Correlation of m6A methylation with immune infiltrates and poor prognosis in non-small cell lung cancer via a comprehensive analysis of RNA expression profiles
Introduction
Lung cancer is the most common cause of cancer-related deaths worldwide and is the deadliest cancer among men (1). In some developed countries, lung cancer (16.3%) has overtaken breast cancer (15.4%) as the deadliest cancer among women (1). Non-small cell lung cancer (NSCLC) is the most common type of lung cancer, and lung adenocarcinoma (LUAD) and lung squamous cell carcinoma (LUSC) are the most common subtypes (2). For patients with locally advanced or advanced NSCLC, current treatment methods cannot significantly improve overall survival (OS). However, emerging novel anti-cancer treatments, such as immunotherapy, provide a broader perspective, and the multidisciplinary treatment modalities of immunotherapy combined with other conventional methods have gradually aroused wide interest and have benefited many NSCLC patients (3). Thus, it is essential to conduct in-depth studies on the tumor immune microenvironment (TIME) to explore potential immunotherapy targets and to further optimize the clinical treatment management of NSCLC patients. 2/3 of immune cells in the lung cancer microenvironment consist of lymphocytes, 80% of which are T cells. The proportion of B cells in NSCLC tumor tissues is significantly higher than that in normal lung tissues, while the proportions of macrophages and natural killer (NK) cells are significantly lower (4). Lung cancer cells can generate immunosuppressive factors, causing immune escape and cancer progression. In addition, controversy continues as to the effect of the TIME on the long-term survival of NSCLC patients (5). Further investigations of the regulation mechanisms of the TIME may reveal effective biomarkers that can be used to accurately predict patient prognosis.
N6-methyladenosine (m6A) RNA modification can affect normal life activities and disease progression (6-8). The m6A abundance of reversible ribonucleic acids (RNAs) depends on the dynamic interplay between methyltransferases (“writers”), binding proteins (“readers”), and demethylases (“erasers”) (9). Writers include methyltransferase-like (METTL)3, METTL14, METTL16, WT1-associated protein (WTAP), Vir-like m6A methyltransferase associated (KIAA1429), zinc finger CCCH-type containing 13 (Zc3h13), RNA-binding motif protein 15 (RBM15), and RBM15B. Readers include the YTH domain-containing 1 (YTHDC1), YTHDC2, YTH m6A RNA-binding protein 1 (YTHDF1), YTHDF2, YTHDF3, RNA-binding motif protein X-linked (RBMX), and heterogeneous nuclear ribonucleoprotein C (HNRNPC), and HNRNPA2B1. Erasers mainly include the fat mass- and obesity-associated protein (FTO) and alkB homolog 5 (ALKBH5).
m6A regulators play a vital regulating role in the tumorigenesis, metastasis, and drug resistance of NSCLC. Lin et al. (10) found that METTL3 overexpression promotes the proliferation and migration of human lung cancer cells, which are significantly upregulated in LUAD tissues. Wang et al. (11) showed that m6A methylation promotes the brain metastasis of lung cancer by inducing the mature splicing of the miR-143-3p precursor. Jin et al. (12) showed that METTL3 induces drug resistance and metastasis in NSCLC. Additionally, they (13) further found that ALKBH5 interfered with the expression and activity of Yes1 Associated Transcriptional Regulator (YAP) and thereby inhibited the growth and metastasis of NSCLC. Shi et al. (14) suggested that YTHDF1 deficiency inhibited the growth of NSCLC cells and the formation of xenograft tumors by regulating the translation efficiencies of CDK2, CDK4, and cyclin D1, and the depletion of YTHDF1 made cancerous cells resistant to cisplatin treatment and restricted the progression of de novo LUAD. However, they observed unexpected results in relation to patient survival, finding that a high expression of YTHDF1 led to better clinical outcomes. Liu et al. conducted a prognostic analysis of m6A regulators in LUAD (15), and found that HNRNPC, RBM15, and KIAA1429 serve as risk genes, while YTHDC2 and METTL3 serve as protective genes. A copy number variation (CNV) analysis revealed that the OS of NSCLC patients with any CNV of m6A regulators was shorter than that of patients with the diploid gene (16). The effects of differences among all the m6A methylation levels on the long-term survival of NSCLC patients has not yet been studied, and correlations with the TIME and transduction signals have not yet been deeply analyzed.
With the increasingly extensive exploration and application of immunotherapy in clinical practice (17,18), the role of m6A methylation in tumor immunity has also attracted great attention. The pharmacological inhibition of demethylase FTO helps to reprogram the immune response of leukemia stem cells/initiating cells by regulating the expression of immune checkpoint genes (19). HNRNPA2B1 was reported to amplify interferon-α/β (IFN-α/β) production and enhance the stimulator of interferon gene (STING)-dependent cytoplasmic signaling to exert an anti-DNA virus effect (20).
In addition to the intrinsic carcinogenic pathway of m6A methylation, many studies have also revealed the special correlation between TIME immune infiltrating cells or immunophenotype and m6A modification. Zhang et al. (21) demonstrated that m6A modification patterns in gastric cancer were highly correlated with tumor immunophenotypes (immune-excluded, immune-inflamed, and immune-desert phenotypes). Han et al. (22) showed that the level of cluster of differentiation 8 positive (CD8+) T cells in YTHDF1-deficient mice exhibited higher cluster of differentiation 8 positive (CD8+) T cells levels and elevated CD8+ T cell antitumour response. Additionally, non-coding (ncRNAs), such as circular RNAs (circRNAs) have also been reported to exert an immune-suppressive effect through m6A modification (23,24). However, whether m6A methylation plays a role in the immunotherapy of NSCLC has not yet been fully explored, and the role of the interaction between ncRNAs and m6A in immunity/prognosis has not yet been clearly elucidated.
Most previous studies have focused on the potential roles of one or more m6A regulators in NSCLC. In this study, a new method was used to assess the holistic m6A modification abundance, and then to systematically analyze the correlation of m6A RNA methylation with prognosis and the TIME in NSCLC. Namely, the Weighted Gene Co-expression Network Analysis (WGCNA) algorithm was used to screen out messenger RNAs (mRNAs) and ncRNAs co-expressed with m6A methylation regulators. Clustering subtypes and prognostic risk models were established based on co-expressed RNAs to further investigate the specific function of m6A methylation in NSCLC and to improve the prognostic risk stratification of NSCLC patients. Thereafter, the correlation between m6A methylation level and the TIME/prognosis was thoroughly explored by analyzing the relationships among the clustering subgroups, the risk score, PD-L1, the immunoscore, and immune cell infiltration. This study also attempted to determine the regulatory mechanisms of m6A methylation level on immune infiltration and prognosis and to provide insights into its prospects in NSCLC immunotherapy. The following article is presented in accordance with the REMARK reporting checklist (available at https://dx.doi.org/10.21037/atm-21-4248).
