A multidimensional nomogram combining imaging features and clinical factors to predict the invasiveness and metastasis of combined hepatocellular cholangiocarcinoma
Introduction
Combined hepatocellular cholangiocarcinoma (CHCC-CCA) is a rare form of primary liver malignancy that exhibits features of both hepatocytic and cholangiocytic differentiation. CHCC-CCA accounts for approximately 2–5% of all primary liver cancer cases, and 5-year survival rate of CHCC-CCA is 17.7% (1,2). Owing to its low incidence rate, many problems regarding its diagnostic, therapeutic, and prognostic features have not yet been thoroughly understood or defined.
Although there have been substantial studies investigating both preoperative clinical or radiologic variables individually or in combination to predict the prognostic factors of HCC preoperatively, such as microvascular invasion (MVI) and lymph node metastasis (LNM) (3-5), the established models targeting HCC are not suitable for CHCC-CCA since the proportions of hepatocytic and cholangiocytic characteristics in the lesion cannot be determined prior to biopsy or surgery. Notably, imaging features can depict a variety of lesion properties. For example, the Liver Imaging Reporting and Data System (LI-RADS) incorporates imaging features into a standardized categorization system for the detection of hepatic lesions (6). The imaging features of CHCC-CCA show considerable variation and can be classified into LR-M or LR-4/LR-5, accounting for 32–63% and 7–72%, respectively, according to recent studies. This system was demonstrated to have both diagnostic and prognostic value, with reported studies suggesting that CHCC-CCA patients categorized as LR-M had a worse prognosis than those categorized as LR-4/LR-5 (7,8).
Moreover, whether the clinical or pathological characteristics of CHCC-CCA resemble HCC or ICC is still controversial (9). Some scholars have reported the presence of hepatitis virus infection and elevated α-fetoprotein (AFP), similar to HCC, while others have reported serum CA19-9 elevation and incomplete capsule formation, similar to ICC (10,11). It was also found that CHCC-CCA had the propensity to mimic HCC with respect to venous invasion and satellite formation, while it resembled ICC with respect to LNM and bile duct involvement (12). Owing to these sophisticated differences, it is crucial to develop a specific model to explore the aggressive biologic behavior associated with the prognosis of CHCC-CCA. To the best of our knowledge, no study has yet combined imaging features with clinical characteristics to predict related prognostic factors. Therefore, the aim of this study was to develop and validate a novel multidimensional nomogram that incorporates both for preoperative prediction of the crucial factors associated with prognosis, including MVI, satellite nodules and LNM, which could facilitate a more individualized treatment approach. The prognostic values of the prediction models were also explored.
We presented the following article in accordance with the TRIPOD reporting checklist (available at https://dx.doi.org/10.21037/atm-21-2500).
Methods
Patient selection and study design
The study flow chart for this study is shown in Figure 1A,1B. We retrospectively screened the clinical records of patients with postoperative pathologically confirmed CHCC-CCA between January 2015 and December 2019, which were retrieved from the information system of Zhongshan Hospital, Fudan University. All of the patients received curative surgery. CHCC-CCA was confirmed according to pathological diagnosis referring to the 2010 WHO classification system for CHCC-CCA. A total of 146 patients were classified as classical type, 18 patients were classified as typical subtype of stem cell features, five patients were classified as intermediate cell subtype of stem cell features and two patients were classified as cholangiolocellular subtype of stem cell features (13). The inclusion criteria were as follows: (I) patients with clear pathologically confirmed CHCC-CCA after liver resection; (II) Child-Pugh A or B liver function; (III) patients with detailed preoperative laboratory data; and (IV) MRI examinations performed within 30 days before hepatectomy of sufficient quality to satisfy the diagnostic criteria. Additionally, patients were excluded if they met the following exclusion criteria: (I) previous treatment history, such as transarterial chemoembolization (TACE) therapy and neoadjuvant chemotherapy, and (II) synchronous or previous malignancies. Subsequently, 171 CHCC-CCA patients were randomly divided into the training set (n=116) and validation set (n=55) using “Caret” R package (14). This retrospective study was conducted in accordance with the Declaration of Helsinki (as revised in 2013). The study was approved by the Clinical Research Ethics Committee of Zhongshan Hospital of Fudan University (B2019-139R) and individual consent for this retrospective analysis was waived.
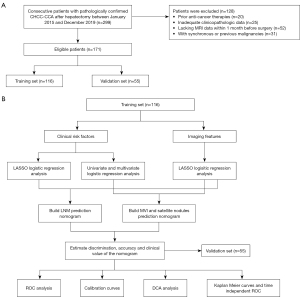
Liver MRI
All patients were scanned with a 1.5-T MR scanner (Magnetom Aera, Siemens Healthcare). Routine liver protocols consisted of transverse T2WI, T1WI in-phase and opposed-phase, and diffusion-weighted imaging (DWI, b value =0, 50, and 500 s/mm2). Dynamic imaging was performed with a T1-weighted fat-suppressed sequence. The arterial phase was acquired when the contrast agent (Gd-DTPA, Magnevist, Bayer HealthCare) reached the ascending aorta after the intravenous administration of Gd-DTPA at a dose of 0.1 mmol/kg at a rate of 2 mL/s. The portal venous phase and delayed phase were operated on at 60–70 and 160–180 s, respectively.
Preoperative clinicopathological and imaging variables
Baseline and clinicopathological variables were collected, including age, sex, etiology, laboratory tests and tumor characteristics (i.e., tumor size, tumor number and tumor location). Receiver operating characteristic (ROC) curves were utilized to determine the optimal cutoff value of the continuous variables for diagnosing MVI, and then, the continuous variables were converted to categorical variables for analysis. Additionally, the following qualitative imaging parameters were evaluated on a plain MR scan: (I) contour; (II) hemorrhage; (III) hepatic capsular retraction; and (IV) peritumoral bile duct dilation. Dynamic enhancement characteristics were as follows: (I) arterial enhancement pattern; (II) washout; (III) peritumoral enhancement pattern, which was defined as the enhancing portion surrounding the tumor border (15); (IV) false capsule; and (V) delayed central enhancement. Other imaging features were as follows: (VI) targetoid diffusion restriction; (VII) corona enhancement; (VIII) nodule in nodule architecture; (IX) mosaic architecture; and (X) diffusion restriction. Reviewers assigned a LI-RADS version 2018 (LI-RADS v2018) category for each hepatic observation in the study population, namely, LR-TIV (tumor in vein), LR-M (definitely or probably malignant, not HCC-specific) or LR 1–5 (1, definitely benign; 2, probably benign; 3, indeterminate probability of HCC; 4, probably HCC; or 5, definitely HCC) (16,17). Two experienced radiologists independently reviewed the MR images. Both radiologists were aware that all patients had a high risk of liver primary cancer but were blinded to the specific diagnosis of each tumor. A consensus was reached after discussion in case of any discrepancies. Only the largest pathologically confirmed CHCC-CCA was evaluated. All surgical specimens were examined histopathologically to identify the presence of MVI, satellite nodules and LNM. MVI was defined as the presence of a tumor in the portal vein, hepatic vein, or large capsular vessel of the surrounding hepatic tissue lined by endothelium that was visible only by microscopy. Satellite nodules were defined as detached micrometastatic nodules of HCC cells embedded in the hepatic parenchyma. The pathological LNM status of each patient was identified according to the histopathological reports.
