Bioinformatics analysis of high frequency mutations in myelodysplastic syndrome-related patients
Introduction
Myelodysplastic syndrome (MDS) is characterized by low hematopoietic function, different degrees of cytopenia, and progression to acute myeloid leukemia (AML) and is a clinically heterogeneous, chronic hematological malignancy (1,2). Development of MDS may occur due through various mechanisms such as environmental exposures to chemicals, various genetic and chromosomal abnormalities and somatic point mutations (3). Identifying potential genetic abnormalities in MDS and combining them with clinical features can promote the correct classification of diseases, aid in developing a prognostic scoring system, and ultimately promote the development of targeted therapy. Due to the rapid recent advances in next generation sequencing (NGS) technologies, there has been a massive influx of data concerning the significance of mutations in the diagnosis and prognostication of MDS. As sequencing data accumulate, in the future, help to establish a primary diagnosis of MDS in cytopenic patients, just as certain specific cytogenetic abnormalities do at the present time.
The emergence of NGS reflects the urgent need for fast, inexpensive, and accurate genomic information (4,5) and can accelerate the research into biology and biomedicine to a great extent. NGS has been widely used in mutation analysis of comprehensive biomarkers (6,7). In the future, NGS can discriminate between MDS and other diseases such as aplastic anaemia, myeloproliferative disorders and idiopathic cytopenias (8). Thus, the structure of cytogenetic data and basic disease characteristics as well as other molecular issues is important for MDS diagnosis. Blood contains many types of biological materials like circulating cells, platelets, extracellular vesicles, mRNA, miRNA, protein, and cell-free DNA (cfDNA) (9). From the blood of cancer patients, a portion of the cfDNA is released by tumor cells through apoptosis, necrosis, or active release (10), and this DNA is called circulating tumor DNA (ctDNA). The half-life of cfDNA in the circulation is between 16 min and 2.5 hours (11). This enables real-time and long-term monitoring of the treatment effect, allowing feasible treatment adjustment and better prognosis.
Since the availability of a wide range of data generated by NGS high-throughput sequencing, Gene Ontology (GO), Kyoto Encyclopedia of Genes and Genomes (KEGG) and protein-protein interaction (PPI) analyses are commonly and widely used in many diseases, such as osteosarcoma (12), AML (13), and diffuse large B-cell lymphoma (DLBCL) (14). Furthermore, PPI analysis has long been used in MDS to identify the pathways for many central genes, which can be used as new targets in treating MDS diseases (15). A recently study have demonstrated the differences of molecular gene mutations between MDS and AML patients, as well as the young and older age groups of MDS and AML patients (16). However, little research has been done when GO, KEGG and PPI methods are used in combination.
In this study, GO, KEGG, and PPI analyses were used mainly to research the mutant genes of MDS-related patients, but also to identify the relationships between the mutation genes, how these relate to clinical features and therefore contribute to a framework for prognosis and treatment which can be used in the clinical setting. We present the following article in accordance with the REMARK reporting checklist (available at https://dx.doi.org/10.21037/atm-21-4094).
Methods
Ethical statement
The study was conducted in accordance with the Declaration of Helsinki (as revised in 2013). The Ethical Committee approved this study of First Affiliated Hospital of Kunming Medical University, Yunnan, China. All patients were required to provide written informed consent.
Study design
Sixty-four patients with MDS-related diseases were admitted to the First Affiliated Hospital of Kunming Medical University between 2017 and 2019 and were enrolled in this study. Among the 64 patients, 32 were diagnosed with MDS, 27 with suspected MDS symptoms, and 5 with metastasis. We analyzed the mutation genes of these three groups of patients before chemotherapy, radiation, or other biological treatments, calculated the mutation frequency, and analyzed the GO, KEGG, PPI, and gene survival curves of mutations in these patients. All patients in this study signed written informed consent.
MICM and positron emission tomography-computed tomography (PET-CT) detection of patients
MICM refers to morphological, immunology, cytogenetical, and molecular biology. In the basic testing protocol, patients were tested for physical signs of disease, routine blood tests, erythrocyte sedimentation rate, and PET-CT, and the results were confirmed by pathologists (17,18). In the morphological protocol, patients were tested by bone marrow biopsy. In the immunology protocol, patients were tested by flow cytometry. In the cytogenetical protocol, karyotype analysis of bone marrow was conducted. In the molecular biological protocol, 32 mutant genes were detected and analyzed by NGS (Illumina Miseq, Santiago, CA, USA), including IDH1, IDH2, TET2, ASXL1, SETBP1, TP53, CBL, FLT3-ITD, NPM1, KIT, DNMT3A, RUNX1, U2AF1, PHF6, NRAS, KRAS, SRSF2, ETV6, MPL, JAK2, EZH2, SF3B1, CEBPA, SH2B3, FBXW7, ATM, FAT1, TNFRSF14, LRP1B, NOTCH1, ZRSR2, and CSF3R.
