Acoustic features as novel predictors of difficult laryngoscopy in orthognathic surgery: an observational study
Introduction
Airway risk assessment is an essential part of the preoperative anaesthetic protocol. As the chief aetiology of airway-related adverse events, difficult or failed airway management accounts for 25% of anaesthesia-related deaths (1). The definition of difficult laryngoscopy given by the American Society of Anesthesiologists (ASA) is that even with multiple attempts it is not possible to visualize any portion of the vocal cords during conventional laryngoscopy (2). The Cormack-Lehane grades describes how visible the vocal cords are during laryngoscopy, ranging from 1 (full view of vocal cords) to 4 (cannot see the epiglottis), which were used to classify difficult laryngoscopy (3). Poor view at laryngoscopy (Cormack and Lehane grade 3 to 4) were also a common definition of difficult intubation. Among all cases, the incidence of difficult laryngoscopy was 4.9% (4). However, this incidence rises considerably (to 15.4%) for patients undergoing oral and maxillofacial surgery (5). Orthognathic surgery is a common type of maxillofacial surgery in which anaesthesiologists frequently encounter difficult intubation due to retrognathia or a short mandible (6). Therefore, airway risk assessment is critical to identifying potential cases of difficult intubation before such procedures.
Numerous investigations have been conducted in an effort to predict the occurrence of difficult airway through physical examination (7-11), using tests including the modified Mallampati test (MMT), inter-incisor gap (IIG), thyromental distance (TMD), sternomental distance (SMD), upper lip bite test (ULBT), and composite scores. However, all the common clinical screening tests currently used have low sensitivity and specificity, and limited predictive value.
Voice formation is a complex physiological process involving interactions between the vocal cords, larynx, pharynx, tongue, epiglottis, palate, hyoid bone, mandible, teeth, and lips, all of which are important in endotracheal intubation (12). Any structural alterations to the vocal tract can lead to changes in the acoustic characteristics of the voice. Formant frequencies and bandwidths, the most frequently discussed voice features in the literature, describe the resonance of the vocal tract and are associated with the internal structures of the upper airway, including its compliance, shape, and dimensions (13). Considering the anatomical landmarks which are known to cause difficult intubation including limited opening of the mouth, bucked teeth, a receding chin, a large tongue and a narrow shape of palate (2), we hypothesized alterations on the corresponding structures may also affect acoustic parameters of patients with difficult intubation. However, there is dearth of studies investigating the potential role of acoustic features in evaluating a difficult airway. de Carvalho et al. reported that vowel formants exhibited a significant association with difficult laryngoscopy (14) and difficult facemask ventilation (15). Therefore, formant frequencies and bandwidths during phonation may provide anatomical and functional information about the superior airway and hold a promising role in the prediction of difficult airway.
In the present study, we set out to investigate whether changes in acoustic features can serve as a reliable measure for predicting the ease or difficulty of laryngoscopy during general anaesthesia in orthognathic surgery.
We present the following article in accordance with the STROBE reporting checklist (available at https://dx.doi.org/10.21037/atm-21-4359).
Methods
Patients
The study was conducted in accordance with the Declaration of Helsinki (as revised in 2013). This cross sectional study was approved by the Ethics Committee of Shanghai Ninth People’s Hospital (approval No.: SH9H-2020-T386-1) and is registered in ClinicalTrials.gov (trial registration No.: NCT 04458220). Between December 2020 and April 2021, 235 patients from Shanghai Ninth People’s Hospital were recruited into the study. Informed consent was obtained from each patient before their inclusion.
The inclusion criteria for the study were as follows: (I) candidates for orthognathic surgery under general anaesthesia; (II) ASA physical status class I–II; (III) aged 18 years and above; (IV) native Mandarin speakers. Participants with a previous history of speech disorders, vocal cord diseases, cleft palate, craniofacial syndromes, craniofacial trauma, previous oral and maxillofacial surgery, hearing or perception abnormalities, mental or central nervous system diseases, or patients participated in other relevant clinical investigation in the past 3 months were excluded from the study. Patients who underwent fibreoptic or video laryngoscopic intubation on the first attempt were excluded from the final analysis.
