Bioinformatic analysis of differentially expressed genes and pathways in idiopathic pulmonary fibrosis
Introduction
Interstitial pulmonary disease (ILD) is a type of lung disease with unknown etiology and basic pathological changes of the diffuse lung parenchyma, alveolar inflammation, and interstitial fibrillation. Idiopathic pulmonary fibrosis (IPF) is a special type of ILD characterized by progressive chronic pulmonary fibrosis. This change leads to decreased pulmonary compliance, and eventually to respiratory failure and even death (1,2). Studies have shown that the occurrence of IPF may be related to epithelial cell formation disorder, fibroblast recruitment, fibroblast differentiation, and abnormal secretion of matrix proteins by pathological mesenchymal cells. Dysregulated repair responses induced by recurrent epithelial cell damage and excessive extracellular matrix accumulation result in pulmonary fibrosis (3). Moreover, increasing evidence indicates that genetic susceptibility plays a part in the development of idiopathic pulmonary fibrosis. Although these studies do not indicate a direct causal link, the potential importance of alterations in host defense (MUC5B, ATP11A, TOLLIP), telomere maintenance (TERT, TERC, OBFC1), and epithelial barrier function (DSP, DPP9) was identified (4). However, the molecular mechanism of various stages of IPF development remains unknown (5).
Gene sequencing technology can obtain the unknown genome sequence of individuals, and bioinformatics makes it possible to process this huge genome sequence information (6). In recent years, several studies have begun to use bioinformatics technology to search for biomarkers related to the incidence, diagnosis, and treatment of IPF from the genome sequence database of patients with IPF (7-10). Although very few differential genes have been found to have therapeutic effects, bioinformatics methods also provide a new way for us to explore potential biomarkers of IPF.
In this study, the data was derived from the GSE110147 profile. Bioinformatics techniques were used to identify differentially expressed genes between fresh frozen lung samples from patients with IPF and normal controls. We aim to elucidate the pathogenesis of IPF from the gene level with new insight and provide new clues to search for potential biomarkers related to diagnosis and treatment.
We present the following article following the STREGA reporting checklist (available at https://dx.doi.org/10.21037/atm-21-4224).
Methods
Acquisition and analysis of datasets
Gene expression profiles GSE110147 were downloaded from the Gene Expression Omnibus database platform was GPL6244[HuGene-1_0-st] Affymetrix Human Gene 1.0 ST Array. This microarray obtained samples from recipients’ organs of 22 patients with IPF, 10 with NSIP, and 5 with mixed IPF-NSIP undergoing lung transplantation. Normal lung tissue (n=11) was obtained from tissue that flanks lung cancer resections. In this study, 22 lung samples from IPF were classified as the IPF group. Meanwhile, 11 normal lung samples were classified as the control group. The Cancer Genome Atlas (TCGA) RNA-seq raw data from 515 unpaired tumor samples and 59 adjacent normal samples of lung adenocarcinoma (LUAD), 498 unpaired tumor samples and 55 adjacent normal samples of lung squamous cell carcinoma (LUSC) were downloaded from TCGA database. The Genotype-Tissue Expression (GTEx) raw RNA-seq of 288 normal lung samples were downloaded from GTEx database. The study was conducted in accordance with the Declaration of Helsinki (as revised in 2013).
DEGs screening and data processing
GERO2R is a GEO online analysis tool that can perform differential analysis of gene expression on two or more data sets in GEO samples. GEO2R (http://www.ncbi.nlm.nih.gov/geo/geo2r) was used to detect differentially expressed genes (DEGs) of IPF compared to normal tissues. The screening condition was P<0.05 and |log fold change| >0.5. The RNAseq data in TPM (transcripts per million reads) format from the TCGA and GTEx databases were firstly converted by log2 and then normalized. Then the converted data were used to compare the expression between samples.
GO and pathway enrichment analysis
Gene ontology (GO) is a major bioinformatics initiative to unify the representation of gene and gene product attributes in all species (11). KEGG (Kyoto Encyclopedia of Genes and Genomes) is a collection of databases dealing with genomes, biological pathways, diseases, drugs, and chemical substances. P<0.01 was set as the threshold value. The KEGG signal map was built in the online tool KEGG Mapper (https://www.kegg.jp/kegg/mapper.html).
