Dysregulation of tumor microenvironment promotes malignant progression and predicts risk of metastasis in bladder cancer
Introduction
Bladder cancer (BCa) is the most common urinary system tumor worldwide, with approximately 380,000 new cases and 150,000 deaths each year (1). While most patients (70–75%) are diagnosed with non-muscle invasive bladder cancer (NMIBC) (2), approximately 25%are diagnosed with muscle-invasive bladder cancer (MIBC), which is more genetically unstable than NMIBC and carries a higher risk of disease progression and metastasis (3). Daizumoto et al. found that in early MIBC cells, binding of nuclear DDX31 to mutp53/SP1 enhances transcriptional activation of mutp53 thereby enhancing MIBC invasion and leading to faster progression of MIBC (4). Therefore, it is increasingly important to find the key genes and related molecular mechanisms for the malignant progression of NMIBC.
The tumor microenvironment (TME) has become a hot spot in tumor study and has been proven to play a key role in the growth and metastasis of tumors (5,6). The TME may vary greatly between tumor types and locations and may change continuously during tumor growth (7), while changes in it can induce the malignant transformation of other non-malignant cells to become highly aggressive and motile (8).
Long non-coding RNAs (lncRNAs) are RNA transcripts ≥200 bp, which can hardly encode proteins. Several studies have shown lncRNAs can affect epigenetics, transcription, and post-transcriptional gene expression, thereby playing a wide range of regulatory roles in the functional activities of cancer cells, including immune response, tumorigenesis, and progression (9). Studies have shown that lncRNA GAS5 can promote the apoptosis of BCa cells by inhibiting EZH2 at the transcription level. At the same time, lncRNA has great research value in the treatment of BCa, such as targeted therapy and chemotherapy resistance (10,11), and is closely related to all stages of tumor immunity, including antigen recognition, antigen exposure, and immune activation (12,13). Therefore, it is very important to identify immune microenvironment-associated lncRNA and to investigate its effect on the malignant progression of BCa.
In this study, the immune microenvironment was assessed using ssGSEA on the expression data of 373 MIBC patients from the TCGA database and combined with the sequencing data of 49 BCa patients in our center to screen for immune lncRNAs associated with the malignant progression of BCa using WGCNA. The TME-related prognostic signature (TMERPS) was subsequently established based on six TME-related lncRNAs. In addition, we further explored the correlation of this signature with the immune system and immune checkpoint inhibitor (ICI) treatment sensitivity and found it was strongly associated with lymph node metastasis and distant metastasis. Finally, the expression of six TME-related lncRNAs was validated at the cellular level, and three nomograms based on TMERPS were established for predicting prognosis, lymph node metastasis, and distant metastasis in BCa patients, respectively.
We present the following article in accordance with the TRIPOD reporting checklist (available at https://dx.doi.org/10.21037/atm-21-4023).
Methods
Extraction of gene and clinical data
We collected 49 BCa specimens (14 cases of MIBC and 35 cases of NMIBC; uroepithelial carcinoma) from patients who underwent transurethral resection of BCa or radical cystectomy in the Shanghai Tenth People’s Hospital from November 2019 to April 2020. All patients provided informed consent in advance. After the collected samples were fixed with formalin and embedded in paraffin, the total RNA was extracted by the RNeasy FFPE kit (Qiagen, Hilden, Germany) after dewaxing, and the paired terminal library then synthesized from 100 ng/mL total RNA using an ABclone all RNA-seq Lib Prep kit. Finally, the sequence data of the sample was obtained using the Illumina NovaSeq 6000 platform. All procedures performed in this study involving human participants were in accordance with the Declaration of Helsinki (as revised in 2013). The study was approved by the ethical standards of Shanghai Tenth People’s Hospital (No: SHSY-IEC-4.1/19-120/01) and informed consent was taken from all the patients.
The transcriptome RNA sequencing datasets (Fragments per kilobase of exon per million reads mapped (FPKM) of 430 patients with BCa (411 cases of tumor tissue and 19 cases of normal tissue; Uroepithelial carcinoma) were download from The Cancer Genome Atlas (TCGA) database (https://cancergenome.nih.gov/). The clinical data of these BCa patients was derived from UCSC (http://genome.ucsc.edu/index.html), and cases that lacked complete data were deleted. The clinicopathological characteristics of the data sets are shown in Table 1.
Table 1
Characteristic | TCGA (n=411) |
---|---|
Subtype | |
Non-muscle invasive bladder cancer (NMIBC) | 35 |
Muscle-invasive bladder cancer (MIBC) | 373 |
Unknown | 3 |
Age | |
≥65 years | 258 |
<65 years | 150 |
Unknown | 3 |
Gender | |
Male | 302 |
Female | 106 |
Unknown | 3 |
Stage | |
I | 2 |
II | 128 |
III | 138 |
IV | 135 |
Unknown | 5 |
Grade | |
Low | 20 |
High | 383 |
Unknown | 8 |
N stage | |
N0 | 236 |
N1–3 | 130 |
Unknown | 45 |
M stage | |
M0 | 398 |
M1 | 10 |
Unknown | 3 |
Immune grouping of samples and validation of grouping
To group transcriptome data from TCGA-derived MIBC patients (T stages from T2 to T4) at the immune level, the ssGSEA function package in the R software genome variation analysis (GSVA) package was used to evaluate the infiltration and function of tumor-infiltrating immune cells (TIICs) subtypes in MIBC samples. To verify the effect of ssGSEA grouping, according to the transcriptional expression profile of MIBC, the immune score, interstitial score, tumor purity, and estimated score were obtained by “ESTIMATE” (R packet), and the related heat map and cluster map were drawn. The composition and infiltration of immunocyte subtypes and activation of the immune response in MIBC samples were determined by the CIBERSORT deconvolution algorithm. The effectiveness of immune grouping was verified by analyzing the expression of human leukocyte antigen (HLA) and programmed cell death protein 1 (PD-L1) in different groups, and the Tumor Immune Dysfunction and Rejection (TIDE) algorithm was applied to assess the predictive validity of TMERPS for ICI response in MIBC (http://tide.dfci.harvard.edu).
