An integrated model of FTO and METTL3 expression that predicts prognosis in lung squamous cell carcinoma patients
Introduction
About 30% of non-small cell lung cancer is lung squamous cell carcinoma (LUSC) (1). The 5-year survival rate and the prognosis of the disease is closely related to the stage; stage IV has a 5-year rate of only 2% while stage I (no metastasis) has a survival rate of 70% (2,3). Thus, the early diagnosis is extremely important in LUSC. As N6-methyladenosine (m6A) is the most prevalent messenger RNA (mRNA) modification, it plays a key role in physiological and pathological processes (4,5). As previously reported, the role of m6A in the clinical diagnosis and treatment prediction performance of lung adenocarcinoma (LUAD) has been increasingly attended (6-8). However, the effect of m6A RNA modification in LUSC remains unclear, especially in relation to prognostic stratification.
In this study, a survival analysis was conducted and multi-omics were identified to screen potential biomarkers in LUSC. Ultimately, the training cohort comprised 504 patients from The Cancer Genome Atlas (TCGA), and a two-gene model of risk score and prognostic nomogram was built to assist clinicians to make individualized clinical decisions (see Figure 1). We present the following article in accordance with the TRIPOD reporting checklist (available at https://dx.doi.org/10.21037/atm-21-4470).
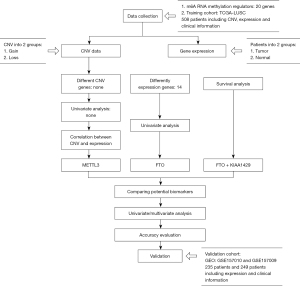
Methods
Participants
20 widely acknowledged m6A RNA methylation regulators were arranged from previous studies. Methyltransferase “writers” include KIAA1429, METTL14, METTL3, RBM15, WTAP, and ZC3H13. “Readers”, such as HNRNPC, RBMX, HNRNPA2B1, YTHDC1, YTHDC2, YTHDF1, YTHDF2, YTHDF3, FMR1, IGF2BP1, IGF2BP2, and IGF2BP3, are involved in RNA processing. “Erasers”, such as ALKBH5 and fat mass- and obesity-associated protein (FTO), are involved demethylation modification (9-12). In this study, we examined their role in the prognosis of LUSC in both a training cohort and a validation cohort.
The mutational profiles and copy number variants (CNVs) of 20 m6A RNA methylation regulators were downloaded from TCGA database. We processed and normalized TCGA level 3 RNA-sequencing (RNA-seq) data, and the fragments per kilobase million (FPKM) values of the exon model from TCGA were used for the gene expression levels. The clinical information of 504 LUSC patients was obtained. The RNA-seq data that was used as the training cohort comprised 504 tumor samples and 49 normal samples. The validation data for FTO and methyltransferase-like 3 (METTL3) expression were obtained from GSE157009 and GSE157010 in the Gene Expression Omnibus (GEO) (http://www.ncbi.nlm.nih.gov/geo/) database. Total RNA data based on GPL570 platforms from squamous cell carcinoma specimens, comprising 249 and 235 patients, were extracted for mRNA profiling and a microarray analysis. The validation data for LUAD were also downloaded from TCGA. To confirm the accuracy of the prognostic model, any patient with missing clinical data was excluded from the training and validation cohorts. The study was conducted in accordance with the Declaration of Helsinki (as revised in 2013).
Differential expression of m6A RNA methylation regulators in LUSC
A comparison of the expression of 19 m6A RNA methylation regulators, excluding RBMX, in the TCGA-LUSC using the “limma” package was performed to identify differentially expressed genes (DEGs) in the 504 tumor samples and 49 normal samples. A cutoff value (P<0.05 and |logFC| >1) was used to select DEGs for mRNA expression. Ultimately, 14 of these genes were identified as DEGs.
Mutation and CNV analysis
The genetic alterations of 20 m6A genes in LUSC were explored in 42 patients with single nucleotide variants (SNVs) and 124 patients with CNVs. We divided the 42 patients into two groups based on each mutational and non-mutational gene. The results of the univariate analysis showed that none of the genes with mutations had any significant relationship with prognosis; however, this was because there were few patients with mutations in each group. A profile of the somatic copy number alteration by GISTIC 2.0 showed that 14q.11.2 was significantly amplified in LUSC patients with METTL3 genes found in a “wide peak” region.
To date, no research appears to have been conducted on whether the high expression of METTL3 is related to CNVs in LUSC; however, the mRNA expression of METTL3 has been shown to be positively correlated with the CNVs in liver hepatocellular carcinoma patients and cancer cell lines (13). We performed Wilcoxon rank-sum tests to analyze CNVs in LUSC, and found that increases in CNVs are correlated with the high expression of METTL3 (P<0.01).
Protein-protein interaction (PPI) network analysis of FTO and METTL3
PPI networks were integrated using the Search Tool for the Retrieval of Interacting Genes (STRING) database (https://string-db.org). Cytoscape software (https://cytoscape.org) was used to establish and visualize the interaction network of FTO and METTL3.
