28 (0.90) | 1.83 (1.10) |
Data are mean ± SD and number with percent of population. †, statistical analysis for outcome presented in Model 1 of
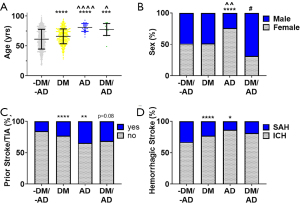
When evaluating comorbidities, a significantly higher percentage of patients with atrial fibrillation (P<0.0001, Figure 2A) and coronary artery disease (P<0.0001, Figure 2B) were seen in DM+, AD+, and DM+/AD+ compared to DM-/AD-. Dyslipidemia was only significantly (P<0.0001) different among the subjects with DM (DM+ and DM+/AD+, Figure 2C) compared to DM-/AD-. Hypertension (P<0.0001, Figure 2D) and obesity (P<0.0001, Figure 2E) increased in subjects with only DM, compared to DM-/AD- and the AD groups. Differences in carotid stenosis (P<0.01) and a history of, but not acute, myocardial infarction (P<0.05) were observed with only the DM group compared to the DM-/AD- group (Table 1). A significantly (P<0.0001, Figure 2F) higher percentage of patients with A1C ≥5.6% were among subjects with DM (DM+ and DM+/AD+) compared to both DM-/AD- and AD. The percentage of prediabetics/diabetics, based on abnormal A1Cs, increase from 39% (DM-/AD-) to 88% (DM+), 50% (AD+), and 100% (DM+/AD+; Figure 2F). Tobacco use (P<0.01, Figure 2G) was significantly higher among DM and AD groups compared to DM-/AD-.
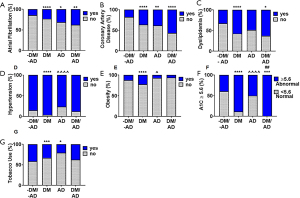
Outcomes
Differences in NIHSS was assessed using ordinal logistic regression. Results are presented in the first three sections of Table 2. Regardless of adjusting for age, sex, and comorbidities, DM+/AD+ patients were significantly more likely to have had a moderate or severe stroke compared to the other three groups. Using parameter estimates from Model 3 (Table 2), DM+/AD+ patients were 10 times (i.e., 1/0.10=10) more likely to have had a moderate or severe stroke based on the admission NIHSS (Figure 3A). At discharge, DM+/AD+ patients were approximately 4.5 times more likely to be in the moderate or severe NIHSS categories compared to DM-/AD- and DM+, which translated into DM+/AD+ subjects having a higher percentage of strokes classified as severe at discharge (Figure 3B). However, even though DM+/AD+ patients had significantly worse admission and discharge NIHSS, their change in NIHSS (admission–discharge) indicating in patient outcome was not significantly different from the other three groups (Figure 3C, Table 2).
