Analysis of the polarization states of the alveolar macrophages in chronic obstructive pulmonary disease samples based on miRNA-mRNA network signatures
Introduction
Chronic obstructive pulmonary disease (COPD) is a severe disabling chronic lung disease that represents a significant and growing cause of morbidity and mortality, with a high prevalence of over 250 million cases worldwide (1,2). The main symptoms of COPD include shortness of breath and coughing with sputum, which is common, preventable, and treatable. It is a disease characterized by significant exposure to cigarette smoking in high-income counties, while indoor cooking and occupational exposures in low-income countries are thought to induce recruitment of inflammatory cells (2). The typical phenotype is the increase of monocytes, lymphocytes, and neutrophils, leading to changes in lung airways and alveoli (3,4). The pathogenesis of COPD includes oxidative stress, inflammation, protease/antiprotease disorders, apoptosis/proliferation imbalance, aging, autophagy, metabolic disorders, and mitochondrial dysfunction (5,6). Timely treatment can improve the deterioration of the condition, but there is no cure to date. The current treatment methods are generalized, which dramatically affect the treatment effect. Therefore, it is of great importance to understand its pathogenesis, find effective treatment methods, and provide personalized treatment for the different pathogenic characteristics.
Airway remodeling, inflammation, and emphysematous destruction in the lung contribute to disease severity and progression, with macrophage activity having an important role in COPD treatment (7). For example, lung macrophages affect COPD by participating the process of removing dead and dying cells, and cell debris (8). Macrophages can differentiate through the induction of cytokines and certain microbial products in the microenvironment and show different phenotypes and functional characteristics due to different inducing factors. For example, early studies have found that interferon-gamma (IFN-γ) alone or synergistically with lipopolysaccharides (LPS), or other cytokines can activate macrophages. The activated cells produce a large number of toxic mediators such as NO and reactive oxygen intermediates (ROI), which enhance the ability of antigen, and secrete IL-12 and IL-13, which further activate the type I immune response and play an important role in pathogen clearance (9). The activation of M1 macrophages occurs through signal transduction of IFN-γ, tumor necrosis factor (TNF), and Toll-like receptors (TLR) (10). Genetic markers related to M1 polarization include interleukin 1 alpha (IL1a), IL1b, IL6, nitric oxide synthase 2 (NOS2), TLR2, TLR4, CD80 molecule (CD80), and CD86. For M2 macrophages, activation is through cytokines such as IL4, IL10, and IL13, with the biomarkers of CD115, CD206, and peroxisome proliferator activated receptor gamma (PPARγ) (11). The study of Li et al. (12) demonstrated that long non-coding RNA (lncRNA) MIR155HG can promote the polarization of M1 macrophages and release of pro-inflammatory cytokines. He et al. (13) revealed that cigarette smoke extract-treated bronchial epithelial cells can alleviate M2 macrophage polarization through reducing the miR-21 level, and eventually relieve the epithelial-mesenchymal transition process of bronchial epithelial cells under COPD pathogenesis, thus revealing their novel compensatory role in COPD. Shaykhiev et al. (14) demonstrated that M1-related genes encoding type I chemokines C-X-C motif chemokine ligand 9 (CXCL9), CXCL10, CXCL11, C-C motif chemokine ligand 5 (CCL5), and M2-related genes such as matrix metalloproteinase (MMP)2, MMP7, and ADORA3 might contribute to global alveolar macrophages and thus affect COPD in a non-inflammatory way. Pro-inflammatory M1, anti-inflammatory M2, and M2-like cells represent the extremes of the polarization pattern of macrophages (15). However, analyses of macrophage cell (M0, M1, and M2) polarization on COPD, healthy smokers, and healthy people who have never smoked need to be widely conducted. In a recent study, Zhao et al. (16) identified the immune-related genes associated with COPD and macrophage polarization. However, they only revealed the genes by RNA-Seq analysis. The regulation of microRNA (miRNA) in COPD has been described in several studies (4,17-20). In a study by Huang et al. (19), let-7, miR-34a/b/c, -146a, -203, and -218 were found to be potential markers for COPD risk. In a study by Lacedonia et al. (20), miRNA-145 and miRNA-338 were emphasized in serum and sputum of patients with COPD. Along with miRNAs, gene expression profiling also plays an essential role in COPD. Studies on airway epithelium and peripheral blood have focused on expression changes related to smoking (21-24) and COPD status (25-28), including targeted RNA sequencing (RNA-Seq) profiling (29,30). Given the putative role macrophages have in inflammatory lung disease (31), whether miRNAs or mRNAs regulate the polarization of macrophages and thus affect COPD is unclear. We speculated that abnormally expressed miRNAs may regulate macrophage polarization and participate in the COPD process.
Despite the conduction of several high-throughput sequencing studies that have analyzed macrophage activation or miRNAs in response to COPD, no correlation analysis has been performed. The present study was performed to analyze the functions of miRNAs or mRNAs on macrophage cells in patients with COPD, healthy smokers, and those who have never smoked by combining the profiles of macrophage cells and expression levels of miRNAs and mRNAs in the RNA-Seq dataset. In conducting this study, we expected to determine a new molecular characteristic of COPD patients, which may be beneficial for their treatment.
We present the following article in accordance with the STREGA reporting checklist (available at https://dx.doi.org/10.21037/atm-21-3815).