Methods
Datasets
The transcriptome data of 1,037 histologically confirmed NSCLC specimens and 108 adjacent normal tissues in The Cancer Genome Atlas (TCGA) database were downloaded for the RNA differential analysis and clustering. In addition, 999 NSCLC patients with corresponding clinical data (for age, gender, TNM stage, tumor subtype, and survival data) were enrolled for further analysis. These patients were randomized into a training cohort (500 patients) and a validation cohort (499 patients) at a ratio of 5:5 using the CreateDataPartition function. The study was conducted in accordance with the Declaration of Helsinki (as revised in 2013).
Bioinformatic analysis
The limma package was used to detect mRNAs and ncRNAs that were differentially expressed between tumor and normal tissues. Next, the count data were converted into Transcripts Per Million (TPM) reads, and the mRNA and ncRNA differential expression data were merged with the m6A regulator matrix. The WGCNA package was used for the co-expression analysis (the minimum number of genes of the mRNA module was set to 20, and the minimum number of genes of the ncRNA module was set to 10). The ConsensusClusterPlus package (1,000 iterations and 80% resample rate) was used to classify the NSCLC patients into different m6A methylation subtypes. A WGCNA and principal component analysis (PCA) were conducted using the R package v4.0.3.
The gene set enrichment analysis (GSEA) was conducted on the hallmark gene sets of MSigDB to explore the potential regulatory mechanisms between the methylation subtypes, immune infiltration level, and OS. The ESTIMATE algorithm was used to calculate the immunoscore with the R “estimate package” (25). The relative proportion of 22 immune cells for each NSCLC sample was yielded through CIBERSORT (https://cibersort.stanford.edu/). The algorithm of random sampling consisted of 1,000 permutations. Only samples with a P value <0.05 were included in the subsequent analysis to compare the different immune infiltration cells among the subgroups (grouped by m6A methylation subtype and risk score). The corrplot and limma packages were used to test the correlations between PD-L1 and m6A regulators.
A total of 98 prognostic-related RNAs (82 mRNAs, 16 ncRNAs) were screened out from 535 RNAs co-expressed with the m6A regulators using the survival package. Subsequently, the prognostic-related RNAs were used for the least absolute shrinkage and selection operator (LASSO) regression analysis (26) with the optimal penalty parameter λ, and 19 core RNAs were finally identified to establish a prognostic risk model. In addition, laser regression analysis was also used to screen RNAs from 82 mRNAs and 16 ncRNAs respectively to construct mRNA model and ncRNA model. The prognostic efficacy of the three models was compared by the area under the curve (AUC), and the optimal model was selected for subsequent analysis. The coefficients generated by the optimal model were used to yield the following risk score equation: risk score = sum of coefficients × co-expressed RNA expression level. The risk scores were calculated separately for patients in the training and validation cohorts. The mean values of the risk scores was set as the cut-off point for dividing patients into high- and low-risk groups.
Statistical analysis
Statistical tests were carried out using R version 4.0.3 and GraphPad Prism 9.0. Based on published literature, 20 m6A RNA methylation regulators were collected (27-32). The expression levels of m6A regulators in tumor tissues and normal tissues were compared using unpaired t tests (the gene “VIRMA” was displayed as “KIAA1429”). A Chi-square test was performed to compare the categorical variables. The Kaplan-Meier method was used to generate survival curves, and the log rank test was used to compare differences between the groups. The correlations between the PD-L1 expression level, immunoscore, and abundance of immune cell infiltration in different subtypes and different risk groups were analyzed using the Pearson correlation test. The independent prognostic value of the risk scores was verified by Cox regression models in both the training and validation cohorts. The predictive efficiency of the 3 prognostic models for 1-, 3-, and 5-year OS was estimated using ROC curves. A P<0.05 was considered statistically significant.
Results
The expression levels of m6A regulators in tumor tissues versus normal tissues in NSCLC
To clarify the role of m6A methylation in NSCLC tumorigenesis, we systematically investigated the expression patterns of 20 m6A regulators in tumor and normal lung tissues based on TCGA database. We downloaded the expression profile data sets of 108 normal samples and 1,037 NSCLC tumor samples and analyzed the distinct expressions of the regulators. The expression levels of the “readers” (i.e., YTHDC1, YTHDF1/2/3, HNRNPC, FMR1, LRPPRC, HNRNPA2B1, and RBMX) showed consistent and significantly higher expression in NSCLC tissues than normal tissues (P<0.01; see Figure 1A). “Writers”, such as METTL3, WTAP, KIAA1429, RBM15, and RBM15B, were highly expressed in NSCLC tissues (P<0.05), while METTL14 and Zc3h13 were expressed at a lower rate in NSCLC tissues than normal tissues (P<0.01; Figure 1B). The expression patterns of “erasers” (i.e., FTO and ALKBH5) differed. Specifically, FTO was significantly downregulated in tumor tissues (P<0.001), while ALKBH5 was highly expressed (P<0.05; see Figure 1C). These results suggest that the expression patterns of various regulators in NSCLC are not consistent, and the extent of their roles in tumorigenesis might also be different. However, the universal expression differences of the regulators in tumor and normal tissues highlighted the prominence of m6A methylation.
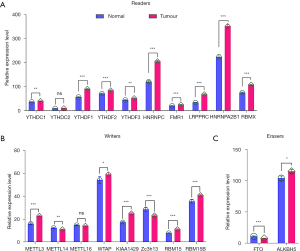
The mRNAs and ncRNAs co-expressed with m6A regulators were screened out through the WGCNA algorithm
Given the inconsistency in the expression patterns of the regulators, we adopted a new approach to investigate the role of m6A methylation in NSCLC based on the integrated RNA expression profiles. First, we filtered out the mRNAs (see Figure 2A) that were significantly differently expressed in tumor tissues versus normal tissues. Subsequently, the WGCNA algorithm was used to construct a co-expression network of m6A regulators and differentially expressed mRNAs. The soft threshold was set to 3 (β=3, R2=0.95) to construct an mRNA scale free network, and 13 modules were then identified (see Figure 2B) through average hierarchical clustering and dynamic tree clipping. The m6A regulators were mainly clustered in the turquoise and green modules. The genes in the modules were extracted (135 co-expressed mRNAs from the green module and 319 mRNAs from the turquoise module). Similarly, the ncRNAs that were differentially expressed in tumors versus normal tissues (see Figure 2C) were used to construct a co-expression network with m6A regulators through the WGCNA algorithm. The soft threshold was set to 4 (β=4, R2=0.93) to create an ncRNA scale free network, and 21 modules were identified (see Figure 2D). m6A regulators were located in the turquoise module, and a total of 400 co-expressed ncRNAs [325 long non-coding RNAs (lncRNAs), 23 microRNA (miRNAs), 28 small nucleolar RNAs (snoRNAs), and 24 small nuclear RNA (snRNAs)] were extracted. Finally, the co-expression patterns of mRNAs (see Figure 2E) and ncRNAs (see Figure 2F) with m6A regulators were further analyzed.
