CCR2 and PTPRC are regulators of tumor microenvironment and potential prognostic biomarkers of lung adenocarcinoma
Introduction
Lung cancer is one of the malignant tumors that threaten human health (1), among which adenocarcinoma is the most common histological type of non-small cell lung cancer (NSCLC), accounting for approximately 60% of NSCLC (2). Given the early symptoms of lung cancer are nonspecific, a vast majority of patients are diagnosed at advanced stages of the disease (3). Although great progress has been made in the early diagnosis and treatment of lung cancer in recent years, the exploration of prognostic biomarker and novel therapeutic targets in lung cancer is still unsatisfactory (4,5). Currently, immunotherapy have brought a new direction to the clinical treatment of lung cancer, and a variety of immune checkpoint inhibitors (ICIs) have shown encouraging efficacy in clinical trials (6,7). However, some patients have not benefited from immunotherapy (8) or developed drug resistance during medication (9), and its side effects and adverse reactions are still a worrying issue. Accordingly, it is still necessary to explore and ascertain the pathogenic mechanism of lung adenocarcinoma (LUAD) to break through the current bottleneck.
Tumor microenvironment (TME) is a complex ecosystem composed of various types of cells, which can be divided into immune components dominated by immune cells and matrix components dominated by fibroblasts (10). Most of the cancer characteristics are activated and sustained by varying degrees through contributions from the distinctive cells of TME. They mediate the recruitment, activation, programming and persistence of tumor cells in a variety of ways (11). A growing body of studies have elucidated that tumor-infiltrating immune cells (TICs) can be used as a predictor of cancer prognosis and treatment target (12,13). Nevertheless, Further studies have found that TME components have great heterogeneity among different tumor stages and individuals, thereby leading to the differences in prognosis (14,15). For instance, in liver cancer, tumors with higher transcriptome diversity have lower T cell cytolytic activity, which was related to the poor prognosis (16). Likewise, in colorectal cancer increased M1 macrophage levels indicated shorter overall survival (OS) of patients (17). This heterogeneity not only results in differences in patient outcome, but also increases the difficulty for clinicians to use targeted drugs (18). Therefore, the extensive search for novel and effective TME targeting sites may provide a new window of opportunity for combined immunotherapy (19).
In this study, we used ESTIMATE algorithm to calculate the TME components of LUAD samples in the Cancer Genome Atlas (TCGA) database, and CIBERSORT algorithms were used to calculate the relative contents of various TICs, combining with univariate Cox regression analysis and protein-protein interaction (PPI) network to screen differentially expressed genes (DEGs) and finally obtained predictive biomarkers CCR2 and PTPRC. CCR2 is the receptor for monocyte chemotactic protein-1 (MCP-1), which were expressed by a variety of cell types, including monocytes, dendritic cells (DC), endothelial cells and cancer cells. The upregulation of MCP-1 was related to formation, metastasis and recurrence of multiple types of cancers (20,21). Nevertheless, some studies have indicated that the high expression of CCR2 inhibited the development of small cell lung cancer (SCLC) (22). The protein tyrosine phosphatase CD45, encoded by the PTPRC gene, which has thought to be a regulator of B cell and T cell receptor signaling, it has been reported that PTPRC expression was significantly down-regulated in acute lymphoblastic leukemia and Parkinson's disease (23,24). In patients with SCLC, its expression was positively correlated with the patients’ survival (25). Here, our study revealed that the CCR2 and PTPRC might be a potential indicator for the alteration of TME status in LUAD. We present the following article in accordance with the REMARK reporting checklist (available at https://dx.doi.org/10.21037/atm-21-3301).
Methods
Extraction of data
Through the TCGA database (https://portal.gdc.cancer.gov/), we obtained RNA-seq data and clinical characteristics of 594 LUAD samples (535 tumor samples and 59 healthy samples). The study was conducted in accordance with the Declaration of Helsinki (as revised in 2013).
Analyzing of ImmuneScore, StromalScore, and ESTIMATEScore
We analyzed the immune and matrix components in the TME using R language version 4.0.3 loaded with estimate package, presented by ImmuneScore, StromalScore, and ESTIMATEScore. The higher the score reflects the higher corresponding component in TME. ESTIMATEScore represents the sum of immune and matrix components.