Methods
Retrieval and process of public data
The gene expression levels of clinical samples of COPD patients (COPD, n=6), healthy smokers (Smoke, n=11), and never smokers (Never, n=4) were downloaded as the verification dataset from the Sequence Read Archive (SRA) with the accession number of PRJNA510951 [Gene Expression Omnibus (GEO) repository: GSE124180] (32). Moreover, the expression levels of mRNAs and miRNAs in macrophage cells of M0 (n=7), M1 (n=7), and M2 (n=7) were downloaded from SRA with the GEO repositories of GSE46903 and GSE51307 (33). The study was conducted in accordance with the Declaration of Helsinki (as revised in 2013).
Differentially expressed mRNA or miRNA analysis
The software edgeR (34) was used to screen the differentially expressed mRNAs (DEmRNAs) in RNA-Seq data, with the threshold of false discovery rate (FDR ≤0.05) and log2fold change (FC) ≥1 (up-regulated) or log2FC ≤−1 (down-regulated). The DEmRNA analysis on the microarray data probe dataset was performed using online GEO2R with default parameters (https://www.ncbi.nlm.nih.gov/geo/geo2r/). An adjusted P value <0.05 and the |log2FC| ≥1 were chosen as the cut-off thresholds. To systematically analyze the DE miRNA (DEmiRNA) expression and function, we used a miRNA analysis pipeline similar to that of a previous report (35).
Functional enrichment analysis
The function of DEmRNAs was analyzed by Gene Ontology (GO, http://geneontology.org/) with the Benjamini-Hochberg FDR controlling procedure used to define the enrichment of each term. Reactome (http://reactome.org) pathway profiling was also used for functional enrichment analysis of DEmRNAs.
Targeted genes of miRNAs
The potential miRNA targets were identified using miRDB (http://mirdb.org/) and TargetScan (v6.0, http://www.targetscan.org/) with default settings. All predicted targeted genes were evaluated by a scoring system, and the sequences were considered as miRNA targets only if the miRanda score was more than 500 or TargetScan context + score was less than 0.
Statistical analysis
Two-dimensional (dim) principal component analysis (2D PCA) was performed by R package factoextra (https://cloud.r-project.org/package=factoextra) to show the clustering of samples with the first 2 components. The pheatmap package (https://cran.r-project.org/web/packages/pheatmap/index.html) in R was used to conduct clustering based on the Euclidean distance. Cytoscape (v3.6.1, https://cytoscape.org) was used for network exhibition. The Student’s t-test was used for comparisons between two groups, and analysis of variance (ANOVA) for three groups.
Results
Abnormal immune responses were present in COPD, Smoke, and Never specific samples
A total of 15,486 common mRNAs were detected among COPD, Smoke, and Never specific samples, with 412, 2,499, and 306 identified to be sample specific, respectively (Figure 1A). The PCA of the normalized mRNAs expression level in COPD, Smoke, and Never samples displayed no distinct difference, being 12.4% and 11.5% for dim1 and dim2, respectively (Figure 1B). The non-normalized mRNA expression level in COPD, Smoke, and Never samples are shown in Figure S1A. These results indicated that the baseline of the normalized mRNAs expression level was consistent, thus providing a basis for further analysis. A total of 99, 58, and 393 genes were up-regulated and 357, 48, and 86 genes were down-regulated in Smoke, Never, and COPD specific samples, respectively (Figure 1C). More up-regulated genes were detected in Never specific samples than the other 2 groups, and more down-regulated genes were identified in the COPD group. It can be seen from the heatmap that the population-specific genes were highly expressed in their own samples (Figure 1D). The DEmRNAs in Smoke specific samples were involved in an immune response (Figure 1E). The DEmRNAs in Never specific samples were mainly involved in GO terms of an inflammatory response, immune response, T cell activation, the cell surface receptor signaling pathway, the T cell receptor signaling pathway, positive regulation of the nitric oxide biosynthetic process, cellular response to lipopolysaccharide, T cell stimulation, positive regulation of mitosis, and positive regulation of interferon-gamma production (Figure 1F). The up-regulated DEmRNAs in COPD specific samples were enriched in GO terms of immune response, cell differentiation, and signal transduction (Figure 1G). It was observed from the GO analysis that the DEmRNAs in clinical samples were all immune response-related. The cellular immune components revealed that the immune cells had a higher proportion at macrophages M0, macrophages M2, and activated mast cells in COPD, Smoke, and Never samples (Figure 1H; Figure S1B). The ratio of macrophages M0 and M2 in Never specific samples was less than that in COPD and Smoke specific samples. The GO terms that down-regulated DEmRNAs enriched in COPD specific and Smoke specific samples are listed in Figure S1C,S1D, respectively.
