Identifying unmet non-COVID-19 health needs during the COVID-19 outbreak based on social media data: a proof-of-concept study in Wuhan city
Introduction
Beyond its direct harm to human health or life, the coronavirus disease 2019 (COVID-19) outbreak had quickly occupied medical resources and led to a crisis in the management of non-COVID-19 diseases (1,2). Non-COVID-19 diseases refer to the acute or chronic diseases not directly caused by SARS-CoV-2 infection. During the COVID-19 outbreak, the healthcare services for non-COVID-19 diseases had decreased almost globally (3), and excess non-COVID-19 deaths had been reported across countries (4-7). Therefore, a viable strategy for the surveillance of non-COVID-19 diseases was necessary to inform the timely management of unmet health needs. Besides, in the post-COVID-19 era, it is essential to understand the characteristics of unmet non-COVID-19 health needs during the COVID-19 outbreak, which would help establish a more resilient healthcare system for the future outbreak (1).
Health needs are essential to prevent dysfunction or death. Herein, non-COVID-19 health needs included the perceived needs for health services experienced by non-COVID-19 patients. Unlike the morbidity or mortality that could be recorded by health statistics (4,6), the nature and magnitude of non-COVID-19 health needs are relatively unmeasurable. Therefore, this observational study based on social media data might serve as an alternative solution to profile health needs. Sina Weibo is the largest microblogging platform in China. During the COVID-19 outbreak, the increase in public engagement and message propagation had made Weibo an available tool for disease surveillance and health statistics (8,9). Patients with unmet health needs had also sought help on Weibo. In this proof-of-concept study, we aimed to explore firstly, whether social media could help surveil the unmet non-COVID-19 health needs; secondly, whether the social media data enabled to characterize the non-COVID-19 urgent and emergent health needs during the COVID-19 outbreak in Wuhan city. The better knowledge of unmet non-COVID-19 health needs might therefore inspire a more flexible and adaptable healthcare system. We presented the study in accordance with the STROBE reporting checklist (available at https://dx.doi.org/10.21037/atm-21-1769).
Methods
Study design and data sources
This was an observational study, aiming to explore whether social media could help surveil and understand the unmet non-COVID-19 health needs during the 3 months of the COVID-19 outbreak. The study period was set from December 31, 2019, to March 31, 2020. Sina Weibo was retrieved from Weibo.com. According to the official data, the active users of Sina Weibo reached 550 million by the first quarter of 2020. Registered users could opt-in to disseminate messages, pictures, videos, and links publicly on this platform. Users could use hashtags to create specific topics on Weibo, or create super-topics to join content-based virtual communities. A total of 10 most popular topics of non-COVID-19 information and one super-topic of COVID-19 were identified. The COVID-19 super-topic covered both COVID-19 and non-COVID-19 Weibo. Although the identities of Weibo users were unknown, patients seeking help on Weibo usually disclosed their demographic and disease information to gain trust and attention. Wuhancrisis.com was an external website collecting and preserving original Weibo from the COVID-19 super-topic.
The epidemiological data of COVID-19 in Wuhan city was obtained from the Health Commission of Hubei Province. The reported data included the daily number of newly confirmed COVID-19 cases and deaths. The study was conducted in accordance with the Declaration of Helsinki (as revised in 2013). All data in this study were publicly available and de-identified. No patient consent was required, and no ethical approval was obtained.
Weibo retrieval, screening, and data extraction
A comprehensive search was performed to identify all Weibo of unmet health needs in Wuhan city. Health needs were defined herein as the perceived needs for health services experienced by individuals that had not been met due to the shortage of healthcare resources during the COVID-19 outbreak. All available Weibo from 10 non-COVID-19 topics and one COVID-19 super-topic were retrieved. Moreover, all relevant Weibo were identified using a list of 114 medical keywords and four cancer keywords. All Weibo and posted figures were retrieved between March 2020 and July 2020 through the Sina Weibo API using a Python-based tool (9), in which the geographical location was set in Wuhan city, Hubei province. Additional Weibo were identified from Wuhancrisis.com, and all Weibo forwarded by the retrieved Weibo.