Screening of differential expressed mRNAs
The differentially expressed mRNAs between MIBC and NMIBC samples in the BCa sample data of our center and the differentially expressed mRNAs between tumor tissue and normal tissue in the TCGA database were screened using EDGER software (http://bioconductor.org/package/EDGER/). After standardizing the original data with Edger Bioconductor, we analyzed the differences between the two groups of gene expression data, with the limited condition set to log2 |fold change| >1 and error detection rate (false discovery rate, FDR) <0.05. A Venn diagram made by jvenn (http://jvenn.toulouse.inra.fr/app/index.html) was also constructed.
Weighted gene co-expression network approach (WGCNA)
The gene co-expression network was constructed using a “WGCNA” R package v1.69 based on the expression matrix of mRNAs. The gene that was differentially expressed in tumor and normal tissues and closely associated with BCa malignant progression is demonstrated in the Venn diagram. Firstly, gene co-expression similarity was used to construct a Pearson correlation coefficient matrix of all paired genes, and a suitable β value was determined to construct a scale-free network when the degree of independence (R2) was 0.9. Subsequently, the weighted adjacency matrix was transformed into a topological overlap matrix (TOM), which measured the network connectivity of a gene. We used a gene dendrogram with a minimum module size of 50, and some highly similar modules with correlation higher than 0.7 were merged. Finally, genes with similar expression profiles were grouped into gene modules by averaging linkage hierarchical clustering based on the TOM-based difference measure. All genes were represented by the expression of module eigengene (ME) in a specific module, and the module most associated with the TME score (ESTIMATEScore) (|r| =0.82) was selected for further analysis.
Establishment of TME-related lncRNAs signature
To obtain the key prognostic related differentially expressed immune microenvironment-related lncRNAs (DEIRLs), univariate Cox regression analysis of DEIRLs was performed using “survival” R software package with the screening criteria set to P<0.01, and the core prognostic DEIRLs were then identified. Multivariate Cox regression analysis of DEIRLs was carried out with the screening criteria of P<0.01, then a Cox regression model with LASSO was used to reduce noise or redundant genes. The optimal values of the penalty parameter λ were determined through 10 cross-validations and the risk model qs constructed in view of the results. The signature formula is as follows:
In this signature, β represents the regression coefficient of lasso Cox regression, and expLncRNA is the expression level of core prognostic related DEIRLs. Based on the median of risk socre we divided the sample into a high-risk group and a low-risk group.
GEO and KEGG gene sets (v7.2) were downloaded from http://software.broadinstitute.org/gsea/downloads.jsp) using a GSEA3.0 application program, and then gene set enrichment analysis was used to screen out the function and pathway of the Normalized Enrichment Score (NES) >2; FDR <0.05.
Verification of prognostic signature of TME-related lncRNA
Over-fitting between the core prognostic DEIRLs and the lasso COX regression was firstly excluded. According to the median risk score of the MIBC samples derived from TCGA, the samples were divided into a high-risk score group and low-risk score group. Survival analysis was carried out through the R “survival” software package and using log2 (normalized value + 1) data format, and the prognostic value of the two groups of samples was further evaluated. Principal component analysis (PCA) was used to visualize the dimensionality reduction of samples in different groups by “limma” and “scatterplot3d” (R packet). The TIMER database provides 10,897 samples from 32 cancers and provides a wealth of TIICs, including CD4+ T cells, B cells, CD8+ T cells, macrophages, dendritic cells, and neutrophils. We further investigated the relationship between the prognostic signature of immune-related lncRNA (risks core) and TIICs.
Nomogram development and validation
Univariate and multivariate Cox regression analyses were invoked to predict the effect of TMERPS and other clinicopathological characteristics on prognosis and metastasis. Factors that were significant in multivariate Cox regression analysis were subsequently used to create prognostic and metastatic nomograms, which were validated by the consistency index (C-index) and calibration plots using the “Rms” R package v5.1 (https://cran.r-project.org/web/packages/rms/index.html) in R.
Cell culture
Human normal bladder epithelial cell lines SV-Huc-1 and BCa cell lines (T24, J82, and MGH-U3) were derived from the cell bank of the Chinese Academy of Sciences. Human cell carcinoma cell line RPMI-1640 (Gibco; Thermo Fisher Science, Inc.). Middle school training. Contains 10% fetal bovine Fisher Science, Inc. (HyClone; GE Healthcare Life Sciences) and 1% penicillin/streptomycin). SV-Huc-1 cells were cultured in a keratinocyte culture medium (Scientific Cell Research Laboratory, Inc.) using 1% keratinocyte growth supplement (Scientific Cell Research Laboratory, Inc.). All cells were cultured in 37 °C and 5% CO2.
RNA isolation and reverse transcription-quantitative PCR (RT-qPCR)
Total RNA of the cell was isolated by using TRIzol® reagents (Thermo Fisher Scientific, Inc.) referring to the manufacturer’s protocol. SuperScript III reverse transcriptase (Thermo Fisher Scientific, Inc.) was used for reverse transcription, and qPCR was performed by SYBR® Green PCR Master Mix (Thermo Fisher Scientific, Inc.) and a 7900HT fast real-time PCR system. The thermal cycle conditions of the corresponding kit were as follow: 95 °C for 10 minutes, 95 °C for 10 minutes followed by 40 cycles of 95 °C for 10 seconds and at 60 °C for 1 minute. The relative mRNA level was normalized with GAPDH as control, and the results were analyzed using the 2−ΔΔCq method. Primer sequences are shown in Table S1.