According to the vendor’s instructions, the NGS sequencing DNA from peripheral blood was extracted by QIAamp DNA Blood Mini Kit (Qiagen Inc., Dusseldorf, Germany). The library was then constructed and validated, and the library’s large-scale parallel cloning and amplification analysis was performed. The sequencing process was then carried out. Quality control (QC) was performed to filter out low-quality reads from the raw FASTQ files. After data comparison, sorting, and de-duplication, the indel region of the gene was re-aligned, and the base quality score recalibrator (BQSR) was carried out immediately. Following any variation detection, variant quality score recalibration (VQSR) was performed.
Bioinformatics method analysis
Bioinformatics methods such as GO, KEGG, and PPI were used to analyze mutant genes and proteins in MDS-related patients. We used two websites for biological analysis, STRING (https://string-db.org) for GO, KEGG, and PPI analyses (19-21), and CBIOPORTAL (http://www.cbioportal.org) for mutation sites and the survival curve of mutant genes (22,23). Genes to query were entered into these two websites, and the results were viewed and collected.
The results of GO analysis can be expressed in three ways: biological process, cellular component, and molecular function. In this paper, we mainly analyzed genes that affect molecular function. According to the false discovery rate (FDR), we selected several items with a smaller FDR (the smaller the FDR, the smaller the error, and the higher the correlation) to analyze the genes commonly involved. A final group of 10 molecular functional items was selected in each group of patients for further analysis. Similarly, there were also 10 items for each group screened by the KEGG pathway analysis. It also uses the principle of small to large FDR concerning correlation. In particular, only two KEGG items were associated with MDS transformation-related patients, as fewer genes were involved.
The information from PPI analysis, gene survival curve, and mutation sites can be obtained by entering the name of each gene in the biological analysis websites used in this study.
Statistical analysis
IBM SPSS statistics software (International Business Machines Statistical Package for the Social Sciences, Version 20.0, IBM Corporation, Armonk, NY, USA) was used to analyze the data. The screening principle used for the data was the value of FDR. The basic screening condition was that the value of FDR could not be greater than 0.05. Since some of the three groups tested include many genes, leading to a large volume of output results, we screened the first 10 for comparative analysis, and these 10 data points were enough for the analysis.
Results
Characteristics of MDS-related patients
There were 64 MDS-related patients enrolled. The types of disease and mutant genes in these patients were analyzed for common findings and trends to provide a prospective basis for future clinical review and treatment.
Diseases types of MDS-related patients
Table 1 shows that 50.00% (32 of 64) of patients were diagnosed as MDS, and 27 patients were suspected of MDS (42.19%). The remaining 5 patients associated with the transformation of MDS included 2 who progressed to acute leukemia (AL), 2 who progressed to AML, and 1 who progressed to acute non-lymphocytic leukemia (ANLL). The proportion of men and women was roughly the same. Epidemiology analysis showed that MDS is usually diagnosed in older patients over the age of 65 (18). In this study, 56.25% of patients aged between 50 and 74.
Table 1
Characteristics | Number (patients) | % (patients) |
---|---|---|
Age (years) | ||
<25 | 5 | 7.81 |
25–49 | 11 | 17.19 |
50–74 | 36 | 56.25 |
≥75 | 12 | 18.75 |
Gender | ||
Female | 26 | 40.63 |
Male | 38 | 59.38 |
Types of disease | ||
MDS | 32 | 50.00 |
Suspected MDS | 27 | 42.19 |
MDS transformation-related | 5 | 7.81 |
MDS, myelodysplastic syndrome.
Gene characteristics of MDS-related patients
We calculated the type and number of mutant genes that appeared in the NGS results for the MDS-related patients, the mutation frequency of each mutant gene, and the mutation thermogram for each patient. The results are outlined in Figure 1, where ASXL1 was the most frequently found mutant gene in MDS-related patients, which was similar to results across the database, and the mutation frequency was 18.75%. The next most frequently found mutant genes after ASXL1 were TP53 and DNMT3A at 8 and 7, respectively, with the mutation frequency of 12.5% and 10.94%, respectively.
Gene characteristics of MDS patients
Figure 2A shows the frequency of the mutant genes in patients with MDS. The mutation frequency for TP53 was the highest (21.88%, 7), followed by ASXL1 (18.75%, 6), and the mutation frequency of DNMT3A, NRAS, and SRSF2 were the same (12.50%, 4). There were 6 genes with a mutation frequency of 3.13%, and these were IDH1, FLT3-ITD, NPM1, KRAS, ETV6, and CEBPA, with the number of mutations being 1.
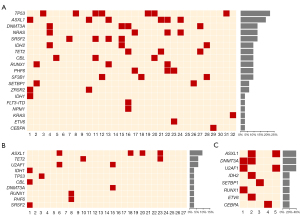
Among the 19 detected mutations, TP53 appeared more frequently alone, while other mutations often accompanied ASXL1. In patients with the ASXL1 mutation, ZRSR2, RUNX1, NARS, and other genes were detected concomitantly. This is also the case for DNMT3A, which is detected with IDH2 and TET2 mutations.
Gene characteristics of suspected MDS patients
Among the 27 suspected MDS patients, the mutation frequency and mutation number of the ASXL1 gene was the highest (14.81%, 4), followed by TET2 and U2AF1 (7.41%, 2). The remaining 7 genes (IDH1, TP53, CBL, DNMT3A, RUNX1, PHF6, and SRSF2) had the same mutation frequency and mutation number (3.7%, 1) (Figure 2B, TET2). The TP53 gene, however, appeared in only one case in suspected MDS patients, and it appeared alone.