Preoperative airway assessment
During preoperative visits, data on the patients’ demographics and known bedside examination tests for difficult airway prediction were collected, including age, sex, body mass index (BMI), MMT, IIG, TMD, ULBT, horizontal length of the mandible (HLM), and cervical perimeter (CP). To prevent interobserver variability, all assessments were performed by the same anaesthesiologist.
The visibility of the oropharyngeal structures was assessed using the modified Mallampati test. The patient was asked to sit down and open their mouth as wide as possible, and then instructed to protrude their tongue without phonation. The results were classified as follows: class 1: soft palate, fauces, uvula, and pillars; class 2: soft palate, fauces, and uvula; class 3: soft palate and base of the uvula; and class 4, soft palate not visible at all (16). The IIG refers to the maximal distance between the upper and lower incisors.For the ULBT, the range of mandibular movement is assessed by asking patients to bite their upper lip with their lower incisors (10). The results of this test are presented in the form of the following 3-point grading system: class 1: the lower incisors extend beyond the vermilion border of the upper lip; class 2: the lower incisors can bite the lip but cannot be extended beyond the vermilion border; and class 3: the lower incisors cannot bite the upper lip at all. The TMD refers to the distance between the uppermost border of the thyroid cartilage and the mentum, and is measured with the neck extended and the mouth closed (11). The SMD refers to the distance between the upper border of the sternum and the tip of the jaw, and is measured with the neck fully extended (7). The HLM is measured from the angle of the mandible to the mentum (11). The CP was measured at the level of the cricothyroid membrane.
Acoustic data and analysis
Recording
All the participants were Chinese, so we choose the Chinese syllables as our recording samples, Chinese syllables are numerous and complex, both vowels and consonants were selected for experiments, which is expected to produce excellent results. All the participants were asked to phonate the Chinese vowels {[a], [o], [e], [i], [u], and [ü]}, consonants {[ci], [qi], [chi], [le], and [ke]}, and nasal vowel [en] (based on the anteroposterior position of the tongue and the location of air friction distributed along the vocal tract). All recordings were performed with the patient sat in a quiet room in comfortable conditions. The participants’ speech was recorded using a 16-bit handheld recorder with a sampling frequency of 44.1 kHz. The recorder was kept at a distance of 5 cm from the mouth for each recording. The duration of each sound and the interval between sounds lasted for approximately 1 second.
Acoustic feature abstraction
The speech samples were first identified and aligned using a Kaldi chain (17) model trained on the AISHELL-2 (18) dataset, which enabled the exact position of pinyin in the speech samples to be determined. Subsequently, clips were extracted from the audio recordings according to the location of the pinyin and imported into the Praat (19) software. The following features were extracted: the first formant (f1), the second formant (f2), the third formant (f3), the fourth formant (f4), and their bandwidths (bw1-bw4). For all the above features, the mean value along with the time of the clip where the pinyin was located was taken as the final result. This acoustic analysis was conducted by a specialist in speech technology who was blinded to the airway evaluation and Cormack-Lehane scores.
Induction of anaesthesia and tracheal intubation
General anaesthesia was administered according to the standard protocol. Patients were routinely monitored throughout the procedure using electrocardiography, oxygen saturation, non-invasive blood pressure measurement, and end-tidal carbon dioxide tension. After adequate pre-oxygenation, midazolam 0.05 mg·kg−1, fentanyl 2 to 4 μg·kg−1, propofol 2 to 2.5 mg·kg−1, and the neuromuscular blocker rocuronium 0.6 mg·kg−1 were administered to induce anaesthesia. After adequate relaxation had been achieved, tracheal intubation was performed by an anaesthesiologist with more than 3 years of experience using conventional direct laryngoscopy with a Macintosh blade number 3 or 4, and the Cormack-Lehane grade was evaluated (grade 1: full view of the glottis; grade 2: partial view of the glottis or arytenoids; grade 3: only the epiglottis visible, and grade 4: epiglottis not visible) with the patient’s head in the sniffing position. Direct laryngoscopy was defined as difficult based on a Cormack-Lehane score of 3–4.