Statistical analysis
The Wilcoxon test was used to compare differences in the proportion of TIICs and the expression level of immune response activation between the high-risk score group and low-risk score group. One-way ANOVA or t-test was used to assess the risk scores of patients grouped by clinical characteristics, and Kaplan-Meier and log-rank tests were used to compare disease-specific survival (DSS), progression-free interval (PFI), and over survival (OS) of BCa patients between the low- and high-risk groups. Receiver operating characteristic (ROC) curves were used to investigate the predictive accuracy of risk scores on prognosis, and an independent t-test was used to test the differences between clinical parameters. The index with statistical significance was P<0.05. Principal PCA was used to investigate differences in gene expression patterns between subgroups of BCa samples. The “corrplot” R package v0.84 and “Pheatmap” R package v1.0.12 were used for correlation of matrices and heatmaps, respectively. In addition, SPSS 22.0 (SPSS, Armonk, NY, USA), GraphPad Prism V7 (GraphPad Software, Inc), and R v3.6.1 (https://www.r-project.org/) were used for statistical analysis of the study results. A two-sided P value <0.05 was considered to be significantly different.
Results
Establishment and analysis of TIICs group in MIBC
In this study, 373 MIBC samples were obtained from the TCGA database, and the ssGSEA score was then applied to the transcriptional group of BCa samples to explore the infiltration of various immune cell subtypes in the samples. Furthermore, by using an unsupervised hierarchical clustering algorithm, the MIBC samples were divided into two groups; a low-level group of immune cell infiltration (n=196) and high-level group of immune cell infiltration (n=175) (Figure 1A). Based on the sample transcriptome data, the estimated score, tumor purity, matrix score, and immune score were obtained by estimation algorithm. The results showed that the low-level group of immune cell infiltration had high tumor purity, but higher estimated scores, matrix scores, and immune scores (Figure 1B). A violin map also shows the difference between the two groups. (Figure 1C). In addition, there was a significant difference in the expression of HLA-related genes and PD-L1 between the high-level group of TIICs and the low-level group of immune cell infiltration (Figure 1D,1E). The CIBERSORT method was then used to further verify the effectiveness of the ssGSEA scoring system, and the results showed that the total number of immune cells in the high TIICs group was higher than that in the low TIICs group, and more importantly, the infiltration level of macrophage 1 (M1) in the high-level group of TIICs infiltration was significantly higher than that in the low-level group of TIICs infiltration (P<0.001). Previous studies have shown that M1 is closely related to the invasion and progression of BCa (Figure 1F). The verification results show that the grouping method is meaningful.
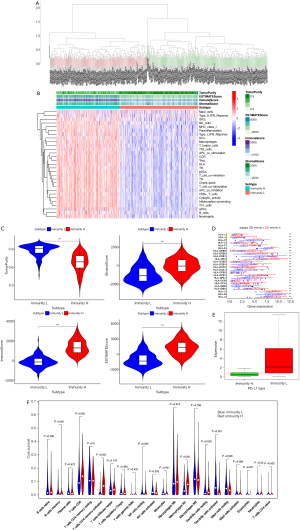
Screening of differentially expressed mRNAs
Based on the filtering criteria of FDR <0.05 and |log2FC| >1, 4,902 differentially expressed genes (DEGs) between BCa samples and normal samples, and 3,239 DEGs between NMIBC and MIBC samples were extracted using the Edger algorithm (Figure 2A,2B). After taking the intersection of these two sets of differential genes using the Venn diagram, 388 genes that were both differentially expressed between MIBC and NMIBC samples and also differentially expressed in BCa and normal tissue samples were obtained (Figure 2C). After KEGG analysis of these 388 genes, the results suggested that “Focal adhesion”, “Fanconi anemia pathway”, “ECM-receptor interaction”, “Primary immunodeficiency”, and “PPAR signaling pathway” were most frequently enriched in the gene set (Figure 2D).
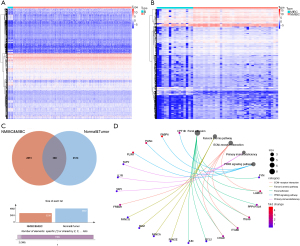
Identification of TME-related lncRNAs
Co-expression analysis was performed for 411 BCa samples in the TCGA dataset. According to the WGCNA method, 395 samples were selected for subsequent analysis after cluster analysis to exclude samples with large differences (Figure 3A). During the power-down analysis, a β value =6 (Free ratio R2 =0.9) was considered the best soft threshold (Figure 3B), and four gene modules were identified (Figure 3C), among which turquoise color module (r=0.87, P<0.001) had the strongest correlation with ImmuneScore (Figure 3D) As the genes in the module were strongly correlated with the module itself (r=0.92; P<0.001) (Figure 3E), this was selected as the most important module. Finally, 166 TME-related mRNAs were extracted from the module, and eight TME-related lncRNAs were obtained by classifying the genes in it.
