Diagnostic biomarkers and potential drug targets for coronary artery disease as revealed by systematic analysis of lncRNA characteristics
Introduction
Coronary artery disease (CAD) is a complex, multifactorial disease that remains 1 of the most common causes of death (1). Many environmental and genetic factors, including age, smoking behavior, hypertension, dyslipidemia, obesity, diabetes, and family history, contribute to CAD (2-4). However, the number of biomarkers used clinically to predict the incidence of CAD is limited at this stage (5). Currently, coronary angiography is a standard for diagnosing coronary artery diseases; however, these tests sometimes cause significant changes and severe complications, such as vagal reflex, vasospasm, puncture site-related complications and kidney dysfunction. The latency and non-specificity of traditional screening programs also limit their use in clinical practice.
Recent studies have shown that lncRNAs are functional RNA molecules with a length of more than 200 nucleotides, which are generally not translated into proteins, and their expression patterns are either high or low, but they can regulate the expression and function of protein-coding genes through different mechanisms, such as ceRNA model. Many lncRNAs are involved in cardiovascular pathophysiology and have been inferred as potential therapeutic targets. Long noncoding RNAs (lncRNAs) are transcriptional products containing 200 nucleotides and are involved in the etiology of many human diseases through epigenetic, transcriptional, and post-transcriptional regulation (6,7). Interestingly, lncRNAs also appear to participate in the development of cardiovascular disease, including heart failure, cardiac hypertrophy, cardiomyopathy, and myocardial infarction (8). The lncRNAs alter the expression of related proteins through RNA interference, gene silencing, chromatin remodeling, DNA methylation, and other pathways (9). In addition, the function of lncRNAs is usually mediated through regulating microRNAs (miRNAs), which regulate gene expression post-transcriptionally by binding to the 3' untranslated region (UTR) of messenger RNA (mRNA) (10).
The gene S1PR1 is a member of the G protein-coupled receptors group, including S1PR1-5, which are widely expressed in endothelial cells and vascular smooth muscle cells, and is involved in the regulation of various vascular physiological activities. Especially in the cardiovascular system, the proportion of S1PR1 is the highest. The lumen S1PR1 can activate Pertussis toxin-sensitive G proteins, activate Rac1 signaling pathway, and eventually lead to endothelial cytoskeleton rearrangement, thereby promoting endothelial cell migration and proliferation (11). The anti-atherosclerosis effect of high density lipoprotein (HDL) is well known, and HDL is the main carrier of S1P in plasma (12). Binding to HDL, S1P promotes the formation of S1P1-β -Arrestin 2 complex on cell surface and inhibits TNF-α production through S1PR1 signaling. Thus inhibiting the pro-inflammatory response of endothelial cells and vascular smooth muscle cells. In addition, an increasing number of studies have shown that S1PR1 couples to Gi and activates downstream signaling pathways, including the PI3K/AKT, PI3K/Rac, Ras/ERK, NF-κB, and PLC signaling pathways (13,14).
The aim of this study was to investigate the potential function of lncRNA, mRNA expressions in CAD using RNA expression profiles of CAD patients. We systematically analyzed lncRNA and mRNA expression profiles between CAD and healthy patients. Furthermore, a novel algorithm was proposed for the identification of dysregulated lncRNA-mRNA (LRM-CAD) during CAD progression, so as to discover biomarkers as potentially effective therapeutic agents for CAD diagnosis and prognosis. We present the following article in accordance with the TRIPOD reporting checklist (available at https://dx.doi.org/10.21037/atm-21-3276).
Methods
This study consisted of data collection, variance analysis, co-expression module identification, enrichment analysis, feature selection, and classifier construction and validation. The workflow is shown in Figure 1.

RNA expression spectrum
The lncRNA expression profiles of CAD were downloaded from the Gene Expression Omnibus (GEO) database (http://www.ncbi.nlm.nih.gov/geo/) under the number GSE113079 (15) on the Agilent-067406 Human CBC lncRNA + mRNA microarray V4.0 (Agilent Tech., Santa Clara, CA, USA), which was a dataset of 141 samples with whole blood samples from 93 CAD patients and 48 normal controls. In addition, we also downloaded the gene expression profile data sets GSE20681 (16) and GSE64566 (17) between CAD and control of 2 other platforms. The GSE20681 dataset was derived from Agilent-014850 Whole Human Genome Microarray 4x44K G4112F (Agilent Tech. Feature Number version) platform and contained 99 CAD samples and 99 controls. The GSE64566 dataset was derived from Illumina HumanHT-12 V3.0 Expression BeadChip platform (Illumina, San Diego, CA, USA) and contained 26 CAD samples and 20 control samples.