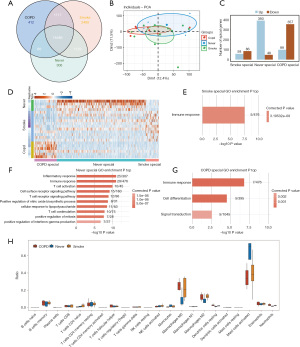
Macrophages M1 specific up-regulated genes contributed to the development of COPD
A total of 1,105, 1,286, and 684 up-regulated, as well as 689, 1,172, and 477 down-regulated DEmRNAs were detected in M0, M1, and M2 specific cells, respectively (Figure 2A). The up-regulated DEmRNAs of M0 specific cells were involved in small molecule metabolic process, cholesterol biosynthetic process, the isoprenoid biosynthetic process, cellular component movement, actin cytoskeleton organization, and the positive regulation of the inflammatory response (Figure 2B). The up-regulated DEmRNAs of M1 specific cells were mainly involved in GO terms of the cytokine-mediated signaling pathway, type I interferon-mediated signaling pathway defense response to viruses, interferon-gamma-mediated signaling pathway response to viruses, and negative regulation of the viral genome replication immune response (Figure 2C). The up-regulated DEmRNAs of M2 specific cells were mainly enriched in GO terms of translational elongation, translation initiation, viral transcription, translation termination, translation, and nuclear-transcribed mRNA catabolic process (Figure 2D). The GO analysis of down-regulated DEmRNAs in M0, M1, and M2 specific cells is displayed in Figure S2. There were 6, 1, and 5 overlapping DEmRNAs between M0 and clinical groups; 4, 3, and 2 between M1 and clinical groups; and 8, 2, and 2 between M2 and clinical groups of COPD, Smoke, and Never, respectively (Figure 2E). Combined with the GO enrichment analysis, we can conclude that DEmRNAs in M1 cells are mostly up-regulated in Never samples. In other words, the immune-related DEmRNAs in M1 specific cells were down-regulated by COPD. The overlapping DEmRNAs are listed in Figure 2F. The overlapping DEmRNAs between M1 specific cells and Never specific samples were: absent in melanoma 2 (AIM2), complement C1s (C1S), complement factor B (CFB), cardiotrophin like cytokine factor 1 (CLCF1), C-X-C motif chemokine ligand 10 (CXCL10), CXCL11, dihydropyrimidinase like 3 (DPYSL3), endothelin 1 (EDN1), coagulation factor III, tissue factor (F3), G0/G1 switch 2 (G0S2), intercellular adhesion molecule 5 (ICAM5), interleukin 36 beta (IL36B), interleukin 6 (IL6), IL2 inducible T cell kinase (ITK), phosphodiesterase 4D (PDE4D), patatin-like phospholipase domain containing 1 (PNPLA1), RAS guanyl releasing protein 1 (RASGRP1), Ras related glycolysis inhibitor and calcium channel regulator (RRAD), signal transducer and activator of transcription 4 (STAT4), and tumor necrosis factor-alpha induced protein 6 (TNFAIP6).
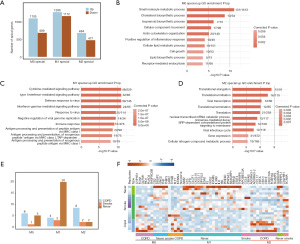
Identification and target prediction of miRNAs in M0, M1, and M2 cells
There were 367 common DEmiRNAs detected in M0, M1, and M2 specific cells of the GSE51307 dataset (Figure 3A). The PCA analysis demonstrated that the normalized miRNAs expression level could distinguish cells into different groups, being 13.8% and 10.2% for dim1 and dim2, respectively (Figure 3B). The non-normalized miRNAs expression levels of PCA analysis are shown in Figure S3A,S3B. The result revealed that the miRNA expression levels were significantly different in M0, M1, and M2 specific cells. The number of down-regulated DEmiRNAs were 61, 45, and 79 in M0, M1, and M2 specific cells, respectively (Figure 3C), and the number of up-regulated DEmiRNAs were 96, 75, and 26 in M0, M1, and M2 specific cells, respectively. The targeted mRNAs of miRNAs were predicted and compared with the database of miRBase and the number of up-regulated and down-regulated targeted mRNAs in M0, M1, and M2 specific cells are shown in Figure 3D. It can be seen from the heatmap that the population-specific genes are down-regulated in their own samples (Figure 3E). The expression heatmap of all significant up-regulated miRNAs among M0, M1, M2 samples showed that the population-specific genes were up-regulated in their own samples (Figure S3C).
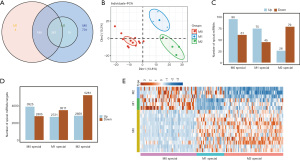
Abnormal expression of macrophages M1 specific genes regulated by miRNAs
The number of up- /down-regulated mRNAs targeted by down-/up-regulated miRNAs in polarization cells of M0, M1, and M2 is shown in Figure 4A. There were 171, 150, and 94 DEmRNAs detected as up-regulated, 128, 243, and 186 DE mRNAs detected as down-regulated in M0, M1, and M2 specific cells, respectively (Figure 4A). The up-regulated DEmRNAs in M0 specific cells were mainly enriched in GO terms of in utero embryonic development, protein transport, the insulin receptor signaling pathway, transmembrane transport, the small molecule metabolic process, and membrane organization (Figure 4B). Meanwhile, the DEmRNAs in M1 specific cells were mainly involved in GO terms of negative regulation of transcription from RNA polymerase II promoter, small GTPase mediated signal transduction, the cell surface receptor signaling pathway, multicellular organism growth, transcription DNA-dependent, positive regulation of transcription from RNA polymerase II promoter, and endosomal transport (Figure 4C). Moreover, the DEmRNAs in M2 specific cells were mostly involved in GO terms of protein transport, intracellular signal transduction, small GTPase mediated signal transduction, regulation of cell proliferation, the epidermal growth factor receptor signaling pathway, and signal transduction (Figure 4D). The top 10 most enriched GO terms which overlapped in the M0, M1, and M2 samples were specific down-regulated genes and the