A novel immune-related ceRNA network that predicts prognosis and immunotherapy response in lung adenocarcinoma
Introduction
Lung cancer is one of the most frequently diagnosed malignancies, and is a leading cause of cancer-related mortality worldwide (1). Lung adenocarcinoma is the most common histological subtype of lung cancer, and accounts for about 50% of cases. Despite significant developments in the diagnosis and clinical treatment of lung adenocarcinoma, the 5-year survival rate of patients remains poor (2). Immune checkpoint blockade therapy is a promising therapeutic strategy for treating lung adenocarcinoma patients. However, patients’ responses vary greatly. There is increasing evidence that the tumor microenvironment plays an important role in the progression and malignancy of lung adenocarcinoma and affects the immunotherapy response (3). Tumor microenvironment is comprised of tumor-infiltrating immune cells, tumor cells, and stroma. Cancer immunotherapy alleviates tumor-associated suppression of anticancer immune response by promoting T lymphocyte activation. Tumor-infiltrating B lymphocytes could generate antibodies and anticancer cytokines, present cancer-related antigens, and kill tumor cells directly. B cells and associated tertiary lymphoid structures contribute to the antitumor activity of immunotherapy (4). Loss of the capacity to attract innate myeloid effector cells into the tumor microenvironment can lead to primary or secondary therapy resistance (5). The complexity and heterogeneity of tumor microenvironment increase the difficulty of cancer immunotherapy and lead to the variable efficacy in immunotherapy (6). Thus, it is important to understand the lung adenocarcinoma immune microenvironment and identify new molecular markers to guide future lung adenocarcinoma treatments.
The Encyclopedia of Deoxyribonucleic Acid Elements (ENCODE) Consortium revealed that over 70% of the human genome can be transcribed into RNA (the landscape of transcription in human cells). Less than 2% of the human genome is comprised of protein-coding genes, while most of the transcripts are non-coding genes, including long non-coding RNAs (lncRNAs), pseudogenes, and microRNAs (miRNAs) (7). LncRNAs are a class of non-coding RNA that are more than 200 nucleotides in length, and which used to be considered transcriptional noise. Recent studies have shown that lncRNAs are involved in various cellular processes, such as differentiation, the immune response, cancer cell metastasis, proliferation, and drug resistance (8-10). Besides modulating the tumor microenvironment and tumor progression, lncRNAs play significant roles in the interactions between tumor cells and stromal cells (11). For example, tumor-derived exosomal lncRNA TUC339 is found to be critical for the regulation of macrophage M1/M2 polarization (12). MiRNAs are a class of small endogenous single-stranded non-coding RNA that play a vital role in the development and metastasis of lung adenocarcinoma. MiRNAs can also have a fundamental regulative impact on both innate and adaptive immune cells by targeting cellular signaling hubs. MiRNAs can be secreted by cancer cells, consecutively taken up by immune cells, and influence immune functions (13). MiRNAs facilitate the degradation of the target messenger RNA (mRNAs) or suppress their translation by binding the miRNA response elements (MREs) of the target mRNAs. MREs are also present in lncRNAs, and transcribed pseudogenes. RNAs that contain MREs can act as sponges that relieve mRNA targets from repression or indirectly induce target mRNA repression by releasing miRNAs from this reservoir. LncRNAs have the potential to sponge miRNAs and thus affect the expression of mRNAs (14). Zhu et al. focused on immune gene-related lncRNA-miRNA-mRNA-competing endogenous RNA (ceRNA) networks that were different between tumors and non-tumor samples in lung adenocarcinoma (15). Wei et al. explored B cell immunity-related ceRNA networks in lung adenocarcinoma (16). However, understandings of the association of immune-related ceRNA networks and immunotherapy response and prognosis of lung adenocarcinoma remain very limited.
In the present study, the expression profiles and clinical information for lung adenocarcinoma were extracted from The Cancer Genome Atlas (TCGA). The immune microenvironment was examined using the single-sample gene set enrichment analysis (ssGSEA). The lung adenocarcinoma tumor samples were divided into high and low immunity groups using unsupervised clustering based on their ssGSEA scores. The differently expressed genes were selected. Survival analysis and predicted RNA interaction pairs were used to construct a prognosis-related-ceRNA network. Additionally, associations between the ceRNA network and tumor microenvironment immune cell infiltration and immunotherapy response were explored. In summary, we constructed a novel ceRNA network (SNHG6-has-miR-30e-5p-CYSLTR1) that has a potential prognostic value for lung adenocarcinoma patients and might facilitate personalized counseling for immunotherapy. We present the following article in accordance with the REMARK reporting checklist (available at https://dx.doi.org/10.21037/atm-21-4151).
