Analysis of whole-exome data of cfDNA and the tumor tissue of non-small cell lung cancer
Introduction
Lung cancer (LC) is a malignant tumor with high mortality, subdivided into non-small cell LC (NSCLC, accounting for ~85% of the total number of lung cancers) and small cell LC (SCLC, accounting for ~15% of the total number of lung cancers). NSCLC is further divided into adenocarcinoma and squamous cell carcinoma, which can be molecularly stratified according to specific gene mutations and their expression on the tumor (1). If NSCLC can be detected early and surgically removed, the prognosis can be good, with a 5-year survival rate of 70–90% (2). However, most patients (~75%) are already at an advanced stage when diagnosed (3), and although in recent years there have been significant advances in treating patients with advanced lung cancer, the survival rate remains low. Currently, some NSCLC patients are given targeted therapy, but the biggest obstacle is the inevitable drug resistance, arising through tumor cells using different mechanisms to resist the drugs, including target gene mutations and activation of complementary bypass pathways, phenotypic transformation, etc. (4,5). Tumor cells will produce different subclones in their development and evolution, which leads to tumor heterogeneity (6-8).
Both the diagnosis and postoperative monitoring of LC are important for the patient’s prognosis, but it is still difficult to diagnose and monitor the development of LC in the early stage, because often there are no obvious symptoms. Although low-dose computed tomography (LDCT) is the LC screening and detection method widely recommended, it has radiation risks. Therefore, a noninvasive screening tool that can be used to detect LC earlier is desired.
Circulating cell-free DNA (cfDNA) refers to small double-stranded DNA fragments released from normal or tumor cells into the peripheral blood or other body fluids (9). In patients with a tumor the cfDNA level will increase notably, and tumor-specific mutations derived from cancer cells can be identified (10). Compared with traditional tissue sampling, blood cfDNA sampling is faster, more convenient, easier to operate, minimally invasive, and inexpensive (11). As a feasible tissue biopsy method, cfDNA liquid biopsy analysis has been used for molecular target identification, response and prognosis prediction, and drug resistance monitoring in targeted therapy for LC. Therefore, it can also be used to dynamically monitor the treatment and prognosis of patients.
Intratumor heterogeneity refers to the subclonal diversity of tumor cells observed in a single tumor, whereas intertumor heterogeneity refers to the diversity between the primary and secondary tumors (12-14). Tumor heterogeneity not only manifests within and between tumors, between primary tumors and secondary tumors, but also between different tumor cells in the same tumor tissue. Different cell populations and cells at different stages of one tumor have many different characteristics, such as gene mutation information, gene expression information, and epigenetic information (15). Tumor heterogeneity can lead to a high degree of complexity and genetic diversity within tumor tissues, causing different treatment sensitivities for the same tumor type (16). The heterogeneity of tumors between patients is related to individual genetic and phenotypic variation, which can explain the different treatment responses of each patient.
The development of high-throughput sequencing technology provides a good method for studying tumor heterogeneity and its development. Due to its high throughput and high accuracy, the second-generation sequencing technology can accurately analyze changes in both tumor gene mutations and expression. The third-generation sequencing technology can more conveniently and accurately detect structural variations at the genomic and transcriptome level due to its long-read sequencing (17). The development of single-cell sequencing technology and spatial transcriptome technology has deepened the ability to investigate the spatial heterogeneity of tumors (18-20).
At present, there is no effective method for dynamic monitoring of NSCLC. In this study, we aimed to provide ideas and methods for real-time monitoring of tumors. Tumor and para-cancer tissue samples, and peripheral blood samples of NSCLC patients were collected and performed whole-exome sequencing. The driver genes in the tumors of NSCLC patients were analyzed by sequencing analysis, and the tumor subclones at different time points were compared and analyzed. After systematic analysis, the gene mutation maps in the tumor tissues and the levels of cfDNA of the NSCLC patients were plotted.
We present the following article in accordance with the MDAR reporting checklist (available at https://dx.doi.org/10.21037/atm-21-4117).
