Identification of the distinctive role of DPT in dilated cardiomyopathy: a study based on bulk and single-cell transcriptomic analysis
Introduction
Dilated cardiomyopathy (DCM), characterized as left ventricular dilation and systolic dysfunction, is a type of irreversible cardiomyopathy (1). DCM may gradually develop into severe congestive heart failure, which seriously threatens the survival of patients and leads to significant medical burdens and socioeconomic costs. However, except for heart transplantation, DCM cannot be effectively treated with existing treatment strategies (2). Therefore, identifying key genes related to the DCM progression is essential to preventing poor prognosis.
Microarray technology can measure global gene expression levels, which helps to identify differentially expressed genes (DEGs) and altered biological processes in DCM, then guiding the diagnosis and treatment of DCM. Barth et al. have analyzed 108 samples by integrating independent microarray studies and identified 27 DEGs in DCM, including CCL2, MYH6, and FRZB (3). Zhao et al. have identified target genes related to fibrosis and cardiac remodeling in DCM such as CTGF, POSTN, CORIN, and FIGF (4). However, as many different types of cells express a unique transcriptome, conventional bulk population sequencing can only provide the average expression levels for all cells and fails to identify the cell types that are potentially involved in gene functions. Single-cell RNA sequencing (scRNA-seq) is a rapidly developing powerful tool that can uncover previously unidentified disease-associated cell populations or functional states, their markers, interactions, and potential regulators (5). Therefore, by integrating bulk and single-cell transcriptome, we can clarify the relationship between novel genes and cells and then carry out a more in-depth exploration of the mechanism of DCM. In addition, due to the lack of experimental verification, previous DEG results for DCM may not be accurate, so it is necessary to combine bioinformatics analysis with laboratory verification.
The purpose of this study was to find potential crucial genes and regulators through bulk and single-cell transcriptomic analysis. We aimed to promote the understanding of the progression mechanism of DCM and identify potential therapeutic targets for DCM. We present the following article in accordance with the STREGA reporting checklist (available at https://dx.doi.org/10.21037/atm-21-2913).
Methods
Data preprocessing and DEGs screening
The gene expression profile data of GSE57338 (6), GSE42955 (7), GSE79962 (8), and GSE116250 (9) were imported from the gene expression omnibus (GEO) database to R (version 4.0) for analysis. The original data of GSE57338, GSE42955, and GSE79962 were read with “oligo” (10) package and “affy” package (11). The RMA algorithm was used for background correction and data standardization. The “sva” (12) package’s combat algorithm was used to remove inter-batch differences. For GSE116250, the original data was transformed log2(RPKM+1). The “limma” (13) package was used to screen the differential genes. After BH correction, genes with P value <0.05 and |fold change (FC)| >1.5 were considered differential genes. The volcano map of DEGs was visualized with “ggplot2” (14) and the heat map was visualized with “pheatmap” (15).
Gene ontology (GO) analysis
The “clusterProfiler” (16) package was used to perform GO enrichment analysis on differential genes. The top 10 biological process pathways of up and down DEGs were visualized in a barplot.
Screening and verification of key genes
The “glmnet” (17) package was used to perform the least absolute shrinkage and selection operator (LASSO) regression on DEGs. The “e1071” (18) package was used to train the support vector machine-recursive feature elimination (SVM-RFE) model. DEGs were imported into the STRING (https://string-db.org/cgi/input.pl; v11.0) (19) website to construct a protein-protein interaction (PPI) network with the cut-off score >0.7. Then PPI network was imported into the Cytoscape (https://cytoscape.org, v3.7.1) software and the Maximal Clique Centrality (MCC) algorithm was used in CytoHubba (v0.1) (20) to calculate the top 50 genes. The R package “venndigram” (21) was used to take the intersection and visualize them.
The dataset GSE116250 are used to verify the expression of key genes and the results were visualized with a violin plot. P values were evaluated by Wilcoxon rank-sum test in R. The “pROC” (22) package was used to calculate receiver operating characteristic (ROC) curve.
Analysis of scRNA-seq dataset
The raw count and metadata of GSE109816 and GSE121893 were downloaded from GEO (5). The R package “Seurat” (23) was used to create objects and calculate the proportion of mitochondrial genes. Cells expressing <200 or >7,000 genes in control heart samples and <200 or >5,000 genes in DCM samples were filtered out for exclusion of noncell or cell aggregates. Then, we used the “CCA” algorithm to merge the 18 samples, performed principal component analysis (PCA) filtering on the data, selected the top 20 PCs for data dimensionality reduction, and used the resolution 0.2 for clustering. The “FindAllMarkers” function (min.pct =0.25, logfc. threshold =0.25) was used to identify marker genes, using marker genes to annotate cell clusters as known cell types.
We divided the fibroblasts into DPT+ fibroblasts and DPT- fibroblasts by the expression of DPT, used the “FindMarkers” function to calculate the difference in gene expression between the two cell groups, used the “clusterProfiler” to perform Gene set enrichment analysis (GSEA) analysis of GO biological process terms, and used a barplot to visualize the pathway of top 10 normalized enrichment score (NES) value. P values were evaluated by the Wilcoxon rank-sum test in R.