Development of the combination nomograms
Univariate and multivariate logistic regression analyses were performed to evaluate the independent clinical risk factors for the presence of MVI and satellite nodules in the training set. Considering multicollinearity among the imaging variables, the least absolute shrinkage and selection operator (LASSO) logistic regression algorithm was used to select the most useful predictive imaging features. With regard to the small number of events relative to the number of clinical variables for predicting LNM, LASSO regression was used to select both the clinical and imaging predictors of LNM. Using the coefficients derived from the LASSO logistic regression models, we then constructed a formula to calculate the risk score for each patient. Formula = expression index1 × βindex1 +…+ expression index n × β index n (where β is the regression coefficient derived from LASSO regression) (18,19). Independent risk clinical factors and imaging risk scores from the LASSO regression model were integrated into the nomogram for predicting MVI and satellite nodules. In addition, both the clinical risk scores and imaging risk scores obtained from the LASSO regression models were used to construct a nomogram for predicting LNM. The clinical model and imaging model were established separately using the independent clinical risk factors and the imaging risk scores obtained from the LASSO regression.
Evaluation and validation of the combination nomograms and their prognostic value
The performance of the proposed nomograms was evaluated in terms of three aspects: discrimination ability, calibration, and clinical utility. ROC curves were plotted, followed by Harrell’s C-index (20) and calibration curves, with the goal of validating the discrimination ability and accuracy of the constructed nomograms in both the training and validation sets (21,22). Decision curve analysis (DCA) assigns a weight to the relative value of the benefit to harm, thereby modeling the consequences of clinical decisions (23). The decision curve was plotted at different threshold probabilities for the overall patients. Kaplan-Meier curves and log rank tests were conducted to determine the recurrence-free survival (RFS) and overall survival (OS) differences between different risk groups based on the median risk score, which was calculated by the formulas constructed by the predicted nomograms of the MVI and satellite nodules. Time-dependent ROC curves were displayed to evaluate the performances of the predicted nomograms for the MVI and satellite nodules.
Statistical analysis
All statistical analyses were performed using R software (version 3.5.2; https://www.r-project.org/). Student’s t test was used to compare continuous variables with a normal distribution, and the Mann-Whitney U test was used to analyze variables with an abnormal distribution. Categorical variables were compared using the chi-square test or Fisher’s exact test. The reported statistical significance levels were all two-sided, and P<0.05 was considered significant.
Results
Study flowchart and clinicopathological characteristics
In total, 171 consecutive patients with pathologically confirmed CHCC-CCA after hepatectomy between January 2015 and December 2019 who met the inclusion criteria were included. Then, 116 and 55 patients were assigned to the training set and validation set, respectively (Figure 1A,1B).
The preoperative clinicopathologic and MR imaging characteristics of the patients are listed in Table 1 and Table 2. Representative CHCC-CCA images assigned as different LI-RADS categories are shown in Figure 2 and Figure 3. There was no significant difference in terms of the clinicopathologic and imaging characteristics between the training and validation sets. Histopathologically identified MVI was observed in 48 (41.4%) and 27 (49.1%) patients in the two sets, respectively, and satellite nodules were observed in 21 (18.1%) and 9 (16.4%) patients in the two sets, whereas LNM was observed in 6 (5.2%) and 5 (9.1%) patients in the two sets, respectively. No statistically significant difference existed in the MVI rate (P=0.342), satellite nodule rate (P=0.780) or LNM rate (P=0.329) between the two sets, verifying their use as training and validation sets.
Table 1
Variable | Training set (N=116) (%) | Validation set (N=55) (%) | P value* |
---|---|---|---|
Age, year | 0.780 | ||
<65 | 95 (81.9) | 46 (83.6) | |
≥65 | 21 (18.1) | 9 (16.4) | |
Gender | 0.232 | ||
Male | 90 (77.6) | 38 (69.1) | |
Female | 26 (22.4) | 17 (30.9) | |
Histopathological type | 0.378 | ||
Classical type | 108 (93.1) | 38 (69.1) | |
Typical subtype of stem cell features | 14 (12.1) | 4 (7.3) | |
Intermediate cell subtype of stem cell features | 4 (3.4) | 1 (1.8) | |
Cholangiolocellular subtype of stem cell features | 2 (1.7) | 0 (0.0) | |
Hepatitis B virus | 0.389 | ||
Negative | 19 (16.4) | 12 (21.8) | |
Positive | 97 (83.6) | 43 (78.2) | |
CA19-9, ng/mL | 0.367 | ||
<37 | 87 (60.3) | 45 (83.6) | |
≥37 | 28 (39.7) | 10 (16.4) | |
AFP, ng/mL | 0.781 | ||
<400 | 90 (78.3) | 42 (76.4) | |
≥400 | 25 (21.7) | 13 (23.6) | |
CEA, ng/mL | 0.761 | ||
<5 | 94 (81.7) | 46 (83.6) | |
≥5 | 21 (18.3) | 9 (16.4) | |
Albumin, g/L | 0.229 | ||
<35 | 3 (2.6) | 0 (0.0) | |
≥35 | 113 (97.4) | 55 (100.0) | |
ALT, U/L | 0.931 | ||
<40 | 83 (71.6) | 39 (70.9) | |
≥40 | 33 (28.4) | 16 (29.1) | |
AST, U/L | 0.738 | ||
<40 | 88 (75.9) | 43 (78.2) | |
≥40 | 28 (24.1) | 12 (21.8) | |
GGT, U/L | 0.390 | ||
<50 | 63 (54.3) | 26 (47.3) | |
≥50 | 53 (45.7) | 29 (52.7) | |
Total bilirubin, μmol/L | 0.190 | ||
<17.1 | 89 (76.7) | 37 (67.3) | |
≥17.1 | 27 (23.3) | 18 (32.7) | |
Direct bilirubin, μmol/L | 0.219 | ||
<6.8 | 95 (82.6) | 41 (74.5) | |
≥6.8 | 20 (17.4) | 14 (25.5) | |
Size, cm | 0.914 | ||
<5 | 70 (60.9) | 33 (60.0) | |
≥5 | 45 (39.1) | 22 (40.0) | |
No. of tumors | 0.479 | ||
Solitary | 94 (81.0) | 42 (76.4) | |
Multiple | 22 (19.0) | 13 (23.6) | |
Cirrhosis | 0.649 | ||
No | 29 (25.0) | 12 (21.8) | |
Yes | 87 (75.0) | 43 (78.2) | |
NLR | 0.205 | ||
<2.19 | 75 (64.7) | 30 (54.5) | |
≥2.19 | 41 (35.3) | 25 (45.5) | |
PLR | 0.173 | ||
<76.74 | 37 (31.9) | 12 (21.8) | |
≥76.74 | 79 (68.1) | 43 (78.2) | |
LMR | 0.244 | ||
<3.03 | 32 (27.6) | 20 (36.4) | |
≥3.03 | 84 (72.4) | 35 (63.6) | |
AAPR | 0.530 | ||
<0.53 | 51 (44.0) | 27 (49.1) | |
≥0.53 | 65 (56.0) | 28 (50.9) | |
APRI | 0.204 | ||
<0.16 | 43 (37.1) | 26 (47.3) | |
≥0.16 | 73 (62.9) | 29 (52.7) | |
ANRI | 0.630 | ||
<14.73 | 89 (76.7) | 44 (80.0) | |
≥14.73 | 27 (23.3) | 11 (20.0) | |
Satellite nodules | 0.780 | ||
Absent | 95 (81.9) | 46 (83.6) | |
Present | 21 (18.1) | 9 (16.4) | |
MVI | 0.342 | ||
Absent | 68 (58.6) | 28 (50.9) | |
Present | 48 (41.4) | 27 (49.1) | |
LNM | 0.329 | ||
Absent | 110 (94.8) | 50 (90.9) | |
Present | 6 (5.2) | 5 (9.1) | |
Capsule | 0.838 | ||
None | 69 (59.5) | 35 (63.6) | |
Complete | 23 (19.8) | 9 (16.4) | |
Incomplete | 24 (20.7) | 11 (20.0) | |
Tumor location | 0.492 | ||
Right liver lobe | 29 (25.0) | 11 (20.0) | |
Left liver lobe | 84 (72.4) | 44 (80.0) | |
Other location | 3 (2.6) | 0 (0.0) |
Data are shown as number of patients, with the percentage in parentheses. *, P value was calculated by χ2 test or Fisher’s exact test. CA 19-9, carbohydrate antigen 19-9; AFP, α‐fetoprotein; CEA, carcinoembryonic antigen; ALT, alanine aminotransferase; AST, aspartate aminotransferase; GGT, γ-glutamyltranspeptidase; NLR, neutrophil to lymphocyte ratio; PLR, platelet to lymphocyte ratio; LMR, lymphocyte to monocyte ratio; AAPR, albumin-to-alkaline phosphatase ratio; APRI, aspartate aminotransferase to platelet ratio index; ANRI, aspartate aminotransferase to neutrophil ratio index; MVI, microvascular invasion; LNM, lymph node metastasis.