Methods
Clinical data collection
From January 2016 to December 2018, 87 patients with NSCLC were enrolled in the Zhujiang Hospital of Southern Medical University as research subjects. All patients were diagnosed by pathological examination, and the para-cancer tissues were confirmed to be free of cancer cells. Table 1 shows the backgrounds and clinicopathological characteristics of the patients. All procedures performed in this study involving human participants were in accordance with the Declaration of Helsinki (as revised in 2013). All patients signed an informed consent form, and the study protocol was approved by the Ethics Committee of the Zhujiang Hospital of Southern Medical University.
Table 1
Characteristic | Concordance patients (n=87) (%) |
---|---|
Age (years) | |
Median (min., max.) | 60 (32, 79) |
<65 | 64 (73.6) |
≥65 | 23 (26.4) |
Gender | |
Male | 50 (57.5) |
Female | 37 (42.5) |
Smoking status | |
Current smoker | 15 (17.2) |
Former smoker | 3 (3.4) |
Never smoker | 69 (79.3) |
Histologic subtype | |
Adenocarcinoma | 75 (86.2) |
Squamous carcinoma | 4 (4.6) |
Other | 8 (9.2) |
Clinical UICC stage before treatment | |
I–II | 12 (13.8) |
III–IV | 75 (86.2) |
Residence area | |
Rural | 33 (37.9) |
Urban | 54 (62.1) |
CEA level (μg/L) | |
≤5.0 | 26 (29.9) |
>5.0 | 61 (70.1) |
Respond to treatment | |
CR | 5 (5.7) |
PR | 4 (4.6) |
SD | 41 (47.1) |
PD | 37 (42.5) |
UICC, Union for International Cancer Control; CEA, carcinoembryonic antigen; CR, complete response; PR, partial response; SD, stable disease; PD, disease progression.
Whole-exome sequencing
We performed whole-exome sequencing (WES) on 47 tumor tissue samples, and on the cfDNA extracted from 36 peripheral blood samples, and 4 patients were performed on both tissue samples and cfDNA samples. We randomly assigned 5 patients to dynamic WES to monitor tumor changes. Novaseq6000 was the sequencing platform, and the exon region was captured by the Agilent SureSelect Human All Exon V6 kit.
Statistical analysis
Quality control and analysis of sequencing data
First, fastp (21) software was used to control the quality of the original sequencing data. Adapters and low-quality bases were removed, then bwa (22) software was used to compare the filtered data with the human reference genome (hg38). Next, GATK (23) software was applied to find the mutation sites of each sample to annotate and filter, and finally, a reliable map related to tumor tissue and cfDNA gene mutations was obtained.
Analysis of purity and ploidy of tumor samples
ABSOLUTE software (https://software.broadinstitute.org/cancer/cga/absolute) was used to analyze the purity and ploidy of each tumor sample following the default parameters, and then the samples with lower tumor purity were removed.
Driver genes prediction
MutSigCV (24) software was used to analyze all the tumor mutation information to predict tumor driver genes.
Results
Quality of sequencing data
In the samples we obtained, samples with a high sequencing repetition rate or with a tumor sample depth of less than 100X were removed, so a total of 174 WES data sets were examined. Of them, the 91 tumor samples (we got both tissue and cfDNA samples in 4 patients) had an average sequencing depth of 406X (103X–812X), 51 tissue samples had an average sequencing depth of 417X (140X–703X), and 40 cfDNA samples had an average depth of 392X (103X–821X).
Consistence of the sequencing data with samples
After analysis and judgment, all data of tumor samples and control samples were consistent with the corresponding patients, and the sexes of the sequencing data were consistent with the corresponding patients as well. No sample confusion events had occurred.
General consistency of the tumor purity and the number of ploidy of tumor tissue with cfDNA
The tumor purity and ploidy analyses were performed on the WES results for the cfDNA and tumor tissue samples, and found to be about the same (Figure 1).