Survival analysis
The survival and survminer R package were used for survival analysis. Kaplan-Meier analysis was used to perform the survival curve as well, P<0.05 was considered significant.
Analysis of correlation between clinical characteristics and scores
We analyzed the relationship between clinical characteristics and different scores of LUAD patients downloaded from the TCGA via R language, and used the Wilcoxon rank sum test or the Kruskal-Wallis rank sum test as a test of significance.
Screening of DEGs
LUAD samples were marked as high or low score group based on the median of ImmuneScore and StromalScore. We used the package “limma” to analyze the DEGs between the high and low score groups. DEGs with |log2FC|>1 and false discovery rate (FDR) <0.05 were statistically significant. R language with package pheatmap was used to construct the heatmaps of DEGs.
Enrichment function analysis
To explore the functions of the DEGs, we used clusterProfiler, enrichplot, and ggplot2 R package for the analysis. Terms with P value and q value <0.05 were considered significant.
PPI network construction
The STRING public database (https://string-db.org/) was used to generate the PPI network, and results were reconstructed with Cytoscape version 3.8.1. The nodes with the confidence of interactive association more than 0.7 were selected to generate the PPI network.
Univariate COX regression analysis
R language combined with “survival” package was used in univariate Cox regression analysis. DEGs with a value of P<0.05 were selected for following analysis.
TICs analysis
We used the CIBERSORT algorithm on 535 tumor samples to calculate the relative contents of 22 types of TICs in each LUAD sample, samples with P<0.05 were used for subsequent analysis.
Statistical analysis
Statistical analysis was performed using with R (version 4.0.3, https://www.r-project.org/). P value <0.05 was considered to be statistically significant.
Results
Survival analysis of LUAD patients in three different scores
We combined ESTIMATE algorithms and Kaplan-Meier survival analysis to profile the relationship between different scores and patients’ prognosis. ImmuneScore, StromalScore and ESTIMATEScore showed a positive correlation with OS (Figure 1). The results above suggested that the immune and matrix components in the TME could reflect the prognosis of LUAD patients, indicating the potential value of each component of TME.
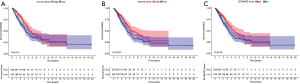
Correlation of scores with clinical characteristics of LUAD patients
Clinical characteristics were downloaded from the TCGA database, and the correlation analysis between three types of scores and clinical characteristics was carried out (Figure 2A-2O). As shown in Figure 2A-2C, males had lower scores relative to females. Meanwhile, three kinds of scores were negatively correlated with tumor stages (Figure 2D-2F). Further analysis demonstrated that scores decreased significantly from T1 to T4 in T classification (Figure 2G-2I), M classification also showed a negative correlation with scores. However, no statistically significant changes of scores were observed in N classification. In a word, results above demonstrated that the immune and matrix components in the TME correlated with tumor progression and metastasis.
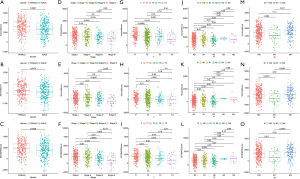
DEGs screening
In order to analyze the changes in the gene expression profile of immune and matrix components, we divided ImmuneScore and StromalScore into high and low score groups according to the median of scores, and the DEGs of the two groups were presented in heat maps (Figure 3A,3B), thus identifying 1,394 genes from ImmuneScore (including 1,097 up-regulated and 297 down-regulated genes), and 1,623 genes from StromalScore (including 1,422 up-regulated and 201 down-regulated genes). The intersection analysis of the two scores obtained 585 up-regulated and 107 down-regulated shared genes (Figure 3C,3D). These shared DEGs may exert dual effect on the immune and matrix component of TME.