Table 2
Variable | Model 1 | Model 2 | Model 3 | ||||||||
---|---|---|---|---|---|---|---|---|---|---|---|
Odds ratio (SE) | 95% CI | P value | Odds ratio (SE) | 95% CI | P value | Odds ratio (SE) | 95% CI | P value | |||
Admission NIHSS† | |||||||||||
-DM/-AD (n=835) | 0.11 (0.78) | 0.02–0.59 | 0.008 | 0.12 (0.78) | 0.02–0.66 | 0.012 | 0.10 (0.80) | 0.02–0.55 | 0.006 | ||
DM+ (n=369) | 0.12 (0.78) | 0.02–0.67 | 0.013 | 0.13 (0.78) | 0.02–0.72 | 0.017 | 0.11 (0.80) | 0.02–0.59 | 0.009 | ||
AD+ (n=23) | 0.13 (0.87) | 0.02–0.87 | 0.033 | 0.12 (0.87) | 0.02–0.80 | 0.026 | 0.09 (0.88) | 0.01–0.63 | 0.013 | ||
DM/AD (n=11) | 1.0 (ref) | 1.0 (ref) | 1.0 (ref) | ||||||||
Discharge NIHSS† | |||||||||||
-DM/-AD (n=835) | 0.21 (0.65) | 0.05–0.86 | 0.028 | 0.25 (0.65) | 0.06–1.03 | 0.055 | 0.22 (0.65) | 0.05–0.92 | 0.036 | ||
DM+ (n=369) | 0.21 (0.65) | 0.05–0.89 | 0.032 | 0.24 (0.65) | 0.06–1.00 | 0.050 | 0.22 (0.66) | 0.05–0.91 | 0.036 | ||
AD+ (n=23) | 0.42 (0.76) | 0.08–2.18 | 0.366 | 0.38 (0.76) | 0.07–1.97 | 0.297 | 0.32 (0.76) | 0.06–1.70 | 0.211 | ||
DM/AD (n=11) | 1.0 (ref) | 1.0 (ref) | 1.0 (ref) | ||||||||
Change NIHSS† | |||||||||||
-DM/-AD (n=835) | 0.46 (0.58) | 0.13–1.62 | 0.270 | 0.41 (0.58) | 0.11–1.46 | 0.195 | 0.37 (0.59) | 0.10–1.35 | 0.151 | ||
DM+ (n=369) | 0.49 (0.59) | 0.14–1.77 | 0.335 | 0.46 (0.59) | 0.13–1.66 | 0.282 | 0.44 (0.59) | 0.12–1.57 | 0.243 | ||
AD+ (n=23) | 0.32 (0.69) | 0.07–1.47 | 0.166 | 0.36 (0.70) | 0.08–1.64 | 0.219 | 0.34 (0.70) | 0.07–1.55 | 0.187 | ||
DM/AD (n=11) | 1.0 (ref) | 1.0 (ref) | 1.0 (ref) | ||||||||
Unfavorable discharge‡ | |||||||||||
-DM/-AD (n=1,459) | 0.21 (0.58) | 0.06–0.76 | 0.014 | 0.33 (0.59) | 0.09–1.19 | 0.097 | 0.26 (0.59) | 0.07–0.96 | 0.040 | ||
DM+ (n=567) | 0.25 (0.58) | 0.07–0.87 | 0.028 | 0.33 (0.59) | 0.09–1.20 | 0.099 | 0.31 (0.59) | 0.08–1.29 | 0.078 | ||
AD+ (n=29) | 0.55 (0.69) | 0.12–2.46 | 0.532 | 0.46 (0.70) | 0.10–2.10 | 0.383 | 0.36 (0.71) | 0.08–1.70 | 0.234 | ||
DM/AD (n=16) | 1.0 (ref) | 1.0 (ref) | 1.0 (ref) | ||||||||
Average stay (log transformed)§ | Mean (SE) | Mean (SE) | Mean (SE) | ||||||||
-DM/-AD (n=1,459) | 1.63 (0.03) | 0.489 | 1.61 (0.03) | 0.177 | 1.95 (0.06) | 0.748 | |||||
DM+ (n=567) | 1.71 (0.05) | 0.684 | 1.74 (0.05) | 0.381 | 1.92 (0.07) | 0.657 | |||||
AD+ (n=29) | 1.28 (0.21) | 0.123 | 1.47 (0.21) | 0.138 | 1.82 (0.21) | 0.515 | |||||
DM/AD (n=16) | 1.83 (0.28) | REF | 1.99 (0.28) | REF | 2.04 (0.27) | REF |
DM+, AD+ served as the reference group for analysis. Data are odds ratios and 95% CI or mean (SE). Both P values and confidence intervals were calculated using Dunnett’s multiple comparison procedure. Model 1: unadjusted; Model 2: adjusted for age and sex; Model 3: adjusted for age, sex, type of hemorrhage, race, and all comorbidities (other than DM and AD). NIHSS was transformed into ordinal variables defined as: 0–5= mild, 6–13= moderate, and >13= severe stroke. The change (Admission-Discharge) in NIHSS was transformed into an ordinal variable based on whether the patient was: declining, staying the same, or improving. Discharge destination transformed into binary variable and classified as favorable or unfavorable. †Ordinal logistic regression. ‡Logistic regression. §Linear Regression. CI, confidence interval; DM, diabetes mellitus; AD, Alzheimer’s disease; NIHSS, National Institutes of Health Stroke Scale; SE, standard error.