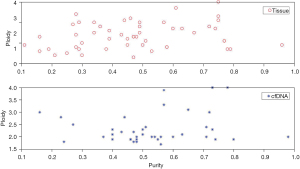
Higher frequency of somatic mutations of TTN, EGFR, and TP53 genes
After analyzing the results of filtered gene mutations, the tumor tissue or cfDNA samples had the largest number of missense mutations compared with the para-cancer tissues, and most of them were single nucleotide polymorphisms (SNP), in which the base C mutated to base A or T accounted for the highest percentage (Figure 2A-2C). The median number of mutations in all samples was 79, and among all genes with mutations, we found that TTN, EGFR, and TP53 had the highest mutation frequency, with an occurrence rate >30% (Figure 2D-2F). Subsequently, we calculated the distribution of genes with higher mutation frequency in each sample (Figure 3).
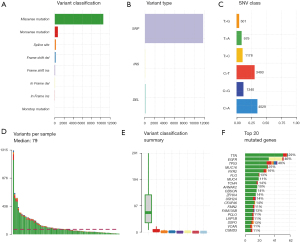
Number of converted versus inverted bases in the mutated samples
We analyzed the SNP changes of all samples and found that among the SNPs of all samples, the number of cases of cytosine mutated to thymine (C>T) was the largest, accounting for about 48% of the total SNPs, followed by cytosine mutated to guanine (C>A, 23%), and thymine mutated to cytosine (T>C, 13%) (Figure 4A). In addition, we counted the number of bases that underwent conversion (Ti) and inversion (Tv), and found that conversion (~55% of the total) was notably more than inversion (~40% of the total) (Figure 4B). The proportion of specific mutation types in each sample is shown in Figure 4C.
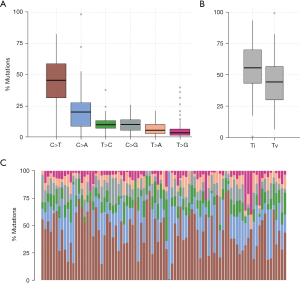
Main relationship between significant mutation signal of the sample and smoking
Maftools (25) software was used to extract mutation features from our measured data, and then the mutation features we extracted, based on the 30 verified mutation features in COMISC, were annotated. We found 4 distinctly enriched mutation signals, which were mutation feature 2, mutation feature 4, mutation feature 5, and mutation feature 6 (Figure 5), respectively. Among them, mutation feature 4 was related to smoking, and its feature was similar to the mutation pattern observed in the experimental system exposed to tobacco carcinogens, so it may be caused by tobacco mutagens, which indicated that there might be tumors caused by smoking among the patient samples we collected.
Sample prediction of TP53, EGFR, FOLR3, LCN10, SPPL2B, STK11, and KRAS genes
MutSigCV (24) software was applied to predict the tumor genes based on the analysis results of all nonsense mutations. In the end, we found that TP53, EGFR, FOLR3, LCN10, SPPL2B, STK11, and KRAS were the tumor driver genes of our tested samples (Table 2). Among them, TP53, EGFR, and KRAS genes have already been predicted as driver genes in a variety of tumors. Mutations of these genes will seriously affect the progression of tumors and the effects of treatment and prognosis.
Table 2
Gene | expr | reptime | hic | N_nonsilent | N_silent | nnei | x | X | p | q |
---|---|---|---|---|---|---|---|---|---|---|
|
2069567 | 213 | 34 | 281,099 | 77,623 | 29 | 13 | 2,618,798 | 0 | 0 |
|
423489 | 336 | −13 | 863,681 | 228,046 | 4 | 8 | 521,885 | 0 | 0 |
|
715293 | 267 | 62 | 165,347 | 40,495 | 50 | 10 | 4,625,075 | 0 | 0 |
|
1898901 | 190 | 39 | 131,768 | 38,857 | 38 | 22 | 4,469,283 | 0 | 0 |
|
1503606 | 267 | 31 | 387,751 | 120,302 | 50 | 16 | 5,227,040 | 0 | 0.0086 |
|
2214581 | 234 | 29 | 286,286 | 78,988 | 26 | 9 | 2,936,934 | 0 | 0.0264 |
|
259193 | 512 | 16 | 155,974 | 37,583 | 50 | 20 | 5,245,240 | 0 | 0.0734 |
nnei: number of adjacent genes; x: number of silent or non-coding mutant bases in adjacent genes; X: total number of bases related to adjacent genes; p: significant P values; q: the corrected P value.