SCENIC analysis
Single-cell regulatory network inference and clustering (SCENIC) analysis was performed on DPT+/DPT− fibroblasts using the “SCENIC” (24) package. SCENIC identified potential TF targets based on the co-expression network and perform TF motif enrichment analysis to identify direct targets (regulators) and score the activity of the regulators (AUCell score). Top 10 relative activity TFs were visualized in a barplot and certain TFs were visualized in a violin plot.
Ligand-receptor interaction analysis
Ligand-receptor interactions were calculated using “CellPhoneDB” (25) with default settings. Visualizing the interactions between cell groups with “circlize” (26) and pheatmap. Top significant (P<0.05) interactions were defined as >3rd quartile and plotted using ggplot2.
Tissue collection
The study was in accordance with the Declaration of Helsinki (as revised in 2013). The heart samples from 19 DCM patients and 4 NF donors admitted to Union Hospital of Tongji Medical College, Huazhong University of Science and Technology were collected. NF heart samples were taken from organ donors whose hearts could not be placed due to size issues, ABO mismatch, or other factors. The studies involving human participants were reviewed and approved by the Ethics Committee of Union Hospital Affiliated of Tongji Medical College of Huazhong University of Science and Technology (Number: UHCT21001). The patients/participants provided their written informed consent to participate in this study.
Real-time polymerase chain reaction (RT-PCR)
The total RNA in heart tissue was extracted with TRIzol reagent (Takara Bio., Shiga, Japan). The RNA sample from total RNA was reverse transcribed into cDNA using HiScript III RT SuperMix (Vazyme, Nanjing, China). RT-PCR was performed using the real-time PCR Kit (Vazyme, Nanjing, China) and the Bio-Rad Real-Time PCR System. GAPDH was used as an internal reference. The relative mRNA expression was calculated using the 2−ΔΔCt method. Gene-specific primers used in the study were: DPT, GGGGCCAGTATGGCGATTATG (forward), CGGTTCAAATTCACCCACCC (reverse); CCL5, CCAGCAGTCGTCTTTGTCAC (forward), CTCTGGGTTGGCACACACTT (reverse); GAPDH, ACAACTTTGGTATCGTGGAAGG (forward), GCCATCACGCCACAGTTTC (reverse).
Western blot
Samples (20 µg) were run on a 10% SDS-PAGE gel followed by blotting to a nitrocellulose membrane. Membranes were blocked and incubated with antibodies as follows: DPT (#10537-1-AP, Proteintech, Wuhan, China), GAPDH (#GB11002, servicebio, Wuhan, China). Corresponding secondary antibodies conjugated to horseradish peroxidase were used for detection. Staining was detected using chemiluminescence and quantified by Image Lab software (Bio-Rad, Richmond, CA, USA).
Immunohistochemical staining
Heart tissues were embedded in OCT and processed for cryo-sections at 12 µm. For immunohistochemistry, cryo-sections were immunostained with primary antibodies against DPT (diluted 1:50, 10537-1-AP, Proteintech, Wuhan, China) and CCL5 (diluted 1:50, AF5151, Affinity Biosciences, Cincinnati, OH, USA) followed by incubation with biotin-conjugated secondary antibodies, and then treated with avidin-peroxidase. The reaction was developed using the DAB substrate kit (Biosci, Wuhan, China), and the sections were counterstained with hematoxylin-eosin. For double immunofluorescent staining, cryo-sections were overlaid with DPT (diluted 1:50, 10537-1-AP, Proteintech, Wuhan, China), Vimentin antibody (diluted 1:150, CY5134, Abway, Beijing, China), Phospho-STAT3 antibody (diluted 1:100, Cell Signaling Technology, Massachusetts, USA) overnight at 4 °C and then were incubated with secondary antibodies for 1 h and stained with DAPI for 10 min.
Cardiac fibroblasts isolation and culture
Animal experiments were performed under a project license ([2020] IACUC Number: 2438) granted by the Animal Research Ethics Committee of Tongji Medical College, Huazhong University of Science and Technology, and were carried out in accordance with the National Institutes of Health guide for the care and use of laboratory animals (National Academy of Sciences Press, 2011).
Primary cultures of cardiac fibroblasts were isolated from the hearts of 6-week -old C57BL/6 mice using an enzymatic digestion solution containing 0.1% collagenase (type II, Worthington Biochemical, NJ) and 0.25% trypsin (Amresco, Cleveland, OH) at 37 °C. The second- or third-generation cells were used in all subsequent experiments. Cells were cultured in Dulbecco’s Modified Eagle’s Medium (DMEM; Gibco, Grand Island, NY, USA) supplemented with 10% fetal bovine serum (FBS, Gibco), 100 U/mL penicillin, and 100 µg/mL streptomycin. Cells were then treated with DMSO (Sigma-Aldrich, St. Louis, MO, USA) or 50 µM S3I-201 (MCE, Shanghai, China) for 2 days.
Statistical analysis
Unless specifically noted, all data were statistically analyzed using a two-tailed t-test to compare differences between different groups, assuming equal variance with PRISM software (GraphPad 6 Software). Asterisks were used as indicators for statistical differences for experimental data; P value <0.05 was considered statistically significant.