Table 2
Variable | Training set (N=116) (%) | Validation set (N=55) (%) | P value* |
---|---|---|---|
Shape | 0.888 | ||
Globular | 44 (37.9) | 23 (41.8) | |
Lobulated | 56 (48.3) | 25 (45.5) | |
Irregular | 16 (13.8) | 7 (12.7) | |
Contour | 0.888 | ||
Non-smooth | 73 (62.9) | 34 (61.8) | |
Smooth | 43 (37.1) | 21 (38.2) | |
Hemorrhage | 0.766 | ||
Absent | 95 (81.9) | 44 (80.0) | |
Present | 21 (18.1) | 11 (20.0) | |
Targetoid restriction | 0.413 | ||
Absent | 89 (76.7) | 39 (70.9) | |
Present | 27 (23.3) | 16 (29.1) | |
Restricted diffusion | 0.764 | ||
Absent | 10 (8.6) | 4 (7.3) | |
Present | 106 (91.4) | 51 (92.7) | |
Arterial enhancement pattern | 0.099 | ||
None | 0 (0.0) | 2 (3.6) | |
Global | 67 (57.8) | 28 (50.9) | |
Peripheral | 49 (42.2) | 25 (45.5) | |
Peritumoral enhancement pattern | 0.413 | ||
None | 71 (61.2) | 28 (50.9) | |
Wedge | 22 (19.0) | 12 (21.8) | |
Irregular | 23 (19.8) | 15 (27.3) | |
Washout | 0.711 | ||
Absent | 37 (31.9) | 16 (29.1) | |
Present | 79 (68.1) | 39 (70.9) | |
Delayed central enhancement | 0.507 | ||
Absent | 83 (71.6) | 42 (76.4) | |
Present | 33 (28.4) | 13 (23.6) | |
Corona enhancement | 0.466 | ||
Absent | 98 (84.5) | 44 (80.0) | |
Present | 18 (15.5) | 11 (20.0) | |
False capsule | 0.191 | ||
None | 56 (48.3) | 19 (34.5) | |
Complete | 21 (18.1) | 15 (27.3) | |
Incomplete | 39 (33.6) | 21 (38.2) | |
Bile duct dilation | 0.165 | ||
Absent | 70 (60.3) | 27 (49.1) | |
Present | 46 (39.7) | 28 (50.9) | |
Surface retraction | 0.755 | ||
Absent | 89 (76.7) | 41 (74.5) | |
Present | 27 (23.3) | 14 (25.5) | |
Nodule in nodule architecture | 0.633 | ||
Absent | 105 (90.5) | 51 (92.7) | |
Present | 11 (9.5) | 4 (7.3) | |
Mosaic architecture | 0.244 | ||
Absent | 70 (60.3) | 28 (50.9) | |
Present | 46 (39.7) | 27 (49.1) | |
LI-RADS categorization | 0.526 | ||
LR-3 | 8 (6.9) | 3 (5.5) | |
LR-M | 32 (27.6) | 21 (38.2) | |
LR-4/LR-5 | 53 (45.7) | 20 (36.4) | |
LR-TIV | 23 (19.8) | 11 (20.0) |
*, P value was calculated by χ2 test or Fisher’s exact test. MR, magnetic resonance; LI-RADS, Liver Imaging Reporting and Data System.
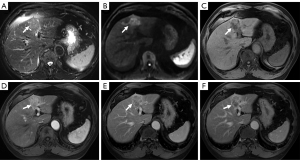
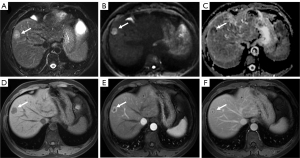
Independent prognostic factors of MVI, satellite nodules and LNM and nomogram construction
Univariate analysis revealed that neutrophil to lymphocyte ratio (NLR) (P=0.049), platelet to lymphocyte ratio (PLR) (P=0.001), lymphocyte to monocyte ratio (LMR) (P=0.047), albumin-to-alkaline phosphatase ratio (AAPR) (P=0.008), tumor size (P=0.006) and AFP level (P=0.01) were associated with the presence of MVI, and tumor size (P<0.001) and carcinoembryonic antigen (CEA) (P=0.045) were associated with the presence of satellite nodules. In the multivariate analysis, PLR (OR, 4.8; 95% CI: 1.1–19.7; P=0.031), AAPR (OR, 4.8; 95% CI: 1.3–17.3; P=0.017) were identified as independent predictors of MVI (Table 3). Additionally, tumor size (OR, 4.3; 95% CI: 1.1–16.1; P=0.003) and CEA (OR, 12.3; 95% CI: 1.4–107.1; P=0.023) were significantly associated with the presence of satellite nodules (Table 4). We utilized the LASSO logistic regression algorithm to select candidate imaging features that were reliably associated with the presence of MVI (Figure 4A,4B), satellite nodules (Figure 4C,4D) and LNM (Figure S1A,S1B) in the training set. The formulas for the imaging scores in the LASSO models are listed in Table S1. Similarly, the LASSO logistic regression algorithm was also used to detect the clinical features associated with LNM in the training set (Figure S1C,S1D), and the formula for clinical scores in the LASSO model is listed in Table S1.