High similarity between cfDNA and tissue samples for secondary clones
Studies have shown that genomic instability promotes the emergence of more competitive subclones, which is the main factor in tumor progression and metastasis and drug resistance during treatment. Before treating LC, there will be drug-resistant clones. After tyrosine kinase inhibitor (TKI) treatment, sensitive clones (primary clones) will be reduced, but drug-resistant clones (secondary clones) gradually become dominant and tumor heterogeneity appears (26). Among the 91 samples from 87 patients we obtained, 5 patients were sampled at different time points to evaluate the significance of WES in monitoring tumor progression (Table 3). After sequencing these samples, we analyzed the subclonal structures of the tumor DNA and cfDNA in the blood at different times, as well as the tumor heterogeneity. The results showed that for the secondary clones, using cfDNA to detect changes in the patient’s tumor subclones was highly consistent with the clonal analysis of the tissue samples (Figure 6).
Table 3
Patient No. | Sample ID | Type | Cellularity | Ploidy estimate |
---|---|---|---|---|
1 | ZY1711073842943000 | Tissue | 0.61 | 3.7 |
ZY1803020787902000 | cfDNA | 0.65 | 3.1 | |
2 | ZY1711073821432000 | cfDNA | 0.8 | 1.9 |
ZY1711076467752000 | cfDNA | 0.43 | 2.2 | |
ZY1711070438002000 | cfDNA | 0.57 | 2.3 | |
ZY1803029704273000 | Tissue | 0.75 | 4 | |
3 | ZY0755443364264940 | Tissue | 0.6 | 2.7 |
ZY0755443364264940-ct | cfDNA | 0.63 | 2.1 | |
4 | ZY1803026037353000-T | Tissue | 0.47 | 2.3 |
ZY1711079369192000 | cfDNA | 0.54 | 2 | |
5 | ZY0755342713044877 | cfDNA | 0.6 | 2 |
ZY1711079244802000 | cfDNA | 0.53 | 2 | |
ZY1803027967203000 | Tissue | 0.38 | 1.7 |
There was a total of 7 subclones in the tumor of patient 1, consisting of 4 primary clones (n>5) (cluster0, cluster1, cluster2, cluster3), and 3 secondary clones (n≤5) (cluster4, cluster5, cluster6). The second subclone (cluster1) was unique to the tissue samples, and the third subclone (cluster2) was unique to the blood samples. The other 2 primary clones and the 3 secondary clones were detected in both the blood and tissue samples, and the effective clone detection rate (the percentage and sensitivity of clones shared in blood and tissue to the detected clones in tissue) was 83.3%. The effective detection rate of secondary clones was 100%, and the false-positive rate (the percentage of specific detection in blood to all clones detected in tissues and blood samples) was 14.3%.
There was a total of 10 subclones in the tumor of patient 2, consisting of 3 primary clones (n>5) (cluster0, cluster1, cluster3), and 7 secondary clones (n≤5) (cluster2, cluster4–cluster9). The second and sixth (cluster1, cluster5) subcloned tissues were not detected. The effective detection rate of all clones was 100%, the effective detection rate of secondary clones was 100%, and false-positive rate was 20%.
There were 6 subclones in patient 3, with 4 primary clones (n>5) (cluster0, cluster1, cluster2, cluster3), and 2 secondary clones (n≤5) (cluster4, cluster5). The unique subclonal type in the tissues was the second subclonal type (cluster1), and the unique subclonal type in the blood samples was the third subclonal type (cluster2). The effective detection rate of all clones was 80%, the effective detection rate of secondary clones was 100%, and false-positive rate was 17%.
There were 6 subclones in patient 4, with 3 primary clones (n>5) (cluster0, cluster1, cluster2), and 3 secondary clones (n≤5) (cluster3, cluster4, cluster5). The first subclone (cluster1) was a unique type of subclone in the tissue, and the second subclone (cluster2) was a unique feature of blood. The effective detection rate of all clones was 80%, the effective detection rate of secondary clones was 100%, and false-positive rate was 17%.