Construction of the PPI network
The STRING online analysis website (http://www.string-db.org/) was used to construct the protein interaction network diagram of DEG (12). All associations obtained in STRING were provided with a confidence score. The credibility was set to 0.700.
Prediction of transcription factors of DEGs
Utilizing NCBI website (https://www.ncbi.nlm.nih.gov/) to query the promoter region of genes. The Genome Browser tool of the University of California Santa Cruz (UCSC) online website (http://genome.ucsc.edu/) was used to predict transcription factors (TFs) of target genes. The TFs were defined to satisfy the following criteria: pack, score >600, regulation direction consistent with the transcription direction of target gene.
Statistical analysis
We used R software (Version 4.0.3) for all statistical analysis and visualization. “clusterProfiler”, a widely used package in the bioinformatics area, has been developed to relate functional terms to gene lists using a clustering algorithm (13). To analyze DEGs at the functional level, we performed GO and KEGG pathway enrichment analysis using the “clusterProfiler” package to obtain enriched biological processes and pathways. The RNA-seq raw data from TCGA and GEO databases were normalized through the normalizeBetweenArray function from R package “Limma” from the Bioconductor project.Ggplot2 [version 3.3.3] was used to analyze and visualize the differential expression of target genes between lung adenocarcinoma, lung squamous cell carcinoma and normal tissues. Survminer package [0.4.9 version] and survival package [3.2-10 version] were used for visualization, statistical analysis of survival data respectively.
Results
Identify differentially expressed genes
The qualified profiling datasets GSE110147 were selected from the GEO database. The GEO2R tool was used for the analysis. In this dataset, 9,183 DEGs met the criteria, of which 4,638 genes were up-regulated, and 4,545 genes were down-regulated. Figure 1 presents all the expressed genes in the form of a volcano. SPP1, MMP1, and BPIFB1 were the three main significant DEGs, while VTRNA1-1, SNORD41, and SNORA3A represented the three most significantly negatively regulated DEGs.
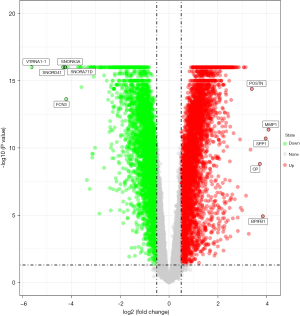
GO and pathway enrichment analysis
Following the analysis of GO and the pathway for DEGs, the terms of BP (biological process) terms of GO were collected and shown in Figure 2A. GO terms were mainly related to neutrophil-mediated immunity, neutrophil activation, and cell cycle phase transition regulation.
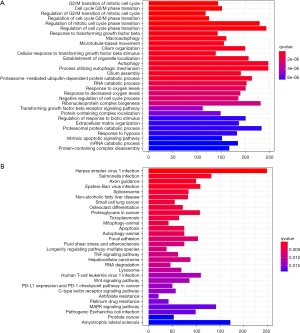
The pathways were mainly related to the MAPK signaling pathway, cancer, the T cell receptor signaling pathway, and the expression of PD-L1 and the PD-1 checkpoint pathway in cancer, including HSV-1 infection and HTLV-1 infection (Figure 2B).
The 50 DEGs associated with PD-L1 expression and the PD-1 checkpoint pathway in cancer were reclustered. The results showed they were associated with the MAPK signaling pathway, the PI3K-Akt signaling pathway, the Ras signaling pathway, and non-small cell lung cancer. The results of the KEGG enrichment analysis are shown in Figure 3. Additionally, AKT1 and PIK3CA are associated with both the PI3K-Akt signaling pathway, Ras signaling pathway, and non-small cell lung cancer.