Table 3
Risk factor | Univariate analysis | Multivariate analysis | |||||
---|---|---|---|---|---|---|---|
OR | 95% CI | P value | OR | 95% CI | P value | ||
Age, year | |||||||
<65 | 1.0 | ||||||
≥65 | 1.1 | 0.4–2.8 | 0.879 | ||||
Gender | |||||||
Male | 1.0 | ||||||
Female | 1.3 | 0.7–2.6 | 0.448 | ||||
Hepatitis B virus | |||||||
Negative | 1.0 | ||||||
Positive | 1.0 | 0.4–2.6 | 0.944 | ||||
Tumor location | |||||||
Right liver lobe | 1.0 | ||||||
Left liver lobe | 1.5 | 0.6–3.6 | 0.369 | ||||
Other location | 1.0 | 0.1–11.8 | 0.968 | ||||
No. of tumors | |||||||
Solitary | 1.0 | ||||||
Multiple | 1.1 | 0.5–2.3 | 0.804 | ||||
Size, cm | |||||||
<5 | 1.0 | 1.0 | |||||
≥5 | 3.0 | 1.4–6.5 | 0.006 | 1.7 | 0.5–5.1 | 0.370 | |
AFP, ng/mL | |||||||
<400 | 1.0 | 1.0 | |||||
≥400 | 3.4 | 1.3–8.5 | 0.010 | 2.6 | 0.8–8.9 | 0.118 | |
CEA, ng/mL | |||||||
<5 | 1.0 | ||||||
≥5 | 1.4 | 0.5–3.6 | 0.488 | ||||
CA19-9, ng/mL | |||||||
<37 | 1.0 | ||||||
≥37 | 0.6 | 0.2–1.5 | 0.283 | ||||
ALT, U/L | |||||||
<40 | 1.0 | ||||||
≥40 | 0.7 | 0.3–1.7 | 0.490 | ||||
AST, U/L | |||||||
<40 | 1.0 | ||||||
≥40 | 1.3 | 0.6–3.1 | 0.534 | ||||
GGT, U/L | |||||||
<50 | 1.0 | ||||||
≥50 | 1.2 | 0.6–2.4 | 0.686 | ||||
Total bilirubin, μmol/L | |||||||
<17.1 | 1.0 | ||||||
≥17.1 | 1.0 | 0.4–2.3 | 0.939 | ||||
Direct bilirubin, μmol/L | |||||||
<6.8 | 1.0 | ||||||
≥6.8 | 0.6 | 0.2–1.6 | 0.281 | ||||
Albumin, g/L | |||||||
<35 | 1.0 | ||||||
≥35 | 1.4 | 0.1–16.2 | 0.775 | ||||
NLR | |||||||
<2.19 | 1.0 | 1.0 | |||||
≥2.19 | 2.2 | 1.0–4.7 | 0.049 | 0.9 | 0.2–3.6 | 0.901 | |
PLR | |||||||
<76.74 | 1.0 | 1.0 | |||||
≥76.74 | 4.6 | 1.8–11.8 | 0.001 | 4.8 | 1.1–19.7 | 0.031 | |
LMR | |||||||
<3.03 | 1.0 | 1.0 | |||||
≥3.03 | 0.4 | 0.2–1.0 | 0.047 | 0.3 | 0.1–1.3 | 0.098 | |
AAPR | |||||||
<0.53 | 1.0 | 1.0 | |||||
≥0.53 | 2.9 | 1.3–6.4 | 0.008 | 4.8 | 1.3–17.3 | 0.017 | |
APRI | |||||||
<0.16 | 1.0 | ||||||
≥0.16 | 0.6 | 0.3–1.3 | 0.212 | ||||
ANRI | |||||||
<14.73 | 1.0 | ||||||
≥14.73 | 1.4 | 0.6–3.4 | 0.416 |
MVI, microvascular invasion; OR, odds ratio; CI, confidence interval; AFP, α‐fetoprotein; CEA, carcinoembryonic antigen; CA 19-9, carbohydrate antigen 19-9; ALT, alanine aminotransferase; AST, aspartate aminotransferase; GGT, γ-glutamyltranspeptidase; NLR, neutrophil to lymphocyte ratio; PLR, platelet to lymphocyte ratio; LMR, lymphocyte to monocyte ratio; AAPR, albumin-to-alkaline phosphatase ratio; APRI, aspartate aminotransferase to platelet ratio index; ANRI, aspartate aminotransferase to neutrophil ratio index.
Table 4
Risk factor | Univariate analysis | Multivariate analysis | |||||
---|---|---|---|---|---|---|---|
OR | 95% CI | P value | OR | 95% CI | P value | ||
Age, year | |||||||
<65 | 1.0 | ||||||
≥65 | 0.7 | 0.2–2.7 | 0.617 | ||||
Gender | |||||||
Male | 1.0 | ||||||
Female | 1.1 | 0.5–2.7 | 0.833 | ||||
Hepatitis B virus | |||||||
Negative | 1.0 | ||||||
Positive | 0.8 | 0.2–2.7 | 0.715 | ||||
Tumor location | |||||||
Right liver lobe | 1.0 | ||||||
Left liver lobe | 1.7 | 0.6–4.8 | 0.315 | ||||
Other location | 1.0 | 0.1–12.1 | 0.992 | ||||
No. of tumors | |||||||
Solitary | 1.0 | ||||||
Multiple | 1.0 | 0.4–2.6 | 0.944 | ||||
Size, cm | |||||||
<5 | 1.0 | 1.0 | |||||
≥5 | 5.8 | 2.4–14.0 | <0.001 | 4.3 | 1.1–16.1 | 0.003 | |
AFP, ng/mL | |||||||
<400 | 1.0 | ||||||
≥400 | 2.0 | 0.8–4.7 | 0.116 | ||||
CEA, ng/mL | |||||||
<5 | 1.0 | 1.0 | |||||
≥5 | 2.9 | 1.0–8.3 | 0.045 | 12.3 | 1.4–107.1 | 0.023 | |
CA19-9, ng/mL | |||||||
<37 | 1.0 | ||||||
≥37 | 1.3 | 0.5–3.3 | 0.533 | ||||
ALT, U/L | |||||||
<40 | 1.0 | ||||||
≥40 | 0.6 | 0.2–1.5 | 0.253 | ||||
AST, U/L | |||||||
<40 | 1.0 | ||||||
≥40 | 1.0 | 0.4–2.5 | 0.993 | ||||
GGT, U/L | |||||||
<50 | 1.0 | ||||||
≥50 | 1.1 | 0.5–2.4 | 0.805 | ||||
Total bilirubin, μmol/L | |||||||
<17.1 | 1.0 | ||||||
≥17.1 | 0.5 | 0.2–1.4 | 0.193 | ||||
Direct bilirubin, μmol/L | |||||||
<6.8 | 1.0 | ||||||
≥6.8 | 0.6 | 0.2–1.7 | 0.320 | ||||
Albumin, g/L | |||||||
<35 | 1.0 | ||||||
≥35 | 1.8 | 0.2–15.2 | 0.991 | ||||
NLR | |||||||
<2.19 | 1.0 | ||||||
≥2.19 | 1.3 | 0.6–2.8 | 0.558 | ||||
PLR | |||||||
<76.74 | 1.0 | ||||||
≥76.74 | 3.0 | 1.0–9.3 | 0.051 | ||||
LMR | |||||||
<3.03 | 1.0 | ||||||
≥3.03 | 0.8 | 0.4–2.0 | 0.702 | ||||
AAPR | |||||||
<0.53 | 1.0 | ||||||
≥0.53 | 1.1 | 0.5–2.5 | 0.782 | ||||
APRI | |||||||
<0.16 | 1.0 | ||||||
≥0.16 | 1.0 | 0.5–2.3 | 0.966 | ||||
ANRI | |||||||
<14.73 | 1.0 | ||||||
≥14.73 | 0.9 | 0.3–2.3 | 0.747 |
OR, odds ratio; CI, confidence interval; AFP, α‐fetoprotein; CEA, carcinoembryonic antigen; CA 19-9, carbohydrate antigen 19-9; ALT, alanine aminotransferase; AST, aspartate aminotransferase; GGT, γ-glutamyltranspeptidase; NLR, neutrophil to lymphocyte ratio; PLR, platelet to lymphocyte ratio; LMR, lymphocyte to monocyte ratio; AAPR, albumin-to-alkaline phosphatase ratio; APRI, aspartate aminotransferase to platelet ratio index; ANRI, aspartate aminotransferase to neutrophil ratio index.