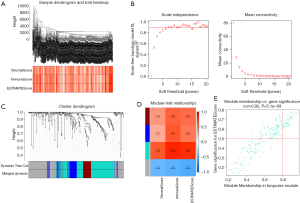
Establishment and verification of TME-related prognostic signature
After integrating the survival data from BCa samples with transcriptomic data, we performed univariate Cox analysis on the integrated dataset (filtering criteria set at P<0.01) to obtain six differentially expressed lncRNAs (Figure 4A). We also performed Lasso regression analysis on these lncRNAs to eliminate intergenic overfitting, and identified six TMEs significantly associated with prognosis related lncRNAs and their corresponding coefficients (Figure 4B,4C). Finally, TMERPS was constructed based on the product of the expression levels of the six genes in the BCa sample transcriptome data and the corresponding LASSO coefficients. The risk score was calculated as follows: [(GATA3-AS1 expression level* − 0.0860650794104812) + (LINC01833 expression level* − 0.0822837147394176) + (AATBC expression level* − 0.164449913716706) + (TRG-AS1 expression level* − 1.62038803393117) + (AL158206.1 expression level* − 0.02743900806555) + (AL390719.2 expression level* − 0.119203625788951)]. Based on the median risk score, 411 BCa samples were divided into a high-risk score group and a low-risk score group, and Kaplan-Meier curves were used to further explore the significance of TMERPS on tumor prognosis. The results showed that the OS (P<0.001) was worse in the high-risk score group than in the low-risk score group (Figure 4D), and the area under the ROC curve (AUC) of the risk score for predicting OS at 1-, 2-, and 3-year was 0.689, 0.708, and 0.723, respectively (Figure 4E). The high-risk score group also had worse DSS (P<0.001) and PFI (P<0.001) than the low-risk fraction group (Figure 4F,4G). The survival curves for the clinical staging subtype samples showed that the genetic model was significant in predicting the prognosis of MIBC (T2–4) patients (P<0.001) but not NMIBC (T0–1) patients (P>0.05) (Figure 4H,4I). The GSEA results against TMERPS showed that the markers of tumor malignancy were the hallmarks of tumors, including cell migration and aggregation; negative regulation of the WNT signaling pathway, and response to inflammatory cytokines. The pathways involved included the transforming growth factor-β (TGF-β) signaling pathway, bladder cancer, and ECM pathway, and these functions and pathways were mainly enriched in high-risk subgroups (Figure 4J,4K).
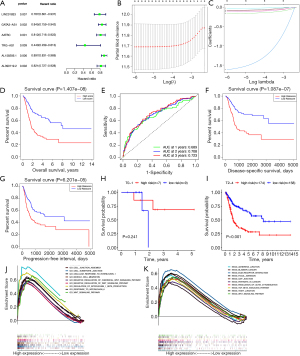
Validation of correlation between TME-related prognostic signature and immune system
The bar graph shows the TIICs in 373 MIBC samples (Figure 5A). The box plot results suggested there was a significant difference in the level of activation of tumor-related immune responses, such as APC- co-stimulation, immune check-point, HLA, T cell-co-stimulation, and Type-I-IFN-Response, between both the high-risk group and low-risk group (P<0.001; Figure 5B). The TIDE algorithm based on MIBC patient transcriptomic data was used to verify whether TMERPS could predict immunotherapy benefit in the TCGA-MIBC cohort. The ICI response results for each patient are shown in Table S2, and the bar graph results show a significant reduction in ICI responders in TMERPS-low risk patients compared to TMERPS-high risk patients (Chi-square test, P=0.031; Figure 5C). The violin plot demonstrates the relationship of immune cell infiltration between the high TMERPS-risk score group and low TMERPS-risk score group, and the results show that B cells native, B cells memory, CD8+T cell, CD4+T cell, CD4+T memory activated, T cells follicular helper, NK cells activated, Monocytes, Macrophages M0, Macrophages M1, Macrophages M2, Dendritic resting, Dendritic resting activated, and Mast cells resting were significantly different between the two groups (P<0.05; Figure 5D). This demonstrates the TMERPS-risk score is positively correlated with immunotherapy response in MIBC patients. In conclusion, TMERPS is closely associated with immune cell infiltration and immune response and has the potential to be used as a predictor of immunotherapy efficacy.
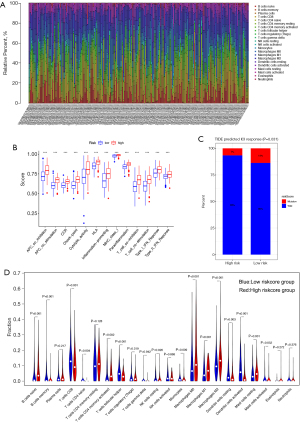
Correlation between TME-related prognostic signature and clinical characteristics
According to the results of PCA, compared with the whole gene dimension, the TME-related prognostic signature could effectively divide the samples into a high-risk score group and low-risk score group (Figure 6A,6B), and the correlation analysis of the TMERPS-risk score with clinical characteristics, such as T/M/N stage, age, and grade, showed that risk scores correlated with the progression of T stage, M stage, STAGE, and N stage (Figure 6C-6F). These results suggested the TMERPS-risk score correlates with the invasion and metastasis of BCa, and guided the next phase of the analysis.
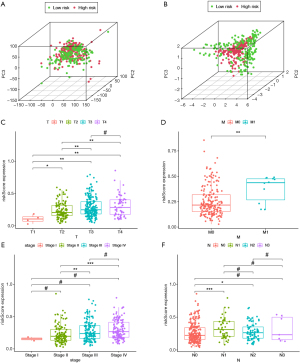
Validation of TME-related prognostic genes expression by in vitro experiments
RT-qPCR was used to validate the expression of the six TME-related prognostic genes GATA3-AS1, LINC01833, AATBC, TRG-AS1, AL158206.1, and AL390719.2 between three human BCa cell lines (T24, U3, and J82) and one human normal bladder epithelial cell line (SV), and the results suggested these six genes were significantly under-expressed in tumor cells (Figure 7).