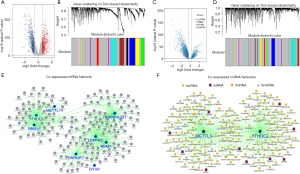
Consensus clustering for RNAs in modules co-expressed with m6A regulators
The NSCLC patients in TCGA database were clustered based on the similarity of the expression levels of the co-expressed RNAs. The lowest Proportion Ambiguous Clustering (PAC) score was generated when k=2, which was regarded as optimal clustering (see Figure 3A). 1037 NSCLC patients were clustered into 2 subtypes; that is, Cluster 1 (n=788) and Cluster 2 (n=249) (see Figure 3B). The expression patterns of RNAs among different subtypes were analyzed, and the mRNA expression levels differed significantly (see Figure 3C). The expression levels of ncRNA, except for SnoRNA, were slightly lower in Cluster 1 than Cluster 2 (see Figure 3D).
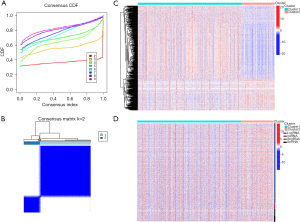
Cluster subtypes were significantly related to the clinical characteristics of NSCLC patients
An analysis of the expression patterns of the m6A regulators among subtypes revealed that some regulators had markedly higher expression levels in Cluster 1 than Cluster 2, especially HNRNPC, LRPPRC, and WTAP, which had relatively high expression levels in tumor tissues. Clustering subtypes were closely related to the clinicopathological characteristics of NSCLC patients (see Figure 4A). Cluster 2 mainly included female LUAD patients (P<0.001), while Cluster 1 mainly included patients with lymph node metastasis (P<0.001), a higher T stage (P<0.05), and at more advanced stages (P<0.05; see Figure 4B). The OS of Cluster 1 was shorter than that of Cluster 2 (P=0.002; see Figure 4C).
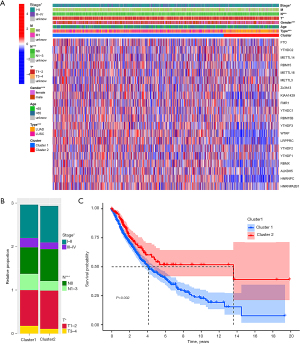
Patients in Cluster 1 had lower immunoscores, differentiated immune cell infiltration levels, and higher PD-L1 expression
To further investigate the effect of m6A methylation on the TIME in NSCLC, we assessed differences in the immunoscores and infiltration levels of 22 immune cells among the subtypes. The immunoscore in Cluster 1 was significantly lower than that in Cluster 2 (P<0.001; see Figure 5A). Cluster 1 showed higher infiltration levels of activated T cell CD4 memory, resting NK cells, M0 macrophages, M1 macrophages, activated mast cells, and eosinophils, while Cluster 2 was more highly correlated with B cell memory, resting T cell CD4 memory, regulatory T cells (Tregs), monocytes, resting dendritic cells, activated dendritic cells, and resting mast cells (see Figure 5B). Further, we found that PD-L1 expression in Cluster 1 was significantly more upregulated than that in Cluster 2 (P<0.001; see Figure 5C). In addition, the correlation between PD-L1 and various m6A regulators was further analyzed. There was a significant positive correlation in the co-expression pattern among the regulators. PD-L1 was positively correlated with WTAP, HNRNPC, FMR1, and many other regulators, while it was negatively correlated with YTHDF3 (P<0.05; see Figure 5D).
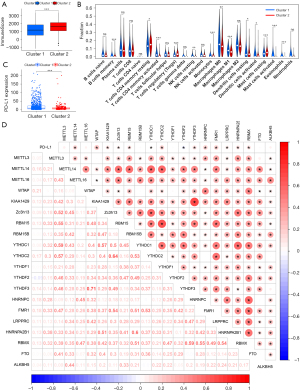
Construction and validation of the prognostic model through the integration of co-expressed mRNAs and ncRNAs
Based on the differential OS of subtypes, we inferred that m6A methylation was associated with poor prognosis in NSCLC. To further reveal the prognostic value of m6A methylation genes in NSCLC patients, we randomly divided 999 patients with survival data in TCGA database into a training cohort (500 patients) and a verification cohort (499 patients). A total of 98 co-expressed RNAs (82 mRNAs, 15 lncRNAs, and 1 miRNA; P<0.05) were screened out via a univariate Cox regression analysis. Subsequently, 19 RNAs (15 mRNAs and 4 lncRNAs) were extracted to establish a prognostic model in the training cohort through a LASSO regression analysis. Additionally, their co-expression relationship with m6A regulators was inspected (see Figure 6). The coefficients obtained by the LASSO algorithm were used to calculate the risk scores of the training and validation cohorts, and the following formula was used: risk score = cumulative addition values of (regression coefficient * RNA expression level) (see Table 1).
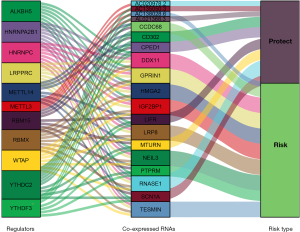
Table 1
mRNAs | Coefficient | mRNAs | Coefficient | mRNAs | Coefficient | lnRNAs | Coefficient |
---|---|---|---|---|---|---|---|
CPED1 | −0.01370 | CCDC68 | 0.02450 | TESMIN | 0.00397 | AL021368.3 | −0.05140 |
CD302 | −0.01160 | MTURN | −0.00098 | RNASE1 | −0.00001 | AC020978.2 | −0.28200 |
SCN1A | −0.03750 | LIFR | −0.00456 | IGF2BP1 | 0.00285 | AC135050.3 | −0.04870 |
NEIL3 | 0.00198 | GPRIN1 | 0.00726 | HMGA2 | 0.00164 | AC138028.6 | 0.11400 |
PTPRM | 0.00231 | DDX11 | 0.00350 | LRP8 | 0.00789 |
The coefficients of RNAs used to construct the prognostic risk model.
Based on the average risk score of the training cohort, the patients were divided into the high-risk group and low-risk group. In the training cohort, the OS of the high-risk group was significantly shorter than that of the low-risk group (P<0.001; see Figure 7A), and similar conclusions were also drawn for the validation cohort (P<0.01; see Figure 7B). To evaluate the accuracy and superiority of the prognostic model, another 2 models were constructed via a separate analysis of mRNA and ncRNA in the training cohort. By mimicking the integrated analysis process, 16 mRNAs (i.e., CPED1, CD302, SCN1A, NEIL3, PTPRM, CCDC68, MTURN, ANLN, LIFR, GPRIN1, DDX11, RNASE1, IGF2BP1, HMGA2, MT-ND6, and LRP8) and 7 ncRNAs (i.e., SH3BP5-AS1, AL122010.1, AL021368.3, AL162586.1, AC020978.2, AC135050.3, and AC138028.6) were screened out with the minimum value of the mean-square error (MSE) (λ = lambda.min). The AUC values of the 3 groups were compared by a receiver operating characteristic (ROC) curve analysis. The 1-year AUC values of the RNA integration analysis group, the mRNA group, and the ncRNA group in the training cohort were 0.71, 0.67, and 0.63, respectively (see Figure 7C). In the validation cohort, the AUC values of these groups were 0.66, 0.65, and 0.64, respectively (see Figure 7D). Additionally, we analyzed the 3-year (see Figure 7E) and 5-year (see Figure 7F) ROC curves in the training cohort to further compare the predictive power of the 3 models. These results demonstrated that the prognosis model constructed through RNA integration analysis was superior to the other 2 models and was better able to predict the prognosis of NSCLC patients.