Probe sequences from the 3 datasets were matched to the genome by microarray re-annotation (version GRCh38.p13) to obtain transcript IDs for probe mapping, and each transcript cluster was assigned with an Ensembl gene ID. For transcript clusters with Ensembl gene IDs, we retained the annotation types “lincRNA”, “sense_intronic”, “sense_overlapping”, “antisense”, “processed_transcript”, “3prime_overlapping_ncRNA” clusters were considered as lncRNAs, and clusters with annotation type “protein_coding” were considered to be coding genes (18). Finally, for mRNA, lncRNA expression profiles, probes were mapped to genes/lncRNAs, and when multiple probes were mapped to the same gene/lncRNA, the median expression value was taken as the expression value of the gene/lncRNA.
Differential expression analysis and weighted co-expression network
The R software package Limma (19) was used to screen differentially expressed genes (DEGs) and lncRNAs between CAD and control samples. Firstly, the expression profile of the GSE113079 dataset was analyzed. To obtain biologically different genes, false discovery rate (FDR) <0.05 and double difference were the thresholds to identify DEGs and lncRNAs (DElncRNAs). At the same time, based on DEG and DElncRNAs expression profiles, the R software package ggfortify was used for principal component analysis (PCA) to determine the classification performance of DEG/DElncRNAs for CAD. In addition, for the purpose of better identifying disease-related genes and lncRNAs, we combined the expression profiles of lncRNAs and genes to build a weighted co-expression module. Specifically, RNA expression data profile of genes/lncRNAs was used to evaluate whether the samples and genes/lncRNAs were qualified subjects. Then, we used the weighted gene co-expression network analysis (WGCNA) (20) package in R to construct a scale-free co-expression network for the genes/lncRNAs. The Pearson's correlation matrices and average linkage method were both performed for all pair-wise analyses. Then, a weighted adjacency matrix was constructed using power function Amn=|Cmn|β (Cmn=Pearson’s correlation between gene/lncRNA m and gene/lncRNA n; Amn=adjacency between gene/lncRNA m and gene/lncRNA n). The β was a soft-thresholding parameter emphasizing strong correlations between gene/lncRNAs and to penalize weak correlations. After choosing the power of β, the adjacency was transformed into a topological overlap matrix (TOM) to measure the network connectivity of a gene/lncRNA that was defined as the sum of its adjacency with all other gene/lncRNA for network gene/lncRNA ratio. Then the corresponding dissimilarity (1-TOM) was calculated. To classify gene/lncRNA with similar expression profiles into gene/lncRNA modules, average linkage hierarchical clustering was conducted for the gene/lncRNAs dendrogram, according to the TOM-based dissimilarity measure with a minimum size (gene/lncRNA group) set at 30. The module was further analyzed by calculating the dissimilarity of module eigengene (ME)/lncRNAs, and a cut line was chosen for module dendrogram to merge some modules.
Identification of disease-related co-expression modules
We defined the module associated with the occurrence of CAD as Co-DGL Module, and the genes and lncRNAs in the Co-DGL Module were differentially co-expressed DEG/L. We used 3 methods to determine the correlation between disease and modules. We first calculated the Spearman correlation coefficient of each gene/lncRNA expression in each co-expression module of CAD, and selected the mean value of the correlation coefficient greater than the overall mean value of all modules. Furthermore, the correlation between CAD and each MEs was calculated, and the significantly correlated modules were selected. Finally, the distribution of each MEs in the CAD and control groups was analyzed to determine the modules with significant differences. The intersection sets were taken based on the above 3 methods to identify the key modules for the disease.
Functional enrichment analyses and gene set enrichment analysis
Gene Ontology (GO) and Kyoto Encyclopedia of Genes and Genomes (KEGG) pathway enrichment analysis was performed using the R packages clusterProfiler (21) on genes significantly associated with CAD-related modules. Then, over-represented GO terms in 3 categories (biological processes, molecular function and cellular component) and KEGG pathway were identified. For both analyses, a q-value of <0.05 was considered to denote statistical significance.
The R software package GSVA (22) was used for enrichment analysis of single sample gene sets, and gene expression profiles were used to evaluate the enrichment scores of each sample in different KEGG pathways.
Regulatory interactions between miRNA-mRNA and miRNA-lncRNA
The miRNA-mRNA regulatory relationships were collected from miRanda (23), miRTarBase (24), TargetScan (25) and starBase (26) databases, and 416,312 non-redundant miRNA-mRNA interactions were obtained. The miRNA-lncRNA interactions were retrieved from starBase (26) and miRcode (27) databases, and 295,601 non-redundant miRNA-lncRNA relationships were retained.
LRM-CAD
We speculated that there were LRM-CAD, and dysfunction of which would possibly lead to the occurrence and development of CAD. Based on the competing endogenous RNA (ceRNA) hypothesis (28,29), a candidate LRM-CAD is defined if it satisfies all the following conditions: (I) the miRNA shared by mRNA and lncRNA is significantly enriched (determined by the hypergeometric test, P<0.05); (II) mRNA-lncRNA is in the same disease-related co-expression module. (III) Both mRNA and lncRNA are differentially expressed in CAD samples.