Results
DEGs screening and functional enrichment analysis
To find potential crucial genes associated with DCM, we first selected three GEO microarray datasets (GSE57338, GSE42955, GSE79962) in our study, including 103 DCM patients and 152 NF donors (Table 1). Data before and after normalization were checked by boxplot and PCA (Figure S1), which showed that the inter-batch difference had been eliminated. By setting the threshold at |FC| >1.5 and P value <0.05, we extracted 281 DEGs, consisting of 146 upregulated genes and 135 downregulated genes (Table S1). Figure 1A,1B show the volcano plots and DEG expression heatmap. GO enrichment analysis reveals that upregulated DEGs in DCM were primarily enriched in the extracellular matrix (ECM) organization, regulation of cell-substrate adhesion, and cardiocyte differentiation (Figure 1C), which is consistent with cardiac structural remodeling during DCM. Relatively, the downregulated DEGs were primarily enriched in neutrophil degranulation and activation, regulation of inflammatory response, and leukocyte chemotaxis, suggesting deregulation of the immune system in DCM (Figure 1D).
Table 1
Dataset | DCM | Normal | Tissue | Platform | Usage here |
---|---|---|---|---|---|
GSE57338 | 82 | 136 | Myocardium | Affymetrix Human Gene 1.1 ST Array | Combined analysis |
GSE42955 | 12 | 5 | Myocardium | Affymetrix Human Gene 1.0 ST Array | Combined analysis |
GSE79962 | 9 | 11 | Myocardium | Affymetrix Human Gene 1.0 ST Array | Combined analysis |
GSE116250 | 37 | 14 | Myocardium | Illumina HiSeq 2500 | Validate key genes |
GSE109816 | 0 | 12 | Myocardium | Illumina NextSeq 500 | Single-cell sequencing analysis |
GSE121893 | 4 | 2 | Myocardium | Illumina NextSeq 500 | Single-cell sequencing analysis |
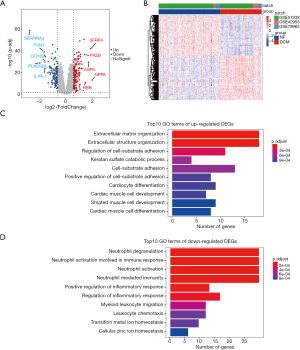
Screening and verification of key genes
To identify the potential genes with strong diagnostic significance value and biological significance from DEGs, we first used the LASSO regression algorithm and the SVM-RFE algorithm. LASSO regression minimizes the residual sum of squares and produces some coefficients that are exactly 0 and selects subsets (27). SVM-RFE is a feature selection algorithm based on SVM, which computes the weights of features and iteratively removes the features with the lowest weights (28). LASSO regression and SVM-RFE identified 26 and 33 candidate genes from DEGs, respectively (Figure 2A,2B). Then, we constructed a PPI network of DEGs with the STRING website and imported them to Cytoscape. The top 50 hub genes with the greatest degree of network connection were identified based on Cytohubba analysis (Figure 2C), implying that these genes might play vital roles in the network. Next, we overlapped the genes identified by these three algorithms and finally obtained two key genes: CCL5 (FC =1.57, P=9.3×10−16) and DPT (FC =1.71, P=9.4×10−21) (Figure 2D). To validate the expression levels and the diagnostic efficacy of CCL5 and DPT, we validated them in the GSE116250 dataset. The violin plot confirmed that the expression levels of CCL5 and DPT were both increased in the DCM samples (both P<0.001, Figure 2E). The ROC curve showed that both CCL5 and DPT had a good diagnostic efficiency (both AUC =0.844) (Figure 2F). The two genes were then fitting into one variable to build a diagnostic model (0.23195 × CCL5 expression + 0.30508 × DPT expression) and reached a higher level in the validation set (AUC =0.917). In conclusion, we have found that CCL5 and DPT have high diagnostic value and may play important roles in DCM.
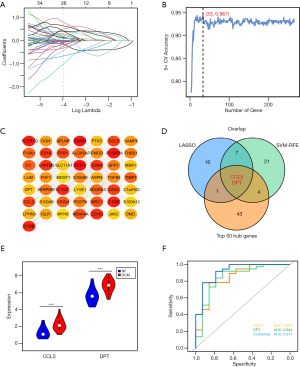
Expression of key genes in the scRNA-seq dataset
We used the scRNA-seq dataset by Wang et al. (5), which contained 14 healthy donors and 4 DCM patients (Table 1). 10,425 cells passed standard quality control and were retained for subsequent analysis. We clustered all cells into eleven subsets (Figure 3A) and annotated the cell population with previously published marker genes (Figure 3B), including cardiomyocytes (TTN, MYH6), endothelial cells (VWF, PECAM1), fibroblasts (DCN, LUM), pericytes (ABCC9, PDGFRB), smooth muscle cells (MYH11, CALD1), macrophages (CD163, MRC1), T cells (CD2, CD3D), epithelial cells (PRG4, ITLN1) lymphatic endothelial cells (lymphatic endo, MMRN1, LYVE1), and red blood cells (RBC, HBD, HBA).