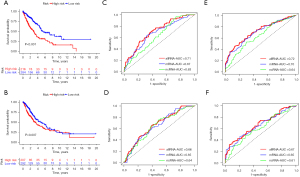
Subsequently, univariate and multivariate Cox regression analyses were performed on each cohort to verify whether the risk score calculated according to the optimal model could be used as an independent prognostic factor for NSCLC patients. In the training cohort, the univariate analysis showed that gender (P<0.01), tumor (T)T stage (P<0.001), and risk score (P<0.001) were strongly correlated with OS (see Figure 8A), while the multivariate analysis results suggested that T stage (P<0.001) and risk score (P<0.001) were closely related to OS (see Figure 8B). In the validation cohort, both the univariate and multivariate analyses (see Figure 8C,8D) showed that age (P<0.05), T stage (P<0.001), and risk score (P<0.001) were prognostic factors. Further, to prove the versatility of the model, we stratified the NSCLC patients in TCGA database by gender (see Figure 8E,8F), age (see Figure 8G,8H), tumor subtype (see Figure 8I,8J), and T stage (see Figure 8K,8L). The Kaplan-Meier curves of OS all showed that patients with high-risk scores had relatively poor survival (P<0.01).
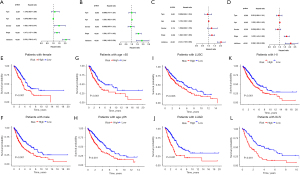
The distribution of the risk scores, OS, survival status, and prognostic-related RNA expression profiles was further investigated. As the risk score increased, the number of deaths gradually increased, and the expression levels of risky RNAs (i.e., NEIL3, GPRIN1, DDX11, TESMIN, IGF2BP1, HMGA2, LRP8, and AC138028.6) increased significantly, while the expression levels of protective RNAs (CPED1, CD302, SCN1A, MTURN, LIFR, RNASE1, AL021368.3, AC020978.2, and AC135050.3) decreased significantly. Tumor subtype, gender, T stage, node stage, and immunoscore differed significantly between the risk subgroups (see Figure 9).
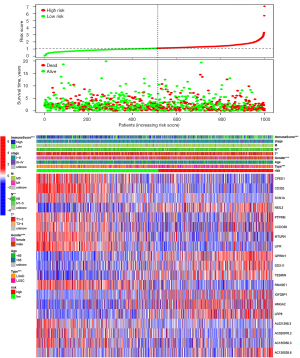
The variation patterns of the TIME in Cluster 1 were associated with higher risk scores
By comprehensively analyzing the risk score, clustering subtypes, and the TIME, we found that the risk score of Cluster 1 was significantly higher than that of Cluster 2 (P<0.001; see Figure 10A). Patients with a low immunoscore had a high-risk score (P<0.001; see Figure 10B), and there was a significant negative correlation between risk scores and immunoscores. (R=−0.342; P<0.001; see Figure 10C). In addition, the expression level of PD-L1 in the high-risk group was significantly upregulated in Cluster 1 (P<0.01; Figure 10D). Based on the correlation analysis between the infiltration level of immune cells and the risk score, we found that immune cells with a higher infiltration level in Cluster 1 [activated T cell CD4 memory (R=0.16), resting NK cells (R=0.15), activated mast cells (R=0.20), M0 macrophages (R=0.27), and M1 macrophages (R=0.15)] had a strong positive correlation with the risk score (P<0.001; see Figure 10E-10I), whereas those with a higher infiltration level in Cluster 2 [B cell memory (R=−0.19), resting T cell CD4 memory (R=−0.15), Tregs (R=−0.16), monocytes (R=−0.26), resting dendritic cells (R=−0.21), and resting mast cells (R=−0.25)] had a significant negative correlation with the risk score (P<0.001; see Figure 10J-10O). Our results indicated that the immune cells with differences in m6A methylation between clustering subtypes might be vital prognostic factors, and illustrated a conceivable mechanism whereby m6A affected the long-term survival of NSCLC patients by altering the TIME.
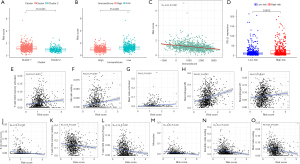
The GSEA suggested that multiple hallmarks were dynamically enriched in Cluster 1, the low-immunoscore group, and the High-Risk group
To elucidate the intrinsic regulatory mechanisms through which m6A methylation and the TIME affected the prognosis of NSCLC patients, we conducted a GSEA on Cluster subtypes, immunoscore groups, and risk stratification. Gene sets with | Normalized Enrichment Score (NES) | >1, normalized p-val <0.05, and False Discovery Rate (FDR) q-val <0.25 were considered significant. A total of 17 hallmarks (e.g., mTORC1 signaling, unfolded protein response (UPR), MYC targets V1, and E2F targets) were significantly enriched in Cluster 1 (see Table 2), 15 hallmarks (e.g., G2M checkpoint, mTORC1 signaling, MYC targets V1, and E2F targets) were enriched in the high-risk group (see Table 3), 9 hallmarks (e.g., MYC targets V2, MYC targets V1, G2M checkpoint, and E2F targets) were enriched in the low-immunoscore group (see Table 4), and no hallmark was significantly enriched in Cluster 2 and the low-risk score group. Notably, MYC targets V1/V2, the G2M checkpoint, E2F targets, DNA repair, glycolysis, UPR, and late estrogen response pathways (see Figure 11A-11H) were significantly enriched in patients with a lower immunoscore and higher risk score in Cluster 1. Our results further demonstrated that the interaction between m6A methylation and the TIME affected the long-term survival of NSCLC patients, and these hallmarks might be dynamically implicated in the poor prognosis of a distinct TIME affected by m6A methylation. Additionally, 11 hallmarks (e.g., complementary and inflammatory responses) were significantly enriched in the high-immunoscore group (see Table 5).