In addition, the R software package GSVA (22) was used for single-sample gene-set enrichment analysis. Gene expression profiles were used to evaluate the gene-set and lncRNAs enrichment scores of each sample in LRM-CAD, and to compare their differences in tumor and control samples and their relationship with the KEGG pathway. Next, the gene sets and lncRNAs in LRM-CAD were mapped to the genome and visualized using the R package OmicCircos (30).
Identification of potential drugs targeting LRM-CAD
To observe potential drugs targeting LRM-CAD, 5,490 drug-gene interaction data were obtained from Drugbank (31) using the method previously described by Peng et al. (32). These proteins and genes in LRM-CAD were mapped to the Search Tool for the Retrieval of Interacting Genes/Proteins (STRING) database (33) to construct drug-protein and protein-protein interaction network (DPPI) and used to define the degree of node of LRM-CAD-related gene set in PPI, T, and drug target gene set. Distance d (S,T) qA the shortest path between S node and T node (where s∈S, is LRM-CAD related gene; t∈T, is a drug target gene), and the calculation method was as follows:
Where ω is the weight of the target gene, if the target gene is a gene in the LRM-CAD related gene set, the calculation method is ω=−ln (D+1), otherwise ω=0.
In addition, a simulated reference distance distribution corresponding to the drug was generated. In short, a group of protein nodes were randomly selected from the network as the simulated drug target, with the same number of nodes as the target size (denoted by R). Then, the distance d (S, R) between the simulated drug targets (representing the simulated drug) and LRM-CAD was calculated. After 10,000 random repeats, the simulated reference distribution was generated. At the same time, the mean and standard deviation (SD) of the μD (S, R) and σ (S, R) reference distribution and the actual observation distance were used to convert to the standardized score, that was, the proximity degree z:
The proximity distribution in the actual network and the random network were evaluated, and the significance P value of the proximity of each drug was calculated. Drugs with a global significance FDR <0.01 were regarded as the final potential drug candidates targeting LRM-CAD.
Evaluation of diagnostic performance and predictive ability of LRM-CAD
For the genes and lncRNAs in LRM-CAD of the training set, the R software package plotROC was used to analyze the expression of each lncRNA and gene, and the Receiver operating characteristic (ROC) analysis of CAD and control sample classification was visualized. For each LRM-CAD, the expression profiles of corresponding genes and LRM-CAD were extracted, and linear discriminant analysis was used to establish a linear model. plotROC was used to analyze each LRM-CAD for ROC analysis and to visualize CAD and control sample classification. The predictive ability of each lncRNA, gene, and LRM-CAD was evaluated using the area under the ROC (AUC). In addition, GSE20681 and GSE124272 served as external validation sets.
Data availability
The discovered Gene Expression data were downloaded from the GEO, numbers GSE64566, GSE20681, and a standardized data set were downloaded. Genome annotation files were download from GENCODE (https://www.gencodegenes.org/human/). The regulatory relationships between miRNA-mRNA were downloaded from miRWalk (http://mirwalk.umm.uni-heidelberg.de/). Regulatory interactions between miRNA-lncRNA were downloaded from starBase (http://starbase.sysu.edu.cn/) and miRcode (http://www.mircode.org/). Drug and protein interaction data were downloaded from Drugbank (https://go.drugbank.com/).
Code availability
We host all the codes involved in the manuscript on GitHub, codes are available at https://github.com/chenzq-star/CADLncRNA.
Ethical statement
The study was conducted in accordance with the Declaration of Helsinki (as revised in 2013).
Statistical analysis
The R package pROC is used for AUC analysis, and the R package ComplexHeatmap is used for heat map drawing. All analysis, except for special instructions, uses default parameters, and data visualization is performed using ggplot2 in version 3.4.3 of R software.
Results
Identification of DEGs/differentially expressed lncRNAs (DElncRNAs) between CAD samples and healthy controls samples
After data standardization and chip reannotation, the expression profiles of 12,826 lncRNAs and 19,586 genes were obtained from the GSE113079 dataset, the expression profiles of 2,307 lncRNAs and 20,045 genes were obtained from the GSE20681 dataset, and the expression profiles of 3,725 lncRNAs and 20,566 genes were obtained from the GSE64566 dataset (Figure 2A,2B). The median expression level of lncRNAs in all samples was generally lower than that of protein-coding genes. In addition, we compared the intersection of lncRNAs in the 3 datasets, and observed that the coincidence degree of the identified lncRNAs was relatively low in the datasets from different platforms (Figure 2C). Therefore, in this study, GSE113079, which was a data set with high coincidence degree of lncRNA with other data sets, was selected as the training set, and GSE20681 and GSE124272 were the verification data sets. A total of 512 DEGs and 308 DElncRNAs were identified in the training set (Figure 2D,2E). The PCA using these differential genes and lncRNA expression profiles showed that the first and second principal components could clearly distinguish CAD samples from control samples (Figure 2F,2G), suggesting that these DEGs and DELs had certain diagnostic performance.