M0, M1, and M2 samples were specific up-regulated miRNA target genes (Figure S4A-S4C). After comparing the up-regulated DEmRNAs in polarization cells with those in clinical specific samples, only 7 common DEmRNAs were retained (Figure 4E). As shown in Figure 4E, none of the DEmRNAs in M0 were verified in clinical samples, implying an irrelevant effect by high expression in M0 cells. A solitary up-regulated DEmRNA in COPD specific samples was also up-regulated in M1 specific cells, which is suggested to be regulatory in M1 cells with COPD. A total of 5 DEmRNAs that were up-regulated in Never specific samples were abnormally regulated genes in COPD and Smoke specific samples. Among the up-regulated DEmRNAs in M2 cells, only 1 was verified by Smoke specific samples. These 7 genes were involved in Reactome terms of neurotrophic growth factor (NGF)-stimulated transcription, nuclear events (kinase and transcription factor activation), signaling by NTRK1, and signaling by receptor tyrosine kinases (Figure 4F). The network between DEmiRNAs (hsa-miR-30a-5p, hsa-miR-200c-3p, hsa-miR-20b-5p, hsa-miR-199b-5p, and hsa-miR-301b-3p) and their targeted DEmRNAs [F3, CLCF1, CALHM1, EDN1, RRAD, fms related receptor tyrosine kinase 1 (FLT1), and PDE4D] are shown in Figure 4G. The expression levels of hsa-miR-30a-5p, hsa-miR-200c-3p, hsa-miR-20b-5p, hsa-miR-199b-5p, and hsa-miR-301b-3p in M0, M1, and M2 are shown in Figure 4H. Their expression levels in M1 macrophages were all lower than that of M0 (P<0.01 or P<0.001). Their expression levels in M2 macrophages compared with M1 varied, with higher expression in hsa-miR-30a-5p, hsa-miR-20b-5p (P<0.001), and lower expression in hsa-miR-200c-3p, and hsa-miR-301b-3p (P<0.05 or P<0.001). The expression levels of F3, CLCF1, CALHM1, EDN1, RRAD, FLT1, and PDE4D in clinical specific samples of COPD, Smoke, and Never were evaluated and are shown in Figure 4I. Among the 7 genes, F3, CLCF1, FLT1, and PDE4D were validated in COPD and Never specific samples, indicating a correlation with COPD. The expression levels of F3, CLCF1, and PDE4D were significantly higher in Never than that in COPD and Smoke (P<0.001). The expression level of PDE4D was significantly higher in Smoke than COPD (P<0.001). The expression level of FLT1 was significantly lower in Smoke than COPD (P<0.001).
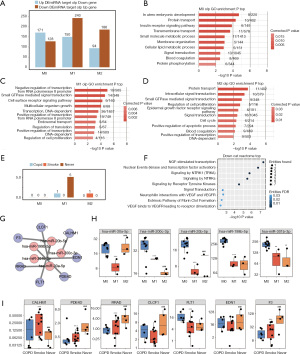
Discussion
In the present work, a comprehensive molecular study was performed to seek biomarkers that might play an essential role in COPD patients. The study found that the specifically up-regulated genes in the three groups were all enriched in an immune response. An inflammatory response was also enriched by up-regulated mRNAs in the Never specific group. Immune and inflammation responses were reported to play essential roles in the development and progression of COPD (36). Cigarette smoke can trigger pattern recognition receptors to release a “danger signal”, which activates innate immune cells and is liganded to toll-like receptors, thus producing cytokines and inducing innate inflammation. Additionally, the number of inflammation-related genes specifically up-regulated in Never samples was more than that in Smoke and COPD patients, indicating an inhibition of gene expression in Smoke and COPD samples. The result demonstrated that the genes regulating immune and inflammation responses were dysregulated in COPD. After verification in clinical specific samples, four genes were detected to be COPD-related: FLT1, CLCF1, PDE4D, and F3.
The gene FLT1, also known as vascular endothelial growth factor receptor-1 (VEGFR-1), is a novel cell surface marker for the monocyte-macrophage lineage in humans (37). Moreover, in another study, it was demonstrated that PMA induces FLT1 gene transcription by introducing Egr-1 in THP-1 cells, thus ensuring the FLT1 gene function on macrophage differentiation (38). These studies confirmed the role of FLT1 in macrophage differentiation, which was also inflammation-related. Kranenburg et al. (39) demonstrated that FLT1 is involved in peripheral vascular and airway remodeling processes in COPD. They also revealed ongoing inflammatory processes resulting in airway and vascular remodeling characterize COPD, which was consistent with the result that FLT1 was enriched in inflammatory processes. Wang et al. (40) suggested that the FLT1 variant could reduce COPD risk by lowering FLT1 mRNA expression. The vascular protecting effects of FLT1 were observed with fluid resuscitation, contributing to the pathophysiological changes seen in hypovolemia (41). Taken together, FLT1 is a vital gene for COPD, which is up-regulated in COPD samples and induces the differentiation of macrophages.
The gene CLCF1 was reported to activate Janus kinase/signal transduction, an activator of transcription (JAK-STAT) signaling cascade (42), thus regulating the processes of inflammation, COPD, and other lung-related diseases (43,44). Banerjee et al. (43) demonstrated that the JAK-STAT pathway transduces downstream of multiple cytokines is critical for the pathogenesis of immune-mediated disease. The Liuweibuqi capsule was suggested to effectively ameliorate the progression of COPD via the JAK/STAT pathway (44). The expression level of CLCF1 was significantly increased in Schwann cells and macrophages (45). However, the expression of CLCF1 in Smoke and COPD was inhibited in the present study, which might induce a suppressed JAK-STAT signaling pathway, thus promoting inflammation.