Table 2
HALLMARK-Name | ES | NES | NOM p-val | FDR q-val |
---|---|---|---|---|
MTORC1_SIGNALING | 0.6471 | 2.2730 | 0.0000 | 0.0000 |
UNFOLDED_PROTEIN_RESPONSE | 0.6027 | 2.2645 | 0.0000 | 0.0000 |
MYC_TARGETS_V1 | 0.7627 | 2.1995 | 0.0000 | 0.0011 |
E2F_TARGETS | 0.7654 | 2.1313 | 0.0000 | 0.0020 |
G2M_CHECKPOINT | 0.7243 | 2.0928 | 0.0000 | 0.0016 |
DNA_REPAIR | 0.5654 | 2.0429 | 0.0000 | 0.0025 |
PI3K_AKT_MTOR_SIGNALING | 0.5054 | 1.9945 | 0.0000 | 0.0049 |
MYC_TARGETS_V2 | 0.7363 | 1.9689 | 0.0000 | 0.0060 |
UV_RESPONSE_UP | 0.4329 | 1.8987 | 0.0000 | 0.0097 |
ESTROGEN_RESPONSE_LATE | 0.3657 | 1.6967 | 0.0018 | 0.0490 |
GLYCOLYSIS | 0.4686 | 1.9176 | 0.0019 | 0.0089 |
OXIDATIVE_PHOSPHORYLATION | 0.5960 | 1.9334 | 0.0060 | 0.0077 |
MITOTIC_SPINDLE | 0.5120 | 1.7669 | 0.0061 | 0.0326 |
HYPOXIA | 0.4221 | 1.7157 | 0.0170 | 0.0463 |
SPERMATOGENESIS | 0.4134 | 1.5733 | 0.0203 | 0.0927 |
P53_PATHWAY | 0.3614 | 1.5926 | 0.0264 | 0.0877 |
REACTIVE_OXYGEN_SPECIES_PATHWAY | 0.4989 | 1.6784 | 0.0273 | 0.0527 |
ES, enrichment score; NES, normalized ES; NOM p-val, normalized p value; FDR q-val, false discovery rate q value.
Table 3
HALLMARK-Name | ES | NES | NOM p-val | FDR q-val |
---|---|---|---|---|
G2M_CHECKPOINT | 0.7624 | 2.2063 | 0.0000 | 0.0023 |
MTORC1_SIGNALING | 0.6346 | 2.1950 | 0.0000 | 0.0012 |
MYC_TARGETS_V1 | 0.7439 | 2.1411 | 0.0000 | 0.0023 |
E2F_TARGETS | 0.7834 | 2.1390 | 0.0000 | 0.0017 |
MYC_TARGETS_V2 | 0.8063 | 2.1147 | 0.0000 | 0.0027 |
GLYCOLYSIS | 0.5009 | 2.0785 | 0.0000 | 0.0031 |
MITOTIC_SPINDLE | 0.5588 | 1.9556 | 0.0020 | 0.0091 |
UNFOLDED_PROTEIN_RESPONSE | 0.5064 | 1.7980 | 0.0021 | 0.0358 |
ESTROGEN_RESPONSE_LATE | 0.3504 | 1.6348 | 0.0084 | 0.0753 |
HYPOXIA | 0.4254 | 1.7375 | 0.0086 | 0.0461 |
DNA_REPAIR | 0.4800 | 1.7443 | 0.0123 | 0.0488 |
UV_RESPONSE_UP | 0.3566 | 1.6012 | 0.0139 | 0.0865 |
REACTIVE_OXYGEN_SPECIES_PATHWAY | 0.4979 | 1.7116 | 0.0203 | 0.0491 |
CHOLESTEROL_HOMEOSTASIS | 0.4188 | 1.5833 | 0.0300 | 0.0893 |
PI3K_AKT_MTOR_SIGNALING | 0.3793 | 1.4975 | 0.0353 | 0.1374 |
Table 4
HALLMARK-Name | ES | NES | NOM p-val | FDR q-val |
---|---|---|---|---|
MYC_TARGETS_V2 | 0.7678 | 2.0505 | 0.0000 | 0.0185 |
MYC_TARGETS_V1 | 0.6698 | 1.9846 | 0.0019 | 0.0108 |
G2M_CHECKPOINT | 0.6815 | 2.0272 | 0.0038 | 0.0108 |
E2F_TARGETS | 0.6982 | 1.9809 | 0.0039 | 0.0082 |
GLYCOLYSIS | 0.4068 | 1.6855 | 0.0145 | 0.0743 |
DNA_REPAIR | 0.4579 | 1.7119 | 0.0214 | 0.0740 |
WNT_BETA_CATENIN_SIGNALING | 0.4643 | 1.5358 | 0.0379 | 0.1080 |
ESTROGEN_RESPONSE_LATE | 0.2963 | 1.4047 | 0.0418 | 0.1766 |
UNFOLDED_PROTEIN_RESPONSE | 0.4209 | 1.5558 | 0.0461 | 0.1060 |
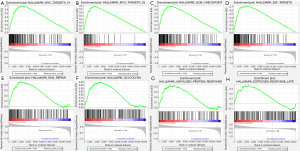
Table 5
HALLMARK-Name | ES | NES | NOM p-val | FDR q-val |
---|---|---|---|---|
ALLOGRAFT_REJECTION | 0.8122 | 2.6791 | 0.0000 | 0.0000 |
COMPLEMENT | 0.6919 | 2.6637 | 0.0000 | 0.0000 |
INFLAMMATORY_RESPONSE | 0.7326 | 2.6573 | 0.0000 | 0.0000 |
INTERFERON_GAMMA_RESPONSE | 0.8162 | 2.5633 | 0.0000 | 0.0000 |
IL6_JAK_STAT3_SIGNALING | 0.7437 | 2.5249 | 0.0000 | 0.0000 |
KRAS_SIGNALING_UP | 0.6180 | 2.5041 | 0.0000 | 0.0000 |
IL2_STAT5_SIGNALING | 0.5761 | 2.4576 | 0.0000 | 0.0000 |
INTERFERON_ALPHA_RESPONSE | 0.8049 | 2.2724 | 0.0000 | 0.0000 |
COAGULATION | 0.5678 | 2.1274 | 0.0000 | 0.0003 |
APOPTOSIS | 0.4463 | 1.9010 | 0.0000 | 0.0079 |
TNFA_SIGNALING_VIA_NFKB | 0.5936 | 2.0888 | 0.0021 | 0.0006 |
Discussion
m6A methylation is the most abundant RNA modification of eukaryotes (33), which can affect RNA metabolism through a variety of mechanisms and is associated with the occurrence and progression of many diseases. Naturally, the effect of m6A methylation on malignant tumors has attracted extensive attention (6,29,34). Shen et al. (34) found that the demethylase ALKBH5 can participate in the occurrence of acute myeloid leukemia by targeting the prognostic-related oncogenes such as transforming acidic coiled-coil containing protein 3 (TACC3). The regulatory role of m6A regulators in the metastasis and drug resistance of NSCLC has been studied (10-14,35); however, the correlation between m6A and the TIME has not been elucidated, and debate continues as to the effect of the total methylation level on the long-term survival of patients and its intrinsic mechanisms (14-16).
Immunotherapy has benefited numerous patients with lung cancer in recent clinical practice. The detection of protein PD-L1 level played an important role in the systemic treatment of NSCLC (36), in this study, we identified that NSCLC patients with elevated m6A methylation abundance tended to have higher levels of PD-L1 expression than patients with reduced m6A abundance, which may provide relevant guidance for PD-L1 therapy. However, many organs (skin, gastrointestinal tract, etc.) may be slightly to moderately affected during immunotherapy (37). Based on the analysis of the effect of m6A RNA methylation on the TIME and the potential regulatory mechanisms, more clinical treatments are likely to be exploited, and more promising immunotherapy targets might be discovered, which could further improve the long-term survival of NSCLC patients, and may also be applied to the precision treatment of tumors to reduce the incidence of adverse reactions.