Construction of weighted co-expression network and identification of CAD-related modules
Gene/lncRNAs with similar expression patterns were grouped into modules by means of average linkage hierarchical clustering using the “WGCNA” package in R. In this study, the clustering distance between each sample (Figure 3A) was firstly analyzed, and the distance was similar without outlier samples. A power of β=7 (scale-free R^2=0.88) was the soft threshold to ensure a scale-free network (Figure 3B,3C). Here, a total of 24 modules were identified (Figure 3D). We used 3 methods to determine the correlation between diseases and modules. The Spearman correlation coefficient between gene/lncRNA and disease occurrence in each module as well as the correlation between modules and disease occurrence were calculated (Figure 3E). We selected modules with a mean value of correlation coefficient greater than the overall mean value of all modules. Furthermore, the correlation between CAD and each module was determined to select the module with significant correlation (Figure 3F). Finally, the distribution difference of the feature vectors of each module in CAD and the control group was analyzed (Figure 3G), and the module with significant difference was selected. Based on the above 3 methods, brown, darkgreen, grey60, lightgreen, lightsteelblue1, mediumpurple3, and orangered4 module were identified as the key modules of the disease.
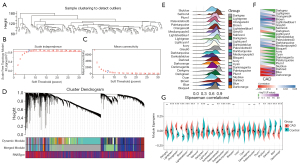
Functional implications of CAD-related modules
To better understand the functional implications of the 7 disease-related modules, GO and KEGG functional enrichment analysis was performed on the genes from the 7 modules. We observed that these 7 modules were enriched in GO terms and KEGG pathways (Figure 4A). Specifically, the brown and orangered4 modules were mainly enriched in cell components; darkgreen was mainly enriched in biological processes and KEGG pathway; grey60 was mainly enriched in cell components and biological processes; lightgreen module was mainly enriched in biological processes and molecular functions; and mediumpurple3 was mainly enriched in biological processes. These results indicated that different modules may be involved in different biological pathways. Similarly, we statistically analyzed the intersection of genes and lncRNAs in these 7 modules with differential genes and lncRNAs (Figure 4B). It was observed that there were 157 up-regulated lncRNAs and 186 up-regulated genes, 30 down-regulated lncRNAs and 67 down-regulated genes in the co-expression module. Further enrichment analysis of these DEGs in KEGG pathways and GO terms showed that these genes were mainly enriched in cytokine and cytokine receptor, salmonella infection, NF-kappa B signaling pathway, interleukin-17 (IL-17) signaling pathway, rheumatoid arthritis, cytokine-cytokine receptor interaction, and tumor necrosis factor (TNF) signaling pathway, legionellosis, NOD-like receptor signaling pathway, and other inflammatory and immune-related pathways (Figure 4C). In addition, they also enriched in G protein-coupled receptor binding, cytokine receptor binding, chemokine receptor binding, cytokine activity, and other immune-related molecular functions (Figure 4D). Inflammation also plays an important role in the occurrence of CAD, and both the innate immune system and adaptive immune system have critical functions in initiation and progression of atherosclerosis. Therefore, these differentially co-expressed genes may be the key genes in CAD.
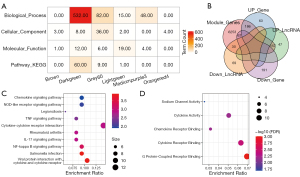
Identification of LRM-CAD and its role in CAD
We employed a new computational method for identifying LRM-CAD of CAD through integrating DEGs/DElncRNA matched expression profiles from disease-associated co-expression modules into a gene expression dataset based on regulatory interactions among mRNAs, lncRNAs, and miRNAs. Firstly, we compared the genomic distances between the DEGs and DElncRNAs in the same co-expression module, and mapped the genes and lncRNAs onto the genome. When lncRNAs were upstream of the genes, they were considered potential cis-regulatory pairs, otherwise they were regarded as trans-regulatory pairs. In this way, 235 cis-regulatory pairs and 25,629 trans-regulatory pairs were obtained. We further analyzed the miRNAs of targeted genes and lncRNAs in each regulation pair, counted the number of miRNAs shared by genes and lncRNAs, and used hypergeometry to evaluate the significance of miRNAs shared by genes and lncRNAs in each regulation pair. Finally, 36 regulatory pairs of significantly shared miRNAs as LRM-CAD (Figure 5A), including 11 lncRNAs and 33 genes, were acquired, and on the whole, trans-regulation was the mainstay. We verified the role of these LRM-CADs in CAD from multiple perspectives. We first calculated the enrichment score of 33 genes (LRM-CAD-GES) and 11 lncRNA enrichment scores (LRM-CAD- LES) (Figure 5B), which revealed significant differences between them in CAD and healthy control samples, especially, lncRNA showed a significant difference in CAD. Furthermore, the single-sample gene set enrichment analysis method was used to calculate the enrichment score of each KEGG pathway, and the correlation between LRM-CAD-GES/LRM-CAD-LES and the enrichment score of each KEGG pathway was evaluated. Here, a total of 13 KEGG pathways were obtained (FDR <0.05, Figure 5C). Specifically, 6 pathways were found to be significantly related to LRM-CAD-GES, including transforming growth factor (TGF)-beta signaling pathway and pathways related to amino acid metabolism, and 7 pathways, including maturity-onset diabetes of the young and multiple pathways related to energy metabolism, were significantly associated with LRM-CAD-LES. These data suggested that the occurrence of CAD may be associated with some metabolic disorders.
