Competing endogenous RNA network for esophageal cancer progression
Introduction
Esophageal cancer (ESCA) is one of the most common cancers worldwide, and a spectacular rise in its incidence has been observed in Western countries over the last four decades (1,2). Barrett’s esophagus is a precursor to ESCA, which sees the replacement of normal esophageal squamous epithelium with columnar epithelia and goblet cells (3). There are several therapeutic approaches for ESCA, among which endoscopic mucosal resection (for early ESCA and precancerous lesions) and radiotherapy are the most common (4). However, due to metastasis, the 5-year survival rate of patients with ESCA is low, standing at only 18.3% (5). Therefore, understanding the mechanisms of ESCA progression is critical to improving the prognosis of patients with this disease.
Long non-coding RNAs (lncRNAs) are noncoding RNAs exceeding 200 nucleotides in length. LncRNAs bind microRNAs (miRNAs/miRs) and act as miRNA sponges, reducing their regulatory abilities (6). Alterations in lncRNA expression profiles are associated with the progression of many diseases, including cancer. For instance, the lncRNA H19 was found to sponge miR-138 and miR-200a to promote epithelial-mesenchymal transition (EMT) in colorectal cancer (7). Kallen et al. also discovered let-7 binding sites in lncRNA H19, which indicates that this lncRNA may influence let-7 regulatory functions (8). Further, Wu et al. reported that the lncRNA PAGBC could suppress miR-133b and miR-511 function and promote gallbladder tumorigenesis (9). Several studies about lncRNAs in ESCA have also been conducted. For instance, Xu et al. described the ability of lncRNA HOTAIR to sponge miR-148a and promote EMT in ESCA (10). In their study, Li et al. quantified the expression levels of the lncRNA UCA1 in ESCA through quantitative real-time PCR, finding it to be a poor prognostic indicator (11). Another study demonstrated that lncRNA PEG10 could inhibit ESCA cell proliferation and invasion, and promote apoptosis (12).
As small non-coding RNAs, miRNAs can down-regulate the expression of mRNAs by inhibiting their translation (13). miRNA abundance can also be influenced by other types of RNA, including lncRNAs, containing tandem repeats of miRNA response elements (MREs) (14). Interactions between MRE-containing RNAs, miRNAs and mRNAs form the competing endogenous RNA (ceRNA) network. An aberrant ceRNA network can result in many diseases, including cancer (15-17). Numerous studies have focused on the roles of ceRNA networks in cancers such as bladder (18), breast (19), and gastric (20) cancer. Research of ceRNA networks is critical to understanding the progression of diseases; however, no such study has been conducted for ESCA.
In this study, we conducted a comprehensive analysis of lncRNA, miRNA, and mRNA expression profiles in ESCA using Gene Expression Omnibus (GEO). A ceRNA network was constructed based on the miRCode and TargetScan databases. From another independent ESCA dataset, several prognostic genes were obtained. This study aimed to identify potential biomarkers for ESCA in order to improve the early diagnosis and treatment of patients with the disease. We present the following article in accordance with the REMARK reporting checklist (available at https://dx.doi.org/10.21037/atm-21-4478).
Methods
Microarray datasets
ESCA lncRNA and mRNA expression profiles were downloaded from GEO (https://www.ncbi.nlm.nih.gov/geo/) (accession No. GSE89102). Five ESCA tissues and five adjacent non-tumor tissues were included. The Agilent-045997 Arraystar human lncRNA microarray V3 (GPL16596) platform was used to profile the expression of lncRNAs and mRNAs in the tissues. The study was conducted in accordance with the Declaration of Helsinki (as revised in 2013). This study was approved by the Ethics Committee of The Affiliated Tumor Hospital of Nantong University (No. 2020811521).
Microarray analysis
Raw microarray data were imported into R programming software and quartile normalization was conducted. Differentially expressed lncRNAs and mRNAs (DElncRNAs and DEmRNAs, respectively) in the ESCA samples compared with the normal samples were identified using the limma package (21), with the thresholds of adjusted P<0.05 and |log2(fold change)| >1.