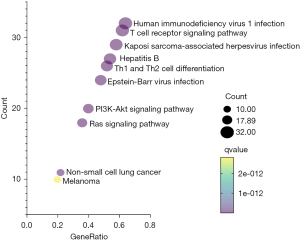
Construction of DEG enrichment PPI networks in the PD-L1 expression and PD-1 checkpoint pathway in cancer
Based on data from the STRING 11.0 version database, the PPI network was constructed. Figure 4 shows the PPI network for 50 DEGs enriched in the PD-L1 expression and PD-1 checkpoint pathway in cancer, consisting of 50 nodes and 278 edges. Figure 5 shows the gene co-expression network for the 50 differentially expressed genes (DEGs) associated with the PD-L1 expression and the PD-1 checkpoint pathway in cancer. Among the total 1,225 gene pairs, 302 gene pairs are significantly co-expressed (P<0.05), and 67.2% of the 302 gene pairs are with spearman correlation coefficient >0.5 or <−0.5. Eleven genes from these 50 DEGs were found to be located in the non-small cell lung cancer pathway, and their positions in the non-small cell lung cancer pathway are shown in Figure 6.

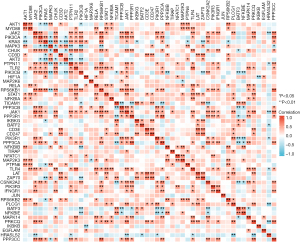
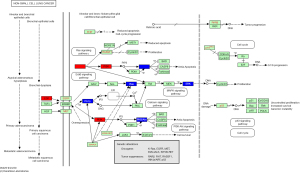
Transcription factor prediction of 11 DEGs in the PD-L1 expression and PD-1 checkpoint pathway in cancer
The transcription factor information of these 11 DEGs is in Table 1. Among them, it could be observed that CTCFL, SP2, and ZNF341 are the three most common transcription factors that participant in regulating these 11 genes’ transcription. In addition, we found that among CTCFL, SP2 and ZNF341, CTCFL may be involved in mediating the regulation of the transcription of the most numerous genes, including KRAS, MAPK3, AKT2, PIK3CB, PIK3R1, PIK3R3. The level of mRNA encoding these three transcription factors is different between the IPF group and the normal group of CSE110147 (Figure 7). Figure 8 shows the gene co-expression network for the 9 DEGs and 3 transcription factors in IPF. Among the total 66 gene pairs, 21 gene pairs are significantly co-expressed (P<0.05), and 81.0% of the 21 gene pairs are with Pearman correlation coefficient >0.5 or <−0.5. The expression level of KRAS, MAPK3, PIK3CB are correlated with each other (P<0.05). Meanwhile, CTCFL has a significant co-expression relationship with KRAS, MAPK3 and PIK3CB, respectively.
Table 1
Gene name | Promoter sequence | Transcription factor |
---|---|---|
|
Chr14:104795649-104797748 | – |
|
Chr3:179146114-179148213 | NFIL3; ZNF384 |
|
Chr12:25250830-25252929 | SP2; CTCFL; ZNF341 |
|
Chr16:30123210-30125309 | CTCFL; ZNF341 |
|
Chr19:40285432-40287531 | CTCFL; ZNF341 |
|
Chr3:138834829-138836928 | SP2; CTCFL; ZNF341 |
|
Chr5:68213737-68215836 | TBX20; SP1; SP2; ZNF740; ASCL1 |
|
Chr1:46174802-46176901 | CTCFL; ZNF341 |
|
Chr20:41135519-41137618 | ZNF263; SP2 |
|
Chr4:109910883-109912982 | ZNF460; ZNF263; SP2 |
|
Chr11:535477-537576 | CTCFL; ZNF341 |
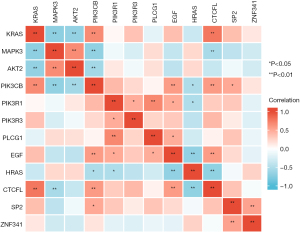
Expression level and prognostic analysis of TFs and target genes in LUAD and LUSC
Figure 9 and Figure 10 show the expression level of these 11 DEGs and 3 transcription factors between LUAD and normal samples. All of them were differentially expressed (P<0.05). Figure 11 and Figure 12 show the expression level of these 11 DEGs and 3 transcription factors between LUSC and normal samples. Except for the EGF, the other genes’ expression level between LUSC and normal samples were differentially significant (P<0.05). Figure 13 shows the overall survival curve of lung adenocarcinoma (LUAD) and lung squamous cell carcinoma (LUAC) stratified by AKT2, KRAS, PIK3R1 and MAPK3.