Methods
Data source
RNA-sequencing (RNA-seq) data (level 3), miRNA-seq data (level 3), and clinical data for lung adenocarcinoma were downloaded from TCGA database (https://cancergenome.nih.gov/), including lung adenocarcinoma tissue and adjacent non-cancerous lung tissue data. The SEQ data were derived from publicly available Illumina HiSeq RNA-seq and miRNA-seq platforms. Approval by an ethics committee was not required for this study. GENCODE (https://www.gencodegenes.org/) was used to convert the RNA-seq data into lncRNAs and mRNAs. The study was conducted in accordance with the Declaration of Helsinki (as revised in 2013).
Statistical analysis
Immune grouping
The 29 immune-related gene sets of the immune cells were obtained from a previous study (17). A ssGSEA was conducted to calculate the immune activity score of each lung adenocarcinoma sample based on the transcriptomic expression data and the 29 immune-related gene sets. Next, unsupervised clustering was used to divide the lung adenocarcinoma tumor samples into two groups. The group with high ssGSEA scores was considered the high immunity group, while the other was the low immunity group (18). To verify the validity of the immune grouping, the stromal, immune score, Estimation of STromal and Immune cells in MAlignant Tumor tissues using Expression data (ESTIMATE), and tumor purity scores were calculated using the ESTIMATE algorithm (https://bioinformatics.mdanderson.org/estimate/) (19). The results were plotted and are shown in the clustering heat map and statistical map.
Identification of differentially expressed mRNAs (DEmRNAs), differentially expressed lncRNAs (DElncRNAs), and differentially expressed miRNAs (DEmiRNAs)
Genes with a low expression level (i.e., an expression value of 0, which accounted for >50% of the genes) were removed from TCGA sequence data. To distinguish between the mRNAs, lncRNAs, and miRNAs that were differentially expressed between the high and low immunity groups, the “limma” package in R software was used to standardize and analyze the expression data with the criteria of |log2 fold change (FC)| >1.0 and adjusted P value <0.05 (20). Heatmap clustering and volcano plots were used to depict the differentially expressed lncRNAs, mRNAs, and miRNAs.
Construction of a weighted gene co-expression network of DEmRNAs and the identification and functional characterization of modules associated with immune status
A weighted gene co-expression network of the DEmRNAs was constructed using the weighted gene co-expression network analysis (WGCNA) package (21). The network modules were generated using the topological overlapping measurement with a power cutoff threshold of 5 and a minimum module size of 50. Pearson’s correlation tests were then used to analyze correlations between clinical traits and the modules. Protein interactions among the DEmRNAs in the immune modules were identified, and a protein-protein interaction (PPI) network was constructed (a minimum required interaction score >0.7 was required) using the search tool for recurring instances of neighboring genes (STRING) online tools (version 11.0) (22). Additionally, Cytoscape software (version 3.8.2) was used to construct the PPI networks (23). The Cytoscape plug-in Molecular Complex Detection (MCODE) was applied to detect notable modules in the PPI network (24). The advanced options were set as degree cutoff =2, node score cutoff =0.2, and K-core =2. Enrichment analysis of the genes in the selected notable modules was performed using Gene Ontology (GO) biological processes (BPs) and Kyoto Encyclopedia of Genes and Genomes (KEGG) pathways (25,26).
Prediction of RNA interaction pairs and ceRNA network construction
The TargetScan v7.2 (http://www.targetscan.org/) and DIANA-microT-CDS v5.0 (http://diana.imis.athena-innovation.gr/DianaTools/index.php?r=microT_CDS/index) databases were used to predict the target genes of DEmiRNAs and miRNA-mRNA interactions (27,28). A “VennDiagram” was used to identify overlapping miRNA-mRNA pairs. The LncRNA-miRNA interactions were predicted by miRcode (http://www.mircode.org/) (29). The lncRNA-miRNA pairs and miRNA-mRNA pairs were screened to construct the lncRNA-miRNA-mRNA network using the Cytoscape software (version 3.8.2).
Survival analysis
To identify the hub ceRNA network associated with patient prognosis, the relationship between overall survival time and the mRNAs, miRNAs, and lncRNAs in the ceRNA network were analyzed using the “survival” and “survminer” packages. Genes with a value <0.05 were considered the key nodes for the construction of the next network.
Identification of the mRNAs in the network associated with the response to immunotherapy
GSE126044 was downloaded from the Gene Expression Omnibus (GEO) database (https://www.ncbi.nlm.nih.gov/geo/query/acc.cgi?acc=GSE126044). Seven-four non-small cell lung cancer patients were administered either nivolumab or pembrolizumab. The mRNAs in the ceRNA network were analyzed using the limma package to screen DEmRNAs between the response group and the non-response group. mRNAs with a P value <0.05 were considered for the construction of the next network.