There were 7 subclones in patient 5, with 5 primary clones (n>5) (cluster0, cluster2, cluster3, cluster4, cluster5), and 2 secondary clones (n≤5) (cluster1, cluster6). The second subclonal type (cluster1) was a unique tumor feature in the tissue sample. The effective detection rate of all clones (was 85.7%, the effective detection rate of secondary clones was 100%, and false-positive rate was 0%.
Discussion
In general, the occurrence of tumors is the result of mutations in one or more genes (27-29), and NSCLC is one of the tumors with a high incidence. A mutation map of the patient’s tumor and the discovery of driver genes can provide effective guidance for treatment (30). WES is an advanced technique and method used for studying the gene mutation of tumors. Through analyzing the WES data of tumor samples, it is possible to accurately obtain the mutation gene map of the tumor cells and discover the tumor driver genes. Precisely detecting tumor somatic mutations and driver genes is critical for treatment and prognosis (31-33). Several studies have identified the main driver genes of a variety of tumors (34), and these results provide essential information for their clinical treatment.
Tumorigenesis is a dynamic process (6). Tumors at the same site in a patient will have higher heterogeneity at different periods (35). The subclones generated during the evolution of tumors lead to extremely high complexity and genetic diversity, which leads to inconsistent tumor sensitivity to treatment, resulting in poor treatment efficacy. Therefore, it is of great significance for the treatment to monitor tumors in real-time. Because the cfDNA of tumor patients contains a large amount of DNA released from the tumor, and the sampling for cfDNA is simple and noninvasive, the dynamic monitoring of tumor changes through cfDNA detection has potential for real-time tumor monitoring (36,37). Studies have shown that (38), in patients with NSCLC, cfDNA is more suitable as a monitoring index than carcinoembryonic antigen (CEA) and neuron specific enolase (NSE), making it a biomarker with significant advantages in lung cancer diagnosis, tumor efficacy and prognosis.
In this study, we simultaneously performed WES on the tumor, para-cancer tissues, and cfDNA of NSCLC patients, as well as systematically analyzing the gene mutation map, and tumor subclones at different time points. We created a gene mutation map of NSCLC and predicted TP53, EGFR, FOLR3, LCN10, SPPL2B, STK11, and KRAS as the driver genes. The false-positive rate of all tumor subclones analyzed by cfDNA was <20%, the sensitivity was >80%, and the effective detection rate of secondary clones was 100%, which were highly similar to the results for the tissue samples; thus, WES can represent the tumor status, providing a feasible method for real-time monitoring of tumors.
The limitation of this study is that the small sample size. There were only 5 patients used to compare the WES results for the cfDNA and tumor tissue samples, and the effective number of samples was only 91, so there may be sampling bias, as well as random deviations in the statistical results. However, even with the limited number of samples, we still found that it is feasible for cfDNA analysis to replace tumor tissue samples in patients with advanced LC (≥ stage III) for real-time monitoring of tumor differentiation.
Acknowledgments
Funding: Natural Fund of Guangdong Province (No. 2019A1515011871).
Footnote
Reporting Checklist: The authors have completed the MDAR reporting checklist. Available at https://dx.doi.org/10.21037/atm-21-4117
Data Sharing Statement: Available at https://dx.doi.org/10.21037/atm-21-4117
Conflicts of Interest: All authors have completed the ICMJE uniform disclosure form (available at https://dx.doi.org/10.21037/atm-21-4117). The authors have no conflicts of interest to declare.
Ethical Statement: The authors are accountable for all aspects of the work in ensuring that questions related to the accuracy or integrity of any part of the work are appropriately investigated and resolved. All procedures performed in this study involving human participants were in accordance with the Declaration of Helsinki (as revised in 2013). All patients signed an informed consent form, and the study protocol was approved by the Ethics Committee of the Zhujiang Hospital of Southern Medical University.
Open Access Statement: This is an Open Access article distributed in accordance with the Creative Commons Attribution-NonCommercial-NoDerivs 4.0 International License (CC BY-NC-ND 4.0), which permits the non-commercial replication and distribution of the article with the strict proviso that no changes or edits are made and the original work is properly cited (including links to both the formal publication through the relevant DOI and the license). See: https://creativecommons.org/licenses/by-nc-nd/4.0/.