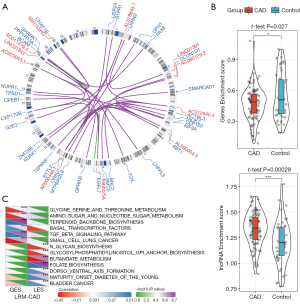
LRM-CAD as a diagnostic biomarker for CAD
Considering the differences between LRM-CAD-GES and LRM-CAD-LES in CAD, we evaluated the CAD classification performance of each LRM-CAD and single LRM-CAD gene and single LRM-CAD lncRNA, respectively. For each LRM-CAD lncRNA, their expression level showed a high diagnostic performance for CAD classification ROC (Figure 6A). Similarly, for each LRM-CAD gene, they also showed a strong diagnostic performance (Figure 6B). Furthermore, linear discriminant analysis was performed to classify and predict each LRM-CAD and ROC analysis. It was observed that compared with a single lncRNA or gene, these LRM-CAD had higher classification performance (Figure 6C) with an average AUC of 0.958, suggesting that these LRM-CAD may be a potential diagnostic marker of CAD.
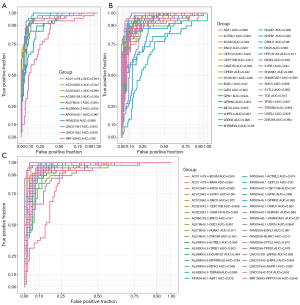
Identification of potential drugs targeting LRM-CAD
To further identify the therapeutic drugs for LRM-CAD, we obtained 5,490 drug-protein interaction data from the Drugbank database, and constructed a drug-protein interaction network using the protein interaction information in the STRING database. The genes in LRM-CAD were mapped to the network to calculate the closeness of the drug to LRM-CAD, and a random network was constructed using stochastic simulation as a background. The proximity distribution of the final drug to LRM-CAD was smaller than that of the random background drug to LRM-CAD (Figure 7A). We selected drugs with a global FDR <0.05, which yielded 3 drugs (Table 1). These 3 drugs directly interact with the S1PR1 gene in LRM-CAD. We used molecular docking methods to verify the binding ability of S1PR1 with DB09105, DB12371, and DB12612. Considering that DB09105 was a protein molecule, we used the 2 small molecule compounds DB12371 and DB12612 for molecular docking. Both DB12371 and DB12612 showed high docking scores, the docking score of compound DB12612 and S1PR1 reached -9.4 kcal/mol (Figure 7B), and DB12371, which had a docking score of -11.1 kcal/mol (Figure 7C). To a certain extent, these data indicated that these 2 compounds and S1PR1 could be relatively tightly combined and had a potential biological activity.
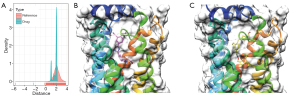

Full table
Verification of diagnostic performance of S1PR1-AC012640.4
The gene S1PR1 may be the direct target of DB09105, DB12371, and DB12612. Here, external data sets GSE20681 and GSE64566 were used as validation sets to evaluate the diagnostic performance of S1PR1 and AC012640.4. The expression profiles of S1PR1 and AC012640.4 were obtained from the GSE20681 data set. The expression level of S1PR1 was used to predict CAD, and the ROC analysis revealed an AUC of 0.773 (Figure 8A). The expression level of AC012640.4 was used to predict CAD, and ROC analysis showed that the AUC was 0.755 (Figure 8B). Combining predictive CAD with S1PR1-AC012640.4, ROC analysis showed an AUC of 0.79 (Figure 8C). In addition, only S1PR1 was detected in the GSE64566 dataset, thus, we also analyzed the diagnostic performance of S1PR1 in the GSE64566 dataset. The ROC showed that S1PR1 expression still had a strong diagnostic performance (AUC =0.754, Figure 8D). These results indicated that S1PR1, AC012640.4 and S1PR1-AC012640.4 could effectively distinguish CAD from control, and that these genes and lncRNAs may be reliable biomarkers for CAD specific diagnosis.