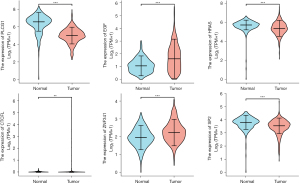
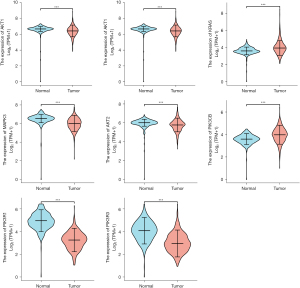
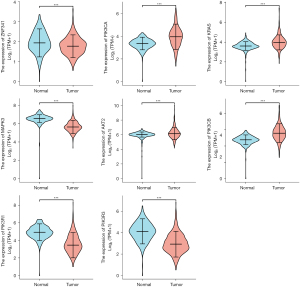
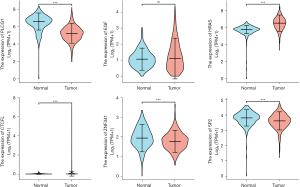
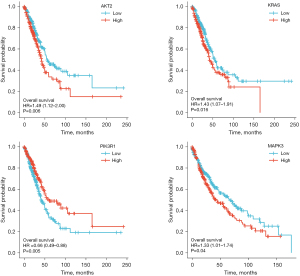
Discussion
IPF is a progressive fibrogenesis disease with a poor prognosis. The median survival time of this disease has been reported to be only 2–3 years after diagnosis (14). Bioinformatics is an emerging professional field in recent years, which can be used to reveal the biological mysteries of a vast amount of complex biological data and is widely used in the analysis of disease-causing genes (6). For many years, the relevant mechanism of the pathogenesis of IPF has not been fully understood (15,16). Therefore, this article aimed to use bioinformatics methods to clarify the key differential genes and signal pathways in the pathogenesis of IPF.
In this study, 9,183 DEGs were identified between the IPF group and the normal control group by analyzing the GSE110147 gene expression profile. Given that the purpose of this study was to elucidate the relationship between gene expression and IPF and explore the possible pathogenic genes of IPF further, the NSIP samples were excluded.
MMP1 was the gene most significantly up-regulated in this study. The previous study has confirmed that MMP1 played a role in two key repair processes: scar tissue regeneration and dissolution (17). The mechanism of MMP1 in the regulation of pulmonary fibrosis is still unclear, and many experiments are being carried out to verify it. The research speculated that these two polymorphic sites of MMP1 (−1,607, −775) play an important role in the pathogenesis of IPF and may regulate the transcriptional regulation in which the AP-1 factor may participate (18). The gene expression profiling of IPF lungs has revealed that one of the most up-regulated MMPs is MMP1 (19). It is mainly localized in the reactive alveolar epithelium of IPF lungs (20). Furthermore, the serum expression level of MMP1 has been shown to be a potential diagnostic biomarker for IPF (21).
The MAPK signaling pathway was the most significant enrichment pathway in KEGG enrichment in this study. Previous studies have shown that p38 MAPK plays a crucial role in LiCl- and Wnt1-induced EMT in A549 cells (22). EMT and fibrin synthesis can be blocked by the interference of SB203580 or p38 MAPK siRNA in the p38 MAPK pathway, and this process can be reversed by transfection of the p38 MAPK expression plasmid (22). Matsuda et al. showed that the progression of pulmonary fibrosis was co-occurring with increased activity of p38 mitogen-activated protein kinase in AEC II, triggering inflammation and improving the immune system (23).