Statistical analysis
Continuous variables with normal distribution were reported as mean ± standard deviation. Non-normal continuous variables were reported as median (interquartile range). Categorical variables were reported as frequency (percentage). The hypothesis was tested using one-way analysis of variance (ANOVA), the Mann-Whitney U test, and Fisher’s exact probability method. Univariate and multivariate logistic regression models were constructed using the general linear model in R 4.0.4. Covariables for multivariate regression included age (continuous variable), sex, and diagnosis. Stepwise logistic models were constructed with SPSS 24.0 (IBM, USA). The correlation between acoustic features and other clinical variables was explored by Spearman correlation analysis. Receiver operating characteristic (ROC) curves were drawn using the pROC package in R 4.0.4. The dataset was randomly split into the training and testing sets at a ratio of 8:2, and the random seed was 2021. The confidence interval (CI) of the area under the curve (AUC) was obtained by applying the bootstrap method.
Results
Baseline characteristics
A total of 235 patients initially met the criteria for inclusion in this cross sectional study. After the exclusion of those without acoustic data and those who could not be intubated by direct laryngoscopy, 225 patients were included in the final analysis (Figure 1). The baseline characteristics of the study participants are shown in Table 1. Of the 225 participants, 59 had a Cormack-Lehane grade of 3 or 4. According to whether they had difficult laryngoscopy, the differences of IIG, MMT, ULBT, TMD, SMD, HLM and the diagnosis between the two groups were statistically significant, while no difference were observed in age, sex, height, weight, BMI and CP.
Table 1
Variable | Overall (N=225) | Easy laryngoscopy (N=166) | Difficult laryngoscopy (N=59) | P |
---|---|---|---|---|
Age [mean (SD)] | 25.42 (4.50) | 25.07 (4.35) | 26.41 (4.81) | 0.050 |
Sex (%) | ||||
Female | 159 (70.7) | 118 (71.1) | 41 (69.5) | 0.868 |
Male | 66 (29.3) | 48 (28.9) | 18 (30.5) | |
Height [mean (SD)] | 1.67 (0.08) | 1.67 (0.09) | 1.66 (0.08) | 0.403 |
Weight [mean (SD)] | 58.84 (11.95) | 59.25 (12.29) | 57.67 (10.94) | 0.384 |
BMI [mean (SD)] | 20.96 (2.99) | 21.02 (2.95) | 20.81 (3.10) | 0.634 |
Diagnosis (%)† | <0.001 | |||
1 | 36 (16.0) | 29 (17.5) | 7 (11.9) | |
2 | 76 (33.8) | 35 (21.1) | 41 (69.5) | |
3 | 113 (50.2) | 102 (61.4) | 11 (18.6) | |
CL grade (%) | <0.001 | |||
1 | 55 (24.4) | 55 (33.1) | 0 (0.0) | |
2 | 111 (49.3) | 111 (66.9) | 0 (0.0) | |
3 | 51 (22.7) | 0 (0.0) | 51 (86.4) | |
4 | 8 (3.6) | 0 (0.0) | 8 (13.6) | |
MMT (%) | 0.003 | |||
1 | 90 (40.2) | 74 (44.6) | 16 (27.6) | |
2 | 53 (23.7) | 41 (24.7) | 12 (20.7) | |
3 | 65 (29.0) | 45 (27.1) | 20 (34.5) | |
4 | 16 (7.1) | 6 (3.6) | 10 (17.2) | |
ULBT (%) | <0.001 | |||
1 | 134 (59.8) | 114 (69.1) | 20 (33.9) | |
2 | 61 (27.2) | 38 (23.0) | 23 (39.0) | |
3 | 29 (12.9) | 13 (7.9) | 16 (27.1) | |
CP [mean (SD)] | 33.20 (3.33) | 33.22 (3.38) | 33.16 (3.20) | 0.905 |
IIP [mean (SD)] | 4.35 (0.78) | 4.49 (0.71) | 3.99 (0.85) | <0.001 |
TMD [mean (SD)] | 9.30 (1.42) | 9.60 (1.27) | 8.44 (1.49) | <0.001 |
SMD [mean (SD)] | 17.62 (2.08) | 18.04 (1.77) | 16.43 (2.41) | <0.001 |
HLM [mean (SD)] | 9.95 (1.51) | 10.16 (1.42) | 9.39 (1.63) | 0.001 |
†, diagnosis 1: lateral mandibular deviation; diagnosis 2: class II skeletal malocclusion; diagnosis 3: class III skeletal malocclusion. SD, standard deviation; BMI, body mass index; CL, Cormack-Lehane; MMT, modified Mallampati test; ULBT, upper lip bite test; CP, cervical perimeter; IIG; inter-incisor gap; TMD, thyromental distance; SMD, sternomental distance; HLM, horizontal length of the mandible.