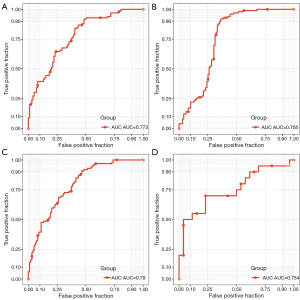
Discussion
In this study, we obtained a relatively large sample (93 CAD patients and 48 healthy controls) of whole-transcriptome lncRNA and mRNA expression data by high-throughput microarray screening. To determine the function of DElncRNAs, 7 CAD-related modules were identified by WGCNA and analyzed for GO and KEGG pathways. It was found that some differentially expressed mRNAs were involved in inflammatory cytokine and immune cell secretion pathways. Proinflammatory cytokines and chemokines have been reported to be involved in all stages of atherosclerotic lesion development. In addition, 36 regulatory pairs of significantly shared by miRNAs were considered as LRM-CAD, including 11 lncRNAs and 33 genes. The ROC analysis demonstrated that LRM-CAD had a higher classification performance. We screened 3 potential therapeutic drugs DB09105, DB12371 and DB12612 by drug-target gene interaction network, and there was a direct interaction with the S1PR1 gene in LRM-CAD. External data sets verified that S1PR1-lncRNA AC012640.4 could effectively distinguish CAD from control.
As a leading cause of death worldwide, and early prevention of CAD can reduce morbidity and mortality. Therefore, this study was designed to identify new biomarkers to improve the prediction and treatment of CAD. Many studies have confirmed that circulating miRNAs can be used as disease markers in blood of cardiovascular diseases such as acute myocardial infarction (AMI) and heart failure (34). Exosomal microRNAs have been reported to play an important role in the progress of CAD. For example, Exosomal microRNA-25-3p inhibits coronary vascular endothelial cell inflammation (35), Exosomal microRNA-21, microRNA-126, and PTEN are novel biomarkers for diagnosis of acute coronary syndrome (36). Similar to miRNAs and Exosomal microRNAs, lncRNAs can also be detected in blood and could also serve as biomarkers (37). In a study by Vausort et al., 5 lncRNAs, including AHIF, ANRIL, KCNQ1QT1, MIAT, and MALAT1, were detected in peripheral blood mononuclear cell (PBMC) of 414 AMI patients and 86 control patients (38). The lncRNAs KCNQ1QT1, AHIF, and MALAT1 in AMI patients were found to be higher than those in the control group, and ANRIL was lower than control group. Meanwhile, ANRIL, KCNQ1OT1, MIAT, and MALAT1 were lower in patients with ST segment elevation MI than in those without. Finally, ANRIL and KCNQ1QT1 were considered capable of predicting left ventricular dysfunction after AMI. Yang et al. detected the expression of lncRNA in 20 patients with CAD and 20 controls (39). Serum lncRNA AC100865.1, monocyte LncPPARd (40), and OTTHUMT00000387022 were detected as markers for the diagnosis of CAD. In this study, bioinformatics study showed that S1PR1-AC012640.4 could effectively distinguish CAD from healthy groups and serve as a biomarker for CAD specific diagnosis.
Overexpression of fibroblast-specific S1PR1 in mouse hearts has been shown to increase cardiac tissue hypertrophy and fibrosis, accompanied by up-regulation of signal transduction and transcriptional activator 3 (STAT3) signal transduction and interleukin-6 (IL-6) production (41). The drug-protein interaction network showed that both DB12371 and DB12612 could bind to S1PR1 well, suggesting that DB12371 and DB12612 might be potential drugs targeting S1PR1.
Although we analyzed and validated the abnormal expression and functional role of genes in CAD through bioinformatics with multiple data coalitions, some limitations of this study should be noted. Firstly, the sample lacked some clinical follow-up information, therefore we did not consider factors such as the presence of patient comorbidities in distinguishing the biomarkers. Secondly, the results were obtained only by bioinformatics analysis, which was insufficient, and experimental validation is needed to confirm these results. Therefore, further genetic and experimental studies with larger sample sizes and experimental validation are required.
Conclusions
In this study, we systematically analyzed gene expression patterns in CAD and conducted a large-scale genome-wide study on RNA expression profiles to identify gene modules closely related to CAD. Through disease association network mining, we found 3 potential drugs in CAD, providing targets and reference for clinicians and biological experimentalists.
Acknowledgments
Funding: None.
Footnote
Reporting Checklist: The authors have completed the TRIPOD reporting checklist. Available at https://dx.doi.org/10.21037/atm-21-3276
Conflicts of Interest: All authors have completed the ICMJE uniform disclosure form (available at https://dx.doi.org/10.21037/atm-21-3276). The authors have no conflicts of interest to declare.
Ethical Statement: The authors are accountable for all aspects of the work in ensuring that questions related to the accuracy or integrity of any part of the work are appropriately investigated and resolved. The study was conducted in accordance with the Declaration of Helsinki (as revised in 2013).