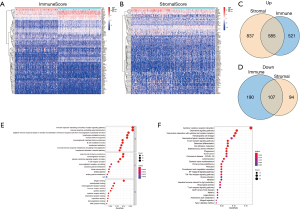
Functional enrichment analysis
Subsequently, we performed functional enrichment analysis for the shared DEGs, the results of gene ontology (GO) analysis showed that the functions of 692 shared DEGs in two groups were mainly concentrated on immune-related terms, including immune response-activating cell surface receptor signaling pathway, immune response-activating signal transduction, lymphocyte mediated immunity, and humoral immune response (Figure 3E). Meanwhile, Kyoto Encyclopedia of Genes and Genomes (KEGG) enrichment analysis indicated DEGs primarily enriched in cytokine-cytokine receptor interaction, chemokine signaling pathway, hematopoietic cell lineage and B cell receptor signaling pathway (Figure 3F). To sum up, DEGs obtained from the intersection analysis of ImmuneScore and StromalScore were enriched in immune function of TME, which may contain the key factors affecting immune state.
Intersection analysis of PPI network and univariate Cox regression analysis
We then constructed PPI network using STRING database to further screen the 692 shared genes. The top 30 genes sorted by the number of nodes were shown in bar plot (Figure 4A,4B). Univariate Cox regression analysis of the 692 shared genes were performed showing that 22 genes were related to the OS of LUAD (Figure 4C). Having performed the intersection analysis of PPI network and univariate Cox regression analysis, we ultimately obtained two overlapping genes CCR2 and PTPRC (Figure 4D).

Integrated analysis of CCR2 and PTPRC
Next, we performed integrated analysis of these two genes. As shown in Figure 5A,5B, the expression of CCR2 and PTPRC in tumors were significantly lower than that of normal samples. Survival analysis showed that patients in the high expression group of the two key genes had longer OS than those in the low expression group (Figure 5C,5D). Next, we further analyzed the correlation between the two genes and clinical characteristics (Figure 6A-6J), manifesting that CCR2 expression was negatively correlated with tumor stage of LUAD (Figure 6B), and its expression decreased as the T and N classification increased (Figure 6C,6D). Similar results were presented in the analysis of PTPRC (Figure 6H,6I). In conclusion, we can conclude that these two genes may affect the prognosis by affecting the immune state in TME based on the previous analysis, and they could be used as reliable biomarkers of LUAD prognosis.
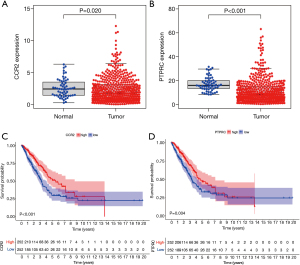
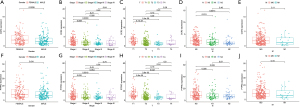
Correlation analysis between key genes and TICs
The CIBERSORT algorithm was performed on 535 tumor samples to calculate the relative content of 22 types of TICs in each sample, the relative content of TICs in tumor samples and correlations analysis between TICs were shown in Figure 7A,7B. The correlation analysis between the high and low expression groups of 2 key genes showed that 15 TICs were closely related to CCR2, including 10 positively related TIC subtypes and 5 negatively related TIC subtypes (Figure 8). 13 types of TICs were correlated with PTPRC, including 7 positively related TIC subtypes and 6 negatively related TIC subtypes (Figure 9). We subsequently divided LUAD patients into high and low groups based on median content of each TIC subtype and found that patients with a higher proportion of γδT cells and plasma cells had a better prognosis, while higher proportion of resting NK cells led to diametrically opposite results (Figure 10). Taken together, these two genes were key factor affecting immune state of LUAD.
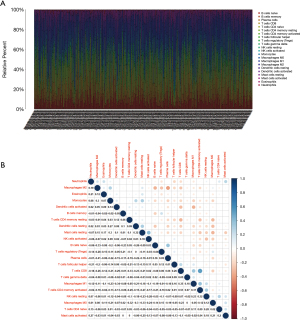

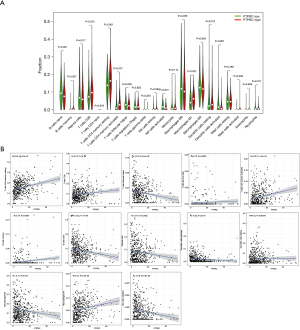
Discussion
Lung cancer is the most common cause of cancer-related deaths in the world’s population with a 5-year survival rate ranges from 4% to 17% depending on tumor stage and regional variation (26). According to statistics, there were 2.1 million lung cancer cases globally leading to 1.761 million deaths in 2018, ranking first in the incidence of malignant tumors (27). Although many advances have been made in understanding the mechanism of this disease, more than 1 million people die from lung cancer each year worldwide (28,29). Currently, the role of TME in tumorigenesis and cancer progression has become clearer (30), making it possible to predict treatment responsiveness and survival prognosis. Notably, the immune components in TME could mediate anti-tumor effect, and studies have indicated the correlation between tumor immune microenvironment and the efficacy of immunotherapy (31,32).