Construction of the ceRNA network associated with patient prognosis and immunotherapy response
The DEmRNAs, DEmiRNAs, and DElncRNAs associated with patient prognosis, and the DEmRNAs associated with immunotherapy response in the lncRNA-miRNA pairs and miRNAs-mRNAs were screened to construct the next ceRNA network and visualized by Cytoscape software (version 3.8.2).
Associations between immune cell infiltration and ceRNA network
The Tumor IMmune Estimation Resource (TIMER) (https://cistrome.shinyapps.io/timer/) algorithm was used to quantify the correlation of hub genes in the ceRNA network with the abundance of six types of infiltrating immune cells in lung adenocarcinoma patients (30). According to the median expression values of hub genes of the ceRNA network, the available data were split into low and high expression groups to evaluate the difference in the proportion of immune cells. The correlations between the hub genes of the ceRNA network and the immune, stromal, and ESTIMATE scores were explored.
Results
Construction and verification of lung adenocarcinoma immune groupings
The RNA-seq data of 513 lung adenocarcinoma tissues, the miRNA-seq data of 507 lung adenocarcinoma tissues, and relative clinical data were obtained from TCGA. The lung adenocarcinoma samples were divided into high and low immune cell infiltration groups using an unsupervised hierarchical clustering algorithm according to immune infiltration. The ESTIMATE algorithm was used to verify the feasibility of the above grouping based on ssGSEA. The high immune cell infiltration group had higher immune, stromal, and ESTIMATE scores, but lower tumor purity scores (see Figure 1).
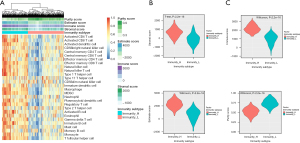
Differential expression of lncRNAs, miRNAs, and mRNAs in lung adenocarcinoma patients
Differentially expressed lncRNAs, miRNAs, and mRNAs were screened by comparing the difference in the expression levels of these RNAs between the high and low immune cell infiltration groups. In total, 7,538 DEmRNAs (2,121 upregulated and 5,417 downregulated), 540 DElncRNAs (104 upregulated and 436 downregulated), and 138 DEmiRNAs (57 upregulated and 81 downregulated) were identified. The heat maps show the most significant genes of the top 30 mRNAs, lncRNAs, and miRNAs (see Figure 2).
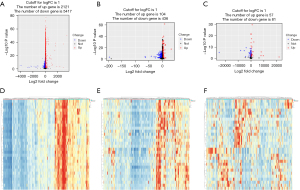
Identification of the most significant modules by WGCNA and hub genes
WGCNA was used to categorize DEmRNAs into different gene modules that may function in the same biological way. A soft threshold of five was selected to construct a scale-free network. A total of 23 gene modules were identified after setting the minimum cluster size as 50. The blue module (R=0.66, P=2e−64) and green module (R=0.63, P=4e−59) were most significantly associated with immune cell infiltration. Pathway enrichment demonstrated that the genes in the two modules were mainly involved in the innate immune response, lymphocyte activation, cytokine-mediated signaling pathway, leukocyte mediated immunity, and inflammatory response (see Figure 3) The 793 DEmRNAs in the blue module and 465 DEmRNAs in the green module were selected to construct the PPI network using the STRING database (see Figure S1).
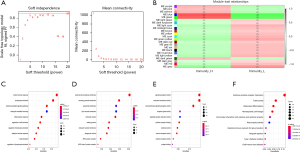
The interactions between the DEmRNAs in the whole network were explored using Cytoscape MCODE. The following four clusters with higher MCODE scores were selected for further analysis: Cluster 1 (74 nodes and 1,350 edges), Cluster 2 (41 nodes and 429 edges), Cluster 3 (54 nodes and 403 edges), and Cluster 4 (10 nodes and 45 edges). The pathway enrichment demonstrated that the four clusters, including 179 DEmRNAs, were mainly involved in regulating the immune response (see Figure 4).
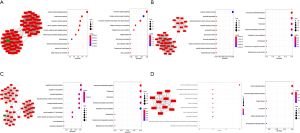
Construction of the ceRNA network
The target genes of the 138 DEmiRNA were predicted by the TargetScan and microT-CDS databases. After overlapping the miRNA-mRNA pairs, we identified 73 miRNAs and 78 mRNAs in the 159 miRNA-mRNA pairs (see Figure 5A). The miRcode matching indicated that 27 lncRNAs and 66 miRNAs effectively generated 467 pairs of associations. Thus the miRNA-mRNA and lncRNA-miRNA pairs were used to build the lncRNA-miRNA-mRNA-ceRNA network, which contained 16 DEmRNAs (16 upregulated), 23 DElncRNAs (6 upregulated and 17 downregulated), and 10 DEmiRNAs (7 upregulated and 3 downregulated). The network was visualized using Cytoscape software (see Figure 5B).