After removing duplicates, the retrieved Weibo were strictly screened based on the inclusion criteria:
- Weibo should contain clearly identified health needs, with primary diseases or conditions and care needs;
- Perceived health needs should be urgent or emergent;
- Perceived health needs were not fulfilled due to the shortage of healthcare resources.
The exclusion criteria were set as follows:
- Weibo describing perceived needs without seeking help or were not of unmet needs;
- Self-contained symptoms without the risk of dysfunction or death, such as toothache;
- Weibo for fundraising purposes, rather than for seeking health services;
- Suspected SARS-CoV-2 infection;
- Needs for medicines;
- Unmet needs merely due to the lockdown of public transportation;
- Unmet needs merely due to the patient’s fear of infection in hospitals;
- Weibo posting time outside the defined period.
After screening, data were extracted from eligible Weibo, including the age, sex, disease, care needs, post time, geographical location, date of onset of symptoms or perceived needs. Data were collected in Excel for the annotation and classification of diseases and needs.
Epidemiological trends and lag correlations
The daily numbers of newly confirmed COVID-19 cases, deaths, and non-COVID-19 Weibo were separately plotted and compared to determine how they matched up with each other throughout the study period. For the lag correlation, the data between January 16 and March 18, 2020, were used because the diagnostic criteria of COVID-19 were firstly defined on January 16 based on epidemiology, clinical manifestations, and laboratory results. On March 18, the number of COVID-19 cases dropped to zero. Lag Spearman correlations were conducted with a 16-day offset at most to find out when the best match occurs between COVID-19 cases, deaths, and non-COVID-19 health needs. The standard linear regressions were used to model the association between COVID-19 cases, deaths, and non-COVID-19 health needs with the time lag offset. The abrupt increase in the number of new cases and deaths on February 12 was due to a change in the diagnostic criteria for COVID-19 in Wuhan city based on epidemiology and clinical manifestations. Data of new cases and deaths on February 12 were replaced with means of data on February 11 and 13 to facilitate the regression modeling.
Diseases and care needs
The actual number of non-COVID-19 patients with unmet health needs was estimated based on the observed number of COVID-19 patients seeking help on Weibo and the official number of COVID-19 patients. Patient’s primary diseases or conditions were annotated and categorized according to the International Classification of Diseases 11th Revision (ICD-11) for Mortality and Morbidity Statistics, 2018 version. Primary diseases or conditions were considered as the diagnosis that occasioned the health needs.
The care needs were extracted from Weibo and divided into two main entities: acute care and cancer care. Acute care included acute surgery care, urgent care, emergency care, critical care, hemodialysis, and blood transfusion. Acute surgery referred to the surgical management of urgent conditions, such as acute abdominal surgery, diabetic foot syndrome, retinal detachment, and traumatic injury. The urgent care delivered timely healthcare services to prevent diseases from deterioration, which would worsen to life-threatening conditions or severe comorbidities if without urgent interventions. The emergency care was for sudden and critical conditions, such as heart attacks and strokes. Critical care involved the life support and comprehensive care of critically ill patients, such as respiratory failure and hepatic failure. Blood transfusion encompassed the transfusion of red blood cells, plasma, and platelets.
Cancer care included standard cancer care, cancer surgery, chemotherapy, radiotherapy, and palliative cancer care. Standard cancer care referred to the start of standard treatment for a newly identified cancer. The needs for cancer surgery, chemotherapy, and radiotherapy were identified through the patient’s treatment plans disclosed on Weibo. Palliative cancer care focused on relieving the symptoms and sufferings of end-staged cancers, such as pain relief and nutritional support.
The delay in seeking help referred to the time interval from the onset of symptoms or perceived needs to the post date of Weibo. The delay in seeking help was analyzed by different care needs. The difference in delays between acute care and cancer care needs was assessed using the Mann-Whitney test; the differences between different types of care needs within each entity were assessed using the Kruskal-Wallis test.