Functional enrichment analysis
To investigate the biological processes involved in ESCA progression, we conducted functional enrichment analysis of DEmRNAs through DAVID (the Database for Annotation, Visualization and Integrated Discovery, https://david.ncifcrf.gov/) (22). Gene Ontology (GO) terms and Kyoto Encyclopedia of Genes and Genomes (KEGG) pathways meeting the criterion of P<0.05 were screened out.
ceRNA network analysis
To identify the miRNAs with which the DElncRNAs interact, the miRCode database (http://www.mircode.org/) (23) was searched for predicted lncRNA-miRNA pairs. Besides, an ESCA miRNA expression profile dataset (containing three ESCA samples and three normal samples) was obtained from GEO (accession no. GSE71043), to identify differentially expressed miRNAs (DEmiRNAs) in ESCA compared with normal samples. Consistent with the analysis of DElncRNA and DEmRNA expression, only miRNAs meeting the criteria of adjusted P<0.05 and |log2(fold change)| >1 were considered as differentially expressed. Then, interactions between DElncRNAs and DEmiRNAs were obtained. Targets of DEmiRNAs were screened using the TargetScan database (http://www.targetscan.org/vert_71/) (24), and only DEmRNAs were included in the ceRNA network. Finally, the DElncRNA-DEmiRNA-DEmRNA ceRNA network was obtained and visualized with the Cytoscape software (25).
Survival analysis
The associations of RNAs contained in the ceRNA network with ESCA prognosis were explored in the ESCA dataset from The Cancer Genome Atlas (TCGA, https://cancergenome.nih.gov/). ESCA samples were divided into two groups based on the median expression value of a specific gene, and the log-rank test was used to test the survival difference between the two groups.
Results
DElncRNAs and DEmRNAs
A total of 1,014 DElncRNAs (531 down-regulated and 483 up-regulated) and 3,677 DEmRNAs (1,408 down-regulated and 2,269 up-regulated) were identified in ESCA samples compared with normal samples. Supervised clustering of the 10 samples based on the DElncRNAs and DEmRNAs was conducted. As shown in Figure 1A,1B, ESCA and normal samples could be distinguished by DElncRNAs and DEmRNAs, respectively. Figure 1C,1D show scatter plots of lncRNA and mRNA expression profiles, respectively; down-regulated and up-regulated genes are represented by red and blue dots, respectively, while the black dots represent non-differentially expressed genes.
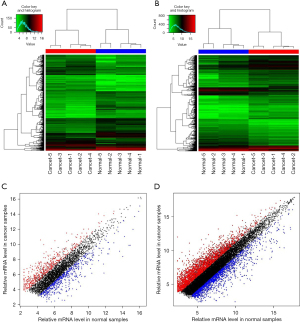
Enriched functions
The functions of the top 1,000 most significant DEmRNAs were analyzed with DAVID . Based on the threshold of P<0.05, 65 GO terms and 6 KEGG pathways were significantly enriched in DEmRNAs. Figure 2 show the top 20 most significantly enriched GO terms and all of the significantly enriched KEGG pathways. The DEmRNAs were closely associated with biological processes related to cell activity and oxygen metabolism.
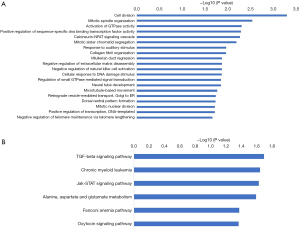
ceRNA network
A total of 35 DEmiRNAs were identified in ESCA samples compared with normal samples. Table 1 shows the full list of DEmiRNAs and their corresponding P value and log2(fold change). By using a combination of the miRCode and TargetScan databases, we obtained a ceRNA network containing 5 DEmiRNAs, 582 DEmRNAs, and 764 DElncRNAs. Figure 3 depicts the ceRNA network, with red, green, and blue circles representing DEmiRNAs, DEmRNAs, and DElncRNAs, respectively.