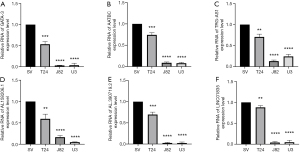
Establishment and validation of OS-related nomograms based on the TMERPS
In the TCGA-BCa cohort, univariate Cox regression analyses demonstrated that age, stage, T-stage, N-stage, and TMERPS-risk score all had prognostic value for OS. These factors were subsequently used in multivariate Cox regression analyses, and the results suggested they remained significantly associated with OS (Figure 8A). Nomograms for OS at 1-, 3-, and 5-year were created based on TMERPS-risks score, age, T stage, and N stage (Figure 8B), and the C-index of the nomogram was 0.71±0.02 (mean ± standard error), indicating that the predicted results were generally consistent with the actual OS of BCa patients. Finally, the calibration plots visually showed that the predicted outcomes of the nomograms were in strong agreement with the actual prognostic outcomes at 1-, 3-, and 5-year (Figure 8C-8E).
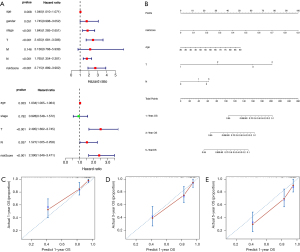
Establishment and validation of metastasis-related nomograms based on the TMERPS
In the TCGA-BCa cohort, univariate Cox regression analysis showed that TMERPS-risk score, grade, T-stage, and age all had prognostic value for the presence or absence of lymph node metastasis. Subsequent use of the above factors in multivariate Cox regression analysis showed that TMERPS-risk score, grade, and T-stage remained significantly associated with the presence of lymph node metastasis (Figure 9A). Nomograms predicting the presence or absence of lymph node metastasis were created based on TMERPS-risk score, grade, and T-stage, respectively (Figure 9B). The C-index of the nomogram associated with lymph node metastasis was 0.74±0.02 (mean ± standard error). The calibration plot visually showed that the predicted results of the lymph node metastasis-related nomogram were consistent with the actual results (Figure 9C). Similarly, based on the results of COX regression analysis, TMERPS-risk score with T-stage was used to construct the nomogram (Figure 9D,9E) to predict distant metastasis, and the C-index of the nomogram associated with distant metastasis was 0.95±0.05 (mean±standard error). Finally, the prediction result of the nomogram associated with distant metastasis was also consistent with the actual result (Figure 9F).
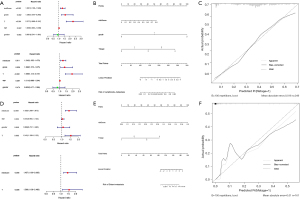
Discussion
MIBC has a poor prognosis, a high risk of lymph node metastasis and distant metastases, and a lack of effective treatment options. Inhibitors of programmed death-ligand 1 (PD-L1)/programmed cell death protein-1 (PD-1) are ICIs of MIBC and can activate the toxicity of immune cells and inhibit the occurrence and development of tumors. While ICIs significantly prolong the survival time and reduce the risk of metastasis of patients with advanced MIBC (14,15), the immunotherapeutic response of MIBC to ICIs is heterogeneous, and the therapeutic effect is difficult to predict. Some investigators have noted that the effect of immunotherapy on MIBC depends on the level of activation of the TME immune response (16,17). However, different TME characteristics have different effects on the tumor. Galon et al. (16) noted that patients with high immune cell infiltration have high levels of immune activation, suggesting that ICI may be effective in these patients and, conversely, patients with low immune cell infiltration are less responsive to ICI. The TME is a complex system composed of immune cells, stromal cells, and microvascular systems, which plays an important role in the occurrence, metastasis, and response to immunotherapy of tumors (18). In studies at the in vivo level, Fantini et al. (19). demonstrated similarities between the N-butyl-N-(4-hydroxybutyl)-nitrosamine (BBN) mouse model and human MIBC, providing strong evidence for subsequent studies of molecular mechanisms and drug discovery studies of MIBC at the in vivo level. Korac-Prlic et al. (20). used the BBN mouse model to demonstrate that IL-6/Stat3 signaling in bladder cancer and gave a rationale for the use of Stat3 inhibitors in human MIBC alone or in combination with anti-PD-L1 and anti-IL-6 therapies as a therapeutic option.
However, at present, the relationship between the TME and the development and immunotherapy of BCa is still unclear.
Current studies have shown that BCa occurs with the involvement of multiple genes with complex pathological mechanisms rather than relying on the involvement of a single gene (21,22), and on this basis, our research has focused on the application of establishing multigene signatures (23,24). The construction of risk models based on the expression levels of immune-related genes for the prediction of prognosis and metastasis as well as the adjustment of immunotherapy strategies is a major research topic (25,26). By combining the TCGA-BCa dataset and our BCa RNA-sequence dataset with differential analysis, WGCNA, Cox regression analysis, Lasso Cox regression analysis, and survival analysis, a TMERPS based on six TME-related prognostic genes differentially expressed between NMIBC and MIBC was established. The results showed that TMERPS had a significant impact on the OS and DSS of BCa patients, and PFI had a significant stratification effect. In addition, the prognosis of the high-risk group was significantly worse than that of the low-risk group. All these results proved that the signature was more effective in predicting the prognosis of MIBC patients.