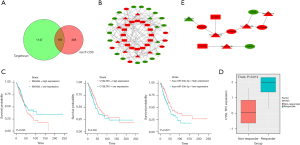
The overall survival rates of the DEmRNAs, DElncRNAs, and DEmiRNAs of the ceRNA network were explored by Kaplan-Meier. Six DEmRNAs [i.e., cluster differentiation (CD) 80, Major Histocompatibility Complex, Class II, DQ Beta 1 (HLA-DQB1), CIITA, CYSLTR1, KETEDS, and GRAP2], 6 DElncRNAs (LINC00324, SNHG6, HLA-DQB1-AS1, CRNDE, LINC00426, and LIN00205), 4 DEmiRNAs (has-miR-140-5p, has-miR-148a-3p, has-miR-199a-3p, and has-miR-30e-5p) met the survival significance criterion for lung adenocarcinoma (see Table 1 and Figure 5C).
Table 1
Gene symbol | Type | Coefficient | HR | 95% CI lower | 95% CI higher | P value |
---|---|---|---|---|---|---|
|
mRNA | 0.310 | 1.363 | 1.013 | 1.833 | 0.041 |
|
mRNA | 0.493 | 1.637 | 1.216 | 2.204 | 0.001 |
|
mRNA | 0.305 | 1.357 | 1.01 | 1.824 | 0.043 |
|
mRNA | 0.321 | 1.378 | 1.027 | 1.849 | 0.033 |
|
mRNA | 0.313 | 1.367 | 1.017 | 1.838 | 0.038 |
|
mRNA | 0.516 | 1.675 | 1.239 | 2.266 | 0.001 |
|
lncRNA | 0.443 | 1.557 | 1.158 | 2.093 | 0.003 |
|
lncRNA | −0.303 | 0.739 | 0.55 | 0.992 | 0.044 |
|
lncRNA | 0.366 | 1.442 | 1.069 | 1.943 | 0.016 |
|
lncRNA | 0.465 | 1.592 | 1.184 | 2.14 | 0.002 |
|
lncRNA | 0.449 | 1.566 | 1.162 | 2.111 | 0.003 |
|
lncRNA | −0.306 | 0.736 | 0.549 | 0.987 | 0.041 |
|
miRNA | 0.307 | 1.36 | 1.011 | 1.829 | 0.042 |
|
miRNA | 0.402 | 1.495 | 1.112 | 2.01 | 0.008 |
|
miRNA | 0.322 | 1.379 | 1.025 | 1.856 | 0.034 |
|
miRNA | 0.491 | 1.633 | 1.213 | 2.199 | 0.001 |
|
miRNA | 0.316 | 1.372 | 1.02 | 1.844 | 0.036 |
HR, hazard ratio; CI, confidence interval.
The DEmRNAs were further analyzed in GSE126044. Four DEmRNAs (i.e., CD80, HLA-DQB1, CIITA, and CYSLTR1) were differentially expressed between the response group and the non-response group in GSE126044 (see Figure 5D and Figure S2). Based on the ceRNA network, the SNHG6-has-miR-30e-3p-CYSLTR1 axis was found to be related to patient prognosis and immunotherapy response (see Figure 5E).
Validation of the correlation of the hub genes in the ceRNA network and immune cell infiltration
CYSLTR1 expression was found to be positively associated with immune cell infiltration, the stromal score, immune score, and ESTIMATE score, but negatively associated with the purity score. SNHG6 expression was found to be negatively associated with immune cell infiltration, the stromal score, immune score, and ESTIMATE score, but positively associated with the purity score. The CYSLTR1 expression levels were positively correlated with the infiltrating levels of CD8+ T cells, CD4+ T cells, B cells, neutrophils, macrophages, and dendritic cells. SNHG6 expression levels were inversely correlated with infiltrating levels of neutrophils, macrophages, and dendritic cells, but not CD8+ T cells, CD4+ T cells, or B cells (see Figure 6).