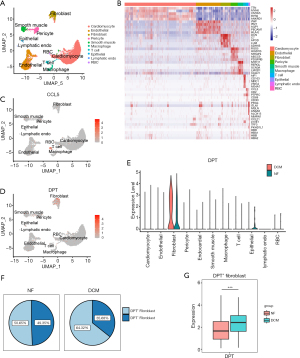
By locating the expression of CCL5 and DPT, we found that CCL5 was mainly expressed in T cells and DPT was mainly expressed in fibroblasts (Figure 3C,3D). Since fibroblastic activation and collagen deposition exert an important role in DCM development, we chose DPT, which is closely related to fibroblasts, as the target of our subsequent analysis. DPT is a small acidic ECM protein involved in skin wound healing and ECM maturation (29). Importantly, the expression level of DPT in DCM fibroblasts was higher than that in the NF group (Figure 3E), indicating that DPT is involved in the fibroblasts function of DCM. In addition, we found that the proportion of DPT+ fibroblasts in DCM patients was relatively increased (Figure 3F) and that DPT+ fibroblasts expressed more DPT (Figure 3G).
Characteristics of DPT+/DPT− fibroblasts
To examine the potential role of DPT+ fibroblasts in DCM, we separated fibroblasts into two sub-groups according to the expression of DPT (DPT+ fibroblasts and DPT− fibroblasts) and analyzed the differential GO pathways between the two cell groups by GSEA (Figure 4A). We found DPT+ fibroblasts were involved in ECM deposition and protein transport such as extracellular structure/matrix organization (NES =2.2, P=2.6×10−7, Figure 4B), collagen fibril organization (NES =2.2, P=6.1×10−4, Figure 4C), SRP-dependent cotranslational protein targeting to membrane, protein targeting to ER, and cotranslational protein targeting to membrane, suggesting a profound association of this population with fibrosis and cardiac remodeling. DPT- fibroblasts were enriched in muscle filament sliding, actin-myosin filament sliding, and mitochondrial ATP synthesis coupled electron transport (Figure 4B), indicating that they were involved in matrix contraction and mechanical tension maintenance.
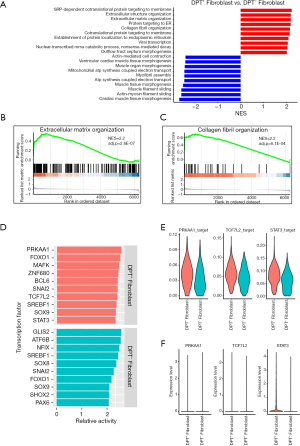
To explore the transcriptional regulator of DPT+/DPT− fibroblasts, the specific TFs of the two cell groups were predicted by SCENIC and the top 10 TFs of relative activity scores are shown (Figure 4D). We found that both cell groups were regulated by FOXO1, SOX9, SNAI2, and SREBF1, which have already been identified as TFs for fibroblast (30-33). However, we found that DPT+ fibroblasts were independently regulated by PRKAA1, TCF7L2, and STAT3 (Figure 4E). PRKAA1 is a catalytic subunit of AMP-activated protein kinase (AMPK), which is involved in fibroblast transformation to myofibroblasts (34). TCF7L2 mediates canonic Wnt/β-catenin signaling and c-Myc upregulation during abnormal cardiac remodeling (35). STAT3 integrates several profibrotic signals and might be a core mediator of fibrosis (36). Furthermore, the STAT3 expression level of DPT+ fibroblast was significantly elevated (Figure 4F) and the ARCHS4 database (https://maayanlab.cloud/archs4/) also showed that STAT3 might be the direct target of DPT (z-score =1.95), suggesting that STAT3 may be an important potential TF for DPT+ fibroblasts.
Ligand-receptor interaction analyses to assess intracellular communication
To explore the potential mechanism of intercellular communication between DPT+/DPT− fibroblasts and other cell types, we examined ligand-receptor interactions based on CellPhone DB v2.0. First, we observed the total interconnection among all cell groups. The number of connections between DPT+ fibroblasts and others was higher than that of DPT- fibroblasts, indicating that DPT+ fibroblasts have much stronger intercellular communication (Figure 5A). Second, both DPT+ fibroblasts and DPT- fibroblasts had more communication with endothelial cells, pericytes, and macrophages, but had less communication with T cells and cardiomyocytes (Figure 5B). Subsequently, we specifically examined the top unique interactions within DPT+/DPT− fibroblasts, split by DPT+/DPT− fibroblasts ligands and receptors. We found that DPT+ fibroblasts interacted with other cell groups through the Ephrin/Eph signaling, such as EPHA3-EPNA5, EFNA1-EPHA3/4, and EFNB2-EPHA4 (Figure 5C,5D). We also found that CXCL1 and CXCL8 interacted with ACKR1 on endothelial cells as well as the JAG-NOTCH pathway, which indicates that DPT+ fibroblasts regulate endothelial function. DPT- fibroblasts mainly interact with other cell groups through the DLK-NOTCH pathway (Figure 5E,5F), which inhibits the NOTCH pathway activity (37).
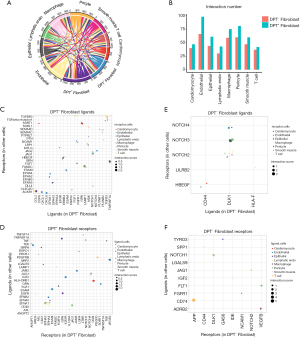
Verification of DPT expression and its regulation by STAT3
To verify the expression of DPT in DCM, we obtained heart samples from 19 DCM patients and 4 NF donors. RT-PCR showed that DPT expression level was higher in DCM patients (Figure 6A), western blot and immunohistochemistry also confirmed the results (Figure 6B,6C). In addition, CCL5 also has an, albeit not statistically significant (P=0.07, Figure S2), upward trend. Furthermore, we performed double-immunofluorescent of DPT and Vimentin (a marker for fibroblasts, Figure 6D) and found that they were co-localized. Then we further verified the regulation of DPT by STAT3. Double-immunofluorescent showed that DPT was co-localized with p-STAT3 (the activated state of STAT3, Figure 6E). We subsequently isolated mouse cardiac fibroblasts, treated them with the STAT3 inhibitor S3I-201, and found that S3I-201 significantly reduced the expression of DPT (Figure 6F).