In our study, key genes, CCR2 and PTPRC, were obtained by analyzing the matrix and immune components of TME in LUAD. CCR2 was a chemokine receptor that regulated the immune response by inducing the recruitment of macrophages and monocytes to the site of inflammation (20). Intriguingly, numerous studies have elucidated that CCR2 was correlated with the occurrence and development of many diseases (20,33). High expression of CCR2 may lead to the poor prognosis of various cancers (21,34,35), and inhibition of CCR2 can enhance the therapeutic effect of PD-1 on tumor suppression (36). Paradoxically, the study by Zheng et al. proved that CCL2 was significantly down-regulated in SCLC and led to the proliferation of cancer cells (22). Our study indicated that the expression of CCR2 in LUAD was significantly lower compared with the corresponding normal tissue, and patients with high CCR2 expression had longer OS. Further analysis of the relationship between the expression of CCR2 and tumor stage shed bright light on the results that with the progress of T classification, the expression of CCR2 decreased. In a word, the above results demonstrated that CCR2 may play an anti-tumor effect in LUAD. In addition, the other key gene, PTPRC, encoded an evolutionarily highly conserved protein tyrosine phosphatase CD45, which was only expressed on nucleated cells of the hematopoietic system and was considered to be a regulator of B and T cell receptor signaling (24,37,38). In recent years, the role of CD45 in cancer and TME remained controversial. Chen et al. indicated that the accumulation of CD45+CD71+ erythroid cells in liver cancer may play an immunosuppressive effect in TME (39). Studies have confirmed that a higher proportion of CD45+ cells was closely related to the poor prognosis of NSCLC patients. However, in other types of tumors, high expression of CD45 may be related to the good prognosis of the tumor, indicating that CD45 may play a completely distinctive role in different tumors (24,25). Our study revealed that the expression of PTPRC in LUAD was significantly down-regulated compared with normal tissue, and patients with high PTPRC expression had better prognosis. Further analysis of the relationship between the expression of PTPRC and clinic characteristics indicated that with the progress of tumor stage, the expression of PTPRC decreased significantly.
We further calculated relative content of TICs of LUAD samples using CIBERSORT algorithm, the results found that patients with high proportion of γδT cells presented a better prognosis. γδT lymphocytes are a subset of T lymphocytes, which can directly inhibit tumor cells through cytotoxicity (40,41). It has been confirmed that γδT cells participated in the anti-tumor effect of lung cancer (42) and prostate cancer (43). Previous studies have confirmed that γδT cells have the property of suppressing and inhibiting a variety of tumor cell lines (44,45). However, some γδT lymphocyte subtypes were unexpected promoters for tumorigenesis and cancer development as their functions were affected by the immunosuppressive signal of TME (46). Our research found that the proportion of γδT cells was significantly correlated with the expression of CCR2 and PTPRC (P<0.05). This may suggest that CCR2 and PTPRC were the key factors that drive the anti-tumor effect of γδT cells in the TME. In summary, CCR2 and PTPRC may affect the function of immune cells, such as γδT cells, by participating in the regulation of the immune activity of TME and exert an impact on the prognosis of LUAD. CCR2 and PTPRC can be used as biomarkers to predict the immune response of TME and provide new therapeutic targets for LUAD. Notwithstanding, our study has some limitations. First, we still need to validate the findings of the study through in vivo and in vitro experiments. Secondly, we didn’t clarify the way in which key genes affect tumor immunity and exert anti-tumor effects.