The F3 gene encodes coagulation factor III, which is a cell surface glycoprotein (46). Abnormal coagulation function can easily cause COPD complications, such as pulmonary embolism and deep vein thrombosis (47,48). The potential impact of inflammation on blood clotting provides the possibility of thrombosis in smokers, however the relationship among smoking, COPD, and blood clotting remain to be verified (49,50). In the present study, we confirmed the relationship among the expression of F3, COPD, and macrophage cells, which provides a basis for further study.
The PDE4D gene is a key subtype of PDE4, which was detected in many inflammatory cells, including T-cells, eosinophils, neutrophils, and monocytes (51). Moreover, PDE4 inhibitors can contribute to anti-inflammatory actions in animal models and reduce markers of inflammation in COPD (52-54). The PDE4 inhibitor roflumilast reduces exacerbations with COPD (55,56). Singh et al. (57) reported that the inhaled PDE4 inhibitor CHF6001 can be a useful biomarker of inflammation in COPD. Lea et al. (58) demonstrated that the expression of PED4D was increased in alveolar macrophages and COPD. PDE4 inhibitor causes decreased collagen and fibroblast activation by reducing the release of profibrotic cytokines from M2 macrophage and inflammatory cell activity (59). Therefore, we assumed that PDE4D might contribute to the inflammatory on COPD and promote M2 macrophage polarization. The PDE4 inhibitor could be used as a therapeutic biomarker for COPD by reducing inflammation. Taken together, these four genes were all correlated with the inflammation of COPD.
As reported, non-typeable Haemophilus influenzae causes macrophage polarization, and the anti-inflammatory action was enhanced by the p38 MAPK inhibitor combined with corticosteroids during exposure of COPD alveolar macrophage (60). Knockdown of MIR155HG can promote M2 macrophage polarization, inhibit M1 macrophage polarization, and release of anti-inflammatory cytokines (12). The polarization state and COPD are both correlated with inflammation. Therefore, we further studied the component of immune cells in clinical samples, and found that the proportion of M0 and M2 in Smoke and COPD samples was much higher than that in Never samples. This result was consistent with the previous finding that the peripheral blood of patients with severe COPD is mainly polarized by M2 macrophages (61). In addition, we also hinted that M1 macrophages can play an important role in the Never population. This brought attention to the study of GO analysis on M1 cells that found that the up-regulated genes were mainly inflammation, immunization, and viral response related, which confirmed the essential regulating role of M1 macrophages in inflammation and immunization (12). Furthermore, the clinical specific sample verification study indicated that the genes up-regulated in M1 were inhibited in Smoke and COPD samples. The GO analysis suggested that those genes are inflammation and immunization related.
As reported, airway viral infections can affect COPD by triggering innate immune responses and elevating inflammation (62). Bauer et al. (63) also confirmed the role of cigarette smoke on the immune system and inflammatory responses. Several viruses such as human rhinovirus (HRV), human coronavirus (HCoV), human metapneumovirus (HMPV), human adenovirus (HAdV), influenza virus (IFV), and respiratory syncytial virus (RSV) have a severe influence on COPD (64), yet the pathogeneses are unknown. In the present study, we found that up-regulated DEmRNAs of M1 specific cells were virus and immune related. According to this, we suspected that the pathogenesis of viruses on COPD might be correlated with mRNAs up-regulated in M1 specific cells, which needs further confirmation.
Not only the mRNA profile but also the miRNA profiles of polarization cells were performed. The PCA analysis on polarization cells revealed that macrophage cells can be clearly distinguished by the expression profile of all miRNAs, which was revealed for the first time in our present study. The result indicated that the overall expression characteristics of miRNAs are obviously different in macrophage cells, suggesting that miRNAs can be used as potential biomarkers for macrophage polarization. Hsa-miR-30a-5p was reported to be a potential biomarker for tobacco exposure (65). The axis of lncRNA ASLNCS5088/miR-200c-3p/GLS/αSMA promotes the M2 macrophages polarization (66). The result was consistent with our present study that the miR-200c-3p was lower in M2 macrophages than other macrophages.
In summary, the present study revealed for the first time the composition of macrophages (M0, M1, and M2) in COPD patients, never smokers, and healthy smokers. Moreover, the proportion of M1 cells was lower than that of M0 and M2 cells in healthy smokers and COPD patients. Most of the genes specifically up-regulated in M1 are related to inflammation/immunity, and these genes were suppressed in Smoke and COPD samples, which suggest that M1 plays a very important role in COPD. The genes FLT1, CLCF1, PDE4D, and F3 were found to be correlated with COPD through inflammation. Therefore, we speculate that miRNA regulates the expression of these four genes to play a role in macrophage polarization and then participates in COPD. This research has established a miRNA-mRNA regulatory network and obtained mRNAs that have critical regulatory functions in macrophage polarization which has laid the foundation for finding effective disease markers and developing reasonable treatment methods. However, the small sample size was a major limitation of the present study, and larger sample studies are needed to validate our findings.
Conclusions
The COPD and polarization macrophage are regulated by immune and inflammation related genes, in which the M1 macrophage might be more important than M0 and M2.
Acknowledgments
Thanks to Dr. Edward C. Mignot at Shandong University, for their linguistic advice.
Funding: This work was supported by research grants from Applied Basic Research Foundation of Yunnan Province (2019FB090), Applied Basic Research (Kunming Medical Joint Special Project) Foundation of Yunnan Province (2017FE468(-058)), Yunnan Provincial Health Committee Medical Reserve Talent Training Plan (H-2018096), and National Natural Science Foundation of China (81860012).