Statistical analysis
Categorical variables were presented as counts with percentages. Continuous variables were expressed as medians with interquartile range (IQR) after the normality test. The epidemiological trends were explored by the lag Spearman correlations and modeled by the standard linear regressions, as illustrated above. The difference of continuous variables in different groups was verified by the Mann-Whitney or Kruskal-Wallis tests. All tests and P values were two-sided. A P value less than 0.05 was considered significant. All calculations and statistics were performed in Microsoft Excel and SPSS (version 22, IBM, USA).
Results
A total of 114,795 Weibo was retrieved after duplicates were removed. After the full-text screening, 229 Weibo seeking help for non-COVID-19 diseases during the COVID-19 outbreak in Wuhan were included in the analysis (Figure 1).
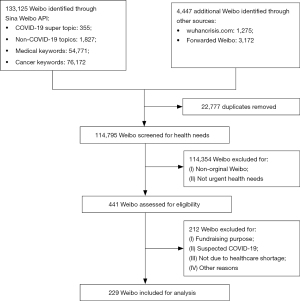
For the epidemiological characteristics of COVID-19, the time trends of daily cases and deaths were shown in Figure 2, which demonstrates the single-wave outbreak. The number of daily cases and deaths increased from January 16, peaked on February 12, and gradually fell to zero cases and single-digit deaths at the end of March. The peak of the outbreak on February 12 recorded 13,436 new cases and 216 deaths.
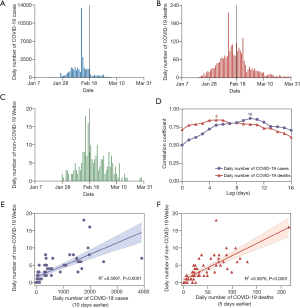
The time trend of non-COVID-19 Weibo was similar to the COVID-19 outbreak, except that the time lag was observed, probably reflecting the delay between the healthcare resources occupancy by COVID-19 and non-COVID-19 health needs. We conducted lag Spearman correlations to find the highest correlation between COVID-19 cases at 10 days earlier and non-COVID-19 Weibo (correlation coefficient r =0.87, P<0.001), indicating that it took 10 days for the adverse effects of COVID-19 to propagate to non-COVID-19 diseases. At a 10-day lag, there was a linear positive association between the daily number of COVID-19 cases and non-COVID-19 Weibo (slope β =3.3, per 1,000 cases increase; coefficient of determination R2 =0.507, P<0.001). The lag correlation between COVID-19 deaths and non-COVID-19 Weibo was similar, but with a shorter lag time of 5 days (r =0.849, P<0.001). A linear positive association was also found between the daily number of COVID-19 deaths at 5 days earlier and non-COVID-19 Weibo (β =7.0, per 100 deaths increase; R2 =0.588, P<0.001).
Weibo from the COVID-19 super-topic were used for data estimation. Among all 1275 Weibo from the COVID-19 super-topic, about 925 were of COVID-19 needs, and 128 were of non-COVID-19 needs. The official number of COVID-19 cases in Wuhan city was 50,340, and so we estimated that the actual number of non-COVID-19 patients with urgent and emergent health needs was about 6,966 (Table 1).