Survival analysis
We sought to examine correlations between genetic alterations and the expression of m6A RNA methylation regulators and prognosis. First, we used the Cox proportional hazards model and R package “survival” and “survminer” to analyze 20 m6A RNA methylation regulators on CNV. The CNV group was divided into gain and loss groups, and Kaplan-Meier curves were generated for each gene. The results revealed that none of the 20 m6A RNA methylation regulators based on CNV were significantly related to prognosis. Next, 20 m6A RNA methylation regulators on expression were screened using the same method outlined above. An analysis of 14 DEGs in relation to the overall survival (OS) curves showed that FTO (P=0.041) and KIAA1429 (P=0.034) were potential prognostic genes (see Figure S1A,S1B).
Risk scores of m6A RNA methylation regulators
Based on the results of the expression and univariate Cox regression analyses, we built a two-gene model of FTO and KIAA1429 to predict prognostic values according to a survival analysis. The minimum criteria determined the regression coefficients. The prognostic risk score of the biomarkers was calculated using the following formula:
where Coefi is the regression coefficient and Xi is the expression of each selected m6A RNA methylation regulator. Based on the coefficients and the minimum criteria of two selected m6A RNA methylation regulators, the regression coefficients were 0.162 and 0.163, respectively. Finally, the two-gene model was constructed to be a prognostic model (P=0.012) as Model-1, which states:
To assess the prediction precision of the prognostic risk-score model, we used a receiver operating characteristic (ROC) curve and calculated the area under the curve (AUC) for 5 years survival (AUC =0.547). The accuracy of FTO and KIAA1249 expression was not satisfactory for prognosis.
Comparing with a biomarker KIAA1249, we took METTL3 into account with FTO expression forming a complex two-gene model. Thus, METTL3 was accepted as a risky gene for evaluating the risk score of the LUSC patients. Integrating two biomarkers into one prognostic risk model could improve the prediction performance of the model.
To investigate the prognostic risk score of the two-gene model, we separated 504 patients from the TCGA-LUSC database into low- and high-risk groups based on median risk scores. The regression coefficients based on the minimum criteria and the coefficients of the two selected m6A RNA methylation regulators were 0.162 and −0.069, respectively. A two-gene model was then constructed to be a prognostic risk-score model (P=0.0023). Model-2 states:
The risk score showed good prediction efficiency with areas under the ROC curve for 3- and 5-year survival in the training cohort of 0.627 and 0.653, respectively, which were higher than those of the FTO and KIAA1429 model and were associated with each other in the PPI network.
As well known, FTO as “erasers” are involved in m6A demethylase modification and METTL3 as “writers” are involved in m6A methylase modification. High FTO expression was associated with a poor prognosis, but high METTL3 expression with associated with a good prognosis. Additionally, as the above study showed, FTO was negatively correlated with METTL3 (P<0.0001). A differential expression analysis showed that FTO was expressed at a significantly low level in tumor samples, but at a high level in normal samples. Conversely, METTL3, a m6A methylase, was not significantly more upregulated relative to normal controls (P>0.05).
Verifying the accuracy of the prognostic model in validation cohort
The prognostic risk-score model was further validated in the independent GSE157010 using the GEO database as the validation cohort. The two-gene model was verified on 235 patients with LUSC from the validation cohort I. A survival analysis was performed, and the AUC was used to evaluate sensitivity and specificity. In order to obtain independent verification, validation cohort II (GSE157009) including 249 patients) was considered into the two-gene model.
Further, the diagnostic risk scores of the two-gene model could be used to diagnose LUAD (mOS: 58.4 months; AUC: 0.54; P<0.05); however, these results were not significant than those for LUSC. Thus, the prognostic risk scores enabled clinicians to predict outcomes for LUSC, but not LUAD. To determine the reason for these different results, FTO and METTL3 expression in LUSC were compared to those in LUAD. There was no significant difference in FTO expression (P=0.7644) between LUSC and LUAD, but the difference in METTL3 expression in LUSC and LUAD were strong and significantly different (P=0.003629). Specifically, a Wilcox test showed that METTL3 expression was higher in LUAD than in LUSC. Thus, METTL3 could have different regulatory mechanisms in LUSC and LUAD.
Statistical analysis
The ‘limma’ package (http://bioinf.wehi.edu.au/limma) was used to identify DEGs in the tumor and normal groups (cutoff: P<0.05 and |logFC| >1). A Spearman correlation analysis was undertaken to examine correlations in gene expression. Wilcoxon rank-sum tests were performed to examine the relationship between CNVs and gene expression. PPI networks were visualized based on the STRING database (https://string-db.org). Univariate and multivariate Cox regression analyses were conducted to evaluate the association between variables with OS in the ‘survival’ R package. The log-rank test was used to compare prognostic values. In this study, the AUC was used as the performance measurement method for the predictive models. AUC was plotted using the ‘survivalROC’ R package. The logistic regression model was built to diagnose in early patients. Using the Cox regression model, the prognostic nomogram for OS was constructed using “regplot” R package, and all statistical tests were performed using R-3.6.3.