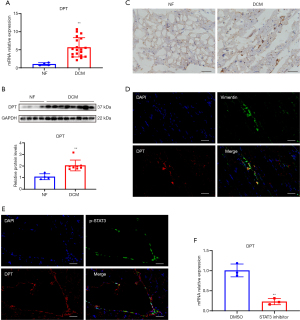
Discussion
In this study, we have integrated microarray and scRNA-seq datasets and conducted an in-depth analysis of the transcriptional changes in DCM. We are the first team to identify and confirm that CCL5 and DPT expression are elevated and have high diagnostic value in DCM and that they may be involved in the functions of T cells and fibroblasts, respectively. We also found that DPT+ fibroblasts may be a crucial cell group that mediates cardiac fibrosis and remodeling and that they are regulated by STAT3 and strongly interact with endothelial cells. The high expression of DPT and its co-localization with Vimentin and p-STAT3 was verified in human tissues and its regulation by STAT3 was verified in mouse cardiac fibroblasts. Our study provides valuable information for future research on the heart remodeling mechanisms of DCM.
The integration of multiple datasets can increase the sample size and maximize statistical power. Our study integrated three datasets and identified 281 DEGs between DCM and NF. GO analysis of DEGs has indicated that ECM organization and cell-substrate adhesion are activated in DCM. Previous studies have shown that mal-regulation of the ECM is associated with the progression of cardiac remodeling and heart failure (38). Cell-substrate adhesion mediates the interaction between cells and the ECM (39). Relatedly, inflammation responses such as neutrophil activation, inflammatory response, and leukocyte migration are down-regulated in DCM, which is consistent with previous studies on DCM (3,40). Chronic inflammation causes adverse cardiac remodeling in the development of DCM. Chemokines and cytokines promote the attraction and invasion of activated leukocytes but are also involved in shaping the ECM as well as the induction of cell apoptosis (41). Therefore, the down-regulation of inflammation response in end-stage DCM may represent an adaptive mechanism to reduce myocardial damage and promote cell survival (3).
In our study, by combining LASSO regression, SVM-RFE, and PPI network methods, we identified CCL5 and DPT as key genes. Although each algorithm has its inherent characteristics, these two key genes were reliable in our further verification, which showed that the integration strategy is feasible. CCL5, also known as RANTES, is mainly expressed and secreted by T cells, platelets, and macrophages. A previous study showed that CCL5 is elevated during the heart of heart failure patients (42). Marino et al. found that CCL5 signaling via CCR5/CCR1 plays a critical role in the migration of T cells and the deposition of fibronectin in chronic cardiomyopathy (43). Our scRNA-seq analysis has shown that CCL5 is mainly expressed on T cells. Previous research verified that CD8 T cells secreted CCL5, which resulted in pro-inflammatory and pro-hypertrophic events, cardiac remodeling, and interstitial fibrosis in chronic Chagas heart disease (44). Therefore, we speculate that CCL5 mediates immune cell infiltration and heart remodeling in DCM.
DPT is a non-collagenous ECM protein that was initially found in the dermis and then detected in various tissues including the heart (29). DPT regulates the formation of collagen and fibronectin fibrils and also promotes blood vessel repair. Previous studies showed that the expression of DPT is increased in the infarct zone of myocardial infarction rats and is co-expressed with decorin and type I collagen (45). Our study found that DPT expression also increased in DCM and co-localized with Vimentin. Liu et al. (46) found that DPT promotes the adhesion, spreading, and migration of cardiac fibroblasts by interacting with integrin α3β1. In the mouse model of liver cirrhosis, DPT knockout mice have decreased pro-fibrotic response and ECM deposition (47), indicating that DPT may mediate cardiac remodeling by regulating the migration or secretion of fibroblasts. We subsequently focused on DPT+ fibroblasts, which had a higher proportion and expressed higher DPT levels in DCM. GSEA analysis showed that this cell population was involved in the organization of the ECM and collagen fibrils. Therefore, DPT+ fibroblasts might be a reliable target to prevent DCM ventricular remodeling. Recent studies have detected a new subpopulation of fibroblasts appearing after heart injury called “matrifibrocyte” (48), characterized by the loss of proliferation ability and α-SMA expression as the collagen-containing ECM and scar fully matured. These cells express common and unique ECM and tendon genes that are more specialized to support the mature scar, which have similar transcription characteristics with DPT+ fibroblasts, indicating that these cells are worthy of further research.