Table 1
Disease | Observed cases | Estimated cases | Frequency (%)‡ |
---|---|---|---|
(I) Non-neoplastic diseases | |||
Infectious diseases | 1 | 30 | 0.4 |
Hematological diseases | 6 | 183 | 2.6 |
Immune diseases | 2 | 61 | 0.9 |
Mental disorders | 2 | 61 | 0.9 |
Nervous system diseases | 30 | 913 | 13.1 |
Stroke | 29 | 882 | 12.7 |
Visual system diseases | 2 | 61 | 0.9 |
Circulatory system diseases | 14 | 426 | 6.1 |
Acute coronary syndrome | 6 | 183 | 2.6 |
Respiratory system diseases | 14 | 426 | 6.1 |
Pneumonia§ | 9 | 274 | 3.9 |
Digestive system diseases | 20 | 608 | 8.7 |
Hepatic failure | 8 | 243 | 3.5 |
Skin diseases | 1 | 30 | 0.4 |
Genitourinary system diseases | 12 | 365 | 5.2 |
Kidney failure | 9 | 274 | 3.9 |
Pregnancy | 3 | 91 | 1.3 |
Developmental anomalies | 2 | 61 | 0.9 |
Injuries | 8 | 243 | 3.5 |
All | 106 | 3,224 | 46.3 |
(II) Neoplasms | |||
Brain tumors | 8 | 243 | 3.5 |
Glioma | 7 | 213 | 3.1 |
Retinoblastoma | 1 | 30 | 0.4 |
Head and neck cancer | 6 | 183 | 2.6 |
Gastrointestinal cancer | 28 | 852 | 12.2 |
Esophageal cancer | 5 | 152 | 2.2 |
Stomach cancer | 5 | 152 | 2.2 |
Colorectal cancer | 7 | 213 | 3.1 |
Liver cancer | 5 | 152 | 2.2 |
Bile duct cancer | 5 | 152 | 2.2 |
Lung cancer | 21 | 639 | 9.2 |
Bone cancer | 1 | 30 | 0.4 |
Breast cancer | 11 | 335 | 4.8 |
Urogenital cancer | 15 | 456 | 6.6 |
Ovarian cancer | 6 | 183 | 2.6 |
Hematological malignancy | 28 | 852 | 12.2 |
Leukemia | 20 | 608 | 8.7 |
Mediastinal cancer, unspecified | 1 | 30 | 0.4 |
Malignant pheochromocytoma | 1 | 30 | 0.4 |
Cancer, unspecified | 2 | 61 | 0.9 |
All | 123 | 3,742 | 53.7 |
†, diseases were categorized according to ICD-11 for Mortality and Morbidity Statistics, 2018 version. ‡, sum of frequencies might not sum to 100% due to data rounding. §, patients reporting pneumonia were excluded from COVID-19, with negative tests or computed tomography. COVID-19, coronavirus disease 2019.
Among patients identified from non-COVID-19 Weibo, the sex ratio was skewed towards males: 55.5% were males, 39.3% were females, and 5.2% were unknown (Figure 3). The median age of patients was 56 years, and the IQR was between 38 and 63 years. The age distribution was skewed towards older age groups, with 48.0% of patients between 51 and 70. Only a minor proportion of patients (4.8%) were younger than 20 years old.
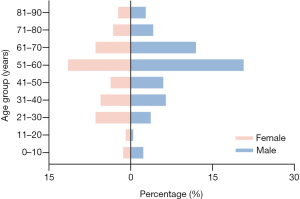
Patients were suffered from diverse diseases (Table 1). Of non-neoplastic diseases, the most common entities included nervous system diseases (13.1%), digestive system diseases (8.7%), respiratory system diseases (6.1%), and circulatory system diseases (6.1%). Stroke patients (12.7%) were most likely to have unmet needs and sought help on Weibo. Gastrointestinal cancer (12.2%) and hematological malignancy (12.2%) were the most common types of neoplasms, followed by lung cancer (9.2%), urogenital cancer (6.6%), breast cancer (4.8%), brain tumors (3.5%), and head and neck cancer (2.6%). Leukemia (8.7%) was the most common hematological malignancy with unmet needs. Patients with rare cancers were also found.
In terms of acute care needs (Figure 4A), most patients (17.0%) required critical care, especially for digestive system diseases (4.8%), respiratory system diseases (3.9%), and neoplasms (3.5%). The second most needed care was urgent care (14.4%). A large proportion of patients seeking urgent care were cancer patients (3.5%) who required catheter or line care for peripherally inserted central catheter (PICC) or implantable venous access port (PORT). Fourteen percent of patients needed emergency care, of which 11.8% had a stroke, and 2.2% had the acute coronary syndrome. The following need was acute surgical care (10.5%), with 2.2% of patients needed acute abdominal surgery and 2.2% of patients required trauma surgery. A total of 5.2% of patients required blood transfusion, and 4.8% had hematological diseases or malignancies. 4.4% of patients were in urgent need of hemodialysis.