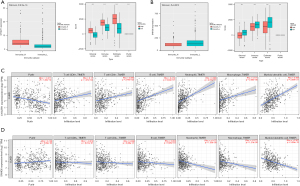
Discussion
Lung adenocarcinoma is the most common type of lung cancer and is one of the leading causes of cancer-related deaths. Because of the substantial heterogeneity of lung adenocarcinoma, it is difficult to develop individualized treatments and predict outcomes. Lung adenocarcinoma tissue is not only composed of lung adenocarcinoma cells, it is also composed of a mixture of many kinds of normal cells, such as immune cells, stromal cells, and fibroblasts. These different types of cells form a complex tumor microenvironment. Given the importance of the interaction between tumor-infiltrating immune cells and tumor cells, it is of crucial significance to mine molecular events that are related to the tumor immune microenvironment to uncover the predictive biomarkers associated with survival and immunotherapy response. In the current study, we found the SNHG6-hsa-miR-30e-5p-CYSLTR1 ceRNA network related to immune cell infiltration was associated with the prognosis of and immunotherapy response in lung adenocarcinoma using the transcriptome seq data and clinical features of lung adenocarcinoma obtained from TCGA.
Various clinical trials have shown that tumor microenvironment immune cell infiltration is critical in predicting the prognosis and immunotherapy response of patients (31,32). We conducted a comprehensive analysis of the tumor microenvironment immune cell infiltration landscape by estimating the abundance of 29 immune cell types in lung adenocarcinoma. The high immune cell infiltration group had higher immune, stromal, and ESTIMATE scores, but lower tumor purity scores than the low immune cell infiltration group. Seven thousand five hundred and thirty-eight mRNAs, 540 lncRNAs, and 138 miRNAs were found to be differentially expressed between the high and low immune cell infiltration groups. WGCNA revealed that two DEmRNA modules were most significantly associated with immune cell infiltration. Those two modules were further analyzed by STRING and Cytoscape MCODE. Four clusters were selected based on the MCODE scores. A pathway enrichment analysis demonstrated that the DEmRNAs selected in the four clusters were mainly involved in regulating the immune response.
Salmena et al. proposed that non-coding RNAs like lncRNAs could act as natural miRNAs sponges by competitively binding to the MREs of target mRNAs. A number of studies have shown that the ceRNA network of lncRNA-miRNA-mRNA interactions is involved in the regulation of tumor progression, prognosis, and therapy responses (14). LncRNA LCAT1 functions as a ceRNA to regulate RAC1 function by sponging miR-4715-5p, which plays an important role in the progression of lung cancer (33). LncRNA UCA1 promoted Gefitinib resistance as a ceRNA to target FOSL2 by sponging miR-143 in non-small cell lung cancer (34). The present study found that the novel immune-related ceRNA network of SNHG6-hsa-miR-30e-5p-CYSLTR1 ceRNA network is associated with the prognosis and immunotherapy response of lung adenocarcinoma. LncRNA SNHG6 plays an oncogenic role in various types of cancer, such as colorectal cancer, breast cancer, and clear cell renal cell carcinoma (35-37). SNHG6 interacts with glucocorticoid-induced tumor necrosis factor receptor-related protein to regulate cisplatin resistance by competitively binding to miR-325-3p in gastric cancer cells (38). SNHG6 is a poor prognostic factor for colorectal cancer patients (39). SNHG6 expression levels were not found to be correlated with infiltrating levels of CD8+ T cells, CD4+ T cells, B cells, but were found to be inversely correlated with infiltrating levels of neutrophils, macrophages, and dendritic cells. CYSLTR1 was widely distributed in a range of cells of the innate immune system, including basophils, mast cells, dendritic cells, eosinophils, and monocytes/macrophages, as well as B cells and CD4+ T cells, and to a lesser extent, neutrophils and CD8+ cells (40). Barrett et al. found that CYSLTR1 promotes nascent Th2 immune responses by facilitating the organization of the immune synapse (41). CYSLTR1 plays a key role in cysteinyl leukotrienes, inducing the activation and migration of human monocytes (42). CYSLTR1 is an indicator of patient prognosis in colorectal cancer, breast cancer, and pancreatic adenocarcinoma (43-45). Hsa-miR-30e-5p has anti-tumor effects on various kinds of cancers, such as bladder cancer, nasopharyngeal carcinoma, squamous cell carcinoma of the head and neck, and non-small cell lung cancer (46-49). Hsa-miR-30e-5p plays an integrated role in the regulation of the innate immune response (50,51). Hsa-miR-30e-5p plays an important role in circMET by driving immunosuppression and anti-PD1 therapy resistance (52).
This study had several limitations. First, the data in our study were obtained from public databases, such as TCGA and GEO databases. Thus, the quality of the data could not be appraised. Second, the ceRNA networks have not been verified in clinical lung adenocarcinoma samples. Multi-centered validation especially large scare prospective studies are still required before the ceRNA network can be applied in clinical practice. The expression of the ceRNAs and immune cell markers should be detected in the same samples via immunohistochemistry analysis or quantitative real-time PCR and analyzed the associations. Finally, further functional studies need to be conducted to explore the molecular functions (MFs) of the SNHG6-hsa-miR-30e-5p-CYSLTR1 ceRNA network in lung adenocarcinoma progression and the immunotherapy response. Gain- and loss-of-function experiments are needed to investigate the biological functions of the ceRNA network both in vitro and in vivo.