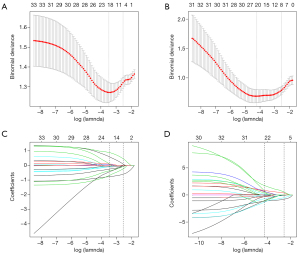
Performance and validation of the nomograms
According to the results of the multivariate logistic regression and LASSO regression, clinical risk factors and imaging features for the prediction of each outcome were identified, and then integrated nomograms were constructed by combining the clinical risk factors and imaging models for MVI (Figure 5A), satellite nodules (Figure 5B) and LNM (Figure S2). The C-index for the nomogram predicting MVI was 0.857 (95% CI: 0.787–0.927) in the training set and 0.795 (95% CI: 0.674–0.916) in the validation set (Figure 6A); the C-index for the prediction of satellite nodules was 0.919 (95% CI: 0.858–0.980) in the training set and 0.883 (95% CI: 0.740–0.906) in the validation set (Figure 6B); and the C-index for the nomogram predicting LNM was 0.872 (95% CI: 0.711–0.900) in the training set and 0.666 (95% CI: 0.605–0.751) in the validation set (Figure S3). We also did subgroup analysis for validating the constructed model for classical type of CHCC-CCA, the C-index for the prediction of MVI, satellite nodules and LNM was 0.788, 0.885 and 0.952 respectively (Figure S4A-S4C). Calibration plots of the training and validation sets all graphically showed good agreement between the actual risk confirmed by pathological examination and the predicted risk for MVI (Figure 7A,7B), satellite nodules (Figure 7C,7D) and LNM (Figure S5) in both the training and validation sets. The decision curves of the combined nomograms, clinical model and imaging model are shown in Figure 8A for MVI, Figure 8B for satellite nodules and Figure S6 for LNM, indicating that our nomograms offered more benefits than the treat-all or treat-none schemes.
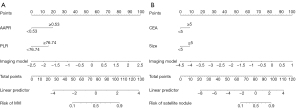
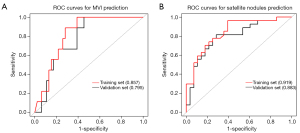
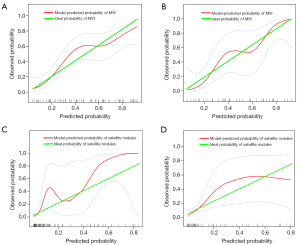
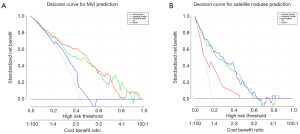
We observed C-indexes corresponding to the area of the ROC curves among different models for predicting MVI, satellite nodules and LNM and found that the combined nomograms were superior to those of both the clinical and imaging models for the prediction of all outcomes in the training set and validation set. According to the ROC analysis, our constructed nomograms had improved predictive value for MVI, satellite nodules and LNM and a higher threshold probability compared to the other models. The specific performances of the combined nomogram, clinical model and imaging model are summarized in Table 5.
Table 5
Outcome | Group | Model | C-index (95% CI) | Sensitivity | Specificity | PPV | NPV |
---|---|---|---|---|---|---|---|
MVI | Training set | Nomogram | 0.857 (0.787–0.927) | 0.854 | 0.765 | 0.719 | 0.881 |
Clinical model | 0.696 (0.607–0.784) | 0.854 | 0.441 | 0.519 | 0.811 | ||
Imaging model | 0.802 (0.720–0.883) | 0.708 | 0.824 | 0.739 | 0.800 | ||
Validation set | Nomogram | 0.795 (0.674–0.916) | 0.815 | 0.714 | 0.733 | 0.800 | |
Clinical model | 0.522 (0.373–0.672) | 0.667 | 0.500 | 0.563 | 0.609 | ||
Imaging model | 0.839 (0.734–0.945) | 0.963 | 0.607 | 0.703 | 0.944 | ||
Satellite nodules | Training set | Nomogram | 0.919 (0.858–0.980) | 0.905 | 0.828 | 0.543 | 0.975 |
Clinical model | 0.751 (0.627–0.874) | 0.762 | 0.688 | 0.356 | 0.928 | ||
Imaging model | 0.914 (0.848–0.980) | 0.810 | 0.903 | 0.654 | 0.955 | ||
Validation set | Nomogram | 0.883 (0.740–0.906) | 1.000 | 0.544 | 0.300 | 1.000 | |
Clinical model | 0.783 (0.641–0.891) | 0.889 | 0.652 | 0.333 | 0.968 | ||
Imaging model | 0.818 (0.702–0.933) | 1.000 | 0.609 | 0.333 | 1.000 | ||
LNM | Training set | Nomogram | 0.872 (0.711–0.900) | 1.000 | 0.842 | 0.288 | 1.000 |
Clinical model | 0.630 (0.628–0.678) | 1.000 | 0.805 | 0.253 | 1.000 | ||
Imaging model | 0.683 (0.625–0.750) | 1.000 | 0.719 | 0.196 | 1.000 | ||
Validation set | Nomogram | 0.666 (0.605–0.751) | 0.666 | 0.881 | 0.235 | 0.980 | |
Clinical model | 0.654 (0.632–0.664) | 0.657 | 0.877 | 0.243 | 0.887 | ||
Imaging model | 0.617 (0.521–0.724) | 0.667 | 0.809 | 0.160 | 0.978 |
CI, confidence interval; PPV, positive predictive value; NPV, negative predictive value; MVI, microvascular invasion; LNM, lymph node metastasis.
Prognostic value of the predicted MVI risk status and satellite nodule status
To evaluate the role of the constructed nomograms in the prediction of RFS and OS, we divided patients into high-risk and low-risk groups based on the median risk score calculated by the nomograms for the prediction of MVI and satellite nodules. The median risk scores for MVI and satellite nodules were −0.546 and −2.539, respectively. Survival analysis showed that patients with a high risk of MVI (P=0.003) (Figure 9A) and satellite nodules (P=0.042) (Figure 9B) had significantly worse RFS than their counterparts. Although there was no statistical difference observed in OS of different risk groups of MVI (P=0.057) (Figure 9C), patients with a high risk of having satellite nodules presented with worse OS than patients from the low-risk group (P=0.006) (Figure 9D). Furthermore, the performances of the predicted risk status of presenting MVI and satellite nodules for the prediction of OS at 1, 2 and 3 years were 0.755, 0.659 and 0.587, and 0.769, 0.722 and 0.681, respectively (Figure 9E,9F).