Follow-up gene function and pathway enrichment analysis showed that the dysregulated expression of these six TME-related prognostic genes was associated with the activity of multiple oncogenic pathways, particularly with extracellular matrix (ECM) components receptor interaction, which is a highly dynamic physiological structure thought to be closely associated with the metastasis and progression of malignancy (27). Imbalance of this structure often accompanies cancer growth and triggers pathological ECM remodeling, which promotes tumor development and metastasis through the induction of pro-fibrotic or integrin-regulated mechanisms (28-32). As important components of the TME, ECM play a central role in tumorigenesis and progression, and an abnormal microenvironment can induce tumor progression (33,34). It has been shown that HER2/neu transformation increases the proportion of immortalized human breast epithelial cells with high CD44/low CD24, giving the cells the stem cell properties required for the EMT initiation process (35). Furthermore, Jeon et al. (36) demonstrated that HER2 expression levels positively correlated with the expression of fibronectin in breast cancer cells, where fibronectin is a major component of the ECM that triggers cell adhesion and cell invasion. And current drug experiments show that rastuzumab or Herceptin can selectively bind to HER2 receptor and inhibit the signaling pathway downstream of this gene. thereby inhibiting the proliferation of tumor cells (37). In addition, malignant features of tumors, including the WNT signaling pathway, TGF-b signaling pathway, intercellular adhesion-related pathways, cancer pathways, and bladder cancer were significantly associated with a subset of high TMERPS scores. Finally, functional analysis for the subgroup with high TMERPS scores suggested that the high scoring group was associated with responses to IL-1 and IL-6, and it was shown that these inflammatory factors, which are closely related to the TME, can influence tumor proliferation, differentiation, and metastasis by chemotactic immune cells or stimulating CRP production (38).
The distribution of TIICs and the activity of the immune system have important implications in immunotherapeutic strategies (39,40). By assessing the response of TIICS to the immune system, we found that TMEPRS correlated with multiple TIICs, with TIMERPS positively correlating with macrophage subtypes and negatively correlating with CD8+ T cells. Macrophages have an immunosuppressive function and have a negative prognostic effect on BCa, whereas CD8+ T cells have significant cancer suppressive effects as tumor-killing T cells. This result side by side supports the finding that TMERPS indicates a negative prognosis for BCa patients (41-43). Furthermore, TMERPS was found to be positively correlated with the activity of the body's immune response, representing a more active immune microenvironment in the high-risk group of tumor patients. The reason behind this activity may be the enhanced tumor immune escape function, which negatively enhances the immune system response. Therefore, TMERPS has the potential to help develop individualized immunotherapy plans for BCa.
Cellular level QPCR results showed that six TME-related prognostic genes were dysregulated in expression between BCa cells and normal bladder epithelium, suggesting that dysregulated expression levels of these genes play an important role in the development of BCa. Zhang et al. (44) reported that GATA3-AS1 promoted the development and metastasis of triple-negative breast cancer by stabilizing tumor immune escape, while Wang et al. (45) found AATBC was closely associated with breast cancer metastasis. Further, Sun et al. (46) found that TGR-AS1 promoted hepatocellular carcinoma invasion and metastasis by regulating the EMT pathway, and Zhang et al. (47) found that LINC01833 promoted EMT function. However, the above genes have not been investigated in BCa, giving direction to our follow-up study.
Having previously demonstrated that TMERPS can well classify BCa patients into subgroups with different immunophenotypes, we combined them with clinical factors to create a new nomogram to predict prognosis, lymph node metastasis, and distant metastasis in BCa patients, respectively. The nomograms were subsequently validated, and the C-index values showed that the prognostic nomograms and lymph node metastasis nomograms were effective in predicting clinical outcomes. The validity of the nomogram model was also confirmed by calibration curve analysis. In contrast, the predictive ability of the distant metastasis nomogram for clinical outcomes was undesirable. Thus, we successfully established models that can effectively predict clinical outcomes of death and lymph node metastasis in BCa patients. Compared to previous prognostic models for bladder cancer, this prediction model uses a novel approach to assess the level of immune microenvironment in Bca samples and uses a more scientific approach to screen key lncRNAs as the basis for modeling the immune risk score. Furthermore, the validation results of TMERPs and Bca immune correlation further explain the better predictive utility of the model and make the model more convincing.
Of course, there are limitations to the current study. First, the immune system is a dynamic and complex system, and simple transcriptome analysis can only reflect certain aspects of an individual’s immune status and is not comprehensive. Second, the molecular regulation of genes has not been further validated in vivo. Third, the prognosis of BCa patients is influenced by multiple factors. The prognostic indicators used in this study do not exclude the influence of other influencing factors, such as metabolism and infection; this study only deals with the role of the immune system. Fourth, some of the samples selected for this study were removed due to incomplete information, resulting in not including all sample sizes in the database, which may have an impact on the experimental results.
Conclusions
In conclusion, our study elucidates the mechanisms underlying the malignant progression of BCa emergence from an immunological perspective. TMERPS established based on six OS-related immune genes, was considered as a signature with some clinical promise, and was shown to be closely associated with prognosis, metastasis, and sensitivity to immunotherapy in BCa patients. Biological functional analysis and cellular level validation against the six lncRNAs comprising TMERPS provided direction for further functional and mechanistic analysis. Subsequently, nomograms established based on TMEPRS were shown to be effective in predicting prognosis, lymph node metastasis, and distant metastasis in BCa patients.
Acknowledgments
The author would like to thank PZ, JZ, KL, and others who have not obtained the qualification of authors for their contributions to the preliminary planning part of this research.
Funding: This work was supported in part by a grant from the National Natural Science Foundation of China (#81472389); Shanghai Science Committee Foundation (#19411967700).
Footnote
Reporting Checklist: The authors have completed the TRIPOD reporting checklist. Available at https://dx.doi.org/10.21037/atm-21-4023
Data Sharing Statement: Available at https://dx.doi.org/10.21037/atm-21-4023
Conflicts of Interest: All authors have completed the ICMJE uniform disclosure form (available at https://dx.doi.org/10.21037/atm-21-4023). The authors have no conflicts of interest to declare.