In this study, the expression level and co-expression pattern with mRNA/ncRNA of m6A regulators were elucidated in NSCLC. The expression levels of METL16, Zc3h13, and FTO in tumor tissues were lower than those in normal lung tissues, while YTHDC2 expression was not significant, and the expression of 16 other regulators was significantly increased. Further, we attempted to stratify NSCLC patients using an additional methodology. Specifically, the WGCNA algorithm was used to screen out RNAs co-expressed with m6A regulators, which were used for clustering to eventually obtain 2 subtypes with differential methylation levels. METTL14, RBM15, YTHDC2, and YTHDF3 were assembled in 1 mRNA co-expression module, while WTAP, HNRNPC, LRPPRC, HNRNPA2B1, RBMX, and ALKBH5 were classified into another module. METTL, and YTHDC2 formed a co-expression network with the ncRNAs. Miscellaneous and ubiquitous ncRNAs are capable of acting as oncogenic drivers and tumor suppressors in organisms. ncRNAs modified by m6A can regulate cell proliferation and migration. Conversely, some ncRNAs can affect the expression of m6A regulators. Studies indicate that METTL3 can induce miRNA maturation by promoting the m6A modification of primary miRNA (38), which in turn can also interfere with METTL3 expression (39). Zhang et al. (40) verified that METTL3 can stimulate excessive miR-25-3p maturation and is closely related to cigarette-induced cancer transformation in patients with pancreatic ductal adenocarcinoma. Wu et al. (41) found that m6A modification can trigger the dissemination of colorectal cancer cells by affecting the expression level of lncRNAs. Based on the new perspective of the mRNA/ncRNA integrated analysis, the m6A modification level can be better assessed, and the role of m6A methylation in NSCLC can also be thoroughly explored.
Based on the subsequent analysis of cluster subtypes, some meaningful conclusions can be drawn. According to the differences in OS, PD-L1, immunoscore, and immune cell infiltration, we preliminarily inferred that the m6A methylation level affected the long-term survival of patients and the TIME in NSCLC. Many regulators (METTL14, WTAP and KIAA1429, etc.) showed a consistent positive correlation with PD-L1. At the clustering level of m6A abundance, it was also concluded that NSCLC patients in cluster 1 with higher methylation abundance tended to have higher expression levels of PDL1 than cluster 2. In hepatocellular carcinoma (42), many regulators (METTL3, RBM15B and LRPPRC, etc.) were negatively correlated with regulatory T cells (Tregs) and monocytes, and positively correlated with activated CD4 T cells. Likewise, in this study, patients in cluster 1 had increased activated CD4 T cells, decreased Tregs and monocytes.
Unexpectedly, gender differences in methylation levels were statistically significant. Relevant studies on this topic are relatively scarce; however, studies have shown that sex hormones can stimulate the growth of tumors, while estrogen receptors are differentially expressed among genders in lung cancer and can affect the prognosis of patients (43,44). Taking the results of the GSEA into consideration, we speculated that the late pathway of estrogen response might be associated with gender differences in m6A methylation, and the hallmark was significantly enriched in Cluster 1, which had a higher proportion of male patients. Using this clustering method, we observed the difference in the proportion of tumor subtypes among the cluster subgroups. Notably, Cluster 1 was closely related to lymph node metastasis and more advanced tumor stages, which are the clinicopathological features of poor prognosis. In addition, some «readers» such as HNRNPA2B1 and HNRNPC had been identified with relatively consistent changes with m6A methylation abundance, that is, patients with high expression of HNRNPA2B1 or HNRNPC were significantly enriched in cluster 1 with higher methylation abundance and poorer prognosis than cluster 2. Therefore, the m6A methylation abundance and the prognosis characteristics may be evaluated through detecting the expression levels of the above-mentioned regulators.
The prognostic risk model constructed by the integrated co-expressed RNAs was used to stratify the NSCLC patients in TCGA database to further investigate the relationship between prognostic characteristics and cluster subtypes. Cluster 1 with a shorter OS had a higher risk score. An in-depth analysis of PD-L1 expression, immunoscore, and immune cell infiltration among the risk subgroups yielded highly consistent results with the cluster subtypes. The PD-L1 expression in Cluster 1 and the high-risk group was significantly increased, and patients in Cluster 1 had lower immunoscores, which showed a significant negative correlation with the risk scores. Immune cells with a high infiltration level in Cluster 1 had a significant positive correlation with the risk score, and immune cells with a high infiltration level in Cluster 2 had a significant negative correlation with the risk score. Consequently, we inferred that the distinct OS among cluster subtypes might be closely related to the transition of the TIME. Studies have shown that lung cancer patients with higher PD-L1 expression tend to have a poor prognosis (45) and benefit more from immunotherapy (46). Research on the correlation between PD-L1 and ubiquitous m6A methylation in NSCLC patients might extend understandings and result in more individualized treatment strategies for immunotherapy. The mutual interference between tumor cells and immune cells could trigger the reprogramming of the lung microenvironment, which may promote tumor progression and metastasis and affect reactivity to immunotherapy (47,48). However, to date, the effect of m6A methylation on the tumor microenvironment of NSCLC has not been studied. Our preliminary research showed that Cluster 2, which had a lower m6A methylation level, had a higher immune infiltration score and longer OS than Cluster 1, and hallmarks, such as complementary and inflammatory responses, were significantly enriched. Thus, m6A methylation may play an important role in immunologically “cold” tumor transition and the improvement of the long-term survival of NSCLC patients. The immunologically transition may be implemented by using “writers” and “erasers” as potential therapeutic targets to reversibly change the m6A modification abundance.
To further explore the potential mechanisms of the interaction among m6A methylation, immune infiltration, and prognosis, we stratified the NSCLC patients in TCGA database for a GSEA through these 3 patterns and found that the hallmarks of the MYC targets, the G2M checkpoint, E2F targets, DNA repair, glycolysis, and the UPR were significantly enriched in patients with higher m6A methylation levels, lower immune infiltration, and higher risk scores. He et al. (29) found that if modified by m6A, the MYC gene interfered with the pathogenesis and progression of tumors. The m6A modification regulated by METTL3 and FTO acts on damaged DNA sites instantly, prompting DNA damage responses (49). Yu et al. (50) revealed that ALKBH5 inhibited the progression of bladder cancer and made it sensitive to cisplatin through the m6a-dependent glycolysis pathway. The UPR pathway in tumor-infiltrating immune cells may have immunosurveillance and immunosuppressive functions (51).