References
- Herbst RS, Heymach JV, Lippman SM. Lung cancer. N Engl J Med 2008;359:1367-80. [Crossref] [PubMed]
- Goldstraw P, Chansky K, Crowley J, et al. The IASLC Lung Cancer Staging Project: Proposals for Revision of the TNM Stage Groupings in the Forthcoming (Eighth) Edition of the TNM Classification for Lung Cancer. J Thorac Oncol 2016;11:39-51. [Crossref] [PubMed]
- Walters S, Maringe C, Coleman MP, et al. Lung cancer survival and stage at diagnosis in Australia, Canada, Denmark, Norway, Sweden and the UK: a population-based study, 2004-2007. Thorax 2013;68:551-64. [Crossref] [PubMed]
- Krook MA, Bonneville R, Chen HZ, et al. Tumor heterogeneity and acquired drug resistance in FGFR2-fusion-positive cholangiocarcinoma through rapid research autopsy. Cold Spring Harb Mol Case Stud 2019;5:a004002 [Crossref] [PubMed]
- Lim ZF, Ma PC. Emerging insights of tumor heterogeneity and drug resistance mechanisms in lung cancer targeted therapy. J Hematol Oncol 2019;12:134. [Crossref] [PubMed]
- Hunter KW, Amin R, Deasy S, et al. Genetic insights into the morass of metastatic heterogeneity. Nat Rev Cancer 2018;18:211-23. [Crossref] [PubMed]
- Su Z, Wang Z, Ni X, et al. Inferring the Evolution and Progression of Small-Cell Lung Cancer by Single-Cell Sequencing of Circulating Tumor Cells. Clin Cancer Res 2019;25:5049-60. [Crossref] [PubMed]
- Rossi G, Russo A, Tagliamento M, et al. Precision Medicine for NSCLC in the Era of Immunotherapy: New Biomarkers to Select the Most Suitable Treatment or the Most Suitable Patient. Cancers (Basel) 2020;12:1125. [Crossref] [PubMed]
- Chan MH, Chow KM, Chan AT, et al. Quantitative analysis of pleural fluid cell-free DNA as a tool for the classification of pleural effusions. Clin Chem 2003;49:740-5. [Crossref] [PubMed]
- Gremel G, Lee RJ, Girotti MR, et al. Distinct subclonal tumour responses to therapy revealed by circulating cell-free DNA. Ann Oncol 2016;27:1959-65. [Crossref] [PubMed]
- Chen K, Sun J, Zhao H, et al. Non-invasive lung cancer diagnosis and prognosis based on multi-analyte liquid biopsy. Mol Cancer 2021;20:23. [Crossref] [PubMed]
- Jamal-Hanjani M, Quezada SA, Larkin J, et al. Translational implications of tumor heterogeneity. Clin Cancer Res 2015;21:1258-66. [Crossref] [PubMed]
- Rich JN. Cancer stem cells: understanding tumor hierarchy and heterogeneity. Medicine (Baltimore) 2016;95:S2-7. [Crossref] [PubMed]
- Burrell RA, McGranahan N, Bartek J, et al. The causes and consequences of genetic heterogeneity in cancer evolution. Nature 2013;501:338-45. [Crossref] [PubMed]
- Kreso A, Dick JE. Evolution of the cancer stem cell model. Cell Stem Cell 2014;14:275-91. [Crossref] [PubMed]
- Easwaran H, Tsai HC, Baylin SB. Cancer epigenetics: tumor heterogeneity, plasticity of stem-like states, and drug resistance. Mol Cell 2014;54:716-27. [Crossref] [PubMed]
- Gordon D, Huddleston J, Chaisson MJ, et al. Long-read sequence assembly of the gorilla genome. Science 2016;352:aae0344 [Crossref] [PubMed]
- Ji AL, Rubin AJ, Thrane K, et al. Multimodal Analysis of Composition and Spatial Architecture in Human Squamous Cell Carcinoma. Cell 2020;182:497-514.e22. [Crossref] [PubMed]