Ethical Statement: The authors are accountable for all aspects of the work in ensuring that questions related to the accuracy or integrity of any part of the work are appropriately investigated and resolved. All procedures performed in this study involving human participants were in accordance with the Declaration of Helsinki (as revised in 2013). The study was approved by the ethical standards of Shanghai Tenth People’s Hospital (No: SHSY-IEC-4.1/19-120/01) and informed consent was taken from all the patients.
Open Access Statement: This is an Open Access article distributed in accordance with the Creative Commons Attribution-NonCommercial-NoDerivs 4.0 International License (CC BY-NC-ND 4.0), which permits the non-commercial replication and distribution of the article with the strict proviso that no changes or edits are made and the original work is properly cited (including links to both the formal publication through the relevant DOI and the license). See: https://creativecommons.org/licenses/by-nc-nd/4.0/.
References
- Zhu CZ, Ting HN, Ng KH, et al. A review on the accuracy of bladder cancer detection methods. J Cancer 2019;10:4038-44. [Crossref] [PubMed]
- Cassell A, Yunusa B, Jalloh M, et al. Non-Muscle Invasive Bladder Cancer: A Review of the Current Trend in Africa. World J Oncol 2019;10:123-31. [Crossref] [PubMed]
- Stenzl A, Cowan NC, De Santis M, et al. The updated EAU guidelines on muscle-invasive and metastatic bladder cancer. Eur Urol 2009;55:815-25. [Crossref] [PubMed]
- Daizumoto K, Yoshimaru T, Matsushita Y, et al. A DDX31/Mutant-p53/EGFR Axis Promotes Multistep Progression of Muscle-Invasive Bladder Cancer. Cancer Res 2018;78:2233-47. [Crossref] [PubMed]
- Zhang Y, Xu J, Zhang N, et al. Targeting the tumour immune microenvironment for cancer therapy in human gastrointestinal malignancies. Cancer Lett 2019;458:123-35. [Crossref] [PubMed]
- Pan H, Yu T, Sun L, et al. LncRNA FENDRR-mediated tumor suppression and tumor-immune microenvironment changes in non-small cell lung cancer. Transl Cancer Res 2020;9:3946-59. [Crossref]
- Tan HY, Wang N, Lam W, et al. Targeting tumour microenvironment by tyrosine kinase inhibitor. Mol Cancer 2018;17:43. [Crossref] [PubMed]
- Pietilä M, Ivaska J, Mani SA. Whom to blame for metastasis, the epithelial-mesenchymal transition or the tumor microenvironment? Cancer Lett 2016;380:359-68. [Crossref] [PubMed]
- Wei C, Liang Q, Li X, et al. Bioinformatics profiling utilized a nine immune-related long noncoding RNA signature as a prognostic target for pancreatic cancer. J Cell Biochem 2019;120:14916-27. [Crossref] [PubMed]
- Chen X, Xie R, Gu P, et al. Long Noncoding RNA LBCS Inhibits Self-Renewal and Chemoresistance of Bladder Cancer Stem Cells through Epigenetic Silencing of SOX2. Clin Cancer Res 2019;25:1389-403. [Crossref] [PubMed]
- Chen X, Liu M, Meng F, et al. The long noncoding RNA HIF1A-AS2 facilitates cisplatin resistance in bladder cancer. J Cell Biochem 2019;120:243-52. [Crossref] [PubMed]
- Yu WD, Wang H, He QF, et al. Long noncoding RNAs in cancer-immunity cycle. J Cell Physiol 2018;233:6518-23. [Crossref] [PubMed]
- Denaro N, Merlano MC, Lo Nigro C. Long noncoding RNAs as regulators of cancer immunity. Mol Oncol 2019;13:61-73. [Crossref] [PubMed]
- Lobo N, Mount C, Omar K, et al. Landmarks in the treatment of muscle-invasive bladder cancer. Nat Rev Urol 2017;14:565-74. [Crossref] [PubMed]
- Aggen DH, Drake CG. Biomarkers for immunotherapy in bladder cancer: a moving target. J Immunother Cancer 2017;5:94. [Crossref] [PubMed]
- Galon J, Bruni D. Approaches to treat immune hot, altered and cold tumours with combination immunotherapies. Nat Rev Drug Discov 2019;18:197-218. [Crossref] [PubMed]
- Carosella ED, Ploussard G, LeMaoult J, et al. A Systematic Review of Immunotherapy in Urologic Cancer: Evolving Roles for Targeting of CTLA-4, PD-1/PD-L1, and HLA-G. Eur Urol 2015;68:267-79. [Crossref] [PubMed]
- Giraldo NA, Sanchez-Salas R, Peske JD, et al. The clinical role of the TME in solid cancer. Br J Cancer 2019;120:45-53. [Crossref] [PubMed]
- Fantini D, Glaser AP, Rimar KJ, et al. A Carcinogen-induced mouse model recapitulates the molecular alterations of human muscle invasive bladder cancer. Oncogene 2018;37:1911-25. [Crossref] [PubMed]
- Korac-Prlic J, Degoricija M, Vilović K, et al. Targeting Stat3 signaling impairs the progression of bladder cancer in a mouse model. Cancer Lett 2020;490:89-99. [Crossref] [PubMed]
- Song BN, Kim SK, Mun JY, et al. Identification of an immunotherapy-responsive molecular subtype of bladder cancer. EBioMedicine 2019;50:238-45. [Crossref] [PubMed]
- Vidotto T, Nersesian S, Graham C, et al. DNA damage repair gene mutations and their association with tumor immune regulatory gene expression in muscle invasive bladder cancer subtypes. J Immunother Cancer 2019;7:148. [Crossref] [PubMed]
- Bruno TC. New predictors for immunotherapy responses sharpen our view of the tumour microenvironment. Nature 2020;577:474-6. [Crossref] [PubMed]
- Kavalieris L, O'Sullivan P, Frampton C, et al. Performance Characteristics of a Multigene Urine Biomarker Test for Monitoring for Recurrent Urothelial Carcinoma in a Multicenter Study. J Urol 2017;197:1419-26. [Crossref] [PubMed]
- Bao X, Shi R, Zhang K, et al. Immune Landscape of Invasive Ductal Carcinoma Tumor Microenvironment Identifies a Prognostic and Immunotherapeutically Relevant Gene Signature. Front Oncol 2019;9:903. [Crossref] [PubMed]
- Deng X, Lin D, Chen B, et al. Development and Validation of an IDH1-Associated Immune Prognostic Signature for Diffuse Lower-Grade Glioma. Front Oncol 2019;9:1310. [Crossref] [PubMed]
- Krishnaswamy VR, Mintz D, Sagi I. Matrix metalloproteinases: The sculptors of chronic cutaneous wounds. Biochim Biophys Acta Mol Cell Res 2017;1864:2220-7. [Crossref] [PubMed]
- Naba A, Clauser KR, Hoersch S, et al. The matrisome: in silico definition and in vivo characterization by proteomics of normal and tumor extracellular matrices. Mol Cell Proteomics 2012;11:M111.014647.