Univariate and multivariate logistic regression
Univariate and multivariate logistic regression models were applied to evaluate the relationship between voice features and Cormack-Lehane grade. Univariate logistic regression analysis revealed 14 acoustic features to differ significantly between the difficult group and non-difficult group, with P values of <0.1 (Table 2). Also, after adjusting for sex (male, female), age (as a continuous variable), and diagnosis, the P values of en_f2, a_f1, and o_bw4 were all less than 0.05.
Table 2
Acoustic features | Unadjusted model | Adjusted model‡ | |||||
---|---|---|---|---|---|---|---|
OR | 95% CI | P | OR | 95% CI | P | ||
en_f2 | 0.996 | 0.994–0.998 | 0.001 | 0.999 | 0.994–0.999 | 0.011* | |
ci_bw4 | 0.997 | 0.994–0.999 | 0.010 | 1.000 | 0.995–1.000 | 0.103 | |
qi_bw4 | 0.997 | 0.994–0.999 | 0.015 | 1.001 | 0.995–1.001 | 0.174 | |
a_f1 | 0.997 | 0.995–1.000 | 0.031 | 1.000 | 0.993–1.000 | 0.029* | |
o_bw1 | 1.003 | 1.000–1.005 | 0.035 | 1.005 | 0.999–1.005 | 0.164 | |
le_f3 | 0.998 | 0.996–1.000 | 0.041 | 1.000 | 0.995–1.000 | 0.065 | |
o_bw4 | 1.001 | 1.000–1.002 | 0.056 | 1.003 | 1.000–1.003 | 0.032* | |
ü_f2 | 0.998 | 0.996–1.000 | 0.068 | 1.001 | 0.996–1.001 | 0.157 | |
chi_f4 | 1.002 | 1.000–1.004 | 0.068 | 1.004 | 0.999–1.004 | 0.316 | |
u_f2 | 0.999 | 0.997–1.000 | 0.079 | 1.001 | 0.997–1.001 | 0.226 | |
i_bw2 | 0.999 | 0.998–1.000 | 0.079 | 1.000 | 0.998–1.000 | 0.134 | |
ü_bw3 | 1.001 | 1.000–1.003 | 0.085 | 1.003 | 1.000–1.003 | 0.107 | |
a_bw4 | 0.999 | 0.998–1.000 | 0.086 | 1.000 | 0.998–1.000 | 0.119 | |
en_f4 | 0.998 | 0.996–1.000 | 0.088 | 1.001 | 0.996–1.001 | 0.178 |
‡, adjusted for sex, age, and diagnosis; *, P<0.05. OR, odds ratio; CI, confidence interval.
Correlation analysis
The results of correlation analysis between clinical predictors (IIG, TMD, SMD, CP, ULBT, HLM, ULBT, MMT) and the eight voice parameters which were used to construct the prediction model (Table 3) was visualized in Figure 2. The highest Spearman correlation coefficient was between CP and le_f3 (−0.37, P<0.01).
Table 3
Model | Acoustic features | OR | 95% CI | P |
---|---|---|---|---|
Forward stepwise model | en_f2 | 0.996 | 0.994–0.999 | 0.002 |
qi_bw4 | 0.996 | 0.993–0.999 | 0.003 | |
a_f1 | 0.997 | 0.995–1.000 | 0.038 | |
o_bw4 | 1.001 | 1.000–1.002 | 0.024 | |
Backward stepwise model | en_f2 | 0.996 | 0.994–0.999 | 0.006 |
ci_bw4 | 0.997 | 0.995–1.000 | 0.057 | |
qi_bw4 | 0.996 | 0.993–0.999 | 0.017 | |
le_f3 | 0.998 | 0.996–1.000 | 0.079 | |
o_bw4 | 1.001 | 1.000–1.003 | 0.014 | |
chi_f4 | 1.003 | 1.000–1.005 | 0.041 | |
a_bw4 | 0.999 | 0.998–1.000 | 0.078 |
OR, odds ratio; CI, confidence interval.