Table 1
miRNA ID | Log2FC | P value |
---|---|---|
let-7d | 1.83 | 2.31E-07 |
miR-3911 | 3.02 | 1.26E-06 |
miR-144 | 5.03 | 5.50E-05 |
miR-1914 | 3.25 | 1.23E-04 |
miR-15a | 4.99 | 2.10E-04 |
miR-16 | 6.34 | 2.83E-04 |
miR-93 | 3.1 | 1.16E-03 |
miR-4665 | −1.93 | 1.35E-03 |
let-7i | 1.74 | 1.39E-03 |
miR-1290 | 1.67 | 1.53E-03 |
miR-5196 | 3.29 | 2.25E-03 |
miR-107 | 3.04 | 2.34E-03 |
miR-130a | 5.73 | 4.13E-03 |
miR-106b | 4.4 | 4.34E-03 |
miR-23a | 3.88 | 5.54E-03 |
miR-191 | −2.43 | 7.14E-03 |
miR-19b | 4.19 | 8.68E-03 |
miR-1238 | −3.01 | 9.44E-03 |
miR-6069 | −2.61 | 9.50E-03 |
miR-4721 | 3.3 | 1.05E-02 |
miR-25 | 4.06 | 1.13E-02 |
miR-4433 | −2.83 | 1.20E-02 |
miR-185 | 3.2 | 1.34E-02 |
miR-21 | 2.5 | 1.34E-02 |
miR-130b | 3.44 | 1.81E-02 |
miR-1228 | −2.29 | 1.95E-02 |
miR-937 | −1.73 | 2.10E-02 |
miR-1234 | −2.24 | 2.44E-02 |
miR-451a | 4.19 | 2.82E-02 |
miR-20a | 2.32 | 2.85E-02 |
miR-3676 | −2.43 | 3.13E-02 |
miR-6508 | −3.4 | 3.44E-02 |
miR-6515 | −2.41 | 3.47E-02 |
miR-345 | 2.41 | 4.43E-02 |
miR-3648 | −2.22 | 4.96E-02 |
Log2FC represents log2(fold change). ESCA, esophageal cancer. DEmRNAs, differentially expressed miRNAs.
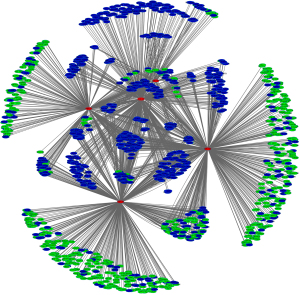
Identification of ESCA prognostic genes
The ESCA dataset from TCGA was used to identify prognostic biomarkers in ESCA from the ceRNA network. A total of 39 genes were found to be significantly associated with survival of ESCA; these 39 genes and their corresponding P values and hazard ratios are shown in Table 2. Figure 4 depicts Kaplan-Meier curves based on the expression of GOLGA7, NFYB, TOP1, and TMTC3. The high expression of each of these genes was associated with a poor prognosis.
Table 2
Gene | P value | HR |
---|---|---|
|
0.001372 | 1.035714 |
|
0.002612 | 1.111111 |
|
0.004158 | 0.965517 |
|
0.004324 | 0.78 |
|
0.004354 | 1.111111 |
|
0.004698 | 0.78125 |
|
0.004975 | 1.035714 |
|
0.005199 | 0.583333 |
|
0.005324 | 0.9 |
|
0.006564 | 0.9 |
|
0.009548 | 0.676471 |
|
0.011359 | 0.965517 |
|
0.012267 | 0.965517 |
|
0.015632 | 0.965517 |
|
0.019227 | 0.965517 |
|
0.019846 | 0.83871 |
|
0.020843 | 0.78125 |
|
0.021467 | 1.478261 |
|
0.021503 | 1.035714 |
|
0.024927 | 1.192308 |
|
0.025207 | 0.83871 |
|
0.027246 | 0.810867 |
|
0.028846 | 0.676471 |
|
0.029623 | 0.9 |
|
0.030387 | 0.83871 |
|
0.031228 | 0.9 |
|
0.034102 | 0.83871 |
|
0.034606 | 1.308132 |
|
0.035199 | 0.73 |
|
0.0363 | 1.111111 |
|
0.036416 | 0.965517 |
|
0.039097 | 1.28 |
|
0.042334 | 0.676471 |
|
0.042671 | 0.78125 |
|
0.043983 | 0.727273 |
|
0.044488 | 0.676471 |
|
0.045206 | 0.9 |
|
0.045968 | 1.035714 |
|
0.048985 | 0.9 |
CeRNA, competing endogenous RNA; ESCA, esophageal cancer; HR, hazard ratio.