Conclusions
In this study, we constructed an immune-related ceRNA network of SNHG6-hsa-miR-30e-5p-CYSLTR1, which was found to be associated with the prognosis of and immunotherapy response in lung adenocarcinoma. However, further experiments are required to verify the clinical and biological functions of the ceRNA network.
Acknowledgments
Funding: This work was supported by grants from the National Natural Science Foundation of China (No. 82003868), Hubei Provincial Natural Science Foundation of China (No. 2020CFB388), and Key Research and Development Program of Hubei Province (No. 2020BCA060).
Footnote
Reporting Checklist: The authors have completed the REMARK reporting checklist. Available at https://dx.doi.org/10.21037/atm-21-4151
Conflicts of Interest: All authors have completed the ICMJE uniform disclosure form (available at https://dx.doi.org/10.21037/atm-21-4151). The authors have no conflicts of interest to declare.
Ethical Statement: The authors are accountable for all aspects of the work in ensuring that questions related to the accuracy or integrity of any part of the work are appropriately investigated and resolved. The study was conducted in accordance with the Declaration of Helsinki (as revised in 2013).
Open Access Statement: This is an Open Access article distributed in accordance with the Creative Commons Attribution-NonCommercial-NoDerivs 4.0 International License (CC BY-NC-ND 4.0), which permits the non-commercial replication and distribution of the article with the strict proviso that no changes or edits are made and the original work is properly cited (including links to both the formal publication through the relevant DOI and the license). See: https://creativecommons.org/licenses/by-nc-nd/4.0/.
References
- Siegel RL, Miller KD, Fuchs HE, et al. Cancer statistics, 2021. CA Cancer J Clin 2021;71:7-33. [Crossref] [PubMed]
- AbdulJabbar K. Geospatial immune variability illuminates differential evolution of lung adenocarcinoma. Nat Med 2020;26:1054-62. [Crossref] [PubMed]
- Lou Y, Diao L, Cuentas ER, et al. Epithelial-mesenchymal transition is associated with a distinct tumor microenvironment including elevation of inflammatory signals and multiple immune checkpoints in lung adenocarcinoma. Clin Cancer Res 2016;22:3630-42. [Crossref] [PubMed]
- Kim SS, Sumner WA, Miyauchi S, et al. Role of B cells in responses to checkpoint blockade immunotherapy and overall survival of cancer patients. Clin Cancer Res 2021; Epub ahead of print. [Crossref] [PubMed]
- Beyranvand Nejad E, Labrie C, Abdulrahman Z, et al. Lack of myeloid cell infiltration as an acquired resistance strategy to immunotherapy. J Immunother Cancer 2020;8:e001326 [Crossref] [PubMed]
- Klemm F, Joyce JA. Microenvironmental regulation of therapeutic response in cancer. Trends Cell Biol 2015;25:198-213. [Crossref] [PubMed]
- Davis CA, Hitz BC, Sloan CA, et al. The encyclopedia of DNA elements (ENCODE): data portal update. Nucleic Acids Res 2018;46:D794-801. [Crossref] [PubMed]
- Gong WJ, Yin JY, Li XP, et al. Association of well-characterized lung cancer lncRNA polymorphisms with lung cancer susceptibility and platinum-based chemotherapy response. Tumour Biol 2016;37:8349-58. [Crossref] [PubMed]
- Gong WJ, Peng JB, Yin JY, et al. Association between well-characterized lung cancer lncRNA polymorphisms and platinum-based chemotherapy toxicity in Chinese patients with lung cancer. Acta Pharmacol Sin 2017;38:581-90. [Crossref] [PubMed]
- Loewen G, Jayawickramarajah J, Zhuo Y, et al. Functions of lncRNA HOTAIR in lung cancer. J Hematol Oncol 2014;7:90. [Crossref] [PubMed]
- Chen D, Lu T, Tan J, et al. Long non-coding RNAs as communicators and mediators between the tumor microenvironment and cancer cells. Front Oncol 2019;9:739. [Crossref] [PubMed]
- Li X, Lei Y, Wu M, et al. Regulation of macrophage activation and polarization by HCC-derived exosomal lncRNA TUC339. Int J Mol Sci 2018;19:2958. [Crossref] [PubMed]