In summary, this study systematically assessed the effect of m6A RNA methylation on the TIME and prognosis of NSCLC patients via an RNA integration analysis. Two subtypes (Clusters 1 and 2) were identified by consensus clustering for RNAs co-expressed with m6A regulators, for which OS, the TIME, and the PD-L1 expression levels differed significantly. Risk score, which was developed from a prognostic risk model jointly constructed by co-expressed RNAs, was an independent prognostic indicator of patients with NSCLC. Subsequently, further investigations of the correlation between clustering subtypes and prognosis revealed that the TIME status of Cluster 1 (which had a higher PD-L1 expression level, lower immunoscore, and a higher level of infiltrating immune cells) was associated with higher risk scores. Thus, we speculated that m6A RNA methylation might lead to the poor prognosis of NSCLC patients by inducing changes in the immune microenvironment. MYC targets, the G2M checkpoint, E2F targets, DNA repair, glycolysis, and UPR pathways are potential regulatory mechanisms. It should be noted that our study had several limitations. First, our extrapolation was not validated externally due to a lack of sufficient available data. Additionally, the regulatory mechanisms of m6A methylation on the TIME warrant further investigation.
Conclusions
In conclusion, our study illustrated the possible mechanisms through which m6A methylation is related to the poor prognosis of NSCLC patients via interference with the TIME. As a reversible modification, m6A methylation might occupy a position in tumor immunotherapy in the future (e.g., by turning immunologically “cold” tumors “hot”), which could further improve the prognosis of patients.
Acknowledgments
Funding: This work was supported by the Joint Construction Project of the Henan Medical Science and Technology Project, China (Grant No. LHGJ20190301 and Grant No. 2018020067) and the National Natural Science Foundation of China (Grant No. 81773045).
Footnote
Reporting Checklist: The authors have completed the REMARK reporting checklist. Available at https://dx.doi.org/10.21037/atm-21-4248
Conflicts of Interest: All authors have completed the ICMJE uniform disclosure form (available at https://dx.doi.org/10.21037/atm-21-4248). The authors have no conflicts of interest to declare.
Ethical Statement: The authors are accountable for all aspects of the work, including ensuring that questions related to the accuracy or integrity of any part of the work have been appropriately investigated and resolved. The study was conducted in accordance with the Declaration of Helsinki (as revised in 2013).
Open Access Statement: This is an Open Access article distributed in accordance with the Creative Commons Attribution-NonCommercial-NoDerivs 4.0 International License (CC BY-NC-ND 4.0), which permits the non-commercial replication and distribution of the article with the strict proviso that no changes or edits are made and the original work is properly cited (including links to both the formal publication through the relevant DOI and the license). See: https://creativecommons.org/licenses/by-nc-nd/4.0/.
References
- Bray F, Ferlay J, Soerjomataram I, et al. Global cancer statistics 2018: GLOBOCAN estimates of incidence and mortality worldwide for 36 cancers in 185 countries. CA Cancer J Clin 2018;68:394-424. [Crossref] [PubMed]
- Yousef M, Tsiani E. Metformin in Lung Cancer: Review of in Vitro and in Vivo Animal Studies. Cancers (Basel) 2017;9:45. [Crossref] [PubMed]
- Forde PM, Chaft JE, Smith KN, et al. Neoadjuvant PD-1 Blockade in Resectable Lung Cancer. N Engl J Med 2018;378:1976-86. [Crossref] [PubMed]
- Stankovic B, Bjørhovde HAK, Skarshaug R, et al. Immune Cell Composition in Human Non-small Cell Lung Cancer. Front Immunol 2019;9:3101. [Crossref] [PubMed]
- Bremnes RM, Busund LT, Kilvær TL, et al. The Role of Tumor-Infiltrating Lymphocytes in Development, Progression, and Prognosis of Non-Small Cell Lung Cancer. J Thorac Oncol 2016;11:789-800. [Crossref] [PubMed]
- Ma S, Chen C, Ji X, et al. The interplay between m6A RNA methylation and noncoding RNA in cancer. J Hematol Oncol 2019;12:121. [Crossref] [PubMed]
- Fu Y, Dominissini D, Rechavi G, et al. Gene expression regulation mediated through reversible m6A RNA methylation. Nat Rev Genet 2014;15:293-306. [Crossref] [PubMed]
- Wang S, Sun C, Li J, et al. Roles of RNA methylation by means of N6-methyladenosine (m6A) in human cancers. Cancer Lett 2017;408:112-20. [Crossref] [PubMed]
- Yang Y, Hsu PJ, Chen YS, et al. Dynamic transcriptomic m6A decoration: writers, erasers, readers and functions in RNA metabolism. Cell Res 2018;28:616-24. [Crossref] [PubMed]
- Lin S, Choe J, Du P, et al. The m(6)A Methyltransferase METTL3 Promotes Translation in Human Cancer Cells. Mol Cell 2016;62:335-45. [Crossref] [PubMed]
- Wang H, Deng Q, Lv Z, et al. N6-methyladenosine induced miR-143-3p promotes the brain metastasis of lung cancer via regulation of VASH1. Mol Cancer 2019;18:181. [Crossref] [PubMed]
- Jin D, Guo J, Wu Y, et al. m6A mRNA methylation initiated by METTL3 directly promotes YAP translation and increases YAP activity by regulating the MALAT1-miR-1914-3p-YAP axis to induce NSCLC drug resistance and metastasis. J Hematol Oncol 2019;12:135. [Crossref] [PubMed]
- Jin D, Guo J, Wu Y, et al. m6A demethylase ALKBH5 inhibits tumor growth and metastasis by reducing YTHDFs-mediated YAP expression and inhibiting miR-107/LATS2-mediated YAP activity in NSCLC. Mol Cancer 2020;19:40. [Crossref] [PubMed]
- Shi Y, Fan S, Wu M, et al. YTHDF1 links hypoxia adaptation and non-small cell lung cancer progression. Nat Commun 2019;10:4892. [Crossref] [PubMed]
- Liu Y, Guo X, Zhao M, et al. Contributions and prognostic values of m6 A RNA methylation regulators in non-small-cell lung cancer. J Cell Physiol 2020;235:6043-57. [Crossref] [PubMed]
- Shi H, Zhao J, Han L, et al. Retrospective study of gene signatures and prognostic value of m6A regulatory factor in non-small cell lung cancer using TCGA database and the verification of FTO. Aging (Albany NY) 2020;12:17022-37. [Crossref] [PubMed]
- Tsao AS, Scagliotti GV, Bunn PA Jr, et al. Scientific Advances in Lung Cancer 2015. J Thorac Oncol 2016;11:613-38. [Crossref] [PubMed]