Results
Participants
The clinical information of 504 patients in the training cohort was put in order and the clinicopathological features [e.g., age, gender, tumor, node and metastasis (TNM) status, T status, N status, M status and smoking grade] were selected as potential prognostic factors. To confirm the accuracy of the prognostic model, any patient with missing clinical data in the training cohort was excluded, and validation cohort I (235 patients) and validation cohort II (249 patients) were performed to verify the accuracy of the prognostic model, respectively (see Table 1).
Table 1
Variables | TCGA-LUSC (n=504), n (%) | GSE157010 (n=235), n (%) | GSE157009 (n=249), n (%) | TCGA-LUAD (n=515), n (%) |
---|---|---|---|---|
Age (years) | ||||
60 | 387 (76.79) | 34 (14.47) | 37 (14.86) | 157 (30.48) |
≤60 | 108 (21.43) | 201 (85.53) | 212 (85.14) | 339 (65.83) |
Missing# | 9 (1.79) | 19 (3.69) | ||
Gender | ||||
Male | 373 (74.01) | 153 (65.11) | 161 (64.66) | 239 (46.41) |
Female | 131 (25.99) | 82 (34.89) | 88 (35.34) | 276 (53.59) |
TNM stage | ||||
Stage I/II | 408 (80.95) | 204 (86.81) | 231 (92.77) | 397 (77.09) |
Stage III/IV | 92 (18.25) | 31 (13.19) | 18 (7.23) | 110 (21.36) |
Missing# | 4 (0.79) | 8 (1.55) | ||
M status | ||||
M0 | 414 (82.14) | 345 (66.99) | ||
M1 | 7 (1.39) | 166 (32.23) | ||
Missing# | 83 (16.47) | 4 (0.78) | ||
N status | ||||
N0 | 320 (63.49) | 332 (64.47) | ||
N1–N3 | 178 (35.32) | 171 (33.20) | ||
Missing# | 6 (1.19) | 12 (2.33) | ||
T status | ||||
T1–T2 | 409 (81.15) | 446 (86.60) | ||
T3–T4 | 95 (18.85) | 69 (13.40) | ||
Smoking grade | ||||
Grade 1–2 | 152 (30.16) | 195 (37.86) | ||
Grade 3–5 | 340 (67.46) | 306 (59.42) | ||
Missing# | 12 (2.38) | 14 (2.72) |
#, ambiguous variables (missing) was excluded. The clinical features of GSE157010 and GSE157009 were not entire like TCGA database, missing M status, N status, T status and smoking grade. TCGA, The Cancer Genome Atlas; LUSC, lung squamous cell carcinoma; LUAD, lung adenocarcinoma; TNM, tumor node metastasis.
Differential expression and co-expression in LUSC
We found that 14 genes showed significant differential expression (cutoff of P<0.05 and |logFC| >1 by “limma” R package). Among these genes, ALKBH5, METTL14, YTHDC1, YTHDC2, YTHDF2, YTHDF3, ZC3H13, and FTO were lowly expressed in LUSC, while HNRNPA2B1, HNRNPC, IGF2BP1, IGF2BP2, IGF2BP3 and ALKBH5 were highly expressed (see Figure S2). In the Spearman correlation analysis, METTL3 “writers” were negatively correlated with FTO “erasers” (R=−0.17 and P<0.0001 by cBioportal for Cancer Genomics); however, this was not the case in LUAD (R=0.57 and P>0.05).
In relation to the prognostic genes, the expression of 14 m6A RNA methylation regulators of differential expression were analyzed by a univariate Cox regression analysis. However, no gene was found to be related to prognosis. FTO had the lowest P value. Thus, we theorized that FTO, a demethylase for m6A modification, was significantly correlated with prognosis, which was a potential prognostic gene [P=0.069 and hazard ratio (HR) >1]. According to previous studies, FTO, a m6A demethylase, facilitates the progression of lung cancer cells by regulating the m6A level of USP7 mRNA (14). Additionally, it promotes tumor growth in LUSC by regulating MZF1 expression (15). These findings suggest that FTO is a potential biomarker of LUSC.
Correlation between expression, mutation, and CNVs
As few patients had somatic mutations (see Table S1), none of the genes with mutations were associated with gene expression. Based on the CNVs in LUSC, METTL3 was detected to be a significant amplification gene (see Figure 2A). A Wilcoxon rank-sum test showed that the gains of CNV prompts METTL3 high expression (P<0.01) (see Figure 2B). METTL3 (the RNA modification enzyme methyltransferase-like 3) promotes tumorigenesis and metastasis in oral squamous cell carcinoma, and high METTL3 expression is related to poor prognosis (16). The results revealed that the expression of METTL3 mRNA was positively correlated with CNVs. However, recent studies suggest that METTL3 promotes breast cancer cell proliferation in vitro (17). In summary, the gain of CNV might up-regulate high METTL3 expression in LUSC compared with LUAD (see Figure S3).