- Hirschberger S, Hinske LC, Kreth S. MiRNAs: dynamic regulators of immune cell functions in inflammation and cancer. Cancer Lett 2018;431:11-21. [Crossref] [PubMed]
- Salmena L, Poliseno L, Tay Y, et al. A ceRNA hypothesis: the Rosetta Stone of a hidden RNA language? Cell 2011;146:353-8. [Crossref] [PubMed]
- Zhu T, Yu Y, Liu J, et al. Identification of a competing endogenous RNA network related to immune signature in lung adenocarcinoma. Front Genet 2021;12:665555 [Crossref] [PubMed]
- Wei B, Kong W, Mou X, et al. Comprehensive analysis of tumor immune infiltration associated with endogenous competitive RNA networks in lung adenocarcinoma. Pathol Res Pract 2019;215:159-70. [Crossref] [PubMed]
- Bindea G, Mlecnik B, Tosolini M, et al. Spatiotemporal dynamics of intratumoral immune cells reveal the immune landscape in human cancer. Immunity 2013;39:782-95. [Crossref] [PubMed]
- Hackl H, Charoentong P, Finotello F, et al. Computational genomics tools for dissecting tumour-immune cell interactions. Nat Rev Genet 2016;17:441-58. [Crossref] [PubMed]
- Yoshihara K, Shahmoradgoli M, Martínez E, et al. Inferring tumour purity and stromal and immune cell admixture from expression data. Nat Commun 2013;4:2612. [Crossref] [PubMed]
- Ritchie ME, Phipson B, Wu D, et al. limma powers differential expression analyses for RNA-sequencing and microarray studies. Nucleic Acids Res 2015;43:e47 [Crossref] [PubMed]
- Langfelder P, Horvath S. WGCNA: an R package for weighted correlation network analysis. BMC Bioinformatics 2008;9:559. [Crossref] [PubMed]
- Szklarczyk D, Gable AL, Lyon D, et al. STRING v11: protein-protein association networks with increased coverage, supporting functional discovery in genome-wide experimental datasets. Nucleic Acids Res 2019;47:D607-13. [Crossref] [PubMed]
- Kohl M, Wiese S, Warscheid B. Cytoscape: software for visualization and analysis of biological networks. Methods Mol Biol 2011;696:291-303. [Crossref] [PubMed]
- Bader GD, Hogue CW. An automated method for finding molecular complexes in large protein interaction networks. BMC Bioinformatics 2003;4:2. [Crossref] [PubMed]
- Harris MA, Clark J, Ireland A, et al. The Gene Ontology (GO) database and informatics resource. Nucleic Acids Res 2004;32:D258-61. [Crossref] [PubMed]
- Kanehisa M, Goto S. KEGG: kyoto encyclopedia of genes and genomes. Nucleic Acids Res 2000;28:27-30. [Crossref] [PubMed]
- Agarwal V, Bell GW, Nam JW, et al. Predicting effective microRNA target sites in mammalian mRNAs. Elife 2015;4:e05005 [Crossref] [PubMed]
- Paraskevopoulou MD, Georgakilas G, Kostoulas N, et al. DIANA-microT web server v5.0: service integration into miRNA functional analysis workflows. Nucleic Acids Res 2013;41:W169-73 [Crossref] [PubMed]
- Jeggari A, Marks DS, Larsson E. miRcode: a map of putative microRNA target sites in the long non-coding transcriptome. Bioinformatics 2012;28:2062-3. [Crossref] [PubMed]
- Li T, Fan J, Wang B, et al. TIMER: A web server for comprehensive analysis of tumor-infiltrating immune cells. Cancer Res 2017;77:e108-10. [Crossref] [PubMed]
- Lee JM, Lee MH, Garon E, et al. Phase I trial of intratumoral injection of CCL21 gene-modified dendritic cells in lung cancer elicits tumor-specific immune responses and CD8+ T-cell infiltration. Clin Cancer Res 2017;23:4556-68. [Crossref] [PubMed]
- Hegde PS, Karanikas V, Evers S. The where, the when, and the how of immune monitoring for cancer immunotherapies in the era of checkpoint inhibition. Clin Cancer Res 2016;22:1865-74. [Crossref] [PubMed]
- Yang J, Qiu Q, Qian X, et al. Long noncoding RNA LCAT1 functions as a ceRNA to regulate RAC1 function by sponging miR-4715-5p in lung cancer. Mol Cancer 2019;18:171. [Crossref] [PubMed]
- Chen X, Wang Z, Tong F, et al. lncRNA UCA1 promotes gefitinib resistance as a ceRNA to target FOSL2 by sponging miR-143 in non-small cell lung cancer. Mol Ther Nucleic Acids 2020;19:643-53. [Crossref] [PubMed]