In the past, the PD-1/PD-L1 pathway was mainly considered involved in the immune escape mechanism of various tumors (24-26) and can affect the treatment and prognosis of patients with lung cancer (27,28). However, the relationship between the pathway (PD-L1 expression and PD-1 checkpoint) and the pathogenesis and prognosis of IPF is rarely questioned. In our research, the 50 up-regulated DEGs were mainly enriched in PD-L1 and the PD-1 checkpoint pathway in cancer. The reenrichment results of the 50 DEGs showed that they were related to the MAPK signaling pathway, the PI3K-Akt signaling pathway, the Ras signaling pathway, and non-small cell lung cancer. Eleven genes from these 50 DEGs were located in the non-small cell lung cancer pathway. The transcription factor information of these 11 DEGs shows that CTCFL, SP2, and ZNF341 are the three most common TFs mediating these 11 genes’ transcription. Gene co-expression network for the 9 DEGs of these 11 DEGs and 3 transcription factors shows that KRAS, MAPK3, PIK3CB are co-expressed with each other, and the Pearson correlation coefficient are all high. Interestingly, their expressions are significantly correlated with the expression levels of CTCFL transcription factors.
KRAS is the most common proto-oncogene associated with NSCLC and has been found in 11.2% and 1.8% of lung cancer cases in Asia (29). KRAS can be dysregulated and lead to tumor growth, playing a key role in controlling interactions between cancer cells and the microenvironment (30). Notably, there is preclinical evidence indicating that programmed cell death ligand 1 (PD-L1) expression is elevated intrinsically, rather than adaptively, through the activation of down-stream KRAS signaling pathways (31). CTCFL (CTCF-like), the paralog of CTCF, is normally transiently expressed in pre-meiotic male germ cells together with ubiquitously expressed CTCF. Genetic alterations in CTCFL have been found in numerous cancers (32). There is now accumulating evidence pointing to a role for CTCFL in the progression, or metastatic potential of cancers via its ability to transcriptionally reprogram cells (33).
Clinical practice has shown that antibody therapy of PD-1 and its ligands has a significant response rate in various cancers, which has brought revolutionary changes in cancer treatment (34,35). In addition, experimental studies have revealed the molecular mechanism of PD-1 targeting. For example, when PD-1 binds to the ligand, the CK2 protein inhibits the PI3-Akt signaling pathway activated by the T cell receptor to play a role. Additionally, tumors can limit the host immune response by increasing the expression of the PD-1 ligand in the microenvironment. One of these mechanisms is abnormalities in tumor cell signaling pathways, including activation of the EGFR, MAPK, or PI3K-Akt signaling pathways, and increased expression of STAT3 and HIF-1 (36).
The potential role of the PD-1/PD-L1 checkpoint in IPF has recently been established, which indicates that the PD-1/PD-L1 checkpoint is induced in IPF, which indicates immune editing that could affect disease progression (37). Blocking the PD-1 pathway can significantly reduce the expression of IL-17A in CD4+ T cells by reducing the expression of PSTAT3, leading to a significant decrease in collagen-1 production (38). There have also been studies that have confirmed the inhibitory effect of gene ablation or antibody-mediated PD-L1 on fibroblasts, leading to a significant decrease in the ability to invade and migrate in vitro and collagen production in vivo (39).
Extensive epidemiological surveys support an association between IPF and lung cancer (40,41). A systematic review indicated that the prevalence of IPF comorbidities in lung cancer ranged from 3% to 48% (42). This suggests that IPF may have the same genetic variation as lung cancer. Compared to IPF patients without a lung cancer diagnosis, the average survival time of IPF patients with lung cancer is reduced by 1.6–1.7 years (43). The mechanism of lung cancer development in IPF patients has received increasing attention in recent years (43,44). Currently, treatment options for IPF-LC are limited (45,46). An individual case of a 62-year-old man diagnosed with lung adenocarcinoma and IPF was reported. After initial chemotherapy failed, he received nilumab and achieved complete remission, with no signs of worsening of the IPF. The response to nivolumab persisted >1 year (47). This also suggests the use of a human immune checkpoint inhibitor antibody to inhibit the PD-1 receptor or PD-L1 from treating lung cancer with IPF comorbidities in the future.