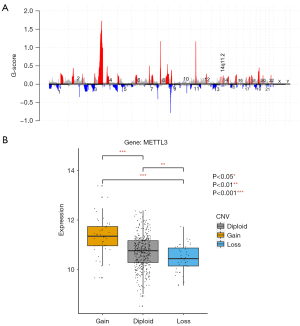
PPI network analysis of FTO and METTL3
Based on the STRING (functional protein association networks) it was predicted that 20 m6A methylation regulators would directly interact with FTO and METTL3 with cutoff: a minimum required interaction score, high confidence (0.70) on-line analysis (string-db.org). The PPI networks of related proteins showed that the METTL3 gene had internal interactions with “writers”, including KIAA1429, METTL14, METTL3, RBM15, WTAP, and ZC3H13, “readers”, including HNRNPC, RBMX, HNRNPA2B1, YTHDC1, YTHDC2, YTHDF1, YTHDF2, YTHDF3, FMR1, and IGF2BP2, and “erasers”, including ALKBH5, and FTO (see Figure 3). Additionally, “writers”, “readers” and “erasers”, particularly FTO and METTL3, had external interactions with each other. We found that METTL3 was strongly associated with KIAA1429, which suggests that METTL3 has the same function as KIAA1429.
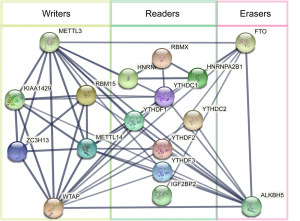
Confirmed the prognostic model
We compared association with OS as well as accuracy of models with different gene combinations in training cohort, The risk score of FTO and METTL3 was stronger associated with OS than FTO and KIAA1429 (P=0.0023 and P=0.012, respectively), and the AUC was also higher than FTO and KIAA1429 (3- and 5-year AUC =0.627, 0.653; AUC =0.530,0.547, respectively) (see Figure 4A-4D). FTO and METTL3 were selected as the optimal combination. After confirming the model, we verified these results in validation cohort I, showing the integrated model of FTO and METTL3 was an optimum selection. The results showed that high-risk patients [median OS (mOS): 47.7 months] had shorter OS than low-risk patients (mOS: 72.0 months; P=0.019). The 3- and 5-year OS AUCs were 0.602 and 0.603, respectively (see Figure 4E,4F). To confirm the model in an independent verification, validation cohort II (249 patients) was considered into the two-gene model, the consequence presented the low-risk group [mOS: 85.9 months] has longer OS than high-risk group (mOS: 61.5 months; P=0.41) (see Table 2).
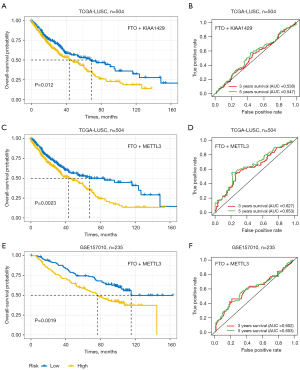
Table 2
Potential biomarkers | Number | Events | Median (months) | 0.95 LCL | 0.95 UCL | P value | AUC | |
---|---|---|---|---|---|---|---|---|
3-year | 5-year | |||||||
FTO + KIAA1429 (TCGA-LUSC) | 0.012 | 0.530 | 0.547 | |||||
Low group | 247 | 92 | 68.8 | 45.9 | 96.1 | |||
High group | 248 | 120 | 43.4 | 32.3 | 60.5 | |||
FTO + METTL3 (TCGA-LUSC) | 0.0023 | 0.627 | 0.653 | |||||
Low group | 246 | 88 | 67.3 | 43.4 | NA | |||
High group | 249 | 124 | 43.4 | 34.1 | 60.5 | |||
FTO + METTL3 (GEO-GSE157010) | 0.0019 | 0.602 | 0.603 | |||||
Low group | 117 | 43 | 72.0 | 65.0 | NA | |||
High group | 118 | 69 | 47.7 | 38.8 | 67.2 | |||
FTO + METTL3 (GEO-GSE157009) | 0.41 | 0.464 | 0.459 | |||||
Low group | 124 | 75 | 85.9 | 63.9 | 102.0 | |||
High group | 125 | 73 | 61.5 | 49.2 | 84.7 | |||
FTO + METTL3 (TCGA-LUAD) | 0.0479 | 0.56 | 0.54 | |||||
Low group | 255 | 76 | 58.4 | 49.2 | 88.1 | |||
High group | 253 | 107 | 41.7 | 36.6 | 50.3 |
Cutoff: P<0.05. LCL, lower confidence limit; UCL, upper confidence limit; AUC, area under the curve; TCGA, The Cancer Genome Atlas; LUSC, lung squamous cell carcinoma; GEO, Gene Expression Omnibus; LUAD, lung adenocarcinoma; CI, confidence interval.