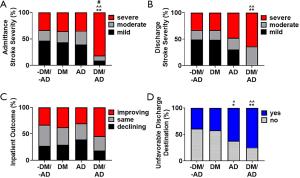
The relationship between comorbidity condition and experiencing an unfavorable discharge was examined using logistic regression. The DM+/AD+ subjects had a significantly higher percentage of unfavorable discharge destinations compared to DM-/AD- and DM+ (Figure 3D). Interestingly, when adjusting for age and sex (model 2), discharge destination was not significantly different among groups. However, in the unadjusted (model 1) and adjusted for age, sex, and comorbidities (model 3; Table 2), the odds of DM+/AD+ having an unfavorable discharge destination was significant (P<0.05) from DM-/AD- and DM+. The average number of days in the hospital (Table 2, regardless of modeling) was not significantly different from the DM+/AD+ group.
Several of the covariates included in Model 3 were significantly related to the outcomes. In particular, older individuals were more likely to have had a moderate to severe stroke based on their discharge NIHSS, they were less likely to remain stable or improve from admission to discharge, and were more likely to have an unfavorable outcome. Additionally, stroke type was a strong predictor of outcomes. Compared to those who experienced a SAH stroke, those who had an ICH stroke were more likely to have experienced a moderate to severe stroke based on both admission and discharge NIHSS, were less likely to remain stable or improve, and were more likely to experience an unfavorable discharge destination.
Discussion
Compared to ischemic stroke, hemorrhagic stroke leads to a higher mortality rate (22,23), increased need for palliative care (24), and higher hospitalization costs (25) associated with higher Medicare expenditures (26). This highlights the need to predict outcomes and manage comorbidities before hemorrhagic strokes occur. Studies have looked at the risk of AD and dementia after stroke (27,28), but few studies have looked at hemorrhagic stroke risk in patients with AD (29). Our study found DM+/AD+ patients had more severe hemorrhagic strokes (higher NIHSS) at admission and discharge that contributed to a larger percentage with unfavorable discharge destinations. No differences between groups were observed for length of hospital stay and inpatient outcomes.
The presence of comorbidities is known to increase mortality following stroke (30,31). Specifically, high blood glucose, as occurs in diabetes, is associated with early mortality (32). An abnormal A1c may be an indicator that the patient is prediabetic or not managing their diabetes with their current regimen, possibly due to a lack of diagnosis, ability to treat, or patient compliance.
DM increases the risk of developing AD (33-36), and the metabolic changes associated with DM can initiate/accelerate AD pathologies through oxidative stress, inflammation, and vascular injury (37). AD is associated with amyloid and tau pathologies that damage neurons or weaken the vasculature. Changes in cognition associated with AD may be associated with the weakening of the cerebrovasculature that can present years before damage is apparent. Higher percentage of prior strokes among the AD population may be due to amyloid deposition (38), which repeatedly weakens the vasculature. Diabetes treatment has been shown to lower amyloid load (39,40). However, the effects of controlling A1c values are controversial in dementia (39-42) due to adverse effects of hypoglycemias on the brain. DM+/AD+ subjects in KApSR have lower A1c’s, indicating controlled diabetes, but significantly worse NIHSS following hemorrhagic stroke. This suggests the importance of controlling diabetes, while preventing hypoglycemia, to prevent vascular injury and altered cognition. Patients with AD have higher relative risk and absolute rates of hemorrhagic stroke compared to non-AD controls (10,29,43-45). In our analysis, when controlling for age, the AD population had the worst outcomes.