Establishment of the assessment model
We used stepwise logistic regression for the whole sample to further screen the voice characteristics with P<0.1 in the univariate logistic regression. Table 3 showed the eight voice features (en_f2, qi_bw4, a_f1, o_bw4, ci_bw4, le_f3, chi_f4, a_bw4) selected to construct a model for difficult airway evaluation. A receiver operating characteristic (ROC) curve was constructed to evaluate the performance of the model for the prediction of DL. The AUC of the forward stepwise model attained a value of 0.716 and 0.702 in the training set and test set, respectively (Figure 3). In the testing set, the model had a sensitivity and specificity of 80.0% and 64.3%, respectively (Table 4). The backward stepwise model attained an AUC value of 0.761 in the training set, but a value of only 0.709 in the test set (Figure 4). The highest point of the Youden index was designated as the threshold, which revealed the sensitivity and specificity of the model in the testing set to be 86.7% and 63.0%, respectively (Table 4). To prove the stability of the model, 100 random splits were performed and the AUC was calculated after 100 different random splits (with the CI obtained using the average of 100 times AUC). The mean value of the AUC was similar compared to that of our special set seed, which indicated that random splitting had little effect on our results.
Table 4
Variable | AUC (95% CI) | AUC100 (95% CI) | Accuracy (%) | Specificity (%) | Sensitivity (%) |
---|---|---|---|---|---|
Forward model | |||||
Training | 0.716 (0.627–0.805) | 0.723 (0.719–0.726) | 71.1 | 72.3 | 67.4 |
Testing | 0.702 (0.541–0.863) | 0.695 (0.679–0.711) | 69.8 | 64.3 | 80.0 |
Backward model | |||||
Training | 0.761 (0.678–0.844) | 0.761 (0.757–0.765) | 71.3 | 68.4 | 76.2 |
Testing | 0.709 (0.550–0.867) | 0.696 (0.679–0.712) | 71.4 | 63.0 | 86.7 |
AUC, area under the receiver operating characteristic curve; CI, confidence interval.
The performance of rational clinical examinations in the prediction of non-difficult or difficult laryngoscopy are shown in Table 5 and Figure 5. For the MMT model, the AUC value was 0.634, and the sensitivity and specificity were 51.7% and 69.3%, respectively. For the TMD model, the AUC value was 0.741, and the sensitivity and specificity were 76.3% and 66.9%, respectively.
Table 5
Variable | AUC (95% CI) | Accuracy (%) | Specificity (%) | Sensitivity (%) |
---|---|---|---|---|
MMT | 0.634 (0.552–0.717) | 64.7 | 69.3 | 51.7 |
ULBT | 0.691 (0.618–0.766) | 68.3 | 69.1 | 66.1 |
SMD | 0.708 (0.622–0.793) | 78.7 | 88.0 | 54.2 |
TMD | 0.741 (0.667–0.815) | 69.3 | 66.9 | 76.3 |
AUC, area under the receiver operating characteristic curve; CI, confidence interval; MMT, modified Mallampati test; ULBT, upper lip bite test; SMD, sternomental distance; TMD, thyromental distance.
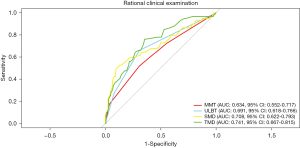
Discussion
To the best of our knowledge, our study is the first to determine the association between acoustic characteristics and patients with difficult laryngoscopy in Chinese population. This study found that patients with difficult laryngoscopy during orthognathic surgery have distinct phonetic characteristics, confirming that acoustic features can allow for accurate prediction of difficult laryngoscopy.
We found that Chinese vowels {[a] and [o]}, nasal vowel {[en]}, and consonants {[ci], [chi], [qi], and [le]} can make a significant contribution to the correct classification of the laryngoscopic view in orthognathic surgery. Studies have speculated that the pronunciation of the vowels [a], [o], and [en], which are uttered without obvious tongue movement (20), is closely related to the shape of the oral cavity, whereas the consonants interdentals [ci], post-alveolar [chi], alveolar [le], alveo-palatals [qi], velars [ke] are determined by the manner and site of articulation (21).