Gene characteristics of MDS transformation-related patients
There were 5 MDS-related patients associated with MDS transformation. Figure 2C was derived by counting the mutation frequencies and mutation numbers of these 5 patients. Among the 8 mutation genes detected, the mutation frequencies of ASXL1, DNMT3A, and U2AF1 were the same (40.00%, 2), and IDH2, SETBP1, RUNX1, ETV6, and CEBPA were the same (20.00%, 1). In patients where ASXL1 was detected, DNMT3A, U2AF1, IDH2, and ETV6 were also detected.
GO analysis of MDS-related patients
We used GO analysis to understand the molecular functions of mutation gene, and the results are shown in Table 2. For MDS patients, we selected the first 10 terms with the smallest values according to FDR, including organic cyclic compound binding, heterocyclic compound binding, nucleic acid binding, transcription regulator activity, and other molecular functions primarily involving ASXL1, CEBPA, DNMT3A, ETV6, NPM1, PHF6, RUNX1, SETBP1, SRSF2, and TP53. For the suspected MDS patients, the FDR value of nucleic acid binding, metal ion binding, and transcription regulator activity was 0.00087, which was the lowest value of all the 10 terms, with the most frequent genes being ASXL1, DNMT3A, PHF6, RUNX1, and TP53. Among the terms selected for the 5 MDS transformation-related patients, there were 10 genes involving molecular functions, of which 9 genes had an FDR value less than 0.01, including mainly CEBPA, ETV6, and RUNX1.
Table 2
Term description | Observed gene count | FDR | Matching proteins in your network (labels) |
---|---|---|---|
The molecular function of MDS patients with GO analysis | |||
Organic cyclic compound binding | 17 | 5.4E–07 | ASXL1, CEBPA, DNMT3A, ETV6, IDH1, IDH2, KRAS, NPM1, NRAS, PHF6, RUNX1, SETBP1, SF3B1, SRSF2, TET2, TP53, ZRSR2 |
Heterocyclic compound binding | 17 | 5.4E-07 | ASXL1, CEBPA, DNMT3A, ETV6, IDH1, IDH2, KRAS, NPM1, NRAS, PHF6, RUNX1, SETBP1, SF3B1, SRSF2, TET2, TP53, ZRSR2 |
Nucleic acid binding | 13 | 1.69E–05 | ASXL1, CEBPA, DNMT3A, ETV6, NPM1, PHF6, RUNX1, SETBP1, SF3B1, SRSF2, TET2, TP53, ZRSR2 |
Transcription regulator activity | 11 | 1.69E–05 | ASXL1, CBL, CEBPA, DNMT3A, ETV6, NPM1, PHF6, RUNX1, SETBP1, SRSF2, TP53 |
Isocitrate dehydrogenase (NADP+) activity | 2 | 0.00016 | IDH1, IDH2 |
DNA binding | 10 | 0.00047 | ASXL1, CEBPA, DNMT3A, ETV6, NPM1, PHF6, RUNX1, SETBP1, TET2, TP53 |
DNA-binding transcription factor activity | 8 | 0.0017 | CBL, CEBPA, DNMT3A, ETV6, PHF6, RUNX1, SETBP1, TP53 |
Binding | 18 | 0.0024 | ASXL1, CBL, CEBPA, DNMT3A, ETV6, IDH1, IDH2, KRAS, NPM1, NRAS, PHF6, RUNX1, SETBP1, SF3B1, SRSF2, TET2, TP53, ZRSR2 |
Transcription factor binding | 5 | 0.0031 | ASXL1, CEBPA, NPM1, RUNX1, TP53 |
Kinase binding | 5 | 0.0046 | CBL, CEBPA, NPM1, SRSF2, TP53 |
The molecular function of suspected MDS patients with GO analysis | |||
Nucleic acid binding | 8 | 0.00087 | ASXL1, DNMT3A, PHF6, RUNX1, SRSF2, TET2, TP53, U2AFBP |
Metal ion binding | 9 | 0.00087 | ASXL1, CBL, DNMT3A, IDH1, PHF6, RUNX1, TET2, TP53, U2AFBP |
Transcription regulator activity | 7 | 0.00087 | ASXL1, CBL, DNMT3A, PHF6, RUNX1, SRSF2, TP53 |
Organic cyclic compound binding | 9 | 0.0017 | ASXL1, DNMT3A, IDH1, PHF6, RUNX1, SRSF2, TET2, TP53, U2AFBP |
Heterocyclic compound binding | 9 | 0.0017 | ASXL1, DNMT3A, IDH1, PHF6, RUNX1, SRSF2, TET2, TP53, U2AFBP |
Pre-mRNA binding | 2 | 0.0033 | SRSF2, U2AFBP |
Receptor tyrosine kinase binding | 2 | 0.0073 | CBL, TP53 |
DNA binding | 6 | 0.0074 | ASXL1, DNMT3A, PHF6, RUNX1, TET2, TP53 |
Phosphoprotein binding | 2 | 0.0091 | CBL, PHF6 |
DNA-binding transcription factor activity | 5 | 0.0107 | CBL, DNMT3A, PHF6, RUNX1, TP53 |