Several limitations should be considered when interpreting these results, including disparities in: diet (46,47), physical activity (48), socio-economic status (49), race (50), and drug use (51-54), all inherent to the rural Appalachian population (55,56). We used the diagnosis of AD rather than the broader umbrella term dementia. While AD is just one type of dementia (57) it accounts for ~60–70% of the cases (58). Diagnosis of AD and other dementias may be severely underreported or underdiagnosed, as occurs nationally (59-61), but may be heightened due to inherit health disparities in rural Appalachia. Additional cognitive and brain imaging diagnosis tools, such as computed tomography (CT), magnetic resonance imaging (MRI), or positron emission tomography (PET), were not available in this study. Any current medications for comorbidities, including diabetes, were also not available for our analysis. Comorbidities can also affect ischemic stroke, which can also lead to a secondary hemorrhagic stroke. As our analysis only included hemorrhagic strokes coded as primary diagnosis, the effect of diabetes and AD on a secondary hemorrhagic stroke was not analyzed and may be considered a limitation in our study. However, previous studies suggest alternate comorbidities may be associated with secondary hemorrhagic stroke following ischemic stroke. Arboix et al. found hemorrhagic lacunar stroke patients were more like to have hypertension, than diabetes (62) and patients with hemorrhagic stroke following cardioembolic stroke had a higher association with atrial fibrillation (63).
In conclusion, diabetes and AD should be considered as risk factors for an unfavorable outcome following hemorrhagic stroke. Diabetic screening and education for the AD population/caregivers is critical in addressing potential therapeutics to prevent hemorrhagic stroke.
Acknowledgments
Funding: None.
Footnote
Reporting Checklist: The authors have completed the STROBE reporting checklist. Available at https://dx.doi.org/10.21037/atm-21-1451
Data Sharing Statement: Available at https://dx.doi.org/10.21037/atm-21-1451
Conflicts of Interest: All authors have completed the ICMJE uniform disclosure form (available at https://dx.doi.org/10.21037/atm-21-1451). The authors have no conflicts of interest to declare.
Ethical Statement: The authors are accountable for all aspects of the work in ensuring that questions related to the accuracy or integrity of any part of the work are appropriately investigated and resolved. The study was conducted in accordance with the Declaration of Helsinki (as revised in 2013). The study was approved by Kentucky Appalachian Stroke Registry (KApSR) (de-identified data provided under IRB# 13-08058) and individual consent for the retrospective analysis was waived.
Open Access Statement: This is an Open Access article distributed in accordance with the Creative Commons Attribution-NonCommercial-NoDerivs 4.0 International License (CC BY-NC-ND 4.0), which permits the non-commercial replication and distribution of the article with the strict proviso that no changes or edits are made and the original work is properly cited (including links to both the formal publication through the relevant DOI and the license). See: https://creativecommons.org/licenses/by-nc-nd/4.0/.
References
- Hendryx M, Luo J, Borders T. Health Disparities In Appalachia. Health Aff (Millwood) 2017;36:2213. [Crossref] [PubMed]
- Martin B, Wilkerson AH, Patterson G, et al. Need for addressing oral health disparities in rural Appalachia. Health Promot Perspect 2017;7:178-80. [Crossref] [PubMed]
- Holben DH, Pheley AM. Diabetes risk and obesity in food-insecure households in rural Appalachian Ohio. Prev Chronic Dis 2006;3:A82. [PubMed]
- American Diabetes Association. Economic Costs of Diabetes in the U.S. in 2017. Diabetes Care 2018;41:917-28. [Crossref] [PubMed]
- Barbagallo M, Dominguez LJ. Type 2 diabetes mellitus and Alzheimer’s disease. World J Diabetes 2014;5:889-93. [Crossref] [PubMed]
- Cheng D, Noble J, Tang MX, et al. Type 2 diabetes and late-onset Alzheimer’s disease. Dement Geriatr Cogn Disord 2011;31:424-30. [Crossref] [PubMed]
- Li W, Wang T, Xiao S. Type 2 diabetes mellitus might be a risk factor for mild cognitive impairment progressing to Alzheimer’s disease. Neuropsychiatr Dis Treat 2016;12:2489-95. [Crossref] [PubMed]
- Lau LH, Lew J, Borschmann K, et al. Prevalence of diabetes and its effects on stroke outcomes: A meta-analysis and literature review. J Diabetes Investig 2019;10:780-92. [Crossref] [PubMed]
- Alzheimer’s A. Alzheimer’s disease facts and figures. Alzheimers Dement 2019;2019:15.