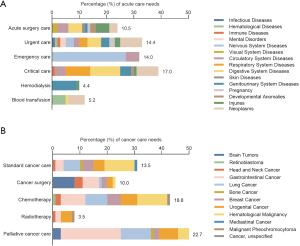
Most cancer patients (22.7%) required palliative cancer care (Figure 4B), especially gastrointestinal cancer (9.6%), lung cancer (4.8%), and urogenital cancer (3.1%). As many as 18.8% of patients required chemotherapy, and the most common types of cancer were hematological malignancy (4.8%), gastrointestinal cancer (3.9%), and lung cancer (3.5%). The standard cancer care was demanded by 13.5% of patients, of which 4.8% had hematological malignancies. Finally, 10.0% of patients needed cancer surgery, while 3.5% of patients required radiotherapy.
The median delay in seeking help on Weibo was 3 days (IQR, 1–15 days) for patients requiring acute care (Figure 5A). The delay was shorter for patients in need of emergency care, with a median of 1.25 days (IQR, 1–2 days). By contrast, the median delay in seeking cancer care on Weibo was 18.5 days (IQR, 6–30 days) (Figure 5B), which was longer than the delay in seeking acute care (P<0.001). There was no significant difference in the delay in seeking help between different types of cancer care.
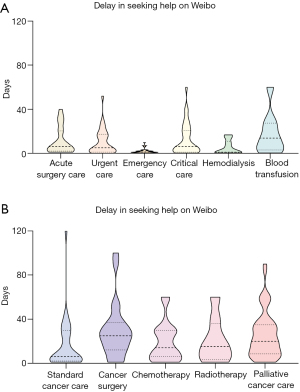
Discussion
This observational study confirmed that patients posted various non-COVID-19 diseases and health needs on social media, providing a viable option to surveil and understand the urgent and emergent health needs during an outbreak. In January 2020, the COVID-19 outbreak quickly overstretched the healthcare system in Wuhan city due to the lack of preparedness. Soon after that, China mobilized domestic resources to contain the epidemic at the end of March. Within the 3 months of the outbreak, Wuhan city could serve as a good study model for non-COVID-19 health needs, which might inspire a more flexible and adaptable healthcare system to address unmet needs promptly.
A comprehensive search for all relevant Weibo was conducted before the strict screening process. This broad-to-narrow strategy resulted in a tiny proportion of eligible Weibo, which were genuinely accurate and representative. Estimating the actual number of non-COVID-19 patients with unmet needs was derived from the observed and officially reported number of COVID-19 patients. Considering that not all patients sought help on Weibo, and patients with COVID-19 needs might be easier to access health services, the estimated magnitude of non-COVID-19 health needs was only an approximation of reality. However, our estimation was on the same scale as the mortality during the 3 months of the COVID-19 outbreak by Liu et al., which confirmed the feasibility and robustness of this proof-of-concept study. According to the nationwide mortality registries, 5,954 additional deaths occurred in Wuhan city: 4,573 were attributed to pneumonia, and 1,381 were due to other causes (4).
The magnitude of non-COVID-19 urgent and emergent health needs had increased with the outbreak of COVID-19, which was in concert with the discontinuation of non-COVID-19 health services (3,10,11). Kiss et al. conducted a systematic review and found that the admissions decreased as high as 50% for acute coronary syndromes and 40% for strokes in multiple studies (10). A significant delay in accessing health services was also observed (10). The WHO surveyed 163 Ministries of Health and found 30% to 50% of countries’ health services were disrupted from cardiovascular emergencies to rehabilitation services (12). The more severe the COVID-19 pandemic, the more essential health services were interrupted (12).
There were three main reasons why non-COVID-19 health needs were not addressed: reduced healthcare resources, lockdown of public transportation, and patient’s fear of COVID-19 infections in hospitals (13-16). In this study, the primary reason for unmet health needs was the shortage of healthcare resources in Wuhan city, which could be inferred from Weibo. We excluded unmet health needs of other reasons to prevent significant biases. The reduced healthcare resources were either due to the occupancy by COVID-19 or strict measures to decrease admission rates to mitigate the in-hospital spread of COVID-19. Those included patients who had failed to be hospitalized before seeking help on Weibo were due to the unavailability of medical staff, hospital services, and hospital beds (1,2). Two random cases were reported in Table 2.