- Brauchle E, Kasper J, Daum R, et al. Biomechanical and biomolecular characterization of extracellular matrix structures in human colon carcinomas. Matrix Biol 2018;68-69:180-93. [Crossref] [PubMed]
- Luo H, Tu G, Liu Z, et al. Cancer-associated fibroblasts: a multifaceted driver of breast cancer progression. Cancer Lett 2015;361:155-63. [Crossref] [PubMed]
- Calon A, Tauriello DV, Batlle E. TGF-beta in CAF-mediated tumor growth and metastasis. Semin Cancer Biol 2014;25:15-22. [Crossref] [PubMed]
- Das A, Monteiro M, Barai A, et al. MMP proteolytic activity regulates cancer invasiveness by modulating integrins. Sci Rep 2017;7:14219. [Crossref] [PubMed]
- Bissell MJ, Hines WC. Why don't we get more cancer? A proposed role of the microenvironment in restraining cancer progression. Nat Med 2011;17:320-9. [Crossref] [PubMed]
- Coussens LM, Zitvogel L, Palucka AK. Neutralizing tumor-promoting chronic inflammation: a magic bullet? Science 2013;339:286-91. [Crossref] [PubMed]
- Morel AP, Lièvre M, Thomas C, et al. Generation of breast cancer stem cells through epithelial-mesenchymal transition. PLoS One 2008;3:e2888 [Crossref] [PubMed]
- Jeon M, Lee J, Nam SJ, et al. Induction of fibronectin by HER2 overexpression triggers adhesion and invasion of breast cancer cells. Exp Cell Res 2015;333:116-26. [Crossref] [PubMed]
- Molina MA, Codony-Servat J, Albanell J, et al. Trastuzumab (herceptin), a humanized anti-Her2 receptor monoclonal antibody, inhibits basal and activated Her2 ectodomain cleavage in breast cancer cells. Cancer Res 2001;61:4744-9. [PubMed]
- Marnell L, Mold C, Du Clos TW. C-reactive protein: ligands, receptors and role in inflammation. Clin Immunol 2005;117:104-11. [Crossref] [PubMed]
- Sjödahl G, Lövgren K, Lauss M, et al. Infiltration of CD3+ and CD68+ cells in bladder cancer is subtype specific and affects the outcome of patients with muscle-invasive tumors. Urol Oncol 2014;32:791-7. [Crossref] [PubMed]
- Necchi A, Madison R, Raggi D, et al. Comprehensive Assessment of Immuno-oncology Biomarkers in Adenocarcinoma, Urothelial Carcinoma, and Squamous-cell Carcinoma of the Bladder. Eur Urol 2020;77:548-56. [Crossref] [PubMed]
- Schneider AK, Chevalier MF, Derré L. The multifaceted immune regulation of bladder cancer. Nat Rev Urol 2019;16:613-30. [Crossref] [PubMed]
- Kitamura T, Qian BZ, Pollard JW. Immune cell promotion of metastasis. Nat Rev Immunol 2015;15:73-86. [Crossref] [PubMed]
- Xue Y, Tong L. Tumor-infiltrating M2 macrophages driven by specific genomic alterations are associated with prognosis in bladder cancer. Oncol Rep 2019;42:581-94. [Crossref] [PubMed]
- Zhang M, Wang N, Song P, et al. LncRNA GATA3-AS1 facilitates tumour progression and immune escape in triple-negative breast cancer through destabilization of GATA3 but stabilization of PD-L1. Cell Prolif 2020;53:e12855 [Crossref] [PubMed]
- Wang M, Dai M, Wang D, et al. The long noncoding RNA AATBC promotes breast cancer migration and invasion by interacting with YBX1 and activating the YAP1/Hippo signaling pathway. Cancer Lett 2021;512:60-72. [Crossref] [PubMed]
- Sun X, Qian Y, Wang X, et al. LncRNA TRG-AS1 stimulates hepatocellular carcinoma progression by sponging miR-4500 to modulate BACH1. Cancer Cell Int 2020;20:367. [Crossref] [PubMed]
- Zhang Y, Li W, Lin Z, et al. The Long Noncoding RNA Linc01833 Enhances Lung Adenocarcinoma Progression via MiR-519e-3p/S100A4 Axis. Cancer Manag Res 2020;12:11157-67. [Crossref] [PubMed]