The SCENIC analysis predicted that PRKKA1, TCF7L2, and STAT3 may regulate the transcription of DPT+ fibroblasts since the expression level of STAT3 is highest in DPT+ fibroblasts and may directly regulate DPT. It has been reported that STAT3 activation enhances STAT3 and SMAD3 interaction in cardiac fibroblasts (49). STAT3 also integrates the activation of JAK2, SRC, c-ABL, and JNK kinases and then induces myofibroblast differentiation and the up-regulation of collagen release (36). Therefore, we speculate that STAT3 may be a key molecular checkpoint for regulating DPT+ fibroblasts activation.
Previous studies have shown that fibroblasts and endothelial cells have strong communication, especially during myocardial remodeling (50). CellPhone DB analysis in this study revealed that DPT+ fibroblasts and endothelial cells have the closest connections through ligand-receptor interaction including the Ephrin-Eph family, ACKR signaling, and the JAG-NOTCH pathway. Ephrin ligands and Eph receptors regulate fundamental biological processes involved in tissue fibrosis including cell migration, myofibroblast activation, angiogenesis, and tissue remodeling (51). The combination of ephrinA1 and Epha on endothelial cells promotes the sprouting, formation, survival, and secretion of MCP-1 and CXCL1 of endothelial cells (52,53). ACKR1 activation in endothelial cells improves the recruitment of leukocytes and cytokines secretion through the binding of chemokine CXCL8. Therefore, DPT+ fibroblasts may regulate inflammation and angiogenesis by acting on endothelial cells in DCM. Meanwhile, endothelial cells may affect the function of fibroblasts. It has been found that JAG1 on endothelial cells combines with the NOTCH pathway on hepatic stellate cells to promote collagen secretion (54). Therefore, DPT+ fibroblasts and endothelial cells may play a key role in the progression of DCM through cell-to-cell interactions which are potentially druggable.
Our research has some limitations. First, due to the lack of clinical information including disease severity and heart function, it is impossible to estimate the relationship between DPT and disease severity. Second, the relationship between the studied genes and cell types has not been confirmed through other functional studies or studies in vivo, which will be the focus of our future research.
Conclusions
In summary, we innovatively combined the bulk and single-cell transcriptome, discovered the relationship between DPT and fibroblasts in DCM, and clarified the effect of DPT+ fibroblasts on myocardial remodeling, as well as their transcriptional regulators and intercellular pathways. We have proposed a novel mechanism of DCM and provided a new target for future translation studies.
Acknowledgments
Dr. YD thanks Mr. Li Xiao for his consistent support during this research and Jenny Xiao (Columbia University, New York, USA) for editing the manuscript and checking for grammatical errors. Dr. YL thanks Youwei Li for his technical support.
Funding: The work is funded by National Natural Science Foundation of China (81700332 and 81770328).
Footnote
Reporting Checklist: The authors have completed the STREGA reporting checklist. Available at https://dx.doi.org/10.21037/atm-21-2913
Data Sharing Statement: Available at https://dx.doi.org/10.21037/atm-21-2913
Conflicts of Interest: All authors have completed the ICMJE uniform disclosure form (available at https://dx.doi.org/10.21037/atm-21-2913). The authors have no conflicts of interest to declare.
Ethical Statement: The authors are accountable for all aspects of the work in ensuring that questions related to the accuracy or integrity of any part of the work are appropriately investigated and resolved. The study was conducted in accordance with the Declaration of Helsinki (as revised in 2013). The study was approved by the Ethics Committee of Union Hospital Affiliated to Tongji Medical College of Huazhong University of Science and Technology (No.: UHCT21001) and informed consent was taken from all individual participants. Animal experiments were performed under a project license ([2020] IACUC Number: 2438) granted by the Animal Research Ethics Committee of Tongji Medical College, Huazhong University of Science and Technology, and were carried out in accordance with the National Institutes of Health guide for the care and use of laboratory animals (National Academy of Sciences Press, 2011).
Open Access Statement: This is an Open Access article distributed in accordance with the Creative Commons Attribution-NonCommercial-NoDerivs 4.0 International License (CC BY-NC-ND 4.0), which permits the non-commercial replication and distribution of the article with the strict proviso that no changes or edits are made and the original work is properly cited (including links to both the formal publication through the relevant DOI and the license). See: https://creativecommons.org/licenses/by-nc-nd/4.0/.