- Asp M, Giacomello S, Larsson L, et al. A Spatiotemporal Organ-Wide Gene Expression and Cell Atlas of the Developing Human Heart. Cell 2019;179:1647-1660.e19. [Crossref] [PubMed]
- Chen WT, Lu A, Craessaerts K, et al. Spatial Transcriptomics and In Situ Sequencing to Study Alzheimer's Disease. Cell 2020;182:976-991.e19. [Crossref] [PubMed]
- Chen S, Zhou Y, Chen Y, et al. fastp: an ultra-fast all-in-one FASTQ preprocessor. Bioinformatics 2018;34:i884-90. [Crossref] [PubMed]
- Li H, Durbin R. Fast and accurate long-read alignment with Burrows-Wheeler transform. Bioinformatics 2010;26:589-95. [Crossref] [PubMed]
- McKenna A, Hanna M, Banks E, et al. The Genome Analysis Toolkit: a MapReduce framework for analyzing next-generation DNA sequencing data. Genome Res 2010;20:1297-303. [Crossref] [PubMed]
- Lawrence MS, Stojanov P, Polak P, et al. Mutational heterogeneity in cancer and the search for new cancer-associated genes. Nature 2013;499:214-8. [Crossref] [PubMed]
- Mayakonda A, Lin DC, Assenov Y, et al. Maftools: efficient and comprehensive analysis of somatic variants in cancer. Genome Res 2018;28:1747-56. [Crossref] [PubMed]
- Zhong R, Li H, Zhang S, et al. Advances on Recognizing and Managing Tumor Heterogeneity. Zhongguo Fei Ai Za Zhi 2018;21:712-8. [PubMed]
- Castellanos E, Feld E, Horn L. Driven by Mutations: The Predictive Value of Mutation Subtype in EGFR-Mutated Non-Small Cell Lung Cancer. J Thorac Oncol 2017;12:612-23. [Crossref] [PubMed]
- Gibbons DL, Byers LA, Kurie JM. Smoking, p53 mutation, and lung cancer. Mol Cancer Res 2014;12:3-13. [Crossref] [PubMed]
- Parikh AR. Lung Cancer Genomics. Acta Med Acad 2019;48:78-83. [Crossref] [PubMed]
- Enokida Y, Shimizu K, Kakegawa S, et al. Single-nucleotide polymorphism (c.309T>G) in the MDM2 gene and lung cancer risk. Biomed Rep 2014;2:719-24. [Crossref] [PubMed]
- Dowlati A, Lipka MB, McColl K, et al. Clinical correlation of extensive-stage small-cell lung cancer genomics. Ann Oncol 2016;27:642-7. [Crossref] [PubMed]
- Mahapatra A. Lung cancer - genomics and personalized medicine. ACS Chem Biol 2010;5:529-31. [Crossref] [PubMed]
- Savli H, Sertdemir N, Aydin D, et al. TP53, EGFR and PIK3CA gene variations observed as prominent biomarkers in breast and lung cancer by plasma cell-free DNA genomic testing. J Biotechnol 2019;300:87-93. [Crossref] [PubMed]
- Nakagawa H, Wardell CP, Furuta M, et al. Cancer whole-genome sequencing: present and future. Oncogene 2015;34:5943-50. [Crossref] [PubMed]
- Kiyohara C, Otsu A, Shirakawa T, et al. Genetic polymorphisms and lung cancer susceptibility: a review. Lung Cancer 2002;37:241-56. [Crossref] [PubMed]
- Pisapia P, Malapelle U, Troncone G. Liquid Biopsy and Lung Cancer. Acta Cytol 2019;63:489-96. [Crossref] [PubMed]
- Cree IA, Uttley L, Buckley Woods H, et al. The evidence base for circulating tumour DNA blood-based biomarkers for the early detection of cancer: a systematic mapping review. BMC Cancer 2017;17:697. [Crossref] [PubMed]
- Fan Y, Shi M, Chen S, et al. Analysis of serum cfDNA concentration and integrity before and after surgery in patients with lung cancer. Cell Mol Biol (Noisy-le-grand) 2019;65:56-63. [Crossref] [PubMed]
(English Language Editor: K. Brown)