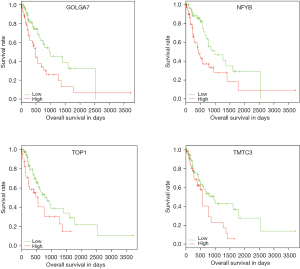
Discussion
As the ninth most common cancer in the world, ESCA affects more than 450,000 people each year, and its incidence is increasing spectacularly (26). Although several therapeutic methods exist for ESCA (26-28), a lack of understanding of the disease’s mechanisms means its 5-year survival rate is still low. In this study, we conducted—for the first time—a ceRNA network analysis for ESCA and identified several prognostic genes, which could be helpful for the diagnosis and treatment of patients with the disease.
In this study, the ceRNA network was restricted to DElncRNAs, DEmiRNAs, and DEmRNAs specific to ESCA. Studies have shown that miR-191, miR-144, and miR-93 interacted with DElncRNAs and could regulate DEmRNAs. All five of these miRNAs have been proved to be deregulated in ESCA. For instance, through quantitative real-time PCR, Ansari et al. reported that miR-93 was down-regulated in human ESCA compared with normal samples (29), while Cui et al. concluded that miR-93 could desensitize ESCA to radiotherapy (30). In Shao et al.’s study, miR-144 was proved to inhibit ESCA proliferation and metastasis via inhibiting cyclooxygenase-2 (31). As for miR-191, Gao et al. demonstrated that its up-regulation promoted cell proliferation and invasion and was predictive of a poor prognosis in ESCA (32). All of these studies suggest the reliability of the miRNAs contained in the ceRNA network for the diagnosis and treatment of ESCA.
The DEmRNAs in ESCA tissues were found to be significantly associated with cell activity and energy metabolism. Furthermore, the oxytocin signaling pathway, which has not been reported to be associated with ESCA, was also enriched in the DEmRNAs. Oxytocin has been proposed as a potential therapeutic agent for cancer (33), and the involvement of oxytocin-related proteins in cancer progression has also been evidenced. In Cassoni et al.’s study (34), nonapeptide oxytocin was found to play an important role in regulating cell proliferation and down-regulating the oxytocin receptor, thus indicating potential roles of oxytocin in primary tumors.
Thirty-nine genes in the ceRNA network were found to be significantly associated with ESCA prognosis. High TMTC3 expression was found to be linked to a poor prognosis of ESCA in this study (Figure 4). TMTC3 encodes a protein belonging to the transmembrane and tetratricopeptide repeat-containing protein family, which is mainly expressed in the skin and esophagus. TMTC3 is associated with reticulum stress response and is necessary for bronchial smooth muscle and alveolar myofibroblast development (35,36). No studies to date have focused on TMTC3 in ESCA, and its role in the disease still needs to be explored. Of the 39 prognostic genes in the ceRNA network, FBOX30 was identified as having the most significant association with ESCA prognosis (P=0.00137). FBOX30 encodes a member of F-box protein family and is most highly abundant in the endometrium . Mutation of FBOX30 is associated with the progression of nasopharyngeal carcinoma (37). The results of our study suggest that FBOX30 might also have potential value in ESCA for diagnosis and as a treatment target.
In conclusion, for the first time, we conducted a comprehensive analysis of lncRNA, miRNA, and mRNA expression profiles in ESCA and identified several significant known and novel prognostic biomarkers. Our findings could aid in improving the early diagnosis and treatment of ESCA.
Acknowledgments
Funding: This study was supported by the mandatory project of Nantong Science and Technology Bureau (No.: MS12020027).
Footnote
Reporting Checklist: The authors have completed the REMARK reporting checklist. Available at https://dx.doi.org/10.21037/atm-21-4478
Conflicts of Interest: All authors have completed the ICMJE uniform disclosure form (available at https://dx.doi.org/10.21037/atm-21-4478). The authors have no conflicts of interest to declare.
Ethical Statement: The authors are accountable for all aspects of the work in ensuring that questions related to the accuracy or integrity of any part of the work are appropriately investigated and resolved. The study was conducted in accordance with the Declaration of Helsinki (as revised in 2013). This study was approved by the Ethics Committee of The Affiliated Tumor Hospital of Nantong University (No. 2020811521).