Footnote
Reporting Checklist: The authors have completed the STREGA reporting checklist. Available at https://dx.doi.org/10.21037/atm-21-3815
Conflicts of Interest: All authors have completed the ICMJE uniform disclosure form (available at https://dx.doi.org/10.21037/atm-21-3815). The authors have no conflicts of interest to declare.
Ethical Statement: The authors are accountable for all aspects of the work in ensuring that questions related to the accuracy or integrity of any part of the work are appropriately investigated and resolved. The study was conducted in accordance with the Declaration of Helsinki (as revised in 2013).
Open Access Statement: This is an Open Access article distributed in accordance with the Creative Commons Attribution-NonCommercial-NoDerivs 4.0 International License (CC BY-NC-ND 4.0), which permits the non-commercial replication and distribution of the article with the strict proviso that no changes or edits are made and the original work is properly cited (including links to both the formal publication through the relevant DOI and the license). See: https://creativecommons.org/licenses/by-nc-nd/4.0/.
References
- World Health Organization. 2019. (Accessed 19 April 2019). Available online: http://www.who.int/respiratory/copd/en/
- Singh D, Agusti A, Anzueto A, et al. Global Strategy for the Diagnosis, Management, and Prevention of Chronic Obstructive Lung Disease: the GOLD science committee report 2019. Eur Respir J 2019;53:1900164 [Crossref] [PubMed]
- Barnes PJ. Inflammatory mechanisms in patients with chronic obstructive pulmonary disease. J Allergy Clin Immunol 2016;138:16-27. [Crossref] [PubMed]
- Dang X, Qu X, Wang W, et al. Bioinformatic analysis of microRNA and mRNA Regulation in peripheral blood mononuclear cells of patients with chronic obstructive pulmonary disease. Respir Res 2017;18:4. [Crossref] [PubMed]
- Qu J, Yue L, Gao J, et al. Perspectives on Wnt Signal Pathway in the Pathogenesis and Therapeutics of Chronic Obstructive Pulmonary Disease. J Pharmacol Exp Ther 2019;369:473-80. [Crossref] [PubMed]
- Rahman I, Kinnula VL, Gorbunova V, et al. SIRT1 as a therapeutic target in inflammaging of the pulmonary disease. Prev Med 2012;54:S20-8. [Crossref] [PubMed]
- Akata K, van Eeden SF. Lung Macrophage Functional Properties in Chronic Obstructive Pulmonary Disease. Int J Mol Sci 2020;21:853. [Crossref] [PubMed]
- Yamasaki K, Eeden SFV. Lung macrophage phenotypes and functional responses: role in the pathogenesis of COPD. Int J Mol Sci 2018;19:582. [Crossref] [PubMed]
- Verreck FA, de Boer T, Langenberg DM, et al. Human IL-23-producing type 1 macrophages promote but IL-10-producing type 2 macrophages subvert immunity to (myco)bacteria. Proc Natl Acad Sci U S A 2004;101:4560-5. [Crossref] [PubMed]
- Vidyarthi A, Khan N, Agnihotri T, et al. TLR-3 Stimulation Skews M2 Macrophages to M1 Through IFN-αβ Signaling and Restricts Tumor Progression. Front Immunol 2018;9:1650. [Crossref] [PubMed]
- Yunna C, Mengru H, Lei W, Weidong C. Macrophage M1/M2 polarization. Eur J Pharmacol 2020;877:173090 [Crossref] [PubMed]
- Li N, Liu Y, Cai J. LncRNA MIR155HG regulates M1/M2 macrophage polarization in chronic obstructive pulmonary disease. Biomed Pharmacother 2019;117:109015 [Crossref] [PubMed]
- He S, Chen D, Hu M, et al. Bronchial epithelial cell extracellular vesicles ameliorate epithelial-mesenchymal transition in COPD pathogenesis by alleviating M2 macrophage polarization. Nanomedicine 2019;18:259-71. [Crossref] [PubMed]
- Shaykhiev R, Krause A, Salit J, et al. Smoking-dependent reprogramming of alveolar macrophage polarization: implication for pathogenesis of chronic obstructive pulmonary disease. J Immunol 2009;183:2867-83. [Crossref] [PubMed]
- Hiemstra PS. Altered macrophage function in chronic obstructive pulmonary disease. Ann Am Thorac Soc 2013;10:S180-5. [Crossref] [PubMed]
- Zhao Y, Li M, Yang Y, et al. Identification of macrophage polarization-related genes as biomarkers of chronic obstructive pulmonary disease based on bioinformatics analyses. Biomed Res Int 2021;2021:9921012 [Crossref] [PubMed]
- Bartel DP. MicroRNAs: target recognition and regulatory functions. Cell 2009;136:215-33. [Crossref] [PubMed]
- Booton R, Lindsay MA. Emerging role of MicroRNAs and long noncoding RNAs in respiratory disease. Chest 2014;146:193-204. [Crossref] [PubMed]
- Huang X, Zhu Z, Guo X, et al. The roles of microRNAs in the pathogenesis of chronic obstructive pulmonary disease. Int Immunopharmacol 2019;67:335-47. [Crossref] [PubMed]
- Lacedonia D, Palladino GP, Foschino-Barbaro MP, et al. Expression profiling of miRNA-145 and miRNA-338 in serum and sputum of patients with COPD, asthma, and asthma-COPD overlap syndrome phenotype. Int J Chron Obstruct Pulmon Dis 2017;12:1811-7. [Crossref] [PubMed]
- Spira A, Beane J, Shah V, et al. Effects of cigarette smoke on the human airway epithelial cell transcriptome. Proc Natl Acad Sci U S A 2004;101:10143-8. [Crossref] [PubMed]
- Tilley AE, O'Connor TP, Hackett NR, et al. Biologic phenotyping of the human small airway epithelial response to cigarette smoking. PLoS One 2011;6:e22798 [Crossref] [PubMed]