The molecular function of MDS transformation-related patients with GO analysis | |||
Nucleic acid binding | 7 | 0.0022 | ASXL1, CEBPA, DNMT3A, ETV6, RUNX1, SETBP1, U2AFBP |
DNA binding | 6 | 0.0022 | ASXL1, CEBPA, DNMT3A, ETV6, RUNX1, SETBP1 |
Organic cyclic compound binding | 8 | 0.0022 | ASXL1, CEBPA, DNMT3A, ETV6, IDH2, RUNX1, SETBP1, U2AFBP |
Transcription regulator activity | 6 | 0.0022 | ASXL1, CEBPA, DNMT3A, ETV6, RUNX1, SETBP1 |
Heterocyclic compound binding | 8 | 0.0022 | ASXL1, CEBPA, DNMT3A, ETV6, IDH2, RUNX1, SETBP1, U2AFBP |
DNA-binding transcription factor activity, RNA polymerase II-specific | 5 | 0.0024 | CEBPA, DNMT3A, ETV6, RUNX1, SETBP1 |
DNA-binding transcription activator activity, RNA polymerase II-specific | 3 | 0.0046 | CEBPA, ETV6, RUNX1 |
RNA polymerase II proximal promoter sequence-specific DNA binding | 3 | 0.0052 | CEBPA, ETV6, RUNX1 |
Transcription factor binding | 3 | 0.0099 | ASXL1, CEBPA, RUNX1 |
Transcription coactivator activity | 2 | 0.0255 | ASXL1, CEBPA |
MDS, myelodysplastic syndrome; GO, Gene Oncology; FDR, false discovery rate.
KEGG analysis of MDS-related patients
KEGG analysis is a comprehensive database resource consisting of 16 main databases, which can be roughly divided into system information, genome information, and chemical information (3). KEGG pathway analysis is usually encoded by location-coupled genes on chromosomes, which is conducive to predicting gene function (24).
We used KEGG analysis to group a large number of differentially expressed genes (DEG) of MDS-related patients to reduce complexity and increase the experiment’s explanatory power by identifying the most affected pathways. The results are shown in Table 3.
Table 3
Term description | Observed gene count | FDR | Matching proteins in your network (labels) |
---|---|---|---|
KEGG analysis of MDS patients | |||
CML | 5 | 8.86E–07 | CBL, KRAS, NRAS, RUNX1, TP53 |
AML | 4 | 2.1E–05 | CEBPA, KRAS, NRAS, RUNX1 |
Central carbon metabolism in cancer | 4 | 2.1E–05 | IDH1, KRAS, NRAS, TP53 |
Pathways in cancer | 6 | 0.00012 | CBL, CEBPA, KRAS, NRAS, RUNX1, TP53 |
Thyroid cancer | 3 | 0.00013 | KRAS, NRAS, TP53 |
Bladder cancer | 3 | 0.00014 | KRAS, NRAS, TP53 |
MicroRNAs in cancer | 4 | 0.00015 | DNMT3A, KRAS, NRAS, TP53 |
Transcriptional misregulation in cancer | 4 | 0.00021 | CEBPA, ETV6, RUNX1, TP53 |
Endometrial cancer | 3 | 0.00025 | KRAS, NRAS, TP53 |
Mitophagy—animal | 3 | 0.00029 | KRAS, NRAS, TP53 |
KEGG analysis of suspected MDS patients | |||
CML | 3 | 0.00048 | CBL, RUNX1, TP53 |
Central carbon metabolism in cancer | 2 | 0.0163 | IDH1, TP53 |
Pathways in cancer | 3 | 0.041 | CBL, RUNX1, TP53 |
MicroRNAs in cancer | 2 | 0.041 | DNMT3A, TP53 |
Herpes simplex infection | 2 | 0.0417 | SRSF2, TP53 |
Transcriptional misregulation in cancer | 2 | 0.0417 | RUNX1, TP53 |
Proteoglycans in cancer | 2 | 0.0417 | CBL, TP53 |
CML | 3 | 0.00048 | CBL, RUNX1, TP53 |
Central carbon metabolism in cancer | 2 | 0.0163 | IDH1, TP53 |
Pathways in cancer | 3 | 0.041 | CBL, RUNX1, TP53 |
KEGG analysis of MDS transformation-related patients | |||
Transcriptional misregulation in cancer | 3 | 0.00058 | CEBPA, ETV6, RUNX1 |
AML | 2 | 0.0026 | CEBPA, RUNX1 |
KEGG, Kyoto Encyclopedia of Genes and Genomes; MDS, myelodysplastic syndrome; FDR, false discovery rate; CML, chronic myeloid leukemia; AML, acute myeloid leukemia.
For MDS patients, the mutant genes can be categorized using 10 terms. The FDR value of all items was less than 0.01, but there were 7 items with FRD values less than 0.00015, which were correlated with chronic myeloid leukemia (CML), AML pathways in cancer, thyroid cancer, bladder cancer, and microRNAs in cancer. The main genes involved were KRAS, NRAS, and TP53.