Open Access Statement: This is an Open Access article distributed in accordance with the Creative Commons Attribution-NonCommercial-NoDerivs 4.0 International License (CC BY-NC-ND 4.0), which permits the non-commercial replication and distribution of the article with the strict proviso that no changes or edits are made and the original work is properly cited (including links to both the formal publication through the relevant DOI and the license). See: https://creativecommons.org/licenses/by-nc-nd/4.0/.
References
- Benjamin EJ, Virani SS, Callaway CW, et al. Heart Disease and Stroke Statistics-2018 Update: A Report From the American Heart Association. Circulation 2018;137:e67-e492. [Crossref] [PubMed]
- Chiu MH, Heydari B, Batulan Z, et al. Coronary artery disease in post-menopausal women: are there appropriate means of assessment? Clin Sci (Lond) 2018;132:1937-52. [Crossref] [PubMed]
- Madhavan MV, Gersh BJ, Alexander KP, et al. Coronary Artery Disease in Patients ≥80 Years of Age. J Am Coll Cardiol 2018;71:2015-40. [Crossref] [PubMed]
- Zhang HW, Jin JL, Cao YX, et al. Heart-type fatty acid binding protein predicts cardiovascular events in patients with stable coronary artery disease: a prospective cohort study. Ann Transl Med 2020;8:1349. [Crossref] [PubMed]
- Wykrzykowska JJ, Garcia-Garcia HM, Goedhart D, et al. Differential protein biomarker expression and their time-course in patients with a spectrum of stable and unstable coronary syndromes in the Integrated Biomarker and Imaging Study-1 (IBIS-1). Int J Cardiol 2011;149:10-6. [Crossref] [PubMed]
- Batista PJ, Chang HY. Long noncoding RNAs: cellular address codes in development and disease. Cell 2013;152:1298-307. [Crossref] [PubMed]
- Yan HX, Du J, Fu J, et al. Microarray-based differential expression profiling of long noncoding RNAs and messenger RNAs in formalin-fixed paraffin-embedded human papillary thyroid carcinoma samples. Transl Cancer Res 2019;8:439-51. [Crossref]
- Schunkert H, König IR, Kathiresan S, et al. Large-scale association analysis identifies 13 new susceptibility loci for coronary artery disease. Nat Genet 2011;43:333-8. [Crossref] [PubMed]
- Amaral PP, Dinger ME, Mercer TR, et al. The eukaryotic genome as an RNA machine. Science 2008;319:1787-9. [Crossref] [PubMed]
- Shukla GC, Singh J, Barik S. MicroRNAs: Processing, Maturation, Target Recognition and Regulatory Functions. Mol Cell Pharmacol 2011;3:83-92. [PubMed]
- Singleton PA, Dudek SM, Chiang ET, et al. Regulation of sphingosine 1-phosphate-induced endothelial cytoskeletal rearrangement and barrier enhancement by S1P1 receptor, PI3 kinase, Tiam1/Rac1, and alpha-actinin. Faseb J 2005;19:1646-56. [Crossref] [PubMed]
- Karliner JS. Sphingosine kinase and sphingosine 1-phosphate in the heart: a decade of progress. Biochim Biophys Acta 2013;1831:203-12. [Crossref] [PubMed]
- Park SJ, Kim JM, Kim J, et al. Molecular mechanisms of biogenesis of apoptotic exosome-like vesicles and their roles as damage-associated molecular patterns. Proc Natl Acad Sci U S A 2018;115:E11721-30. [Crossref] [PubMed]
- Zervantonakis IK, Iavarone C, Chen HY, et al. Systems analysis of apoptotic priming in ovarian cancer identifies vulnerabilities and predictors of drug response. Nat Commun 2017;8:365. [Crossref] [PubMed]
- Li L, Wang L, Li H, et al. Characterization of LncRNA expression profile and identification of novel LncRNA biomarkers to diagnose coronary artery disease. Atherosclerosis 2018;275:359-67. [Crossref] [PubMed]
- Elashoff MR, Wingrove JA, Beineke P, et al. Development of a blood-based gene expression algorithm for assessment of obstructive coronary artery disease in non-diabetic patients. BMC Med Genomics 2011;4:26. [Crossref] [PubMed]
- Vacca M, Di Eusanio M, Cariello M, et al. Integrative miRNA and whole-genome analyses of epicardial adipose tissue in patients with coronary atherosclerosis. Cardiovasc Res 2016;109:228-39. [Crossref] [PubMed]
- Birney E, Stamatoyannopoulos JA, Dutta A, et al. Identification and analysis of functional elements in 1% of the human genome by the ENCODE pilot project. Nature 2007;447:799-816. [Crossref] [PubMed]