Conclusions
Through bioinformatics to evaluate data in TCGA, our research showed that CCR2 and PTPRC may be potential prognostic markers in LUAD, which may affect the immune function of γδT cells and other immune cells by participating in the regulation of TME immune activity.
Acknowledgments
Funding: None.
Footnote
Reporting Checklist: The authors have completed the REMARK reporting checklist. Available at https://dx.doi.org/10.21037/atm-21-3301
Conflicts of Interest: All authors have completed the ICMJE uniform disclosure form (available at https://dx.doi.org/10.21037/atm-21-3301). The authors have no conflicts of interest to declare.
Ethical Statement: The authors are accountable for all aspects of the work in ensuring that questions related to the accuracy or integrity of any part of the work are appropriately investigated and resolved. The study was conducted in accordance with the Declaration of Helsinki (as revised in 2013).
Open Access Statement: This is an Open Access article distributed in accordance with the Creative Commons Attribution-NonCommercial-NoDerivs 4.0 International License (CC BY-NC-ND 4.0), which permits the non-commercial replication and distribution of the article with the strict proviso that no changes or edits are made and the original work is properly cited (including links to both the formal publication through the relevant DOI and the license). See: https://creativecommons.org/licenses/by-nc-nd/4.0/.
References
- Siegel RL, Miller KD, Jemal A. Cancer statistics, 2020. CA Cancer J Clin 2020;70:7-30. [Crossref] [PubMed]
- Warth A, Muley T, Meister M, et al. The novel histologic International Association for the Study of Lung Cancer/American Thoracic Society/European Respiratory Society classification system of lung adenocarcinoma is a stage-independent predictor of survival. J Clin Oncol 2012;30:1438-46. [Crossref] [PubMed]
- Lubuzo B, Ginindza T, Hlongwana K. Exploring barriers to lung cancer patient access, diagnosis, referral and treatment in Kwazulu-Natal, South Africa: the health providers’ perspectives. Transl Lung Cancer Res 2019;8:380-91. [Crossref] [PubMed]
- Buddharaju LN, Ganti AK. Immunotherapy in lung cancer: the chemotherapy conundrum. Chin Clin Oncol 2020;9:59. [Crossref] [PubMed]
- Bensussan AV, Lin J, Guo C, et al. Distinguishing Non-Small Cell Lung Cancer Subtypes in Fine Needle Aspiration Biopsies by Desorption Electrospray Ionization Mass Spectrometry Imaging. Clin Chem 2020;66:1424-33. [Crossref] [PubMed]
- Reck M, Rodriguez-Abreu D, Robinson AG, et al. Pembrolizumab versus Chemotherapy for PD-L1-Positive Non-Small-Cell Lung Cancer. N Engl J Med 2016;375:1823-33. [Crossref] [PubMed]
- Reck M, Rodriguez-Abreu D, Robinson AG, et al. Updated Analysis of KEYNOTE-024: Pembrolizumab Versus Platinum-Based Chemotherapy for Advanced Non-Small-Cell Lung Cancer With PD-L1 Tumor Proportion Score of 50% or Greater. J Clin Oncol 2019;37:537-46. [Crossref] [PubMed]
- Brahmer J, Reckamp KL, Baas P, et al. Nivolumab versus Docetaxel in Advanced Squamous-Cell Non-Small-Cell Lung Cancer. N Engl J Med 2015;373:123-35. [Crossref] [PubMed]
- Boyero L, Sanchez-Gastaldo A, Alonso M, et al. Primary and Acquired Resistance to Immunotherapy in Lung Cancer: Unveiling the Mechanisms Underlying of Immune Checkpoint Blockade Therapy. Cancers (Basel) 2020;12:3729. [Crossref] [PubMed]