For the 27 suspected MDS patients, the mutant genes can be divided into 7 pathways. The FDR values of all 7 pathways are less than 0.05, but the FDR values associated with the CML pathway are less than 0.01, that is, 0.00048. The genes involved in this pathway were CBL, RUNX1, and TP53.
For the 5 patients associated with MDS transformation, the mutant genes can be divided into 2 pathways, transcriptional misregulation in cancer and AML, with FDR values of 0.00058 and 0.0026, respectively. The genes involved were CEBPA, ETV6, and RUNX1.
PPI analysis of MDS-related patients
Since proteins affect drug therapy, more than 80% of proteins do not work alone in the body (25). Instead, they interact with other proteins participating in the same cellular process to perform specific cellular tasks and identify drug targets (26). Therefore, it is particularly important to research PPI in diseases.
We performed a PPI analysis of major genes in MDS-related patients, as depicted in Figure 3. Each node (colored circle) in the graph represents a protein, and the lines between nodes represent the interaction between the two proteins.
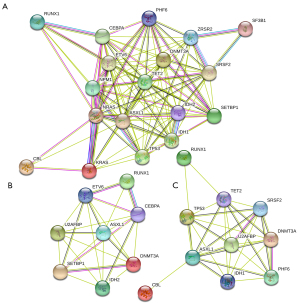
Based on our previous finding on genes with higher mutation frequency in MDS-related patients, we found several proteins interacted strongly. These were NRAS and KRAS, ASXL1 and TP53, and IDH1 and IDH2 in MDS patients. In suspected MDS patients, these were DNMT3A and PHF6, and SRSF2 and U2AFBP. RUNX1 and CEBPA, DNMT3A and SETBP1, and RUNX1 and ETV6 proteins interacted strongly in MDS transformation-related patients.
Key gene mutation sites and survival curve in MDS patients
According to the previous analysis, we screened several key genes in MDS-related patients to figure out the mutation sites and survival curve (Figures 4,5). According to the survival curves, we can conclude that when mutations occur in DNMT3A, ASXL1, RUNX1, and U2AF1, their survival time decreases significantly.
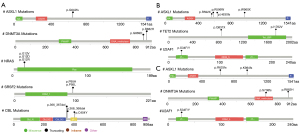
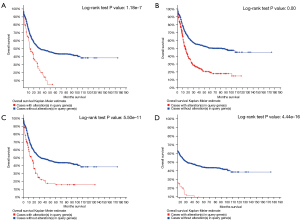
Discussion
MDS, also known as a myelodysplastic disorder, is a chronic, clinical heterogeneous disease manifesting as a persistent decrease in peripheral blood cells and is a challenging bone marrow pathology to manage (27). Relying solely on routine tests to diagnose MDS can lead to delayed diagnosis and treatment of the condition.
The most suitable method to study gene function and regulation of gene expression would be using transcriptomics such as RNA sequencing. However, it is often difficult to directly relate transcriptional information using mutational sequencing information such as data presented in this study. With the advancement of genome sequencing (28), information about gene pathways, mutation processes, and the key factors influencing the fundamental roles of genes has been discovered. Analysis of this data can be achieved by bioinformatics methods such as GO, KEGG, and PPI analysis (13).
The pathophysiology of MDS and its progression to AML involve cytogenetic, genetic, and epigenetic aberrations (29). NGS can simultaneously detect signals of thousands of channels, thus greatly improving efficiency. More and more genetic mutations in MDS patients have been detected and these mutations may serve as potential markers to extend the prognostic parameters in AML. Detailed selection of targeted therapies can help us to explore more about the potential pathways or resistance mechanisms (16). We have found many somatic cell mutations in MDS through NGS, which can be grouped into different functional pathways, such as RNA splicing factors (SF3B1, U2AF1, SRSF2, and ZRSR2) (30), DNA methylation (DNMT3A, TET2, and IDH1/2) (31), histone modification (ASXL1 and EZH2) (32), transcription factors (RUNX1, ETV6, and WT1) (33), and others. Based on the results of gene frequencies and the PPI network, we obtained several key genes with high mutation frequency and strong protein interaction to observe their relationship with disease prognosis, and these were ASXL1, DNMT3A, KRAS, NRAS, TP53, SF3B1, and SRSF2. GO analysis showed that these genes were mainly related to biological life process, in which nucleic acid binding components can participate in gene expression and replication (34), DNA-binding transcription factor activity can regulate cancer stem cell characteristics in liver hepatocellular carcinoma (35), and transcriptional regulatory functioning can react to specific cell signals in response to nutritional status (36). KEGG analysis shows that the detected genes not only play a role in MDS but also participate in the development of many other diseases, such as chronic myelogenous leukemia and acute myelogenous leukemia, which is consistent with the finding that MDS is easily transformed into AML.
In this study, we found that ASXL1 was the most frequent mutant gene among all detected genes. ASXL1 is found in many myeloid malignant tumors, such as AML (37), MDS (32), chronic myelomonocytic leukemia (CMML) (38), and myeloproliferative neoplasms (MPN) (39). Researchers analyzed existing data and found that in most cases of secondary AML with multilineage dysplasia, ASXL1 mutation exists not only in the chronic phase but also in the acute phase in confirmed cases of MDS transformation, inferring that an ASXL1 mutation may be an early mutation gene in leukemia (39). In MDS, the progression time of AML in patients with the ASXL1 mutation was significantly shortened, and it was related to poor prognostic outcomes. Detection of this gene in patients is helpful for clinical risk stratification and treatment planning (40). Moreover, we can see that ASXL1, RUNX1 or NARS, and other genes often appeared concurrently, further illustrating the interaction between genes.