- Tolppanen AM, Lavikainen P, Solomon A, et al. Incidence of stroke in people with Alzheimer disease: a national register-based approach. Neurology 2013;80:353-8. [Crossref] [PubMed]
- Kochanek KD, Murphy SL, Xu J, et al. Deaths: Final Data for 2017. Hyattsville, MD: National Center for Health Statistics2019 Contract No.: 9.
- Global Health Estimates 2016: Deaths by Cause A, Sex, by Country and by Region, 2000-2016. Geneva, World Health Organization; 2018.
- Kitzman P, Wolfe M, Elkins K, et al. The Kentucky Appalachian Stroke Registry (KApSR). J Stroke Cerebrovasc Dis 2018;27:900-7. [Crossref] [PubMed]
- Dobbs MR, Krishnamohan P, Jicha G, et al. Innovation in Stroke Care Quality: NIH Stroke Scale Change and Shewhart Charts. Qual Manag Health Care 2015;24:135-9. [Crossref] [PubMed]
- Gillett MJ. International Expert Committee report on the role of the A1c assay in the diagnosis of diabetes: Diabetes Care 2009; 32(7): 1327-1334. Clin Biochem Rev 2009;30:197-200. [PubMed]
- Evron JM, Herman WH, McEwen LN. Changes in Screening Practices for Prediabetes and Diabetes Since the Recommendation for Hemoglobin A1c Testing. Diabetes Care 2019;42:576-84. [Crossref] [PubMed]
- American Diabetes Association. Diagnosis and classification of diabetes mellitus. Diabetes Care 2010;33:S62-9. [Crossref] [PubMed]
- Dhamoon MS, Moon YP, Paik MC, et al. Long-term functional recovery after first ischemic stroke: the Northern Manhattan Study. Stroke 2009;40:2805-11. [Crossref] [PubMed]
- Schlegel D, Kolb SJ, Luciano JM, et al. Utility of the NIH Stroke Scale as a predictor of hospital disposition. Stroke 2003;34:134-7. [Crossref] [PubMed]
- Nagel S, Pfaff J, Herweh C, et al. Distal arterial occlusions in patients with mild strokes–is endovascular therapy superior to thrombolysis alone? J Stroke Cerebrovasc Dis 2020;29:104868 [Crossref] [PubMed]
- Dunnett CW. A Multiple Comparison Procedure for Comparing Several Treatments with a Control. J Am Stat Assoc 1955;50:1096-121. [Crossref]
- Virani SS, Alonso A, Benjamin EJ, et al. Heart Disease and Stroke Statistics-2020 Update: A Report From the American Heart Association. Circulation 2020;141:e139-596. [Crossref] [PubMed]
- Koton S, Schneider AL, Rosamond WD, et al. Stroke incidence and mortality trends in US communities, 1987 to 2011. JAMA 2014;312:259-68. [Crossref] [PubMed]
- Singh T, Peters SR, Tirschwell DL, et al. Palliative Care for Hospitalized Patients With Stroke: Results From the 2010 to 2012 National Inpatient Sample. Stroke 2017;48:2534-40. [Crossref] [PubMed]
- Wang G, Zhang Z, Ayala C, et al. Costs of hospitalization for stroke patients aged 18-64 years in the United States. J Stroke Cerebrovasc Dis 2014;23:861-8. [Crossref] [PubMed]
- Lee WC, Christensen MC, Joshi AV, et al. Long-term cost of stroke subtypes among Medicare beneficiaries. Cerebrovasc Dis 2007;23:57-65. [Crossref] [PubMed]
- Pendlebury ST, Klaus SP, Thomson RJ, et al. Methodological Factors in Determining Risk of Dementia After Transient Ischemic Attack and Stroke: (III) Applicability of Cognitive Tests. Stroke 2015;46:3067-73. [Crossref] [PubMed]
- Pendlebury ST, Rothwell PM. Prevalence, incidence, and factors associated with pre-stroke and post-stroke dementia: a systematic review and meta-analysis. Lancet Neurol 2009;8:1006-18. [Crossref] [PubMed]