- Pan H, Yu T, Sun L, et al. LncRNA FENDRR-mediated tumor suppression and tumor-immune microenvironment changes in non-small cell lung cancer. Transl Cancer Res 2020;9:3946-59. [Crossref]
- Hanahan D, Coussens LM. Accessories to the crime: functions of cells recruited to the tumor microenvironment. Cancer Cell 2012;21:309-22. [Crossref] [PubMed]
- Djenidi F, Adam J, Goubar A, et al. CD8+CD103+ tumor-infiltrating lymphocytes are tumor-specific tissue-resident memory T cells and a prognostic factor for survival in lung cancer patients. J Immunol 2015;194:3475-86. [Crossref] [PubMed]
- Kinoshita T, Muramatsu R, Fujita T, et al. Prognostic value of tumor-infiltrating lymphocytes differs depending on histological type and smoking habit in completely resected non-small-cell lung cancer. Ann Oncol 2016;27:2117-23. [Crossref] [PubMed]
- Maynard A, McCoach CE, Rotow JK, et al. Therapy-Induced Evolution of Human Lung Cancer Revealed by Single-Cell RNA Sequencing. Cell 2020;182:1232-51.e22. [Crossref] [PubMed]
- Netea-Maier RT, Smit JWA, Netea MG. Metabolic changes in tumor cells and tumor-associated macrophages: A mutual relationship. Cancer Lett 2018;413:102-9. [Crossref] [PubMed]
- Ma L, Hernandez MO, Zhao Y, et al. Tumor Cell Biodiversity Drives Microenvironmental Reprogramming in Liver Cancer. Cancer Cell 2019;36:418-30 e6. [Crossref] [PubMed]
- Ge P, Wang W, Li L, et al. Profiles of immune cell infiltration and immune-related genes in the tumor microenvironment of colorectal cancer. Biomed Pharmacother 2019;118:109228 [Crossref] [PubMed]
- Roma-Rodrigues C, Mendes R, Baptista PV, et al. Targeting Tumor Microenvironment for Cancer Therapy. Int J Mol Sci 2019;20:840. [Crossref] [PubMed]
- Belli C, Trapani D, Viale G, et al. Targeting the microenvironment in solid tumors. Cancer Treat Rev 2018;65:22-32. [Crossref] [PubMed]
- Hao Q, Vadgama JV, Wang P. CCL2/CCR2 signaling in cancer pathogenesis. Cell Commun Signal 2020;18:82. [Crossref] [PubMed]
- Nagarsheth N, Wicha MS, Zou W. Chemokines in the cancer microenvironment and their relevance in cancer immunotherapy. Nat Rev Immunol 2017;17:559-72. [Crossref] [PubMed]
- Zheng Y, Wang Z, Wei S, et al. Epigenetic silencing of chemokine CCL2 represses macrophage infiltration to potentiate tumor development in small cell lung cancer. Cancer Lett 2021;499:148-63. [Crossref] [PubMed]
- Bottero V, Santiago JA, Potashkin JA. PTPRC Expression in Blood is Downregulated in Parkinson's and Progressive Supranuclear Palsy Disorders. J Parkinsons Dis 2018;8:529-37. [Crossref] [PubMed]
- Porcu M, Kleppe M, Gianfelici V, et al. Mutation of the receptor tyrosine phosphatase PTPRC (CD45) in T-cell acute lymphoblastic leukemia. Blood 2012;119:4476-9. [Crossref] [PubMed]
- Zhao X, Kallakury B, Chahine JJ, et al. Surgical Resection of SCLC: Prognostic Factors and the Tumor Microenvironment. J Thorac Oncol 2019;14:914-23. [Crossref] [PubMed]
- Hirsch FR, Scagliotti GV, Mulshine JL, et al. Lung cancer: current therapies and new targeted treatments. Lancet 2017;389:299-311. [Crossref] [PubMed]
- Bray F, Ferlay J, Soerjomataram I, et al. Global cancer statistics 2018: GLOBOCAN estimates of incidence and mortality worldwide for 36 cancers in 185 countries. CA Cancer J Clin 2018;68:394-424. [Crossref] [PubMed]