Gene methylation can significantly modify temporal and spatial gene expression leading to change in protein function. In our manuscript, we have addressed that DNMT3A was found to be significantly mutated among MDS patients and such could potentially alter disease related gene expression and protein function. In MDS, past studies have reported that methylation related gene TET2 and IDH1/2 can dramatically change the overall genome methylation patterns and could be used as biomarkers for methylation related diagnostic techniques (41). Interestingly, the overall survival (OS) of patients with the DNMT3A mutation was worse (consistent with our analysis in Figure 5), and the progress was faster than those without the DNMT3A mutation, which may indicate the prognostic value of DNMT3A mutation in new-onset MDS (31), and it was similar to previous studies (42). The literature pointed out that patients with more than two gene mutations (DNMT3A and KRAS/NRAS) had poorer progression-free survival (PFS) rates and OS.
RAS, FLT3, and TP53 genes played an important role in regulating proliferation, differentiation, and apoptosis, and their abnormalities were related to the pathogenesis of MDS (43). In our study, a 21.88% mutation frequency for TP53 was found in MDS patients, which is similar to a previous study (44). Results found that TP53 mutations are highly associated with high risk and type of treatment and are often associated with complex karyotypes. The discovery of a TP53 mutation may lead to a poor prognosis for some hematological malignant tumors such as AML and MDS and may affect the progress of the disease and cause adverse reactions to treatment (45-47). Studies have shown that TP53 mutations can occur several years before the disease progresses and are responsible for the increased risk of leukemia evolution (41-43). Conventional clinical features cannot predict these mutations, and its previously unrecognized heterogeneity may significantly impact clinical decision-making (48). The activation of RAS proto-oncogene is the most common molecular abnormality in MDS (49), and RAS and TP53 frequently occur in AML and MDS pathways, which illustrates their importance in diagnosis and treatment.
SRSF2 was associated with a poor prognosis of MDS (50), which had a mutation frequency of 12.5% in MDS patients in this study, indicating that it could become valuable in future clinical risk stratification and treatment decision-making models. The mutation frequency of SF3B1 was 9.38%. Studies have shown that the SF3B1 mutation was independently associated with better OS and a lower risk of AML evolution (51). Since the SF3B1 mutation is an independent factor that can predict clinical outcomes, incorporating it into a hierarchical system may improve the risk assessment of MDS.
Conclusions
In conclusion, MDS is a group of clonal hematopoietic stem cell diseases and tends to transform into AL. By employing bioinformatics analytical methodology, detecting and monitoring genes with high mutation frequency at the genetic level can prompt the early detection of this disease. This would provide timely clinical guidance on prognosis and treatment and improve survival rates for these patients.
Acknowledgments
We appreciate the staff of the First Affiliated Hospital of Kunming Medical University who participated in this research.
Funding: This work was supported by Yunnan Joint Special Fund Subsidized Projects [2017FE468(-035)], Yunnan Health Science and Technology Project (2018NS0129), and Scientific Research Fund Project of Yunnan Provincial Department of Education (2020J0172).
Footnote
Reporting Checklist: The authors have completed the REMARK reporting checklist. Available at https://dx.doi.org/10.21037/atm-21-4094
Data Sharing Statement: Available at https://dx.doi.org/10.21037/atm-21-4094
Conflicts of Interest: All authors have completed the ICMJE uniform disclosure form (available at https://dx.doi.org/10.21037/atm-21-4094). The authors have no conflicts of interest to declare.
Ethical Statement: The authors are accountable for all aspects of the work in ensuring that questions related to the accuracy or integrity of any part of the work are appropriately investigated and resolved. All procedures performed in this study involving human participants were in accordance with the Declaration of Helsinki (as revised in 2013). The study was approved by ethics board of First Affiliated Hospital of Kunming Medical University. All patients were required to provide written informed consent.
Open Access Statement: This is an Open Access article distributed in accordance with the Creative Commons Attribution-NonCommercial-NoDerivs 4.0 International License (CC BY-NC-ND 4.0), which permits the non-commercial replication and distribution of the article with the strict proviso that no changes or edits are made and the original work is properly cited (including links to both the formal publication through the relevant DOI and the license). See: https://creativecommons.org/licenses/by-nc-nd/4.0/.
References
- Papaemmanuil E, Gerstung M, Malcovati L, et al. Clinical and biological implications of driver mutations in myelodysplastic syndromes. Blood 2013;122:3616-27; quiz 3699. [Crossref] [PubMed]
- Haferlach T, Nagata Y, Grossmann V, et al. Landscape of genetic lesions in 944 patients with myelodysplastic syndromes. Leukemia 2014;28:241-7. [Crossref] [PubMed]
- Dotson JL, Lebowicz Y. Myelodysplastic syndrome. Treasure Island (FL). PMID: 30480932.