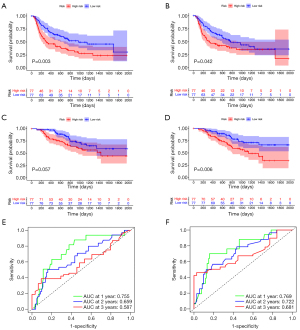
Discussion
CHCC-CCA has several overlapping imaging features with ICC and HCC because of hepatocellular or cholangiocellular differentiation in variable proportion, which could lead to misdiagnosis before surgery. As a result, the misdiagnosis could not guarantee the optimal treatment choice for patients with CHCC-CCA. Recently, abundant researches have proposed diagnostic algorithm of CHCC-CCA. Gigante et al. (24) developed a two-step strategy combining imaging and tumor biopsy, achieving satisfactory diagnostic performance. Moreover, according to the LI-RADS (25), tumor with imaging features of malignancy but are not specific for HCC are categorized as LR-M, and of definitive features for HCC are categorized as LR-4/5, which means that if CHCC-CCA could be categorized as LR-M, it can be correctly diagnosed with the help of biopsy before surgery. It was reported that CHCC-CCAs were categorized as LR-M and LR-5/4 in 61.4% and 37.1% respectively (8). Using LI-RADS, a substantial proportion of CHCC-CCAs can be categorized as LR-M. In this scenario, given that advances in the preoperative diagnosis of CHCC-CCA could be conveniently applied in the clinical cases, our nomograms for predicting MVI, satellite nodules and LNM preoperatively could further facilitate treatment strategy optimization for a subset of patients who can have definitive diagnosis using strategy of combined imaging and biopsy, or patients who are categorized as LR-M.
The intrinsic properties of CHCC-CCA differ from those of HCC and ICC. Moreover, its OS rate has been reported to be even lower than that of ICC (26-28). As a result, it is critical to separately map prediction models for strong prognostic parameters, including MVI, satellite nodules and LNM. Our results demonstrated that the combined nomogram models constructed for MVI, satellite nodules and LNM had satisfactory prognostication performance in both the training and validation sets and showed generally higher predictive value than the clinical and imaging models.
MVI is defined as the presence of tumor cells within a vascular lumen that is visible only by microscopy (29,30). In contrast with MVI, satellite nodules are separate micrometastatic lesions considered to be direct malignant cell invasion of the hepatic parenchyma (31). Activation of epithelial-mesenchymal transition (EMT) transcriptional programs has been proven to be an essential pathogenic step leading to the development of MVI and satellitosis (32). Since both are associated with aggressive tumor behavior, they probably have overlapping prognostic outcomes to some extent, which can be inferred from the multiple studies in which MVI was reported to be an independent risk factor for recurrence, similar to satellite nodules (33,34). Although there has been no precise classification system for MVI burden, several studies have identified the necessity of proposing novel stage criteria, as they found that the severity of MVI was highly associated with different prognoses (35,36). Therefore, adverse factors for predicting different degrees of MVI are of great importance and should be analyzed further. LNM is another important predictor of a poor prognosis in CHCC-CCA patients. Hilar LNM is rarely observed in HCC patients but is often observed in patients with CHCC-CCA and ICC (10). An accurate understanding of lymph node status enables surgeons to better tailor treatment. Although our established combined nomogram showed high C-indexes for LNM prediction in both the training and validation sets, these findings should be further validated in larger cohorts.
The proposed nomograms integrated clinical and imaging risk factors and were demonstrated to be more reliable for the prediction of MVI, satellite nodules and LNM than the nomograms constructed from clinical factors or imaging factors alone. A recent study integrated clinical risk factors and laboratory blood indicators to investigate the diagnostic accuracy of MVI and satellite nodules for CHCC-CCA, yielding C-indexes of 0.826 and 0.771, respectively (37), which were lower than those of the nomograms we built. In another study, imaging features of intratumoral fat deposition and irregular peritumoral enhancement combined with the AFP level for the prediction of MVI yielded a specificity of 98.2% but a sensitivity of 12.5% (38).
The inflammatory response plays a decisive role in cancer initiation, malignant transformation and MVI by promoting epigenetic changes, cell proliferation, angiogenesis, and cell invasion (39). In our study, the level of PLR was significant serum inflammatory markers of MVI in CHCC-CCA, which was consistent with prior studies. A meta-analysis showed that elevated PLR suggested poor OS in HCC, with a pooled HR of 1.63 (40). Albumin is also a useful marker for determining the inflammatory response, and alkaline phosphatase hydrolase enzymes are primarily found in the liver, bile duct and bone. In our nomogram, AAPR was an independent predictor of MVI, concordant with the findings reported in the study by Zhang et al. (41), who identified that AAPR was a strong indicator of OS in CHCC-CCA, with an optimal threshold value of 0.43. Similarly, utilizing these inflammatory indexes in our nomogram could improve its predictive power. Interestingly, PLR and AAPR were not independent risk factors for the prediction of satellite nodules in our study, probably because of the smaller number of events in satellite nodules compared with that in MVI, which led to weakened statistical power.
Imaging can help to visualize the entire profile of the relative proportions of tumor features. Several studies have reported that lesions harboring a hypervascular pattern had a larger proportion of HCC features and less fibrotic stroma (42,43), while lesions with a targeted appearance, as defined by LI-RADS, were more likely to have a larger proportion of CC features and fibrotic stroma (38). The intrinsic heterogeneity of CHCC-CCA histology contributes to the discordance in survival outcomes. This idea was based on the evidence reported by Park et al. (42), demonstrating that CHCC-CCA patients with hypervascular features had better survival outcomes than CHCC-CCA patients with nonhypervascular features; simultaneously, patients categorized as having LR-M or LR-4/5 were found to have significant differences in survival outcomes (7,8). The multiple imaging biomarkers selected by LASSO logistic regression in our study yielded satisfactory prediction performance. However, interobserver variability hampers the quality of imaging feature evaluations, and radiomics has begun to emerge as a useful tool to predict the status of MVI and LNM in HCC (44,45); however, such a method has not yet been applied to CHCC-CCA. In the future, the establishment of the genomic, transcriptomic, proteomic and radiomic landscapes of CHCC-CCA is desirable for a better understanding of the progression of CHCC-CCA and adverse prognostic factors.
Unfortunately, there is no globally accepted standard first-line therapy for CHCC-CCA, and comparative data on different therapeutic tools are sparse. Thus, the nomograms we constructed for predicting the most predominant prognostic factors might serve as an effective tool to guide appropriate clinical treatment selection. Evidence from a multicenter analysis showed that compared with resection, the outcomes of patients with CHCC-CCA who underwent transplantation were superior (46); however, they exhibited inferior 5-year survival outcomes compared to those with HCC who underwent transplantation (47). Moreover, patients with a high MVI status were reported to be unsuitable for transplantation since unsatisfactory OS outcomes were observed compared with patients without MVI (48). Therefore, preoperative estimated models could facilitate clinicians in recruiting candidates who could obtain the most benefit from transplantation. For patients who present with MVI or satellitosis, a larger resection margin could potentially clear intrahepatic metastases. In this scenario, RFA, as an alternative ablative therapy of hepatic resection, showed less favorable surgical outcomes due to inferior radical tumor clearance. Neoadjuvant therapy for ICC with LNM is expected to improve survival (49), providing supportive evidence that patients could receive neoadjuvant therapy for CHCC-CCA. There is also accumulating evidence suggesting that aggressive surgical treatment, including lymph node dissection, can prolong the survival of patients with CHCC-CCA (28). In this scenario, successful preoperative prediction of LNM risk could result in improved postoperative survival outcomes.