Table 2
Case 1: male patient, 58 years old, stroke |
Non-pneumonia patients with severe cerebral infarction. [Name] xxx. [Age] 58. [City] Wuhan. [Address] xxx. [Onset time] February 25. [Contact information] xxx. [Description of condition] Urgent help! Please promote! [A patient with cerebral infarction in Wuhan, urgently in need of hospitalization] A patient with cerebral infarction in Wuhan city, Hubei Province is currently in critical condition and urgently needs hospitalization. The patient is now paralyzed because of the cerebral infarction. I contacted many hospitals but could not be admitted. I am the patient’s son. My father is currently paralyzed, unable to speak, eat or drink, or take oral medicines for treatment. It has been 3 days now. I am afraid that if he cannot receive treatment, he will not be able to stay for a few more days. Now I can’t get help from hospitals, I hope to ask for help on Weibo. I hope to find a hospital to admit and treat my father. Grateful! #Pneumonia# #Wuhan Non-COVID-19 patients seeking help# #Wuhan# #Confirmed novel coronavirus cases nationwide# |
Case 2: male patient, 54 years old, lung cancer |
Patients seeking help. [Name] xxx. [Age] 54. [City] Wuhan. [Address] xxx. [Onset time] June, 2019. [Contact information] xxx. [Description of condition] The patient is my father. He was diagnosed with advanced lung cancer last year. Due to the discontinuation of treatment, his condition has recently deteriorated and he began to vomit blood. Now he cannot eat, urinate and move. All hospitals contacted had no beds. The patient is in critical condition now! Please help us! |
COVID-19, coronavirus disease 2019.
The indirect impact of COVID-19 was indicated by the time lag between the COVID-19 outbreak and non-COVID-19 health needs. This phenomenon confirmed that the social media data might provide a viable option to surveil the unmet non-COVID-19 health needs. Unlike the earlier burst of social media data before the pandemic, Weibo seeking help lagged behind the COVID-19 outbreak (8). Henceforth, a flexible healthcare system should scale up the preparedness and response to COVID-19 and initiate proper measures for the upcoming non-COVID-19 health needs. However, the time trend of non-COVID-19 health needs was multifaceted, depending on the severity and duration of COVID-19, the prevalence of non-COVID-19 diseases, appropriate public health measures, viable non-COVID-19 care frameworks, and continuous medical supplies. Therefore, the time lag of non-COVID-19 needs might be different in other countries. The lack of strictly defined training and validation cohorts also restricted using the time lags to predict non-COVID-19 health needs. Anyhow, the COVID-19 pandemic was accompanied by non-COVID-19 health needs, which emphasized the importance of a flexible healthcare system that could respond to public health crises quickly and dynamically.
The increase in non-COVID-19 health needs had promoted the Wuhan Municipal Health Commission to designate a list of hospitals for non-COVID-19 diseases on February 18 after the peak of the COVID-19 outbreak. The designated hospitals provided various services for the cardiovascular emergency, traumatic injury, critical illness, hemodialysis, pregnancy and children, cancer and noncommunicable diseases, oral and eye diseases, and burns. This strategic adaptation reallocated healthcare resources and reduced the number of patients seeking help on Weibo, as indicated in the transition point of the trend of non-COVID-19 health needs. This also confirmed that online platforms would reflect the health needs promptly and might serve to inform healthcare policies.
Stroke and the acute coronary syndrome were among the most common non-neoplastic entities, which were time-sensitive and life-threatening emergencies. The minimal delay in seeking help could still lead to undesirable consequences, which were also reflected by the extra mortality of cardiovascular diseases in Wuhan city during the COVID-19 outbreak (4). Therefore, an effective emergency care system should be maintained to prevent delays even in an outbreak.