- Beineke P, Fitch K, Tao H, et al. A whole blood gene expression-based signature for smoking status. BMC Med Genomics 2012;5:58. [Crossref] [PubMed]
- Huan T, Joehanes R, Schurmann C, et al. A whole-blood transcriptome meta-analysis identifies gene expression signatures of cigarette smoking. Hum Mol Genet 2016;25:4611-23. [Crossref] [PubMed]
- Steiling K, Lenburg ME, Spira A. Airway gene expression in chronic obstructive pulmonary disease. Proc Am Thorac Soc 2009;6:697-700. [Crossref] [PubMed]
- Steiling K, van den Berge M, Hijazi K, et al. A dynamic bronchial airway gene expression signature of chronic obstructive pulmonary disease and lung function impairment. Am J Respir Crit Care Med 2013;187:933-42. [Crossref] [PubMed]
- Obeidat M, Nie Y, Chen V, et al. Network-based analysis reveals novel gene signatures in peripheral blood of patients with chronic obstructive pulmonary disease. Respir Res 2017;18:72. [Crossref] [PubMed]
- Reinhold D, Morrow JD, Jacobson S, et al. Meta-analysis of peripheral blood gene expression modules for COPD phenotypes. PLoS One 2017;12:e0185682 [Crossref] [PubMed]
- Yeo J, Morales DA, Chen T, et al. RNAseq analysis of bronchial epithelial cells to identify COPD-associated genes and SNPs. BMC Pulm Med 2018;18:42. [Crossref] [PubMed]
- Parker MM, Chase RP, Lamb A, et al. RNA sequencing identifies novel non-coding RNA and exon-specific effects associated with cigarette smoking. BMC Med Genomics 2017;10:58. [Crossref] [PubMed]
- Hiemstra PS, McCray PB Jr, Bals R. The innate immune function of airway epithelial cells in inflammatory lung disease. Eur Respir J 2015;45:1150-62. [Crossref] [PubMed]
- Morrow JD, Chase RP, Parker MM, et al. RNA-sequencing across three matched tissues reveals shared and tissue-specific gene expression and pathway signatures of COPD. Respir Res 2019;20:65. [Crossref] [PubMed]
- Xue J, Schmidt SV, Sander J, et al. Transcriptome-based network analysis reveals a spectrum model of human macrophage activation. Immunity 2014;40:274-88. [Crossref] [PubMed]
- Robinson MD, McCarthy DJ, Smyth GK. edgeR: a Bioconductor package for differential expression analysis of digital gene expression data. Bioinformatics 2010;26:139-40. [Crossref] [PubMed]
- Zheng X, Wu K, Liao S, et al. MicroRNA-transcription factor network analysis reveals miRNAs cooperatively suppress RORA in oral squamous cell carcinoma. Oncogenesis 2018;7:79. [Crossref] [PubMed]
- Rovina N, Koutsoukou A, Koulouris NG. Inflammation and immune response in COPD: where do we stand? Mediators Inflamm 2013;2013:413735 [Crossref] [PubMed]
- Sawano A, Iwai S, Sakurai Y, et al. Flt-1, vascular endothelial growth factor receptor 1, is a novel cell surface marker for the lineage of monocyte-macrophages in humans. Blood 2001;97:785-91. [Crossref] [PubMed]
- Akuzawa N, Kurabayashi M, Ohyama Y, et al. Zinc finger transcription factor Egr-1 activates Flt-1 gene expression in THP-1 cells on induction for macrophage differentiation. Arterioscler Thromb Vasc Biol 2000;20:377-84. [Crossref] [PubMed]
- Kranenburg AR, de Boer WI, Alagappan VK, et al. Enhanced bronchial expression of vascular endothelial growth factor and receptors (Flk-1 and Flt-1) in patients with chronic obstructive pulmonary disease. Thorax 2005;60:106-13. [Crossref] [PubMed]
- Wang H, Yang L, Deng J, et al. Genetic variant in the 3'-untranslated region of VEGFR1 gene influences chronic obstructive pulmonary disease and lung cancer development in Chinese population. Mutagenesis 2014;29:311-7. [Crossref] [PubMed]
- Ekerbicer N, Tarakci F, Barut T, et al. Immunolocalization of VEGF, VEGFR-1 and VEGFR-2 in lung tissues after acute hemorrhage in rats. Acta Histochem 2008;110:285-93. [Crossref] [PubMed]
- Dagoneau N, Bellais S, Blanchet P, et al. Mutations in cytokine receptor-like factor 1 (CRLF1) account for both Crisponi and cold-induced sweating syndromes. Am J Hum Genet 2007;80:966-70. [Crossref] [PubMed]
- Banerjee S, Biehl A, Gadina M, et al. JAK-STAT signaling as a target for inflammatory and autoimmune diseases: current and future prospects. Drugs 2017;77:521-46. [Crossref] [PubMed]
- Wang C, Ding H, Tang X, et al. Effect of Liuweibuqi Capsules in Pulmonary Alveolar Epithelial Cells and COPD Through JAK/STAT Pathway. Cell Physiol Biochem 2017;43:743-56. [Crossref] [PubMed]
- Choi K, Komurov K, Fletcher JS, et al. An inflammatory gene signature distinguishes neurofibroma Schwann cells and macrophages from cells in the normal peripheral nervous system. Sci Rep 2017;7:43315. [Crossref] [PubMed]
- Houreld NN, Ayuk SM, Abrahamse H. Expression of genes in normal fibroblast cells (WS1) in response to irradiation at 660nm. J Photochem Photobiol B 2014;130:146-52. [Crossref] [PubMed]
- Wang YT, Yu NW. Effects of low molecular weight heparin on the function of blood coagulation and serum levels of TNF-α, CK-MB, CRP of patients with acute exacerbations of chronic obstructive pulmonary diseases and respiratory failur. Journal of Hainan Medical University 2017;23:52-5.