- Waziry R, Chibnik LB, Bos D, et al. Risk of hemorrhagic and ischemic stroke in patients with Alzheimer disease: A synthesis of the literature. Neurology 2020;94:265-72. [Crossref] [PubMed]
- Bar B, Hemphill JC 3rd. Charlson comorbidity index adjustment in intracerebral hemorrhage. Stroke 2011;42:2944-6. [Crossref] [PubMed]
- Goldstein LB, Samsa GP, Matchar DB, et al. Charlson Index comorbidity adjustment for ischemic stroke outcome studies. Stroke 2004;35:1941-5. [Crossref] [PubMed]
- Lee SH, Kim BJ, Bae HJ, et al. Effects of glucose level on early and long-term mortality after intracerebral haemorrhage: the Acute Brain Bleeding Analysis Study. Diabetologia 2010;53:429-34. [Crossref] [PubMed]
- Exalto LG, Whitmer RA, Kappele LJ, et al. An update on type 2 diabetes, vascular dementia and Alzheimer’s disease. Exp Gerontol 2012;47:858-64. [Crossref] [PubMed]
- Stanciu GD, Bild V, Ababei DC, et al. Link Between Diabetes and Alzheimer’s Disease due to the Shared Amyloid Aggregation and Deposition Involving both Neurodegenerative Changes and Neurovascular Damages. J Clin Med 2020;9:1713. [Crossref] [PubMed]
- Chornenkyy Y, Wang WX, Wei A, et al. Alzheimer’s disease and type 2 diabetes mellitus are distinct diseases with potential overlapping metabolic dysfunction upstream of observed cognitive decline. Brain Pathol 2019;29:3-17. [Crossref] [PubMed]
- Fukazawa R, Hanyu H, Sato T, et al. Subgroups of Alzheimer’s disease associated with diabetes mellitus based on brain imaging. Dement Geriatr Cogn Disord 2013;35:280-90. [Crossref] [PubMed]
- Craft S. The role of metabolic disorders in Alzheimer disease and vascular dementia: two roads converged. Arch Neurol 2009;66:300-5. [Crossref] [PubMed]
- Cordonnier C, Leys D, Dumont F, et al. What are the causes of pre-existing dementia in patients with intracerebral haemorrhages? Brain 2010;133:3281-9. [Crossref] [PubMed]
- Beeri MS, Schmeidler J, Silverman JM, et al. Insulin in combination with other diabetes medication is associated with less Alzheimer neuropathology. Neurology 2008;71:750-7. [Crossref] [PubMed]
- Sonnen JA, Larson EB, Brickell K, et al. Different patterns of cerebral injury in dementia with or without diabetes. Arch Neurol 2009;66:315-22. [Crossref] [PubMed]
- Weinstein G, Davis-Plourde KL, Conner S, et al. Association of metformin, sulfonylurea and insulin use with brain structure and function and risk of dementia and Alzheimer’s disease: Pooled analysis from 5 cohorts. PLoS One 2019;14:e0212293 [Crossref] [PubMed]
- Hatano Y, Araki A, Matsumoto M, et al. Low hemoglobin A1c and low body mass index are associated with dementia and activities of daily living disability among Japanese nursing home residents with diabetes. Geriatr Gerontol Int 2019;19:854-60. [Crossref] [PubMed]
- Chi NF, Chien LN, Ku HL, et al. Alzheimer disease and risk of stroke: a population-based cohort study. Neurology 2013;80:705-11. [Crossref] [PubMed]
- Imfeld P, Bodmer M, Schuerch M, et al. Risk of incident stroke in patients with Alzheimer disease or vascular dementia. Neurology 2013;81:910-9. [Crossref] [PubMed]
- Cook M, Baker N, Lanes S, et al. Incidence of stroke and seizure in Alzheimer’s disease dementia. Age Ageing 2015;44:695-9. [Crossref] [PubMed]