Open Access Statement: This is an Open Access article distributed in accordance with the Creative Commons Attribution-NonCommercial-NoDerivs 4.0 International License (CC BY-NC-ND 4.0), which permits the non-commercial replication and distribution of the article with the strict proviso that no changes or edits are made and the original work is properly cited (including links to both the formal publication through the relevant DOI and the license). See: https://creativecommons.org/licenses/by-nc-nd/4.0/.
References
- Kroep S, Lansdorp-Vogelaar I, Rubenstein JH, et al. An Accurate Cancer Incidence in Barrett's Esophagus: A Best Estimate Using Published Data and Modeling. Gastroenterology 2015;149:577-85.e4; quiz e14-5. [Crossref] [PubMed]
- Xu J, Kang D, Xu M, et al. Multiphoton microscopic imaging of esophagus during the early phase of tumor progression. Scanning 2013;35:387-91. [Crossref] [PubMed]
- Clark RJ, Craig MP, Agrawal S, et al. microRNA involvement in the onset and progression of Barrett's esophagus: a systematic review. Oncotarget 2018;9:8179-96. [Crossref] [PubMed]
- Kaimakliotis PZ, Falk GW. Radiofrequency ablation for Barrett's esophagus. Curr Opin Gastroenterol 2014;30:415-21. [Crossref] [PubMed]
- Hiripi E, Jansen L, Gondos A, et al. Survival of stomach and esophagus cancer patients in Germany in the early 21st century. Acta Oncol 2012;51:906-14. [Crossref] [PubMed]
- Paraskevopoulou MD, Hatzigeorgiou AG. Analyzing MiRNA-LncRNA Interactions. Methods Mol Biol 2016;1402:271-86. [Crossref] [PubMed]
- Liang WC, Fu WM, Wong CW, et al. The lncRNA H19 promotes epithelial to mesenchymal transition by functioning as miRNA sponges in colorectal cancer. Oncotarget 2015;6:22513-25. [Crossref] [PubMed]
- Kallen AN, Zhou XB, Xu J, et al. The imprinted H19 lncRNA antagonizes let-7 microRNAs. Mol Cell 2013;52:101-12. [Crossref] [PubMed]
- Wu XS, Wang F, Li HF, et al. LncRNA-PAGBC acts as a microRNA sponge and promotes gallbladder tumorigenesis. EMBO Rep 2017;18:1837-53. [Crossref] [PubMed]
- Xu F, Zhang J. Long non-coding RNA HOTAIR functions as miRNA sponge to promote the epithelial to mesenchymal transition in esophageal cancer. Biomed Pharmacother 2017;90:888-96. [Crossref] [PubMed]
- Li JY, Ma X, Zhang CB. Overexpression of long non-coding RNA UCA1 predicts a poor prognosis in patients with esophageal squamous cell carcinoma. Int J Clin Exp Pathol 2014;7:7938-44. [PubMed]
- Zang W, Wang T, Huang J, et al. Long noncoding RNA PEG10 regulates proliferation and invasion of esophageal cancer cells. Cancer Gene Ther 2015;22:138-44. [Crossref] [PubMed]
- Zeng Y, Yi R, Cullen BR. MicroRNAs and small interfering RNAs can inhibit mRNA expression by similar mechanisms. Proc Natl Acad Sci U S A 2003;100:9779-84. [Crossref] [PubMed]
- Cesana M, Daley GQ. Deciphering the rules of ceRNA networks. Proc Natl Acad Sci U S A 2013;110:7112-3. [Crossref] [PubMed]
- Qi X, Zhang DH, Wu N, et al. ceRNA in cancer: possible functions and clinical implications. J Med Genet 2015;52:710-8. [Crossref] [PubMed]
- Poliseno L, Pandolfi PP. PTEN ceRNA networks in human cancer. Methods 2015;77-78:41-50. [Crossref] [PubMed]
- Zhang Y, Xu Y, Feng L, et al. Comprehensive characterization of lncRNA-mRNA related ceRNA network across 12 major cancers. Oncotarget 2016;7:64148-67. [Crossref] [PubMed]
- Wang H, Niu L, Jiang S, et al. Comprehensive analysis of aberrantly expressed profiles of lncRNAs and miRNAs with associated ceRNA network in muscle-invasive bladder cancer. Oncotarget 2016;7:86174-85. [Crossref] [PubMed]