- Liu S, Guo H, Song Z. Correlation analysis of immune function, coagulation function and pulmonary function in patients with pulmonary tuberculosis complicated with COPD. International Journal of Laboratory Medicine 2018;39:312-5.
- Tapson VF. The role of smoking in coagulation and thromboembolism in chronic obstructive pulmonary disease. Proc Am Thorac Soc 2005;2:71-7. [Crossref] [PubMed]
- Aleva F. Inflammation, coagulation and their interaction in chronic obstructive pulmonary disease. Radboud Repository. 2018; Available online: http://hdl.handle.net/2066/182821
- Phillips JE. Inhaled Phosphodiesterase 4 (PDE4) Inhibitors for Inflammatory Respiratory Diseases. Front Pharmacol 2020;11:259. [Crossref] [PubMed]
- Dunne AE, Kawamatawong T, Fenwick PS, et al. Direct Inhibitory Effect of the PDE4 Inhibitor Roflumilast on Neutrophil Migration in Chronic Obstructive Pulmonary Disease. Am J Respir Cell Mol Biol 2019;60:445-53. [Crossref] [PubMed]
- Gamble E, Grootendorst DC, Brightling CE, et al. Antiinflammatory effects of the phosphodiesterase-4 inhibitor cilomilast (Ariflo) in chronic obstructive pulmonary disease. Am J Respir Crit Care Med 2003;168:976-82. [Crossref] [PubMed]
- Grootendorst DC, Gauw SA, Verhoosel RM, et al. Reduction in sputum neutrophil and eosinophil numbers by the PDE4 inhibitor roflumilast in patients with COPD. Thorax 2007;62:1081-7. [Crossref] [PubMed]
- Rennard SI, Calverley PM, Goehring UM, et al. Reduction of exacerbations by the PDE4 inhibitor roflumilast--the importance of defining different subsets of patients with COPD. Respir Res 2011;12:18. [Crossref] [PubMed]
- Martinez FJ, Calverley PM, Goehring UM, et al. Effect of roflumilast on exacerbations in patients with severe chronic obstructive pulmonary disease uncontrolled by combination therapy (REACT): a multicentre randomised controlled trial. Lancet 2015;385:857-66. [Crossref] [PubMed]
- Singh D, Beeh KM, Colgan B, et al. Effect of the inhaled PDE4 inhibitor CHF6001 on biomarkers of inflammation in COPD. Respir Res 2019;20:180. [Crossref] [PubMed]
- Lea S, Metryka A, Li J, et al. The modulatory effects of the PDE4 inhibitors CHF6001 and roflumilast in alveolar macrophages and lung tissue from COPD patients. Cytokine 2019;123:154739 [Crossref] [PubMed]
- Maier C, Ramming A, Bergmann C, et al. Inhibition of phosphodiesterase 4 (PDE4) reduces dermal fibrosis by interfering with the release of interleukin-6 from M2 macrophages. Ann Rheum Dis 2017;76:1133-41. [Crossref] [PubMed]
- Khalaf RM, Lea SR, Metcalfe HJ, et al. Mechanisms of corticosteroid insensitivity in COPD alveolar macrophages exposed to NTHi. Respir Res 2017;18:61. [Crossref] [PubMed]
- Cornwell WD, Kim V, Fan X, et al. Activation and polarization of circulating monocytes in severe chronic obstructive pulmonary disease. BMC Pulm Med 2018;18:101. [Crossref] [PubMed]
- Matsumoto K, Inoue H. Viral infections in asthma and COPD. Respir Investig 2014;52:92-100. [Crossref] [PubMed]
- Bauer CMT, Morissette MC, Stämpfli MR. The influence of cigarette smoking on viral infections: translating bench science to impact COPD pathogenesis and acute exacerbations of COPD clinically. Chest 2013;143:196-206. [Crossref] [PubMed]
- Frickmann H, Jungblut S, Hirche TO, et al. The influence of virus infections on the course of COPD. Eur J Microbiol Immunol (Bp) 2012;2:176-85. [Crossref] [PubMed]
- Singh KP, Maremanda KP, Li D, et al. Exosomal microRNAs are novel circulating biomarkers in cigarette, waterpipe smokers, E-cigarette users and dual smokers. BMC Med Genomics 2020;13:128. [Crossref] [PubMed]
- Chen J, Zhou R, Liang Y, et al. Blockade of lncRNA-ASLNCS5088-enriched exosome generation in M2 macrophages by GW4869 dampens the effect of M2 macrophages on orchestrating fibroblast activation. FASEB J 2019;33:12200-12. Erratum in: FASEB J 2020;34:11307-10. [Crossref] [PubMed]
(English Language Editor: J. Jones)