Our investigation of formant frequencies revealed that four formants (a_f1, en_f2, le_f3, and chi_f4) were significantly correlated with the laryngoscopic view. The f1 formant is related to tongue height and the mandibular opening; f2 is correlated with forward and backward movements of the tongue during speech production; f3 is correlated with the anterior region of the vocal tract (i.e., the space between the tongue and the mandibular incisors); and f4 is likely related to the length of the laryngeal tube (22), which provides a plausible rationale for our results.
Aside from formant frequency, formant bandwidth is the other important acoustic characteristic of speech (23). Bandwidth is inversely related to amplitude: the wider the bandwidth, the smaller the amplitude, and the lower the energy of the formant. Interestingly, only bw4 (a_bw4, o_bw4, qi_bw4, and ci_bw4) was correlated with the laryngoscopic view in this study.
It is known that acoustic features are intimately related to the size and configuration of the vocal tract (12,13). In the correlation analysis in our study, the neck circumference and the length of the mandible were shown relationship to formant frequencies and bandwidths for the evaluated phonemes. The association can be explained as follows: both the neck circumference and the length of the mandible play an important role in the length, internal diameter, and internal area of the vocal tract, which affect voice production and resonance and contribute to abnormal voice features (22).
We used the MMT, ULBT, TMD, and SMD to evaluate the airway risk of patients. Similar to other studies, we found that none of the rational clinical examinations had high sensitivity or specificity, which suggests that a single factor is unlikely to be successful in predicting a difficult airway (8). However, the predictive model including acoustic features performed better than the Mallampati classification. Some studies have proposed that analysis of voice parameters reflects not only the morphology but also the functional traits of the airway, which may explain its efficacy.
Our study differs from previous studies that used voice analysis for airway evaluation (14). First, our study sample comprised patients with lateral mandibular deviation and class II and class III skeletal abnormalities. The shape of the oral cavity, movement of the tongue and length of the mandible are known to vary between the different types of malocclusion, and these structural differences may affect patients’ voices (24,25). Previous studies have reported that individuals with class III malocclusions often encounter difficulty in producing labiodental and lingual-alveolar consonants (26). Therefore, we adjusted for diagnosis in the multivariate regression. Table 2 shows that after minimisation of the possible effect of diagnosis, a significant difference only remained for a_f1, o_bw4, and en_f2, but the differences for the consonants ci_bw4, qi_bw4, and le_f3 disappears. Notably, only a_f1, o_bw4, and en_f2 were correlated with difficult laryngoscopy. Furthermore, to eliminate the effect of additional confounding factors, patients with a history of cleft palate, craniofacial syndromes, or craniofacial trauma were excluded from our study, as well as those who had previously undergone oral and maxillofacial surgeries. Second, different speech features have been reported to be correlated with age, sex, height, and weight, and have also been linked to anatomical and physiological changes in the speech production system (27-29). Most patients in our study were young and healthy adults who wished to improve their facial appearance via orthognathic surgery. The mean age of the study population was 25.4 years, which is substantially lower than the mean age of 44.4 years in Carvalho et al.’s study. Also, none of our patients had a BMI above 30. The differences in our findings may be attributable to the above-mentioned differences in baseline characteristics, which can influence voice production and articulation. Finally, the speech samples used in our study consisted of Chinese syllables, hence, differences in pronunciation between languages may have also influenced the results.
Limitations
Our study has several limitations. One limitation of the study is the relatively small sample size. Larger patient populations are needed to explore the further applications of acoustic parameters in patients with difficult intubation. Moreover, our study was limited to Chinese participants undergoing orthognathic surgery, which may limit the generalisability of our results to the global population. Furthermore, considering the limited accuracy of the measurement of formant frequencies and bandwidths, future work should combine acoustic parameters and speech technology, such as speaker recognition, to enhance the effectiveness of the predictive model.
In summary, the use of acoustic parameters may be a feasible method for predicting difficult laryngoscopy in patients undergoing orthognathic surgery. Compared to other assess methods, acoustic features-based methods are very attractive as they are nonintrusive and provide objective information automatically, only requiring a few minutes to evaluate several voice parameters, which could even be recorded just before the interview with a physician. Thus could have an promising role in facilitating intelligent and telematic airway evaluation. Future studies based on a large and diverse population are essential to fully realize the applicability of acoustic model in the assessment of difficult airway.