- Metzker ML. Sequencing technologies - the next generation. Nat Rev Genet 2010;11:31-46. [Crossref] [PubMed]
- Hussaini M. Biomarkers in hematological malignancies: a review of molecular testing in hematopathology. Cancer Control 2015;22:158-66. [Crossref] [PubMed]
- Au CH, Wa A, Ho DN, et al. Clinical evaluation of panel testing by next-generation sequencing (NGS) for gene mutations in myeloid neoplasms. Diagn Pathol 2016;11:11. [Crossref] [PubMed]
- Bacher U, Kohlmann A, Haferlach T. Mutational profiling in patients with MDS: ready for every-day use in the clinic? Best Pract Res Clin Haematol 2015;28:32-42. [Crossref] [PubMed]
- Tobiasson M, Kittang AO. Treatment of myelodysplastic syndrome in the era of next-generation sequencing. J Intern Med 2019;286:41-62. [Crossref] [PubMed]
- Labib M, Mohamadi RM, Poudineh M, et al. Single-cell mRNA cytometry via sequence-specific nanoparticle clustering and trapping. Nat Chem 2018;10:489-95. [Crossref] [PubMed]
- Stroun M, Lyautey J, Lederrey C, et al. About the possible origin and mechanism of circulating DNA apoptosis and active DNA release. Clin Chim Acta 2001;313:139-42. [Crossref] [PubMed]
- Cabanero M, Tsao MS. Circulating tumour DNA in EGFR-mutant non-small-cell lung cancer. Curr Oncol 2018;25:S38-s44. [Crossref] [PubMed]
- Zhao L, Zhang J, Tan H, et al. Gene function analysis in osteosarcoma based on microarray gene expression profiling. Int J Clin Exp Med 2015;8:10401-10. [PubMed]
- Huang R, Liao X, Li Q. Identification of key pathways and genes in TP53 mutation acute myeloid leukemia: evidence from bioinformatics analysis. Onco Targets Ther 2018;11:163-73. [Crossref] [PubMed]
- Luo B, Gu YY, Wang XD, et al. Identification of potential drugs for diffuse large b-cell lymphoma based on bioinformatics and Connectivity Map database. Pathol Res Pract 2018;214:1854-67. [Crossref] [PubMed]
- Ali A, Junaid M, Khan A, et al. Identification of novel therapeutic targets in myelodysplastic syndrome using protein-protein interaction approach and neural networks. J Comput Sci Syst Biol 2018;11:184-9.
- Yu J, Li Y, Li T, et al. Gene mutational analysis by NGS and its clinical significance in patients with myelodysplastic syndrome and acute myeloid leukemia. Exp Hematol Oncol 2020;9:2. [Crossref] [PubMed]
- Hany TF, Steinert HC, Goerres GW, et al. PET diagnostic accuracy: improvement with in-line PET-CT system: initial results. Radiology 2002;225:575-81. [Crossref] [PubMed]
- Beyer T, Antoch G, Müller S, et al. Acquisition protocol considerations for combined PET/CT imaging. J Nucl Med 2004;45:25S-35S. [PubMed]
- Ding Z, Kihara D. Computational methods for predicting protein-protein interactions using various protein features. Curr Protoc Protein Sci 2018;93:e62 [Crossref] [PubMed]
- André-Grégoire G, Bidère N, Gavard J. Temozolomide affects extracellular vesicles released by glioblastoma cells. Biochimie 2018;155:11-5. [Crossref] [PubMed]
- Yang J, Zhou L, Zhang Y, et al. DIAPH1 is upregulated and inhibits cell apoptosis through ATR/p53/Caspase-3 signaling pathway in laryngeal squamous cell carcinoma. Dis Markers 2019;2019:6716472 [Crossref] [PubMed]
- Gu Y, Zhou JD, Xu ZJ, et al. Promoter methylation of the candidate tumor suppressor gene TCF21 in myelodysplastic syndrome and acute myeloid leukemia. Am J Transl Res 2019;11:3450-60. [PubMed]
- Leroy B, Anderson M, Soussi T. TP53 mutations in human cancer: database reassessment and prospects for the next decade. Hum Mutat 2014;35:672-88. [Crossref] [PubMed]
- Kanehisa M, Goto S, Furumichi M, et al. KEGG for representation and analysis of molecular networks involving diseases and drugs. Nucleic Acids Res 2010;38:D355-60. [Crossref] [PubMed]
- Ogata H, Goto S, Sato K, et al. KEGG: Kyoto Encyclopedia of Genes and Genomes. Nucleic Acids Res 1999;27:29-34.