- Ritchie ME, Phipson B, Wu D, et al. limma powers differential expression analyses for RNA-sequencing and microarray studies. Nucleic Acids Res 2015;43:e47 [Crossref] [PubMed]
- Langfelder P, Horvath S. WGCNA: an R package for weighted correlation network analysis. BMC Bioinformatics 2008;9:559. [Crossref] [PubMed]
- Yu G, Wang LG, Han Y, et al. clusterProfiler: an R package for comparing biological themes among gene clusters. OMICS 2012;16:284-7. [Crossref] [PubMed]
- Hanzelmann S, Castelo R, Guinney J. GSVA: gene set variation analysis for microarray and RNA-seq data. BMC Bioinformatics 2013;14:7. [Crossref] [PubMed]
- Miranda KC, Huynh T, Tay Y, et al. A pattern-based method for the identification of MicroRNA binding sites and their corresponding heteroduplexes. Cell 2006;126:1203-17. [Crossref] [PubMed]
- Huang HY, Lin YC, Li J, et al. miRTarBase 2020: updates to the experimentally validated microRNA-target interaction database. Nucleic Acids Res 2020;48:D148-D54. [PubMed]
- Lewis BP, Burge CB, Bartel DP. Conserved seed pairing, often flanked by adenosines, indicates that thousands of human genes are microRNA targets. Cell 2005;120:15-20. [Crossref] [PubMed]
- Li JH, Liu S, Zhou H, et al. starBase v2.0: decoding miRNA-ceRNA, miRNA-ncRNA and protein-RNA interaction networks from large-scale CLIP-Seq data. Nucleic Acids Res 2014;42:D92-7. [Crossref] [PubMed]
- Jeggari A, Marks DS, Larsson E. miRcode: a map of putative microRNA target sites in the long non-coding transcriptome. Bioinformatics 2012;28:2062-3. [Crossref] [PubMed]
- Salmena L, Poliseno L, Tay Y, et al. A ceRNA hypothesis: the Rosetta Stone of a hidden RNA language? Cell 2011;146:353-8. [Crossref] [PubMed]
- Tay Y, Rinn J, Pandolfi PP. The multilayered complexity of ceRNA crosstalk and competition. Nature 2014;505:344-52. [Crossref] [PubMed]
- Hu Y, Yan C, Hsu CH, et al. OmicCircos: A Simple-to-Use R Package for the Circular Visualization of Multidimensional Omics Data. Cancer Inform 2014;13:13-20. [Crossref] [PubMed]
- Wishart DS, Feunang YD, Guo AC, et al. DrugBank 5.0: a major update to the DrugBank database for 2018. Nucleic Acids Res 2018;46:D1074-82. [Crossref] [PubMed]
- Peng Y, Yuan M, Xin J, et al. Screening novel drug candidates for Alzheimer's disease by an integrated network and transcriptome analysis. Bioinformatics 2020;36:4626-32. [Crossref] [PubMed]
- Szklarczyk D, Gable AL, Lyon D, et al. STRING v11: protein-protein association networks with increased coverage, supporting functional discovery in genome-wide experimental datasets. Nucleic Acids Res 2019;47:D607-13. [Crossref] [PubMed]
- Shin VY, Chu KM. MiRNA as potential biomarkers and therapeutic targets for gastric cancer. World J Gastroenterol 2014;20:10432-9. [Crossref] [PubMed]
- Yao Y, Sun W, Sun Q, et al. Platelet-Derived Exosomal MicroRNA-25-3p Inhibits Coronary Vascular Endothelial Cell Inflammation Through Adam10 via the NF-κB Signaling Pathway in ApoE(-/-) Mice. Front Immunol 2019;10:2205. [Crossref] [PubMed]
- Ling H, Guo Z, Shi Y, et al. Serum Exosomal MicroRNA-21, MicroRNA-126, and PTEN Are Novel Biomarkers for Diagnosis of Acute Coronary Syndrome. Front Physiol 2020;11:654. [Crossref] [PubMed]
- Reis EM, Verjovski-Almeida S. Perspectives of Long Non-Coding RNAs in Cancer Diagnostics. Front Genet 2012;3:32. [Crossref] [PubMed]
- Vausort M, Wagner DR, Devaux Y. Long noncoding RNAs in patients with acute myocardial infarction. Circ Res 2014;115:668-77. [Crossref] [PubMed]
- Yang Y, Cai Y, Wu G, et al. Plasma long non-coding RNA, CoroMarker, a novel biomarker for diagnosis of coronary artery disease. Clin Sci (Lond) 2015;129:675-85. [Crossref] [PubMed]
- Cai Y, Yang Y, Chen X, et al. Circulating "LncPPARδ" From Monocytes as a Novel Biomarker for Coronary Artery Diseases. Medicine (Baltimore) 2016;95:e2360 [Crossref] [PubMed]
- Ohkura SI, Usui S, Takashima SI, et al. Augmented sphingosine 1 phosphate receptor-1 signaling in cardiac fibroblasts induces cardiac hypertrophy and fibrosis through angiotensin II and interleukin-6. PLoS One 2017;12:e0182329 [Crossref] [PubMed]
(English Language Editor: J. Jones)