Conclusions
In conclusion, our study indicated that DEGs, including MPP1, could be potential targets and biomarkers of IPF, and the MAPK signaling pathway is related to the occurrence and development of IPF. The development of lung cancer complications in IPF may be related to the activation of PD-L1 expression and PD-1 checkpoint pathway. KRAS, MAPK3, PIK3CB and CTCFL may play potentially important roles in the course of IPF complicated by lung cancer. There may exists in unidentified form of protein-protein interaction among KRAS, MAPK3 and PIK3CB, meanwhile their expression may all be regulated by CTCFL. However, no basic experiments or clinical practice are conducted in this study; therefore, further exploration is needed in the future.
Acknowledgments
We acknowledge the GEO database for providing its platforms and contributors for uploading their meaningful datasets.
Funding: This study was supported by grants from the Henan Provincial Key Laboratory for Interstitial Lung Disease and Lung Transplantation.
Footnote
Reporting Checklist: The authors have completed the STREGA reporting checklist. Available at https://dx.doi.org/10.21037/atm-21-4224
Conflicts of Interest: All authors have completed the ICMJE uniform disclosure form (available at https://dx.doi.org/10.21037/atm-21-4224). The authors have no conflicts of interest to declare.
Ethical Statement: The authors are accountable for all aspects of the work in ensuring that questions related to the accuracy or integrity of any part of the work are appropriately investigated and resolved. The study was conducted in accordance with the Declaration of Helsinki (as revised in 2013). The data of our study was derived from the public database, no ethical approval is needed.
Open Access Statement: This is an Open Access article distributed in accordance with the Creative Commons Attribution-NonCommercial-NoDerivs 4.0 International License (CC BY-NC-ND 4.0), which permits the non-commercial replication and distribution of the article with the strict proviso that no changes or edits are made and the original work is properly cited (including links to both the formal publication through the relevant DOI and the license). See: https://creativecommons.org/licenses/by-nc-nd/4.0/.
References
- King TE Jr, Pardo A, Selman M. Idiopathic pulmonary fibrosis. Lancet 2011;378:1949-61. [Crossref] [PubMed]
- Richeldi L, Collard HR, Jones MG. Idiopathic pulmonary fibrosis. Lancet 2017;389:1941-52. [Crossref] [PubMed]
- Inui N, Sakai S, Kitagawa M. Molecular Pathogenesis of Pulmonary Fibrosis, with Focus on Pathways Related to TGF-β and the Ubiquitin-Proteasome Pathway. Int J Mol Sci 2021;22:6107. [Crossref] [PubMed]
- Noth I, Zhang Y, Ma S-F, et al. Genetic variants associated with idiopathic pulmonary fibrosis susceptibility and mortality: a genome-wide association study. The Lancet Respiratory Medicine. 2013;1:309-17. [Crossref] [PubMed]
- Wolters PJ, Collard HR, Jones KD. Pathogenesis of idiopathic pulmonary fibrosis. Annu Rev Pathol 2014;9:157-79. [Crossref] [PubMed]
- Gauthier J, Vincent AT, Charette SJ, et al. A brief history of bioinformatics. Brief Bioinform 2019;20:1981-96. [Crossref] [PubMed]
- Wang H, Wang M, Xiao K, et al. Bioinformatics analysis on differentially expressed genes of alveolar macrophage in IPF. Exp Lung Res 2019;45:288-96. [Crossref] [PubMed]
- Cecchini MJ, Hosein K, Howlett CJ, et al. Comprehensive gene expression profiling identifies distinct and overlapping transcriptional profiles in non-specific interstitial pneumonia and idiopathic pulmonary fibrosis. Respir Res 2018;19:153. [Crossref] [PubMed]