More than half of health needs in Weibo came from cancer patients, and most were derived from the deferral of standard cancer care, cancer surgery, chemotherapy, and palliative cancer care. Nearly a quarter of patients needed palliative cancer care for advanced cancer or complications. The suspension of cancer services was similar in other countries. In the Netherlands, the number of newly diagnosed cancer patients dropped by 25% (17). In Britain, bowel cancer screening was halted, which affected more than 1 million people and might interrupt the standard cancer care for 850 patients with colorectal cancer (18). In the survey conducted by WHO, almost 40% of countries had disrupted cancer services (12). The discontinuation of cancer care would probably lead to a poor prognosis and a substantial increase in avoidable cancer deaths. Due to the delayed cancer diagnosis in England, the number of cancer deaths would likely increase by 4.8–16.6% within 5 years (19). A 3-month delay of cancer surgery would probably cause 5% of attributable deaths per year (20). The survival of cancer patients in Wuhan was not yet understood and was also not revealed by the short-term mortality data (4). More research on the long-term health impacts of COVID-19 is necessary (21).
The impact of the COVID-19 outbreak on non-COVID-19 diseases was far-reaching and relatively unmeasurable. To our knowledge, this was the first study that used social media data to surveil and describe the nature and magnitude of non-COVID-19 health needs. There were several limitations in this study. Firstly, a more intelligent and automatic algorithm should be developed to facilitate real-time surveillance for non-COVID-19 health needs. Secondly, the Chinese New Year in January 2020 also affected the delay in seeking help, especially for some cancer patients, which should be differentiated from the impact of COVID-19. Thirdly, there was no follow-up data on Weibo, which restricted the knowledge of the outcomes of patients. Lastly, but not least, the lack of strictly defined training and validation cohorts limited the generalizability of our findings. Nevertheless, a strict validation cohort was nearly impossible in the research of an outbreak. Anyhow, our study confirmed the valuable role of Weibo for the surveilling and understanding of non-COVID-19 urgent and emergent health needs during the COVID-19 outbreak.
Conclusions
The COVID-19 outbreak in Wuhan city has produced an unprecedented impact on the current healthcare system. Our preliminary findings confirmed that patients posted various non-COVID-19 diseases and health needs on Weibo, thereby providing a viable option to surveil and understand the unmet health needs during an outbreak. Heterogeneous health needs derived from the social media data might inspire a more resilient healthcare system to address unmet needs promptly.
Acknowledgments
Funding: None.
Footnote
Reporting Checklist: The authors have completed the STROBE reporting checklist. Available at https://dx.doi.org/10.21037/atm-21-1769
Conflicts of Interest: All authors have completed the ICMJE uniform disclosure form (available at https://dx.doi.org/10.21037/atm-21-1769). The authors have no conflicts of interest to declare.
Ethical Statement: The authors are accountable for all aspects of the work in ensuring that questions related to the accuracy or integrity of any part of the work are appropriately investigated and resolved. The study was conducted in accordance with the Declaration of Helsinki (as revised in 2013). All data in this study were publicly available and de-identified. No patient consents were required and no ethical approval was obtained.
Open Access Statement: This is an Open Access article distributed in accordance with the Creative Commons Attribution-NonCommercial-NoDerivs 4.0 International License (CC BY-NC-ND 4.0), which permits the non-commercial replication and distribution of the article with the strict proviso that no changes or edits are made and the original work is properly cited (including links to both the formal publication through the relevant DOI and the license). See: https://creativecommons.org/licenses/by-nc-nd/4.0/.