Our study had some limitations that should be mentioned. First, since our validation set was derived from a single institution, our available sample size was relatively small. Hence, analysis based on a larger cohort and external validation in other centers is necessary. Second, a prospective study is required to further confirm the reliability of our nomograms. Third, we did not categorize CHCC-CCA into different subtypes according to the latest WHO classification system; more specific prognostication among various types should be conducted to further elucidate the biological behavior of CHCC-CCA. Finally, since qualitative imaging features suffer from some limitations, including interobserver variability and lack of external validation, studies incorporating radiomics or genomics into predictive nomograms should be conducted in the future.
Conclusions
Nomograms incorporating clinical factors and imaging features achieved satisfactory performance in individualized prediction of preoperative status of MVI, the presence of satellite nodules and LNM, allowing treatment strategy optimization in patients with CHCC-CCA. Furthermore, these investigations need to be validated in more prospective studies.
Acknowledgments
Funding: This work was supported by grants from the National Natural Science Foundation of China (grant number 91859107), the Shanghai Science and Technology Committee (grant number 19411965500), the Shanghai Municipal Key Clinical Specialty (shslczdzk03202), Clinical Research Plan of SHDC (SHDC2020CR1029B).
Footnote
Reporting Checklist: The authors have completed the TRIPOD reporting checklist. Available at https://dx.doi.org/10.21037/atm-21-2500
Data Sharing Statement: Available at https://dx.doi.org/10.21037/atm-21-2500
Conflicts of Interest: All authors have completed the ICMJE uniform disclosure form (available at https://dx.doi.org/10.21037/atm-21-2500). The authors have no conflicts of interest to declare.
Ethical Statement: The authors are accountable for all aspects of the work in ensuring that questions related to the accuracy or integrity of any part the work are appropriately investigated and resolved. This retrospective study was conducted in accordance with the Declaration of Helsinki (as revised in 2013). The study was approved by the Clinical Research Ethics Committee of Zhongshan hospital of Fudan University (B2019-139R) and individual consent for this retrospective analysis was waived.
Open Access Statement: This is an Open Access article distributed in accordance with the Creative Commons Attribution-NonCommercial-NoDerivs 4.0 International License (CC BY-NC-ND 4.0), which permits the non-commercial replication and distribution of the article with the strict proviso that no changes or edits are made and the original work is properly cited (including links to both the formal publication through the relevant DOI and the license). See: https://creativecommons.org/licenses/by-nc-nd/4.0/.
References
- Ramai D, Ofosu A, Lai JK, et al. Combined Hepatocellular Cholangiocarcinoma: A Population-Based Retrospective Study. Am J Gastroenterol 2019;114:1496-501. [Crossref] [PubMed]
- Seehawer M, D'Artista L, Zender L. The Worst from Both Worlds: cHCC-ICC. Cancer Cell 2019;35:823-4. [Crossref] [PubMed]
- Ma X, Wei J, Gu D, et al. Preoperative radiomics nomogram for microvascular invasion prediction in hepatocellular carcinoma using contrast-enhanced CT. Eur Radiol 2019;29:3595-605. [Crossref] [PubMed]
- Zhang X, Ruan S, Xiao W, et al. Contrast-enhanced CT radiomics for preoperative evaluation of microvascular invasion in hepatocellular carcinoma: A two-center study. Clin Transl Med 2020;10:e111 [Crossref] [PubMed]
- Yan Y, Zhou Q, Zhang M, et al. Integrated Nomograms for Preoperative Prediction of Microvascular Invasion and Lymph Node Metastasis Risk in Hepatocellular Carcinoma Patients. Ann Surg Oncol 2020;27:1361-71. [Crossref] [PubMed]
- Mitchell DG, Bruix J, Sherman M, et al. LI-RADS (Liver Imaging Reporting and Data System): summary, discussion, and consensus of the LI-RADS Management Working Group and future directions. Hepatology 2015;61:1056-65. [Crossref] [PubMed]
- Choi SH, Lee SS, Park SH, et al. LI-RADS Classification and Prognosis of Primary Liver Cancers at Gadoxetic Acid-enhanced MRI. Radiology 2019;290:388-97. [Crossref] [PubMed]
- Jeon SK, Joo I, Lee DH, et al. Combined hepatocellular cholangiocarcinoma: LI-RADS v2017 categorisation for differential diagnosis and prognostication on gadoxetic acid-enhanced MR imaging. Eur Radiol 2019;29:373-82. [Crossref] [PubMed]
- Brunt E, Aishima S, Clavien PA, et al. cHCC-CCA: Consensus terminology for primary liver carcinomas with both hepatocytic and cholangiocytic differentation. Hepatology 2018;68:113-26. [Crossref] [PubMed]
- Yin X, Zhang BH, Qiu SJ, et al. Combined hepatocellular carcinoma and cholangiocarcinoma: clinical features, treatment modalities, and prognosis. Ann Surg Oncol 2012;19:2869-76. [Crossref] [PubMed]
- Kim KH, Lee SG, Park EH, et al. Surgical treatments and prognoses of patients with combined hepatocellular carcinoma and cholangiocarcinoma. Ann Surg Oncol 2009;16:623-9. [Crossref] [PubMed]
- Lu XY, Xi T, Lau WY, et al. Hepatocellular carcinoma expressing cholangiocyte phenotype is a novel subtype with highly aggressive behavior. Ann Surg Oncol 2011;18:2210-7. [Crossref] [PubMed]
- Kim TH, Kim H, Joo I, et al. Combined Hepatocellular-Cholangiocarcinoma: Changes in the 2019 World Health Organization Histological Classification System and Potential Impact on Imaging-Based Diagnosis. Korean J Radiol 2020;21:1115-25. [Crossref] [PubMed]
- Zhou ZR, Wang WW, Li Y, et al. In-depth mining of clinical data: the construction of clinical prediction model with R. Ann Transl Med 2019;7:796. [Crossref] [PubMed]
- Kim KA, Kim MJ, Jeon HM, et al. Prediction of microvascular invasion of hepatocellular carcinoma: usefulness of peritumoral hypointensity seen on gadoxetate disodium-enhanced hepatobiliary phase images. J Magn Reson Imaging 2012;35:629-34. [Crossref] [PubMed]
- Kamath A, Roudenko A, Hecht E, et al. CT/MR LI-RADS 2018: clinical implications and management recommendations. Abdom Radiol (NY) 2019;44:1306-22. [Crossref] [PubMed]
- Lee SM, Lee JM, Ahn SJ, et al. LI-RADS Version 2017 versus Version 2018: Diagnosis of Hepatocellular Carcinoma on Gadoxetate Disodium-enhanced MRI. Radiology 2019;292:655-63. [Crossref] [PubMed]