- Xu Z, Mo L, Feng X, et al. Using bioinformatics approach identifies key genes and pathways in idiopathic pulmonary fibrosis. Medicine (Baltimore) 2020;99:e22099 [Crossref] [PubMed]
- C L. Common molecular pathways targeted by nintedanib in cancer and IPF: A bioinformatic study. Pulm Pharmacol Ther 2020;64:101941 [Crossref] [PubMed]
- Al-Shahrour F, Díaz-Uriarte R, Dopazo J. FatiGO: a web tool for finding significant associations of Gene Ontology terms with groups of genes. Bioinformatics 2004;20:578-80. [Crossref] [PubMed]
- Peng X, Wang J, Peng W, et al. Protein-protein interactions: detection, reliability assessment and applications. Brief Bioinform 2017;18:798-819. [PubMed]
- Kanehisa M, Furumichi M, Tanabe M, et al. KEGG: new perspectives on genomes, pathways, diseases and drugs. Nucleic Acids Res 2017;45:D353-61. [Crossref] [PubMed]
- Martinez FJ, Collard HR, Pardo A, et al. Idiopathic pulmonary fibrosis. Nat Rev Dis Primers 2017;3:17074. [Crossref] [PubMed]
- Mora AL, Rojas M, Pardo A, et al. Emerging therapies for idiopathic pulmonary fibrosis, a progressive age-related disease. Nat Rev Drug Discov 2017;16:755-72. [Crossref] [PubMed]
- Spagnolo P, Cottin V. Genetics of idiopathic pulmonary fibrosis: from mechanistic pathways to personalised medicine. J Med Genet 2017;54:93-9. [Crossref] [PubMed]
- Rohani MG, Parks WC. Matrix remodeling by MMPs during wound repair. Matrix Biol 2015;44-46:113-21. [Crossref] [PubMed]
- Checa M, Ruiz V, Montaño M, et al. MMP-1 polymorphisms and the risk of idiopathic pulmonary fibrosis. Hum Genet 2008;124:465-72. [Crossref] [PubMed]
- Zuo F, Kaminski N, Eugui E, et al. Gene expression analysis reveals matrilysin as a key regulator of pulmonary fibrosis in mice and humans. Proc Natl Acad Sci U S A 2002;99:6292-7. [Crossref] [PubMed]
- Pardo A, Cabrera S, Maldonado M, et al. Role of matrix metalloproteinases in the pathogenesis of idiopathic pulmonary fibrosis. Respir Res 2016;17:23. [Crossref] [PubMed]
- Morais A, Beltrão M, Sokhatska O, et al. Serum metalloproteinases 1 and 7 in the diagnosis of idiopathic pulmonary fibrosis and other interstitial pneumonias. Respir Med 2015;109:1063-8. [Crossref] [PubMed]
- Fang CX, Ma CM, Jiang L, et al. p38 MAPK is Crucial for Wnt1- and LiCl-Induced Epithelial Mesenchymal Transition. Curr Med Sci 2018;38:473-81. [Crossref] [PubMed]
- Matsuda S, Kim JD, Sugiyama F, et al. Transcriptomic Evaluation of Pulmonary Fibrosis-Related Genes: Utilization of Transgenic Mice with Modifying p38 Signal in the Lungs. Int J Mol Sci 2020;21:6746. [Crossref] [PubMed]
- Cha JH, Chan LC, Li CW, et al. Mechanisms Controlling PD-L1 Expression in Cancer. Mol Cell 2019;76:359-70. [Crossref] [PubMed]
- Hu Q, Ye Y, Chan LC, et al. Oncogenic lncRNA downregulates cancer cell antigen presentation and intrinsic tumor suppression. Nat Immunol 2019;20:835-51. [Crossref] [PubMed]
- Jiang X, Wang J, Deng X, et al. Role of the tumor microenvironment in PD-L1/PD-1-mediated tumor immune escape. Mol Cancer 2019;18:10. [Crossref] [PubMed]
- Yu H, Boyle TA, Zhou C, et al. PD-L1 Expression in Lung Cancer. J Thorac Oncol 2016;11:964-75. [Crossref] [PubMed]
- Peng S, Wang R, Zhang X, et al. EGFR-TKI resistance promotes immune escape in lung cancer via increased PD-L1 expression. Mol Cancer 2019;18:165. [Crossref] [PubMed]