Risk score as an independent prognostic indicator
Risk score was calculated to determine whether or not was an independent prognostic indicator of LUSC. Clinicopathological features including, age, gender, TNM stage, M status, T status, N status and smoking grade, and risk score, were analyzed using a univariate Cox hazard regression analysis. The results indicated that TNM stage, smoking grade, and risk score were significantly correlated with OS. Additionally, the results of the multivariate Cox hazard regression revealed that some potential prognostic factors, including smoking grade [HR: 1.634; 95% confidence interval (CI): 1.038–2.572; P=0.00098] and risk score [HR: 1.699; 95% CI: 1.240–2.3309; P=0.0014] were independent prognostic factors of OS (see Table 3). Thus, the two-gene model of FTO and METTL3 was an independent prognostic risk-score model of OS.
Table 3
Variables | Patients (n=504), n (%) | Univariate analysis | Multivariate analysis | |||
---|---|---|---|---|---|---|
HR (95% CI) | P value | HR (95% CI) | P value | |||
Age (years) | 1.25 (0.881–1.791) | 0.207 | ||||
60 | 387 (76.79) | |||||
≤60 | 108 (21.43) | |||||
Missing# | 9 (1.79) | |||||
Gender | 1.197 (0.871–1.644) | 0.266 | ||||
Male | 373 (74.01) | |||||
Female | 131 (25.99) | |||||
TNM stage | 1.560 (1.135–2.143) | 0.006** | 1.237 (0.780–1.964) | 0.366 | ||
Stage I/II | 408 (80.95) | |||||
Stage III/IV | 92 (18.25) | |||||
Missing# | 4 (0.79) | |||||
M status | 3.065 (1.253–7.500) | 0.014* | 2.316 (0.886–6.054) | 0.086 | ||
M0 | 414 (82.14) | |||||
M1 | 7 (1.39) | |||||
Missing# | 83 (16.47) | |||||
N status | 1.151 (0.872–1.520) | 0.318 | ||||
N0 | 320 (63.49) | |||||
N1–N3 | 178 (35.32) | |||||
Missing# | 6 (1.19) | |||||
T status | 1.648 (1.196–2.270) | 0.0022** | 1.369 (0.858–2.182) | 0.1872 | ||
T1–T2 | 409 (81.15) | |||||
T3–T4 | 95 (18.85) | |||||
Smoking grade | 1.540 (1.161–2.042) | 0.0027** | 1.699 (1.240–2.3309) | 0.00098** | ||
Grade 1–2 | 152 (30.16) | |||||
Grade 3–5 | 340 (67.46) | |||||
Missing# | 12 (2.38) | |||||
FTO (eraser) | 1.175 (0.986–1.400) | 0.069 | ||||
Low | 252 (50.00) | |||||
High | 252 (50.00) | |||||
FTO (CNV) | 0.783 (0.464–1.321) | 0.359 | ||||
Loss | 15 (50.00) | |||||
Gain | 18 (50.00) | |||||
METTL3 (writer) | 0.932 (0.769–1.130) | 0.477 | ||||
Low | 252 (50.00) | |||||
High | 252 (50.00) | |||||
METTL3 (CNV) | 0.917 (0.663–1.268) | 0.601 | ||||
Loss | 43 (50.00) | |||||
Gain | 44 (50.00) | |||||
KIAA1429 (writers) | 1.177 (0.970–1.426) | 0.098 | ||||
Low | 249 (50.30) | |||||
High | 246 (49.70) | |||||
Two-gene model (FTO + METTL) | 1.44 (1.095–1.891) | 0.0089** | 1.634 (1.038–2.572) | 0.0014** | ||
Low | 246 (48.81) | |||||
High | 249 (49.40) |
#, ambiguous variables (missing) were excluded. *, P<0.05; **, P<0.01. HR, hazard ratio; CI, confidence interval; TNM, tumor, node and metastasis; CNV, copy number variant.
Association to clinical characteristics with OS
To increase the overall accuracy, survival analysis was performed on clinical characteristics including age, gender, TNM stage, M status, and T status, N status and smoking grade. Kaplan-Meier plot presented TNM, M status, T status, and smoking grade were strong association with OS (P=0.02; P=0.017; P=0.0098; P=0.0072, respectively) (see Figure S4). Therefore, we constructed a Cox proportional hazards regression model based on risk factors including TNM stage, T status, M status, smoking grade and risk score, and forest plot was drawn to reveal that smoking grade and risk score were significantly higher than other risk factors (P<0.001; P=0.004, respectively) and concordance index (C-index) was 0.6 in internal verification (see Figure 5). Additional, the risk score showed stronger prediction OS in Stage TNM I than other stages (P=0.0011) (see Figure S5), that means it will be one of the ways to assess early prognosis.
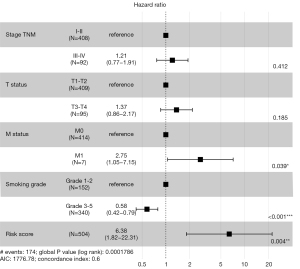
Relation between risk score and early diagnosis
According to the consequence above, we investigated risk score was associated with early diagnosis. The samples were divided into two groups (normal group and tumor group) in TCGA-LUSC, and t-test on risk score of two groups showed quite significant in two groups (P<2.22e−16) (see Figure 6A). Moreover, the logistic regression model was applied to distinguish patients from healthy persons. The AUC was 0.856, meaning strong detection capabilities in diagnosis (see Figure 6B). In a word, this two-gene model can be used as potential markers on both early diagnostic basis and prognostic assessment.