- Cheng TY, Cramb SM, Baade PD, et al. The International Epidemiology of Lung Cancer: Latest Trends, Disparities, and Tumor Characteristics. J Thorac Oncol 2016;11:1653-71. [Crossref] [PubMed]
- Sung H, Ferlay J, Siegel RL, et al. Global cancer statistics 2020: GLOBOCAN estimates of incidence and mortality worldwide for 36 cancers in 185 countries. CA Cancer J Clin 2021;71:209-49. [Crossref] [PubMed]
- Bader JE, Voss K, Rathmell JC. Targeting Metabolism to Improve the Tumor Microenvironment for Cancer Immunotherapy. Mol Cell 2020;78:1019-33. [Crossref] [PubMed]
- Giraldo NA, Sanchez-Salas R, Peske JD, et al. The clinical role of the TME in solid cancer. Br J Cancer 2019;120:45-53. [Crossref] [PubMed]
- Gao S, Yang D, Fang Y, et al. Engineering Nanoparticles for Targeted Remodeling of the Tumor Microenvironment to Improve Cancer Immunotherapy. Theranostics 2019;9:126-51. [Crossref] [PubMed]
- Tan X, Hu L, Shu Z, et al. Role of CCR2 in the Development of Streptozotocin-Treated Diabetic Cardiomyopathy. Diabetes 2019;68:2063-73. [Crossref] [PubMed]
- Niiya M, Niiya K, Kiguchi T, et al. Induction of TNF-alpha, uPA, IL-8 and MCP-1 by doxorubicin in human lung carcinoma cells. Cancer Chemother Pharmacol 2003;52:391-8. [Crossref] [PubMed]
- Soria G, Ben-Baruch A. The inflammatory chemokines CCL2 and CCL5 in breast cancer. Cancer Lett 2008;267:271-85. [Crossref] [PubMed]
- Tu MM, Abdel-Hafiz HA, Jones RT, et al. Inhibition of the CCL2 receptor, CCR2, enhances tumor response to immune checkpoint therapy. Commun Biol 2020;3:720. [Crossref] [PubMed]
- Courtney AH, Shvets AA, Lu W, et al. CD45 functions as a signaling gatekeeper in T cells. Sci Signal 2019;12:eaaw8151 [Crossref] [PubMed]
- Rheinlander A, Schraven B, Bommhardt U. CD45 in human physiology and clinical medicine. Immunol Lett 2018;196:22-32. [Crossref] [PubMed]
- Chen J, Qiao YD, Li X, et al. Intratumoral CD45(+)CD71(+) erythroid cells induce immune tolerance and predict tumor recurrence in hepatocellular carcinoma. Cancer Lett 2021;499:85-98. [Crossref] [PubMed]
- Lafont V, Sanchez F, Laprevotte E, et al. Plasticity of gammadelta T Cells: Impact on the Anti-Tumor Response. Front Immunol 2014;5:622. [Crossref] [PubMed]
- Murayama M, Tanaka Y, Yagi J, et al. Antitumor activity and some immunological properties of gammadelta T-cells from patients with gastrointestinal carcinomas. Anticancer Res 2008;28:2921-31. [PubMed]
- Kang N, Zhou J, Zhang T, et al. Adoptive immunotherapy of lung cancer with immobilized anti-TCRgammadelta antibody-expanded human gammadelta T-cells in peripheral blood. Cancer Biol Ther 2009;8:1540-9. [Crossref] [PubMed]
- Dieli F, Vermijlen D, Fulfaro F, et al. Targeting human {gamma}delta} T cells with zoledronate and interleukin-2 for immunotherapy of hormone-refractory prostate cancer. Cancer Res 2007;67:7450-7. [Crossref] [PubMed]
- Chien YH, Meyer C, Bonneville M. gammadelta T cells: first line of defense and beyond. Annu Rev Immunol 2014;32:121-55. [Crossref] [PubMed]
- Pauza CD, Liou ML, Lahusen T, et al. Gamma Delta T Cell Therapy for Cancer: It Is Good to be Local. Front Immunol 2018;9:1305. [Crossref] [PubMed]
- Galati D, Zanotta S, Bocchino M, et al. The subtle interplay between gamma delta T lymphocytes and dendritic cells: is there a role for a therapeutic cancer vaccine in the era of combinatorial strategies? Cancer Immunol Immunother 2021;70:1797-809. [Crossref] [PubMed]
(English Language Editor: J. Jones)