References
- Zhuang Z, Cao P, Zhao S, et al. The shortage of hospital beds for COVID-19 and non-COVID-19 patients during the lockdown of Wuhan, China. Ann Transl Med 2021;9:200. [Crossref] [PubMed]
- Callison K, Ward J. Associations Between Individual Demographic Characteristics And Involuntary Health Care Delays As A Result Of COVID-19. Health Aff (Millwood) 2021;40:837-43. [Crossref] [PubMed]
- Roy CM, Bollman EB, Carson LM, et al. Assessing the indirect effects of COVID-19 on healthcare delivery, utilization and health outcomes: a scoping review. Eur J Public Health 2021;31:634-40. [Crossref] [PubMed]
- Liu J, Zhang L, Yan Y, et al. Excess mortality in Wuhan city and other parts of China during the three months of the covid-19 outbreak: findings from nationwide mortality registries. BMJ 2021;372: [PubMed]
- Clough HE, McIntyre KM, Patterson GE, et al. Use of routine death and illness surveillance data to provide insight for UK pandemic planning: lessons from COVID-19. BMJ Open 2021;11:e044707 [Crossref] [PubMed]
- Woolf SH, Chapman DA, Sabo RT, et al. Excess Deaths From COVID-19 and Other Causes in the US, March 1, 2020, to January 2, 2021. JAMA 2021;325:1786-9. [Crossref] [PubMed]
- Odone A, Delmonte D, Gaetti G, et al. Doubled mortality rate during the COVID-19 pandemic in Italy: quantifying what is not captured by surveillance. Public Health 2021;190:108-15. [Crossref] [PubMed]
- Li C, Chen LJ, Chen X, et al. Retrospective analysis of the possibility of predicting the COVID-19 outbreak from Internet searches and social media data, China, 2020. Euro Surveill 2020;25:2000199 [Crossref] [PubMed]
- Tao ZY, Chu G, McGrath C, et al. Nature and Diffusion of COVID-19-related Oral Health Information on Chinese Social Media: Analysis of Tweets on Weibo. J Med Internet Res 2020;22:e19981 [Crossref] [PubMed]
- Kiss P, Carcel C, Hockham C, et al. The impact of the COVID-19 pandemic on the care and management of patients with acute cardiovascular disease: a systematic review. Eur Heart J Qual Care Clin Outcomes 2021;7:18-27. [Crossref] [PubMed]
- De Filippo O, D'Ascenzo F, Angelini F, et al. Reduced Rate of Hospital Admissions for ACS during Covid-19 Outbreak in Northern Italy. N Engl J Med 2020;383:88-9. [Crossref] [PubMed]
- Dyer O. Covid-19: Pandemic is having "severe" impact on non-communicable disease care, WHO survey finds. BMJ 2020;369:m2210. [Crossref] [PubMed]
- Lazzerini M, Barbi E, Apicella A, et al. Delayed access or provision of care in Italy resulting from fear of COVID-19. Lancet Child Adolesc Health 2020;4:e10-1. [Crossref] [PubMed]
- Mantica G, Riccardi N, Terrone C, et al. Non-COVID-19 visits to emergency departments during the pandemic: the impact of fear. Public Health 2020;183:40-1. [Crossref] [PubMed]
- Mantica G, Riccardi N, Terrone C, et al. Non-COVID-19 admissions to the emergency department during the pandemic second wave in Italy: What is changed from the first wave? Am J Emerg Med 2021;45:625-6. [Crossref] [PubMed]
- Balestrino A, Fiaschi P, Melloni I, et al. In Reply: The Coronavirus Disease 2019 Global Pandemic: A Neurosurgical Treatment Algorithm. Neurosurgery 2020;87:E214-5. [Crossref] [PubMed]
- Dinmohamed AG, Visser O, Verhoeven RHA, et al. Fewer cancer diagnoses during the COVID-19 epidemic in the Netherlands. Lancet Oncol 2020;21:750-1. [Crossref] [PubMed]
- . The Lancet Gastroenterology Hepatology. Resuming bowel cancer screening post-COVID-19. Lancet Gastroenterol Hepatol 2020;5:711. [Crossref]
- Maringe C, Spicer J, Morris M, et al. The impact of the COVID-19 pandemic on cancer deaths due to delays in diagnosis in England, UK: a national, population-based, modelling study. Lancet Oncol 2020;21:1023-34. [Crossref] [PubMed]
- Sud A, Jones ME, Broggio J, et al. Collateral damage: the impact on outcomes from cancer surgery of the COVID-19 pandemic. Ann Oncol 2020;31:1065-74. [Crossref] [PubMed]
- Schuivens PME, Buijs M, Boonman-de Winter L, et al. Impact of the COVID-19 Lockdown Strategy on Vascular Surgery Practice: More Major Amputations than Usual. Ann Vasc Surg 2020;69:74-9. [Crossref] [PubMed]