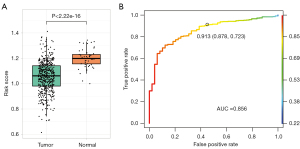
Prognostic nomogram construction
Using a Cox regression model, a prognostic nomogram for 3- and 5-year OS was constructed (see Figure 7). A score was assigned to each score level for each influencing factor (e.g., TNM stage, T status, N status, M status, smoking grade, and risk score) according to the contribution degree of each influencing factor in the model in relation to the outcome variable (the size of the regression coefficient), and a total score was then obtained by summing each score. Finally, the predicted value of an individual’s outcome event was calculated by the function transformation between the total score and the probability of the occurrence of the outcome event. This prognostic nomogram is a potentially valuable tool that can help clinicians make individualized treatment decisions.
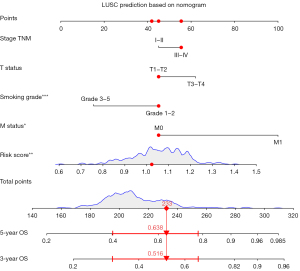
Discussion
Recently, m6A RNA methylation has become a popular epigenetic regulation topic that has been studied in multiple cancers, such as melanoma, leukemia, prostate, breast cancer, and lung cancer (18-20). m6A RNA methylation modification has been clearly shown to be involved in multiple cellular processes, such as the regulation of mRNA methylation and demethylation. However, a prognostic value (i.e., a risk score), which enables clinicians to predict the outcome of LUAD, could not be applied to LUSC. Thus, we investigated potential prognostic biomarkers by mining data from public databases.
In our study, we used two public databases (i.e., the TCGA for LUSC and the GEO for GSE157010 and GSE157009) to create training and validation cohorts. The differential expression of 1 of the 20 mRNA methylation regulators (i.e., FTO) was analyzed and identified by a univariate Cox regression analysis of 504 tumors paired with normal samples. METTL3 was recruited because of the significant correlation between expression and CNVs. Then, a two-gene model for FTO and METTL3 was constructed as a prognostic prediction model using the regression coefficients and based on a minimum criteria. The coefficients of the two selected m6A RNA methylation regulators were 0.162 and −0.069. Based on the correlations of 14 DEGs and “writers” and “erasers”, FTO was found to be negatively correlated with METTL3. These two selected m6A RNA methylation regulators have been previously reported in multiple cancers.
Single-nucleotide polymorphisms (SNPs) in FTO was identified and not associated with body mass index (BMI), but was associated with melanoma risk in the first report in 2013 (21). However, it has been reported that rs9939609 polymorphism in the FTO gene is associated with a risk of breast and prostate cancer (22). Both genetic changes and FTO expression are thought to be involved in the biological process of cancer. As a m6A RNA demethylase, FTO plays a role in carcinogenesis, and its high expression promotes leukemic oncogene-mediated cell transformation and leukemogenesis in acute myeloid leukemia, and promotes cell proliferation and migration in esophageal squamous cell carcinoma via the upregulation of MMP13 (23-25). Similarly, FTO expression is associated with the occurrence and prognosis of gastric cancer and papillary thyroid carcinoma (26). In addition, the regulatory factor of m6A RNA methylation has been shown to promote the malignant progression of LUAD and has clinical diagnostic, progressive and prognostic properties (7,8). FTO induces NELL2 expression, which leads to the metastasis of non-small cell lung cancer via the inhibition of E2F1 m6A modification (27,28) and regulates melanoma tumorigenicity and response to anti-PD-1 blockade (29). Thus, in our study, FTO was identified as a potential prognostic biomarker of LUSC.
In recent years, there has been increasing evidences that METTL3 plays a crucial role in many types of cancers, either independently or dependently of its m6A RNA methyltransferase activity. METTL3 promotes the translation of m6A methyltransferase in human cancer cells, significantly promotes the progression of hepatocellular carcinoma and breast cancer, and occurs and metastases in oral squamous cell carcinoma and pancreatic cancer cell proliferation and invasion through BMI m6A methylation and pancreatic cancer cell proliferation and invasion (30-32). METTL3 also acts as a tumor suppressor in renal cell carcinoma (33,34). RNA m6A methyltransferase METTL3 promotes colorectal cancer by activating the m6A-GLUT1-mTORC1 axis, and is a therapeutic target. METTL3 has been recognized as a potential prognostic prediction biomarker in colorectal cancer (35).