- Berggård T, Linse S, James P. Methods for the detection and analysis of protein-protein interactions. Proteomics 2007;7:2833-42. [Crossref] [PubMed]
- Pedamallu CS, Posfai J. Open source tool for prediction of genome wide protein-protein interaction network based on ortholog information. Source Code Biol Med 2010;5:8. [Crossref] [PubMed]
- Ma X, Does M, Raza A, et al. Myelodysplastic syndromes: incidence and survival in the United States. Cancer 2007;109:1536-42. [Crossref] [PubMed]
- Hasserjian RP. Myelodysplastic Syndrome Updated. Pathobiology 2019;86:7-13. [Crossref] [PubMed]
- Boultwood J, Dolatshad H, Varanasi SS, et al. The role of splicing factor mutations in the pathogenesis of the myelodysplastic syndromes. Adv Biol Regul 2014;54:153-61. [Crossref] [PubMed]
- Walter MJ, Ding L, Shen D, et al. Recurrent DNMT3A mutations in patients with myelodysplastic syndromes. Leukemia 2011;25:1153-8. [Crossref] [PubMed]
- Gelsi-Boyer V, Trouplin V, Adélaïde J, et al. Mutations of polycomb-associated gene ASXL1 in myelodysplastic syndromes and chronic myelomonocytic leukaemia. Br J Haematol 2009;145:788-800. [Crossref] [PubMed]
- Sperling AS, Gibson CJ, Ebert BL. The genetics of myelodysplastic syndrome: from clonal haematopoiesis to secondary leukaemia. Nat Rev Cancer 2017;17:5-19. [Crossref] [PubMed]
- Berg JM. Potential metal-binding domains in nucleic acid binding proteins. Science 1986;232:485-7. [Crossref] [PubMed]
- Bai KH, He SY, Shu LL, et al. Identification of cancer stem cell characteristics in liver hepatocellular carcinoma by WGCNA analysis of transcriptome stemness index. Cancer Med 2020;9:4290-8. [Crossref] [PubMed]
- Petranovic D, Guédon E, Sperandio B, et al. Intracellular effectors regulating the activity of the Lactococcus lactis CodY pleiotropic transcription regulator. Mol Microbiol 2004;53:613-21. [Crossref] [PubMed]
- Schnittger S, Eder C, Jeromin S, et al. ASXL1 exon 12 mutations are frequent in AML with intermediate risk karyotype and are independently associated with an adverse outcome. Leukemia 2013;27:82-91. [Crossref] [PubMed]
- Cui Y, Tong H, Du X, et al. Impact of TET2, SRSF2, ASXL1 and SETBP1 mutations on survival of patients with chronic myelomonocytic leukemia. Exp Hematol Oncol 2015;4:14. [Crossref] [PubMed]
- Carbuccia N, Murati A, Trouplin V, et al. Mutations of ASXL1 gene in myeloproliferative neoplasms. Leukemia 2009;23:2183-6. [Crossref] [PubMed]
- Thol F, Friesen I, Damm F, et al. Prognostic significance of ASXL1 mutations in patients with myelodysplastic syndromes. J Clin Oncol 2011;29:2499-506. [Crossref] [PubMed]
- Inoue S, Lemonnier F, Mak TW. Roles of IDH1/2 and TET2 mutations in myeloid disorders. Int J Hematol 2016;103:627-33. [Crossref] [PubMed]
- Xu Y, Li Y, Xu Q, et al. Implications of mutational spectrum in myelodysplastic syndromes based on targeted next-generation sequencing. Oncotarget 2017;8:82475-90. [Crossref] [PubMed]
- Fidler C, Watkins F, Bowen DT, et al. NRAS, FLT3 and TP53 mutations in patients with myelodysplastic syndrome and a del(5q). Haematologica 2004;89:865-6. [PubMed]
- Kaneko H, Misawa S, Horiike S, et al. TP53 mutations emerge at early phase of myelodysplastic syndrome and are associated with complex chromosomal abnormalities. Blood 1995;85:2189-93. [Crossref] [PubMed]
- Kita-Sasai Y, Horiike S, Misawa S, et al. International prognostic scoring system and TP53 mutations are independent prognostic indicators for patients with myelodysplastic syndrome. Br J Haematol 2001;115:309-12. [Crossref] [PubMed]
- Ok CY, Patel KP, Garcia-Manero G, et al. TP53 mutation characteristics in therapy-related myelodysplastic syndromes and acute myeloid leukemia is similar to de novo diseases. J Hematol Oncol 2015;8:45. [Crossref] [PubMed]
- Sebaa A, Ades L, Baran-Marzack F, et al. Incidence of 17p deletions and TP53 mutation in myelodysplastic syndrome and acute myeloid leukemia with 5q deletion. Genes Chromosomes Cancer 2012;51:1086-92. [Crossref] [PubMed]
- Jädersten M, Saft L, Smith A, et al. TP53 mutations in low-risk myelodysplastic syndromes with del(5q) predict disease progression. J Clin Oncol 2011;29:1971-9. [Crossref] [PubMed]
- Paquette RL, Landaw EM, Pierre RV, et al. N-ras mutations are associated with poor prognosis and increased risk of leukemia in myelodysplastic syndrome. Blood 1993;82:590-9. [Crossref] [PubMed]
- Thol F, Kade S, Schlarmann C, et al. Frequency and prognostic impact of mutations in SRSF2, U2AF1, and ZRSR2 in patients with myelodysplastic syndromes. Blood 2012;119:3578-84. [Crossref] [PubMed]
- Malcovati L, Papaemmanuil E, Bowen DT, et al. Clinical significance of SF3B1 mutations in myelodysplastic syndromes and myelodysplastic/myeloproliferative neoplasms. Blood 2011;118:6239-46. [Crossref] [PubMed]
(English Language Editors: M. Bucci and J. Chapnick)