References
- Fatkin D, Huttner IG, Kovacic JC, et al. Precision Medicine in the Management of Dilated Cardiomyopathy: JACC State-of-the-Art Review. J Am Coll Cardiol 2019;74:2921-38. [Crossref] [PubMed]
- Yancy CW, Jessup M, Bozkurt B, et al. 2017 ACC/AHA/HFSA Focused Update of the 2013 ACCF/AHA Guideline for the Management of Heart Failure: A Report of the American College of Cardiology/American Heart Association Task Force on Clinical Practice Guidelines and the Heart Failure Society of America. Circulation 2017;136:e137-61. [Crossref] [PubMed]
- Barth AS, Kuner R, Buness A, et al. Identification of a common gene expression signature in dilated cardiomyopathy across independent microarray studies. J Am Coll Cardiol 2006;48:1610-7. [Crossref] [PubMed]
- Zhao J, Lv T, Quan J, et al. Identification of target genes in cardiomyopathy with fibrosis and cardiac remodeling. J Biomed Sci 2018;25:63. [Crossref] [PubMed]
- Wang L, Yu P, Zhou B, et al. Single-cell reconstruction of the adult human heart during heart failure and recovery reveals the cellular landscape underlying cardiac function. Nat Cell Biol 2020;22:108-19. [Crossref] [PubMed]
- Liu Y, Morley M, Brandimarto J, et al. RNA-Seq identifies novel myocardial gene expression signatures of heart failure. Genomics 2015;105:83-9. [Crossref] [PubMed]
- Molina-Navarro MM, Roselló-Lletí E, Ortega A, et al. Differential gene expression of cardiac ion channels in human dilated cardiomyopathy. PLoS One 2013;8:e79792 [Crossref] [PubMed]
- Matkovich SJ, Al Khiami B, Efimov IR, et al. Widespread Down-Regulation of Cardiac Mitochondrial and Sarcomeric Genes in Patients With Sepsis. Crit Care Med 2017;45:407-14. [Crossref] [PubMed]
- Sweet ME, Cocciolo A, Slavov D, et al. Transcriptome analysis of human heart failure reveals dysregulated cell adhesion in dilated cardiomyopathy and activated immune pathways in ischemic heart failure. BMC Genomics 2018;19:812. [Crossref] [PubMed]
- Carvalho BS, Irizarry RA. A framework for oligonucleotide microarray preprocessing. Bioinformatics 2010;26:2363-7. [Crossref] [PubMed]
- Gautier L, Cope L, Bolstad BM, et al. affy--analysis of Affymetrix GeneChip data at the probe level. Bioinformatics 2004;20:307-15. [Crossref] [PubMed]
- Leek JT, Johnson WE, Parker HS, et al. The sva package for removing batch effects and other unwanted variation in high-throughput experiments. Bioinformatics 2012;28:882-3. [Crossref] [PubMed]
- Smyth GK. limma: Linear Models for Microarray Data. In: Gentleman R, Carey VJ, Huber W, et al. editors. Bioinformatics and Computational Biology Solutions Using R and Bioconductor. New York: Springer, 2005:397-420.
- Ginestet C. ggplot2: Elegant Graphics for Data Analysis. J R Stat Soc Ser A-Stat Soc 2011;174:245-6. [Crossref]
- Kolde R. Pheatmap: pretty heatmaps. R package version 2012;1.
- Yu G, Wang LG, Han Y, et al. clusterProfiler: an R package for comparing biological themes among gene clusters. OMICS 2012;16:284-7. [Crossref] [PubMed]
- Friedman J, Hastie T, Tibshirani R. Regularization Paths for Generalized Linear Models via Coordinate Descent. J Stat Softw 2010;33:1-22. [Crossref] [PubMed]
- Dimitriadou E, Hornik K, Leisch F, et al. Misc functions of the Department of Statistics (e1071), TU Wien. R package 2008;1:5-24.
- Szklarczyk D, Morris JH, Cook H, et al. The STRING database in 2017: quality-controlled protein-protein association networks, made broadly accessible. Nucleic Acids Res 2017;45:D362-8. [Crossref] [PubMed]
- Chin CH, Chen SH, Wu HH, et al. cytoHubba: identifying hub objects and sub-networks from complex interactome. BMC Syst Biol 2014;8:S11. [Crossref] [PubMed]
- Chen H. VennDiagram: Generate high-resolution Venn and Euler plots. R package version 2018;1:1.
- Robin X, Turck N, Hainard A, et al. pROC: an open-source package for R and S+ to analyze and compare ROC curves. BMC Bioinformatics 2011;12:77. [Crossref] [PubMed]
- Stuart T, Butler A, Hoffman P, et al. Comprehensive Integration of Single-Cell Data. Cell 2019;177:1888-1902.e21. [Crossref] [PubMed]
- Aibar S, González-Blas CB, Moerman T, et al. SCENIC: single-cell regulatory network inference and clustering. Nat Methods 2017;14:1083-6. [Crossref] [PubMed]
- Efremova M, Vento-Tormo M, Teichmann SA, et al. CellPhoneDB: inferring cell-cell communication from combined expression of multi-subunit ligand-receptor complexes. Nat Protoc 2020;15:1484-506. [Crossref] [PubMed]
- Gu Z, Gu L, Eils R, et al. circlize Implements and enhances circular visualization in R. Bioinformatics 2014;30:2811-2. [Crossref] [PubMed]
- Tibshirani R. Regression Shrinkage and Selection Via the Lasso. J R Stat Soc Series B Stat Methodol 1996;58:267-88.