Both LUAD and LUSC are non-small cell lung cancers. The biggest difference between LUAD and LUSC is that it is easy to detect early metastasis in LUAD (1,36). For example, even while the lung lesions are relatively small, there will be brain metastasis, bone metastasis, and even liver metastasis at diagnosis. LUSC can easily lead to the formation of a relatively large mass locally, cause obstructive pneumonia, and is associated with pulmonary hemorrhage and other conditions. However, its rate of growth and metastasis is slow, and it is also sensitive to radiotherapy and chemotherapy. The sensitivity of LUAD to radiotherapy and chemotherapy is worse than that of LUSC, and LUAD is prone to gene mutation, especially epidermal growth factor receptor (EGFR) gene mutation. LUAD can be treated with targeted drugs if there is a mutation in the EGFR gene. Han et al. and Hou et al. demonstrate the de novo transdifferentiation of lung LUAD to LUSC in mice with Lkb1 deficiency and provide mechanistic insight that may have important implications for lung cancer treatment (37,38). Previous studies are explored based on expression level analysis and survival analysis, Gene Ontolog analysis and pathway enrichment to reveal the differences and similarities, reporting 4 potential gene markers including ZWINT, A2M, POLR2H and KIF11 related with miRNA miR-16- 5p (39-41). Based on variants, both of them have different diver genes, gene fusions. The main driving genes in LUAD are EGFR mutation, ALK fusion, ROS-1 fusion, etc., while these in LUSC are PTEN mutation, FGFR1 amplification and 20 common mutated genes including TP53, RB1, ARID1A, CDKN2A, PIK3CA and NF1. Among them, TP53, CDKN2A and PIK3CA mutated more frequently in LUSC than in lung LUAD (42). These differences can lead to different treatment options, such patients could benefit more from nivolumab of immunological therapy in LUSC than LUAD (43). It has been reported in both previous studies and this study that LUSC is strongly linked to smoking (P=0.00098; see Table 3), which the mutation rate is about 15% (3). This rate is significantly lower than that of LUAD in China. Those are the main differences between LUAD and LUSC.
The two-gene model is a prognostic score model in LUSC; however, our study had several disadvantages. First, we reached this conclusion using only public databases and bioinformatics. Thus, there is a bias in the model due to the clinical information bias of TCGA-LUSC. As Table S2 shows, there was no universal rate for 5-year OS in stage I (21.22%); however, a recent report stated that 70% of patients had no metastasis. Additionally, the rate of M0 is 82.14% (414 patients) compared to 1.39% (7 patients) in training cohort. As is well known, clinical information needs to be based on authentic material in clinical research to guarantee the accuracy of the prognostic results. Second, the accuracy of the prognostic risk score of the two-gene model was not high. Indeed, it was not more than 70% in both the training and validation cohorts, but it appeared robust diagnosis in early patients (AUC =0.856). Therefore, larger samples and independent validations are still required to develop the prognostic biomarkers in future studies.
Thus, the regulatory mechanism of m6A modification in LUSC and LUAD is quite unclear in terms of it biology and pathology processes, and further research in laboratory and clinical settings is required.
Acknowledgments
Funding: None.
Footnote
Reporting Checklist: The authors have completed the TRIPOD reporting checklist. Available at https://dx.doi.org/10.21037/atm-21-4470
Conflicts of Interest: All authors have completed the ICMJE uniform disclosure form (available at https://dx.doi.org/10.21037/atm-21-4470). HZ and SL are from Chosen Med Technology (Beijing) Co., Ltd., Beijing, China. The other authors have no conflicts of interest to declare.
Ethical Statement: The authors are accountable for all aspects of the work, including ensuring that any questions related to the accuracy or integrity of any part of the work have been appropriately investigated and resolved. The study was conducted in accordance with the Declaration of Helsinki (as revised in 2013). The data released by TCGA database and GEO database are publicly available, and thus informed patient consent was not required.
Open Access Statement: This is an Open Access article distributed in accordance with the Creative Commons Attribution-NonCommercial-NoDerivs 4.0 International License (CC BY-NC-ND 4.0), which permits the non-commercial replication and distribution of the article with the strict proviso that no changes or edits are made and the original work is properly cited (including links to both the formal publication through the relevant DOI and the license). See: https://creativecommons.org/licenses/by-nc-nd/4.0/.
References
- Chanvorachote P, Chunhacha P. Chapter 4 - Lung cancer metastasis. In: Ahmad A. editor. Introduction to cancer metastasis. Cambridge: Academic Press, 2017:61-76.