- Wang X, Lai Q, He J, et al. LncRNA SNHG6 promotes proliferation, invasion and migration in colorectal cancer cells by activating TGF-β/Smad signaling pathway via targeting UPF1 and inducing EMT via regulation of ZEB1. Int J Med Sci 2019;16:51-9. [Crossref] [PubMed]
- Jafari-Oliayi A, Asadi MH. SNHG6 is upregulated in primary breast cancers and promotes cell cycle progression in breast cancer-derived cell lines. Cell Oncol (Dordr) 2019;42:211-21. [Crossref] [PubMed]
- Zhao P, Deng Y, Wu Y, et al. Long noncoding RNA SNHG6 promotes carcinogenesis by enhancing YBX1-mediated translation of HIF1α in clear cell renal cell carcinoma. FASEB J 2021;35:e21160 [Crossref] [PubMed]
- Sun T, Li K, Zhu K, et al. SNHG6 interacted with miR-325-3p to regulate cisplatin resistance of gastric cancer by targeting GITR. Onco Targets Ther 2020;13:12181-93. [Crossref] [PubMed]
- Li M, Bian Z, Yao S, et al. Up-regulated expression of SNHG6 predicts poor prognosis in colorectal cancer. Pathol Res Pract 2018;214:784-9. [Crossref] [PubMed]
- Kowal-Bielecka O, Kowal K, Distler O, et al. Mechanisms of disease: leukotrienes and lipoxins in scleroderma lung disease--insights and potential therapeutic implications. Nat Clin Pract Rheumatol 2007;3:43-51. [Crossref] [PubMed]
- Barrett NA, Rahman OM, Fernandez JM, et al. Dectin-2 mediates Th2 immunity through the generation of cysteinyl leukotrienes. J Exp Med 2011;208:593-604. [Crossref] [PubMed]
- Woszczek G, Chen LY, Nagineni S, et al. Leukotriene D(4) induces gene expression in human monocytes through cysteinyl leukotriene type I receptor. J Allergy Clin Immunol 2008;121:215-21.e1. [Crossref] [PubMed]
- Deryusheva IV, Tsyganov M, Garbukov EY, et al. Genome-wide association study of loss of heterozygosity and metastasis-free survival in breast cancer patients. Exp Oncol 2017;39:145-50. [Crossref] [PubMed]
- Osman J, Savari S, Chandrashekar NK, et al. Cysteinyl leukotriene receptor 1 facilitates tumorigenesis in a mouse model of colitis-associated colon cancer. Oncotarget 2017;8:34773-86. [Crossref] [PubMed]
- He QL, Jiang HX, Zhang XL, et al. Relationship between a 7-mRNA signature of the pancreatic adenocarcinoma microenvironment and patient prognosis (a STROBE-compliant article). Medicine (Baltimore) 2020;99:e21287 [Crossref] [PubMed]
- Zhang Z, Qin H, Jiang B, et al. miR-30e-5p suppresses cell proliferation and migration in bladder cancer through regulating metadherin. J Cell Biochem 2019;120:15924-32. [Crossref] [PubMed]
- Ma YX, Zhang H, Li XH, et al. MiR-30e-5p inhibits proliferation and metastasis of nasopharyngeal carcinoma cells by target-ing USP22. Eur Rev Med Pharmacol Sci 2018;22:6342-9. [PubMed]
- Zhang S, Li G, Liu C, et al. miR-30e-5p represses angiogenesis and metastasis by directly targeting AEG-1 in squamous cell carcinoma of the head and neck. Cancer Sci 2020;111:356-68. [Crossref] [PubMed]
- Xu G, Cai J, Wang L, et al. MicroRNA-30e-5p suppresses non-small cell lung cancer tumorigenesis by regulating USP22-mediated Sirt1/JAK/STAT3 signaling. Exp Cell Res 2018;362:268-78. [Crossref] [PubMed]
- Mishra R, Bhattacharya S, Rawat BS, et al. MicroRNA-30e-5p has an integrated role in the regulation of the innate immune response during virus infection and systemic lupus erythematosus. iScience 2020;23:101322 [Crossref] [PubMed]
-
Mishra R Bhattacharya S Rawat BS Integrated role of microRNA-30e-5p through targeting negative regulators of innate immune pathways during HBV infection and SLE. 2020 . Available online: https://ssrn.com/abstract=354440910.2139/ssrn.3544409 - Huang XY, Zhang PF, Wei CY, et al. Circular RNA circMET drives immunosuppression and anti-PD1 therapy resistance in hepatocellular carcinoma via the miR-30-5p/snail/DPP4 axis. Mol Cancer 2020;19:92. [Crossref] [PubMed]
(English Language Editor: L. Huleatt)