- Zeng C, Thomas DC, Lewinger JP. Incorporating prior knowledge into regularized regression. Bioinformatics 2021;37:514-21. [Crossref] [PubMed]
- Goeman JJ. L1 penalized estimation in the Cox proportional hazards model. Biom J 2010;52:70-84. [PubMed]
- Uno H, Cai T, Pencina MJ, et al. On the C-statistics for evaluating overall adequacy of risk prediction procedures with censored survival data. Stat Med 2011;30:1105-17. [Crossref] [PubMed]
- Iasonos A, Schrag D, Raj GV, et al. How to build and interpret a nomogram for cancer prognosis. J Clin Oncol 2008;26:1364-70. [Crossref] [PubMed]
- Balachandran VP, Gonen M, Smith JJ, et al. Nomograms in oncology: more than meets the eye. Lancet Oncol 2015;16:e173-80. [Crossref] [PubMed]
- Vickers AJ, Cronin AM, Elkin EB, et al. Extensions to decision curve analysis, a novel method for evaluating diagnostic tests, prediction models and molecular markers. BMC Med Inform Decis Mak 2008;8:53. [Crossref] [PubMed]
- Gigante E, Ronot M, Bertin C, et al. Combining imaging and tumour biopsy improves the diagnosis of combined hepatocellular-cholangiocarcinoma. Liver Int 2019;39:2386-96. [Crossref] [PubMed]
- Chernyak V, Fowler KJ, Kamaya A, et al. Liver Imaging Reporting and Data System (LI-RADS) Version 2018: Imaging of Hepatocellular Carcinoma in At-Risk Patients. Radiology 2018;289:816-30. [Crossref] [PubMed]
- Wakizaka K, Yokoo H, Kamiyama T, et al. Clinical and pathological features of combined hepatocellular-cholangiocarcinoma compared with other liver cancers. J Gastroenterol Hepatol 2019;34:1074-80. [Crossref] [PubMed]
- Xue R, Chen L, Zhang C, et al. Genomic and Transcriptomic Profiling of Combined Hepatocellular and Intrahepatic Cholangiocarcinoma Reveals Distinct Molecular Subtypes. Cancer Cell 2019;35:932-947.e8. [Crossref] [PubMed]
- Leoni S, Sansone V, Lorenzo S, et al. Treatment of Combined Hepatocellular and Cholangiocarcinoma. Cancers (Basel) 2020;12:794. [Crossref] [PubMed]
- Roayaie S, Blume IN, Thung SN, et al. A system of classifying microvascular invasion to predict outcome after resection in patients with hepatocellular carcinoma. Gastroenterology 2009;137:850-5. [Crossref] [PubMed]
- Andreana L, Burroughs AK. Treatment of early hepatocellular carcinoma: How to predict and prevent recurrence. Dig Liver Dis 2010;42:S249-57. [Crossref] [PubMed]
- Erstad DJ, Tanabe KK. Prognostic and Therapeutic Implications of Microvascular Invasion in Hepatocellular Carcinoma. Ann Surg Oncol 2019;26:1474-93. [Crossref] [PubMed]
- Kang Y, Massagué J. Epithelial-mesenchymal transitions: twist in development and metastasis. Cell 2004;118:277-9. [Crossref] [PubMed]
- Villanueva A, Hoshida Y, Battiston C, et al. Combining clinical, pathology, and gene expression data to predict recurrence of hepatocellular carcinoma. Gastroenterology 2011;140:1501-12.e2. [Crossref] [PubMed]
- Kim AY, Sinn DH, Jeong WK, et al. Hepatobiliary MRI as novel selection criteria in liver transplantation for hepatocellular carcinoma. J Hepatol 2018;68:1144-52. [Crossref] [PubMed]
- Yamashita Y, Tsuijita E, Takeishi K, et al. Predictors for microinvasion of small hepatocellular carcinoma ≤2 cm. Ann Surg Oncol 2012;19:2027-34. [Crossref] [PubMed]
- Zhao H, Chen C, Gu S, et al. Anatomical versus non-anatomical resection for solitary hepatocellular carcinoma without macroscopic vascular invasion: A propensity score matching analysis. J Gastroenterol Hepatol 2017;32:870-8. [Crossref] [PubMed]
- Wang T, Yang X, Tang H, et al. Integrated nomograms to predict overall survival and recurrence-free survival in patients with combined hepatocellular cholangiocarcinoma (cHCC) after liver resection. Aging (Albany NY) 2020;12:15334-58. [Crossref] [PubMed]
- Wang X, Wang W, Ma X, et al. Combined hepatocellular-cholangiocarcinoma: which preoperative clinical data and conventional MRI characteristics have value for the prediction of microvascular invasion and clinical significance? Eur Radiol 2020;30:5337-47. [Crossref] [PubMed]
- Zheng BH, Ma JQ, Tian LY, et al. The distribution of immune cells within combined hepatocellular carcinoma and cholangiocarcinoma predicts clinical outcome. Clin Transl Med 2020;10:45-56. [Crossref] [PubMed]
- Ma W, Zhang P, Qi J, et al. Prognostic value of platelet to lymphocyte ratio in hepatocellular carcinoma: a meta-analysis. Sci Rep 2016;6:35378. [Crossref] [PubMed]
- Zhang F, Lu S, Tian M, et al. Albumin-to-Alkaline Phosphatase Ratio is an Independent Prognostic Indicator in Combined Hepatocellular and Cholangiocarcinoma. J Cancer 2020;11:5177-86. [Crossref] [PubMed]
- Park SH, Lee SS, Yu E, et al. Combined hepatocellular-cholangiocarcinoma: Gadoxetic acid-enhanced MRI findings correlated with pathologic features and prognosis. J Magn Reson Imaging 2017;46:267-80. [Crossref] [PubMed]
- Fowler KJ, Sheybani A, Parker RA 3rd, et al. Combined hepatocellular and cholangiocarcinoma (biphenotypic) tumors: imaging features and diagnostic accuracy of contrast-enhanced CT and MRI. AJR Am J Roentgenol 2013;201:332-9. [Crossref] [PubMed]
- Yang L, Gu D, Wei J, et al. A Radiomics Nomogram for Preoperative Prediction of Microvascular Invasion in Hepatocellular Carcinoma. Liver Cancer 2019;8:373-86. [Crossref] [PubMed]
- Xu X, Zhang HL, Liu QP, et al. Radiomic analysis of contrast-enhanced CT predicts microvascular invasion and outcome in hepatocellular carcinoma. J Hepatol 2019;70:1133-44. [Crossref] [PubMed]
- Dageforde LA, Vachharajani N, Tabrizian P, et al. Multi-Center Analysis of Liver Transplantation for Combined Hepatocellular Carcinoma-Cholangiocarcinoma Liver Tumors. J Am Coll Surg 2021;232:361-71. [Crossref] [PubMed]
- Groeschl RT, Turaga KK, Gamblin TC. Transplantation versus resection for patients with combined hepatocellular carcinoma-cholangiocarcinoma. J Surg Oncol 2013;107:608-12. [Crossref] [PubMed]
- Iguchi T, Shirabe K, Aishima S, et al. New Pathologic Stratification of Microvascular Invasion in Hepatocellular Carcinoma: Predicting Prognosis After Living-donor Liver Transplantation. Transplantation 2015;99:1236-42. [Crossref] [PubMed]
- Le Roy B, Gelli M, Pittau G, et al. Neoadjuvant chemotherapy for initially unresectable intrahepatic cholangiocarcinoma. Br J Surg 2018;105:839-47. [Crossref] [PubMed]