- Paci P, Colombo T, Farina L. Computational analysis identifies a sponge interaction network between long non-coding RNAs and messenger RNAs in human breast cancer. BMC Syst Biol 2014;8:83. [Crossref] [PubMed]
- Xia T, Liao Q, Jiang X, et al. Long noncoding RNA associated-competing endogenous RNAs in gastric cancer. Sci Rep 2014;4:6088. [Crossref] [PubMed]
- Diboun I, Wernisch L, Orengo CA, et al. Microarray analysis after RNA amplification can detect pronounced differences in gene expression using limma. BMC Genomics 2006;7:252. [Crossref] [PubMed]
- Sherman BT. DAVID Knowledgebase: a gene-centered database integrating heterogeneous gene annotation resources to facilitate high-throughput gene functional analysis. BMC Bioinformatics 2007;8:426. [Crossref] [PubMed]
- Jeggari A, Marks DS, Larsson E. miRcode: a map of putative microRNA target sites in the long non-coding transcriptome. Bioinformatics 2012;28:2062-3. [Crossref] [PubMed]
- Thomson DW, Bracken CP, Goodall GJ. Experimental strategies for microRNA target identification. Nucleic Acids Res 2011;39:6845-53. [Crossref] [PubMed]
- Su G, Morris JH, Demchak B, et al. Biological network exploration with Cytoscape 3. Curr Protoc Bioinformatics 2014;47:8 13 1-24.
- Shafaee A, Dastyar DZ, Islamian JP, et al. Inhibition of tumor energy pathways for targeted esophagus cancer therapy. Metabolism 2015;64:1193-8. [Crossref] [PubMed]
- Malafaia O. The future of the esophagus cancer. Arq Gastroenterol 2013;50:79-80. [Crossref] [PubMed]
- Khat'kov IE, Izrailov RE, Domrachev SA, et al. Thoracolaparoscopic simultaneous operations on esophagus. Khirurgiia (Mosk) 2014;45-51. [PubMed]
- Ansari MH, Irani S, Edalat H, et al. Deregulation of miR-93 and miR-143 in human esophageal cancer. Tumour Biol 2016;37:3097-103. [Crossref] [PubMed]
- Cui H, Zhang S, Zhou H, et al. Direct Downregulation of B-Cell Translocation Gene 3 by microRNA-93 Is Required for Desensitizing Esophageal Cancer to Radiotherapy. Dig Dis Sci 2017;62:1995-2003. [Crossref] [PubMed]
- Shao Y, Li P, Zhu ST, et al. MiR-26a and miR-144 inhibit proliferation and metastasis of esophageal squamous cell cancer by inhibiting cyclooxygenase-2. Oncotarget 2016;7:15173-86. [Crossref] [PubMed]
- Gao X, Xie Z, Wang Z, et al. Overexpression of miR-191 Predicts Poor Prognosis and Promotes Proliferation and Invasion in Esophageal Squamous Cell Carcinoma. Yonsei Med J 2017;58:1101-10. [Crossref] [PubMed]
- Imanieh MH, Bagheri F, Alizadeh AM, et al. Oxytocin has therapeutic effects on cancer, a hypothesis. Eur J Pharmacol 2014;741:112-23. [Crossref] [PubMed]
- Cassoni P, Marrocco T, Deaglio S, et al. Biological relevance of oxytocin and oxytocin receptors in cancer cells and primary tumors. Ann Oncol 2001;12:S37-9. [Crossref] [PubMed]
- Racapé M, Duong Van Huyen JP, Danger R, et al. The involvement of SMILE/TMTC3 in endoplasmic reticulum stress response. PLoS One 2011;6:e19321 [Crossref] [PubMed]
- Yun EJ, Vu TH. mSmile is necessary for bronchial smooth muscle and alveolar myofibroblast development. Anat Rec (Hoboken) 2012;295:167-76. [Crossref] [PubMed]
- Xiong W, Zeng ZY, Shen SR, et al. Studies on the relationship between D6S1581, a high frequency allele imbalance locus, and genetic susceptibility to nasopharyngeal carcinoma. Zhonghua Yi Xue Yi Chuan Xue Za Zhi 2003;20:311-4. [PubMed]
(English Language Editor: J. Reynolds)