- Lutfiyya MN, Chang LF, Lipsky MS. A cross-sectional study of US rural adults’ consumption of fruits and vegetables: do they consume at least five servings daily? BMC Public Health 2012;12:280. [Crossref] [PubMed]
- Seguin R, Connor L, Nelson M, et al. Understanding barriers and facilitators to healthy eating and active living in rural communities. J Nutr Metab 2014;2014:146502 [Crossref] [PubMed]
- Parks SE, Housemann RA, Brownson RC. Differential correlates of physical activity in urban and rural adults of various socioeconomic backgrounds in the United States. J Epidemiol Community Health 2003;57:29-35. [Crossref] [PubMed]
- The Appalachian Regional Commision. Economic Assessment of Appalachia: An Appalachian Regional Development Inititive Report June 2010. Available online: https://www.arc.gov/publications
- Paskett ED, Fisher JL, Lengerich EJ, et al. Disparities in underserved white populations: the case of cancer-related disparities in Appalachia. Oncologist 2011;16:1072-81. [Crossref] [PubMed]
- Swan LET, Auerbach SL, Ely GE, et al. Family Planning Practices in Appalachia: Focus Group Perspectives on Service Needs in the Context of Regional Substance Abuse. Int J Environ Res Public Health 2020;17:1198. [Crossref] [PubMed]
- Moody L, Satterwhite E, Bickel WK. Substance Use in Rural Central Appalachia: Current Status and Treatment Considerations. Rural Ment Health 2017;41:123-35. [Crossref] [PubMed]
- Goodin A, Talbert J, Freeman PR, et al. Appalachian disparities in tobacco cessation treatment utilization in Medicaid. Subst Abuse Treat Prev Policy 2020;15:5. [Crossref] [PubMed]
- Brown JD, Goodin AJ, Talbert JC. Rural and Appalachian Disparities in Neonatal Abstinence Syndrome Incidence and Access to Opioid Abuse Treatment. J Rural Health 2018;34:6-13. [Crossref] [PubMed]
- Hoogland AI, Hoogland CE, Bardach SH, et al. Health Behaviors in Rural Appalachia. South Med J 2019;112:444-9. [Crossref] [PubMed]
- Griffith BN, Lovett GD, Pyle DN, et al. Self-rated health in rural Appalachia: health perceptions are incongruent with health status and health behaviors. BMC Public Health 2011;11:229. [Crossref] [PubMed]
- Association As. 2019 Alzheimer’s disease fact and figures. Alzheimers Dement 2019;15:321-87. [Crossref]
- WHO, World Health Organisation. (2012). Dementia: A public health priority; World Health Organisation.
- Boustani M, Peterson B, Hanson L, et al. Screening for dementia in primary care: a summary of the evidence for the U.S. Preventive Services Task Force. Ann Intern Med 2003;138:927-37. [Crossref] [PubMed]
- Bradford A, Kunik ME, Schulz P, et al. Missed and delayed diagnosis of dementia in primary care: prevalence and contributing factors. Alzheimer Dis Assoc Disord 2009;23:306-14. [Crossref] [PubMed]
- Kotagal V, Langa KM, Plassman BL, et al. Factors associated with cognitive evaluations in the United States. Neurology 2015;84:64-71. [Crossref] [PubMed]
- Arboix A, García-Eroles L, Massons J, et al. Hemorrhagic lacunar stroke. Cerebrovasc Dis 2000;10:229-34. [Crossref] [PubMed]
- Arboix A, Alió J. Acute cardioembolic stroke: an update. Expert Rev Cardiovasc Ther 2011;9:367-79. [Crossref] [PubMed]