- Guyon I, Weston J, Barnhill S, et al. Gene Selection for Cancer Classification using Support Vector Machines. Mach Learn 2002;46:389-422. [Crossref]
- Okamoto O, Fujiwara S. Dermatopontin, a novel player in the biology of the extracellular matrix. Connect Tissue Res 2006;47:177-89. [Crossref] [PubMed]
- Xin Z, Ma Z, Hu W, et al. FOXO1/3: Potential suppressors of fibrosis. Ageing Res Rev 2018;41:42-52. [Crossref] [PubMed]
- Lacraz GPA, Junker JP, Gladka MM, et al. Tomo-Seq Identifies SOX9 as a Key Regulator of Cardiac Fibrosis During Ischemic Injury. Circulation 2017;136:1396-409. [Crossref] [PubMed]
- Yang Z, Yang X, Xu S, et al. Reprogramming of stromal fibroblasts by SNAI2 contributes to tumor desmoplasia and ovarian cancer progression. Mol Cancer 2017;16:163. [Crossref] [PubMed]
- Shichino S, Ueha S, Hashimoto S, et al. Transcriptome network analysis identifies protective role of the LXR/SREBP-1c axis in murine pulmonary fibrosis. JCI Insight 2019;4:e122163 [Crossref] [PubMed]
- Noppe G, Dufeys C, Buchlin P, et al. Reduced scar maturation and contractility lead to exaggerated left ventricular dilation after myocardial infarction in mice lacking AMPKα1. J Mol Cell Cardiol 2014;74:32-43. [Crossref] [PubMed]
- Hou N, Ye B, Li X, et al. Transcription Factor 7-like 2 Mediates Canonical Wnt/β-Catenin Signaling and c-Myc Upregulation in Heart Failure. Circ Heart Fail 2016; [Crossref] [PubMed]
- Chakraborty D, Šumová B, Mallano T, et al. Activation of STAT3 integrates common profibrotic pathways to promote fibroblast activation and tissue fibrosis. Nat Commun 2017;8:1130. [Crossref] [PubMed]
- Nueda ML, González-Gómez MJ, Rodríguez-Cano MM, et al. DLK proteins modulate NOTCH signaling to influence a brown or white 3T3-L1 adipocyte fate. Sci Rep 2018;8:16923. [Crossref] [PubMed]
- Louzao-Martinez L, Vink A, Harakalova M, et al. Characteristic adaptations of the extracellular matrix in dilated cardiomyopathy. Int J Cardiol 2016;220:634-46. [Crossref] [PubMed]
- Schroer AK, Merryman WD. Mechanobiology of myofibroblast adhesion in fibrotic cardiac disease. J Cell Sci 2015;128:1865-75. [Crossref] [PubMed]
- Jiang Z, Guo N, Hong K. A three-tiered integrative analysis of transcriptional data reveals the shared pathways related to heart failure from different aetiologies. J Cell Mol Med 2020;24:9085-96. [Crossref] [PubMed]
- Mann DL. Innate immunity and the failing heart: the cytokine hypothesis revisited. Circ Res 2015;116:1254-68. [Crossref] [PubMed]
- Wang C, Yang H, Gao C. Potential biomarkers for heart failure. J Cell Physiol 2019;234:9467-74. [Crossref] [PubMed]
- Marino AP, da Silva A, dos Santos P, et al. Regulated on activation, normal T cell expressed and secreted (RANTES) antagonist (Met-RANTES) controls the early phase of Trypanosoma cruzi-elicited myocarditis. Circulation 2004;110:1443-9. [Crossref] [PubMed]
- Caballero EP, Santamaría MH, Corral RS. Endogenous osteopontin induces myocardial CCL5 and MMP-2 activation that contributes to inflammation and cardiac remodeling in a mouse model of chronic Chagas heart disease. Biochim Biophys Acta Mol Basis Dis 2018;1864:11-23. [Crossref] [PubMed]
- Takemoto S, Murakami T, Kusachi S, et al. Increased expression of dermatopontin mRNA in the infarct zone of experimentally induced myocardial infarction in rats: comparison with decorin and type I collagen mRNAs. Basic Res Cardiol 2002;97:461-8. [Crossref] [PubMed]
- Liu X, Meng L, Shi Q, et al. Dermatopontin promotes adhesion, spreading and migration of cardiac fibroblasts in vitro. Matrix Biol 2013;32:23-31. [Crossref] [PubMed]
- Lefebvre P, Lalloyer F, Baugé E, et al. Interspecies NASH disease activity whole-genome profiling identifies a fibrogenic role of PPARα-regulated dermatopontin. JCI Insight 2017;2:e92264 [Crossref] [PubMed]
- Fu X, Khalil H, Kanisicak O, et al. Specialized fibroblast differentiated states underlie scar formation in the infarcted mouse heart. J Clin Invest 2018;128:2127-43. [Crossref] [PubMed]
- Su SA, Yang D, Wu Y, et al. EphrinB2 Regulates Cardiac Fibrosis Through Modulating the Interaction of Stat3 and TGF-β/Smad3 Signaling. Circ Res 2017;121:617-27. [Crossref] [PubMed]
- Howard CM, Baudino TA. Dynamic cell-cell and cell-ECM interactions in the heart. J Mol Cell Cardiol 2014;70:19-26. [Crossref] [PubMed]
- Wu B, Rockel JS, Lagares D, et al. Ephrins and Eph Receptor Signaling in Tissue Repair and Fibrosis. Curr Rheumatol Rep 2019;21:23. [Crossref] [PubMed]
- Funk SD, Yurdagul A Jr, Albert P, et al. EphA2 activation promotes the endothelial cell inflammatory response: a potential role in atherosclerosis. Arterioscler Thromb Vasc Biol 2012;32:686-95. [Crossref] [PubMed]
- Cheng N, Brantley DM, Liu H, et al. Blockade of EphA receptor tyrosine kinase activation inhibits vascular endothelial cell growth factor-induced angiogenesis. Mol Cancer Res 2002;1:2-11. [Crossref] [PubMed]
- Ramachandran P, Dobie R, Wilson-Kanamori JR, et al. Resolving the fibrotic niche of human liver cirrhosis at single-cell level. Nature 2019;575:512-8. [Crossref] [PubMed]