- Dearden S, Stevens J, Wu YL, et al. Mutation incidence and coincidence in non small-cell lung cancer: meta-analyses by ethnicity and histology (mutMap). Ann Oncol. 2013;24:2371-6. [Crossref] [PubMed]
- Reck M, Carbone DP, Garassino M, et al. Targeting KRAS in non-small-cell lung cancer: recent progress and new approaches. Ann Oncol 2021;32:1101-10. [Crossref] [PubMed]
- Chen N, Fang W, Lin Z, et al. KRAS mutation-induced upregulation of PD-L1 mediates immune escape in human lung adenocarcinoma. Cancer Immunol Immunother 2017;66:1175-87. [Crossref] [PubMed]
- Debaugny RE, Skok JA. CTCF and CTCFL in cancer. Curr Opin Genet Dev 2020;61:44-52. [Crossref] [PubMed]
- Hoivik EA, Kusonmano K, Halle MK, et al. Hypomethylation of the CTCFL/BORIS promoter and aberrant expression during endometrial cancer progression suggests a role as an Epi-driver gene. Oncotarget. 2014;5:1052-61. [Crossref] [PubMed]
- Topalian SL, Taube JM, Pardoll DM. Neoadjuvant checkpoint blockade for cancer immunotherapy. Science 2020;367:eaax0182 [Crossref] [PubMed]
- Marabelle A, Fakih M, Lopez J, et al. Association of tumour mutational burden with outcomes in patients with advanced solid tumours treated with pembrolizumab: prospective biomarker analysis of the multicohort, open-label, phase 2 KEYNOTE-158 study. Lancet Oncol 2020;21:1353-65. [Crossref] [PubMed]
- Boussiotis VA. Molecular and Biochemical Aspects of the PD-1 Checkpoint Pathway. N Engl J Med 2016;375:1767-78. [Crossref] [PubMed]
- Duitman J, van den Ende T, Spek CA. Immune Checkpoints as Promising Targets for the Treatment of Idiopathic Pulmonary Fibrosis? J Clin Med 2019;8:1547. [Crossref] [PubMed]
- Celada LJ, Kropski JA, Herazo-Maya JD, et al. PD-1 up-regulation on CD4+ T cells promotes pulmonary fibrosis through STAT3-mediated IL-17A and TGF-β1 production. Sci Transl Med 2018;10:eaar8356 [Crossref] [PubMed]
- Geng Y, Liu X, Liang J, et al. PD-L1 on invasive fibroblasts drives fibrosis in a humanized model of idiopathic pulmonary fibrosis. JCI Insight 2019;4:e125326 [Crossref] [PubMed]
- Tzouvelekis A, Spagnolo P, Bonella F, et al. Patients with IPF and lung cancer: diagnosis and management. Lancet Respir Med 2018;6:86-8. [Crossref] [PubMed]
- Tzouvelekis A, Karampitsakos T, Gomatou G, et al. Lung cancer in patients with Idiopathic Pulmonary Fibrosis. A retrospective multicenter study in Greece. Pulm Pharmacol Ther 2020;60:101880 [Crossref] [PubMed]
- Raghu G, Amatto VC, Behr J, et al. Comorbidities in idiopathic pulmonary fibrosis patients: a systematic literature review. Eur Respir J 2015;46:1113-30. [Crossref] [PubMed]
- Ballester B, Milara J, Cortijo J. Idiopathic Pulmonary Fibrosis and Lung Cancer: Mechanisms and Molecular Targets. Int J Mol Sci 2019;20:593. [Crossref] [PubMed]
- Tzouvelekis A, Gomatou G, Bouros E, et al. Common Pathogenic Mechanisms Between Idiopathic Pulmonary Fibrosis and Lung Cancer. Chest 2019;156:383-91. [Crossref] [PubMed]
- Ogura T, Takigawa N, Tomii K, et al. Summary of the Japanese Respiratory Society statement for the treatment of lung cancer with comorbid interstitial pneumonia. Respir Investig 2019;57:512-33. [Crossref] [PubMed]
- Shirasawa M, Fukui T, Kusuhara S, et al. Efficacy and risk of cytotoxic chemotherapy in extensive disease-small cell lung cancer patients with interstitial pneumonia. BMC Cancer 2019;19:163. [Crossref] [PubMed]
- Ide M, Tanaka K, Sunami S, et al. Durable response to nivolumab in a lung adenocarcinoma patient with idiopathic pulmonary fibrosis. Thorac Cancer 2018;9:1519-21. [Crossref] [PubMed]
(English Language Editor: J. Chapnick)