- Osmani L, Askin F, Gabrielson E, et al. Current WHO guidelines and the critical role of immunohistochemical markers in the subclassification of non-small cell lung carcinoma (NSCLC): Moving from targeted therapy to immunotherapy. Semin Cancer Biol 2018;52:103-9. [Crossref] [PubMed]
- Su R, Dong L, Li Y, et al. Targeting FTO Suppresses Cancer Stem Cell Maintenance and Immune Evasion. Cancer Cell 2020;38:79-96.e11. [Crossref] [PubMed]
- Wang L, Wen M, Cao X. Nuclear hnRNPA2B1 initiates and amplifies the innate immune response to DNA viruses. Science 2019;365:eaav0758 [Crossref] [PubMed]
- Zhang B, Wu Q, Li B, et al. m6A regulator-mediated methylation modification patterns and tumor microenvironment infiltration characterization in gastric cancer. Mol Cancer 2020;19:53. [Crossref] [PubMed]
- Han D, Liu J, Chen C, et al. Anti-tumour immunity controlled through mRNA m6A methylation and YTHDF1 in dendritic cells. Nature 2019;566:270-4. [Crossref] [PubMed]
- Huang H, Weng H, Chen J. m6A Modification in Coding and Non-coding RNAs: Roles and Therapeutic Implications in Cancer. Cancer Cell 2020;37:270-88. [Crossref] [PubMed]
- Chen YG, Chen R, Ahmad S, et al. N6-Methyladenosine Modification Controls Circular RNA Immunity. Mol Cell 2019;76:96-109.e9. [Crossref] [PubMed]
- Yoshihara K, Shahmoradgoli M, Martínez E, et al. Inferring tumour purity and stromal and immune cell admixture from expression data. Nat Commun 2013;4:2612. [Crossref] [PubMed]
- Bøvelstad HM, Nygård S, Størvold HL, et al. Predicting survival from microarray data--a comparative study. Bioinformatics 2007;23:2080-7. [Crossref] [PubMed]
- An S, Huang W, Huang X, et al. Integrative network analysis identifies cell-specific trans regulators of m6A. Nucleic Acids Res 2020;48:1715-29. [Crossref] [PubMed]
- Chen M, Wong CM. The emerging roles of N6-methyladenosine (m6A) deregulation in liver carcinogenesis. Mol Cancer 2020;19:44. [Crossref] [PubMed]
- He L, Li H, Wu A, et al. Functions of N6-methyladenosine and its role in cancer. Mol Cancer 2019;18:176. [Crossref] [PubMed]
- Li Y, Xiao J, Bai J, et al. Molecular characterization and clinical relevance of m6A regulators across 33 cancer types. Mol Cancer 2019;18:137. [Crossref] [PubMed]
- Worpenberg L, Paolantoni C, Longhi S, et al. Ythdf is a N6-methyladenosine reader that modulates Fmr1 target mRNA selection and restricts axonal growth in Drosophila. EMBO J 2021;40:e104975 [Crossref] [PubMed]
- Arguello AE, DeLiberto AN, Kleiner RE. RNA Chemical Proteomics Reveals the N6-Methyladenosine (m6A)-Regulated Protein-RNA Interactome. J Am Chem Soc 2017;139:17249-52. [Crossref] [PubMed]
- Yue Y, Liu J, He C. RNA N6-methyladenosine methylation in post-transcriptional gene expression regulation. Genes Dev 2015;29:1343-55. [Crossref] [PubMed]
- Shen C, Sheng Y, Zhu AC, et al. RNA Demethylase ALKBH5 Selectively Promotes Tumorigenesis and Cancer Stem Cell Self-Renewal in Acute Myeloid Leukemia. Cell Stem Cell 2020;27:64-80.e9. [Crossref] [PubMed]
- Choe J, Lin S, Zhang W, et al. mRNA circularization by METTL3-eIF3h enhances translation and promotes oncogenesis. Nature 2018;561:556-60. [Crossref] [PubMed]
- Arbour KC, Riely GJ. Systemic Therapy for Locally Advanced and Metastatic Non-Small Cell Lung Cancer: A Review. Jama 2019;322:764-74. [Crossref] [PubMed]
- Wang J, Li J, Cai L, et al. The safety and efficacy of neoadjuvant programmed death 1 inhibitor therapy with surgical resection in stage IIIA non-small cell lung cancer. Ann Transl Med 2021;9:486. [Crossref] [PubMed]
- Alarcón CR, Lee H, Goodarzi H, et al. N6-methyladenosine marks primary microRNAs for processing. Nature 2015;519:482-5. [Crossref] [PubMed]
- Cai X, Wang X, Cao C, et al. HBXIP-elevated methyltransferase METTL3 promotes the progression of breast cancer via inhibiting tumor suppressor let-7g. Cancer Lett 2018;415:11-9. [Crossref] [PubMed]
- Zhang J, Bai R, Li M, et al. Excessive miR-25-3p maturation via N6-methyladenosine stimulated by cigarette smoke promotes pancreatic cancer progression. Nat Commun 2019;10:1858. [Crossref] [PubMed]
- Wu Y, Yang X, Chen Z, et al. m6A-induced lncRNA RP11 triggers the dissemination of colorectal cancer cells via upregulation of Zeb1. Mol Cancer 2019;18:87. [Crossref] [PubMed]
- Shen S, Yan J, Zhang Y, et al. N6-methyladenosine (m6A)-mediated messenger RNA signatures and the tumor immune microenvironment can predict the prognosis of hepatocellular carcinoma. Ann Transl Med 2021;9:59. [Crossref] [PubMed]
- Schwartz AG, Prysak GM, Murphy V, et al. Nuclear estrogen receptor beta in lung cancer: expression and survival differences by sex. Clin Cancer Res 2005;11:7280-7. [Crossref] [PubMed]
- Frega S, Dal Maso A, Ferro A, et al. Heterogeneous tumor features and treatment outcome between males and females with lung cancer (LC): Do gender and sex matter? Crit Rev Oncol Hematol 2019;138:87-103. [Crossref] [PubMed]
- Zhang M, Li G, Wang Y, et al. PD-L1 expression in lung cancer and its correlation with driver mutations: a meta-analysis. Sci Rep 2017;7:10255. [Crossref] [PubMed]
- Mok TSK, Wu YL, Kudaba I, et al. Pembrolizumab versus chemotherapy for previously untreated, PD-L1-expressing, locally advanced or metastatic non-small-cell lung cancer (KEYNOTE-042): a randomised, open-label, controlled, phase 3 trial. Lancet 2019;393:1819-30. [Crossref] [PubMed]
- Zhao S, Ren S, Jiang T, et al. Low-Dose Apatinib Optimizes Tumor Microenvironment and Potentiates Antitumor Effect of PD-1/PD-L1 Blockade in Lung Cancer. Cancer Immunol Res 2019;7:630-43. [Crossref] [PubMed]
- Altorki NK, Markowitz GJ, Gao D, et al. The lung microenvironment: an important regulator of tumour growth and metastasis. Nat Rev Cancer 2019;19:9-31. [Crossref] [PubMed]
- Xiang Y, Laurent B, Hsu CH, et al. RNA m6A methylation regulates the ultraviolet-induced DNA damage response. Nature 2017;543:573-6. [Crossref] [PubMed]
- Yu H, Yang X, Tang J, et al. ALKBH5 Inhibited Cell Proliferation and Sensitized Bladder Cancer Cells to Cisplatin by m6A-CK2α-Mediated Glycolysis. Mol Ther Nucleic Acids 2021;23:27-41. [Crossref] [PubMed]
- Vanacker H, Vetters J, Moudombi L, et al. Emerging Role of the Unfolded Protein Response in Tumor Immunosurveillance. Trends Cancer 2017;3:491-505. [Crossref] [PubMed]
(English Language Editor: L. Huleatt)