Acknowledgments
Funding: This study was supported by the Interdisciplinary Program of Shanghai Jiao Tong University (No. ZH2018ZDA14), the Science & Technology Innovation fund of Shanghai Ninth People’s Hospital, Shanghai Jiao Tong University School of Medicine (No. CK2019008) and the Health Industry Clinical Research Specialized Project of Shanghai Municipal Health Commission (No. 202140410).
Footnote
Reporting Checklist: The authors have completed the STROBE reporting checklist. Available at https://dx.doi.org/10.21037/atm-21-4359
Data Sharing Statement: Available at https://dx.doi.org/10.21037/atm-21-4359
Conflicts of Interest: All authors have completed the ICMJE uniform disclosure form (available at https://dx.doi.org/10.21037/atm-21-4359). The authors have no conflicts of interest to declare.
Ethical Statement: The authors are accountable for all aspects of the work in ensuring that questions related to the accuracy or integrity of any part of the work are appropriately investigated and resolved. The study was conducted in accordance with the Declaration of Helsinki (as revised in 2013). This cross sectional study was approved by the Ethics Committee of Shanghai Ninth People’s Hospital (approval No.: SH9H-2020-T386-1). Informed consent was obtained from each patient before their inclusion.
Open Access Statement: This is an Open Access article distributed in accordance with the Creative Commons Attribution-NonCommercial-NoDerivs 4.0 International License (CC BY-NC-ND 4.0), which permits the non-commercial replication and distribution of the article with the strict proviso that no changes or edits are made and the original work is properly cited (including links to both the formal publication through the relevant DOI and the license). See: https://creativecommons.org/licenses/by-nc-nd/4.0/.
References
- Cook TM, Woodall N, Frerk C. Major complications of airway management in the UK: results of the Fourth National Audit Project of the Royal College of Anaesthetists and the Difficult Airway Society. Part 1: anaesthesia. Br J Anaesth 2011;106:617-31. [Crossref] [PubMed]
- Apfelbaum JL, Hagberg CA, Caplan RA, et al. Practice guidelines for management of the difficult airway: an updated report by the American Society of Anesthesiologists Task Force on Management of the Difficult Airway. Anesthesiology 2013;118:251-70. [Crossref] [PubMed]
- Cormack RS, Lehane J. Difficult tracheal intubation in obstetrics. Anaesthesia 1984;39:1105-11. [Crossref] [PubMed]
- Heinrich S, Birkholz T, Irouschek A, et al. Incidences and predictors of difficult laryngoscopy in adult patients undergoing general anesthesia: a single-center analysis of 102,305 cases. J Anesth 2013;27:815-21. [Crossref] [PubMed]
- Tuzuner-Oncul AM, Kucukyavuz Z. Prevalence and prediction of difficult intubation in maxillofacial surgery patients. J Oral Maxillofac Surg 2008;66:1652-8. [Crossref] [PubMed]
- Rodrigo C. Anesthetic considerations for orthognathic surgery with evaluation of difficult intubation and technique for hypotensive anesthesia. Anesth Prog 2000;47:151-6. [PubMed]
- Detsky ME, Jivraj N, Adhikari NK, et al. Will This Patient Be Difficult to Intubate?: The Rational Clinical Examination Systematic Review. Jama 2019;321:493-503. [Crossref] [PubMed]
- Roth D, Pace NL, Lee A, et al. Airway physical examination tests for detection of difficult airway management in apparently normal adult patients. Cochrane Database Syst Rev 2018;5:Cd008874 [Crossref] [PubMed]
- Naguib M, Scamman FL, O'Sullivan C, et al. Predictive performance of three multivariate difficult tracheal intubation models: a double-blind, case-controlled study. Anesth Analg 2006;102:818-24. [Crossref] [PubMed]
- Khan ZH, Kashfi A, Ebrahimkhani E. A comparison of the upper lip bite test (a simple new technique) with modified Mallampati classification in predicting difficulty in endotracheal intubation: a prospective blinded study. Anesth Analg 2003;96:595-9. table of contents. [Crossref] [PubMed]
- Shobha D, Adiga M, Rani DD, et al. Comparison of Upper Lip Bite Test and Ratio of Height to Thyromental Distance with Other Airway Assessment Tests for Predicting Difficult Endotracheal Intubation. Anesth Essays Res 2018;12:124-9. [Crossref] [PubMed]
- Sataloff RT, Heman-Ackah YD, Hawkshaw MJ. Clinical anatomy and physiology of the voice. Otolaryngol Clin North Am 2007;40:909-29. v. [Crossref] [PubMed]
- Montero Benavides A, Blanco Murillo JL, Fernández Pozo R, et al. Formant Frequencies and Bandwidths in Relation to Clinical Variables in an Obstructive Sleep Apnea Population. J Voice 2016;30:21-9. [Crossref] [PubMed]
- de Carvalho CC, da Silva DM, de Carvalho AD Junior, et al. Pre-operative voice evaluation as a hypothetical predictor of difficult laryngoscopy. Anaesthesia 2019;74:1147-52. [Crossref] [PubMed]
- de Carvalho CC, da Silva DM, de Carvalho AD Jr, et al. Evaluation of the association between voice formants and difficult facemask ventilation. Eur J Anaesthesiol 2019;36:972-3. [Crossref] [PubMed]
- Samsoon GL, Young JR. Difficult tracheal intubation: a retrospective study. Anaesthesia 1987;42:487-90. [Crossref] [PubMed]
- Povey D, Ghoshal A, Boulianne G, et al. The Kaldi Speech Recognition Toolkit. 2011.
- Du J, Na X, Liu X, et al. Aishell-2: Transforming mandarin asr research into industrial scale. arXiv preprint arXiv:1808.10583, 2018.
- Boersma P Weenink D. Praat. Amsterdam: University of Amsterdam Praat: doing phonetics by computer. 2017. http://www.praat.org/
- Arnela M, Dabbaghchian S, Blandin R, et al. Influence of vocal tract geometry simplifications on the numerical simulation of vowel sounds. J Acoust Soc Am 2016;140:1707. [Crossref] [PubMed]
- Ghaemi H, Khoddami SM, Soleymani Z, et al. The Vocal Fold Dysfunction Questionnaire: Validity and Reliability of the Persian Version. J Voice 2018;32:710-4. [Crossref] [PubMed]
- Macari AT, Karam IA, Tabri D, et al. Formants frequency and dispersion in relation to the length and projection of the upper and lower jaws. J Voice 2015;29:83-90. [Crossref] [PubMed]
- Kent RD, Vorperian HK. Static measurements of vowel formant frequencies and bandwidths: A review. J Commun Disord 2018;74:74-97. [Crossref] [PubMed]
- de Almeida Prado DG, Filho HN, Berretin-Felix G, et al. Speech Articulatory Characteristics of Individuals With Dentofacial Deformity. J Craniofac Surg 2015;26:1835-9. [Crossref] [PubMed]
- Viegas F, Viegas D, Serra Guimarães G, et al. Acoustic Analysis of Voice and Speech in Men with Skeletal Class III Malocclusion: A Pilot Study. Folia Phoniatr Logop 2021;73:117-25. [PubMed]
- Ghaemi H, Emrani E, Labafchi A, et al. The Effect of Bimaxillary Orthognathic Surgery on Nasalance, Articulation Errors, and Speech Intelligibility in Skeletal Class III Deformity Patients. World J Plast Surg 2021;10:8-14. [Crossref] [PubMed]
- Torre P 3rd, Barlow JA. Age-related changes in acoustic characteristics of adult speech. J Commun Disord 2009;42:324-33. [Crossref] [PubMed]
- Takaki PB, Vieira MM, Said AV, et al. Does Body Mass Index Interfere in the Formation of Speech Formants? Int Arch Otorhinolaryngol 2018;22:45-9. [Crossref] [PubMed]
- Evans S, Neave N, Wakelin D. Relationships between vocal characteristics and body size and shape in human males: an evolutionary explanation for a deep male voice. Biol Psychol 2006;72:160-3. [Crossref] [PubMed]
(English Language Editor: J. Reynolds)