- Bray F, Ferlay J, Soerjomataram I, et al. Global cancer statistics 2018: GLOBOCAN estimates of incidence and mortality worldwide for 36 cancers in 185 countries. CA Cancer J Clin 2018;68:394-424. [Crossref] [PubMed]
- Wakelee H, Kelly K, Edelman MJ. 50 years of progress in the systemic therapy of non-small cell lung cancer. Am Soc Clin Oncol Educ Book 2014;177-89. [Crossref] [PubMed]
- Fang X, Li M, Yu T, et al. Reversible N6-methyladenosine of RNA: the regulatory mechanisms on gene expression and implications in physiology and pathology. Genes Dis 2020;7:585-97. [Crossref] [PubMed]
- Zhou Y, Kong Y, Fan W, et al. Principles of RNA methylation and their implications for biology and medicine. Biomed Pharmacother 2020;131:110731 [Crossref] [PubMed]
- Du J, Hou K, Mi S, et al. Malignant evaluation and clinical prognostic values of m6A RNA methylation regulators in glioblastoma. Front Oncol 2020;10:208. [Crossref] [PubMed]
- Zhuang Z, Chen L, Mao Y, et al. Diagnostic, progressive and prognostic performance of m6A methylation RNA regulators in lung adenocarcinoma. Int J Biol Sci 2020;16:1785-97. [Crossref] [PubMed]
- Li F, Wang H, Huang H, et al. m6A RNA methylation regulators participate in the malignant progression and have clinical prognostic value in lung adenocarcinoma. Front Genet 2020;11:994. [Crossref] [PubMed]
- Shi H, Wei J, He C. Where, when, and how: context-dependent functions of RNA methylation writers, readers, and erasers. Mol Cell 2019;74:640-50. [Crossref] [PubMed]
- Zhao W, Xie Y. KIAA1429 promotes the progression of lung adenocarcinoma by regulating the m6A level of MUC3A. Pathol Res Pract 2021;217:153284 [Crossref] [PubMed]
- Ma L, Chen T, Zhang X, et al. The m6A reader YTHDC2 inhibits lung adenocarcinoma tumorigenesis by suppressing SLC7A11-dependent antioxidant function. Redox Biol 2021;38:101801 [Crossref] [PubMed]
- Mendel M, Delaney K, Pandey RR, et al. Splice site m6A methylation prevents binding of U2AF35 to inhibit RNA splicing. Cell 2021;184:3125-42.e25. [Crossref] [PubMed]
- Liu GM, Zeng HD, Zhang CY, et al. Identification of METTL3 as an adverse prognostic biomarker in hepatocellular carcinoma. Dig Dis Sci 2021;66:1110-26. [Crossref] [PubMed]
- Li J, Han Y, Zhang H, et al. The m6A demethylase FTO promotes the growth of lung cancer cells by regulating the m6A level of USP7 mRNA. Biochem Biophys Res Commun 2019;512:479-85. [Crossref] [PubMed]
- Liu J, Ren D, Du Z, et al. m6A demethylase FTO facilitates tumor progression in lung squamous cell carcinoma by regulating MZF1 expression. Biochem Biophys Res Commun 2018;502:456-64. [Crossref] [PubMed]
- Liu L, Wu Y, Li Q, et al. METTL3 promotes tumorigenesis and metastasis through BMI1 m6A methylation in oral squamous cell carcinoma. Mol Ther 2020;28:2177-90. [Crossref] [PubMed]
- Zhang B, Gu Y, Jiang G. Expression and prognostic characteristics of m6A RNA methylation regulators in breast cancer. Front Genet 2020;11:604597 [Crossref] [PubMed]
- Yi L, Wu G, Guo L, et al. Comprehensive analysis of the PD-L1 and immune infiltrates of m6A RNA methylation regulators in head and neck squamous cell carcinoma. Mol Ther Nucleic Acids 2020;21:299-314. [Crossref] [PubMed]
- Sun T, Wu R, Ming L. The role of m6A RNA methylation in cancer. Biomed Pharmacother 2019;112:108613 [Crossref] [PubMed]
- Huang J, Chen Z, Chen X, et al. The role of RNA N 6-methyladenosine methyltransferase in cancers. Mol Ther Nucleic Acids 2021;23:887-96. [Crossref] [PubMed]
- Iles MM, Law MH, Stacey SN, et al. A variant in FTO shows association with melanoma risk not due to BMI. Nat Genet 2013;45:428-32, 432e1.
- Salgado-Montilla JL, Rodríguez-Cabán JL, Sánchez-García J, et al. Impact of FTO SNPs rs9930506 and rs9939609 in prostate cancer severity in a cohort of Puerto Rican men. Arch Cancer Res 2017;5:148. [Crossref] [PubMed]
- da Cunha PA, de Carlos Back LK, Sereia AF, et al. Interaction between obesity-related genes, FTO and MC4R, associated to an increase of breast cancer risk. Mol Biol Rep 2013;40:6657-64. [Crossref] [PubMed]
- Li Z, Weng H, Su R, et al. FTO plays an oncogenic role in acute myeloid leukemia as a N6-methyladenosine RNA demethylase. Cancer Cell 2017;31:127-41. [Crossref] [PubMed]
- Liu S, Huang M, Chen Z, et al. FTO promotes cell proliferation and migration in esophageal squamous cell carcinoma through up-regulation of MMP13. Exp Cell Res 2020;389:111894 [Crossref] [PubMed]
- Hou J, Shan H, Zhang Y, et al. m6A RNA methylation regulators have prognostic value in papillary thyroid carcinoma. Am J Otolaryngol 2020;41:102547 [Crossref] [PubMed]
- Wang Y, Li M, Zhang L, et al. m6A demethylase FTO induces NELL2 expression by inhibiting E2F1 m6A modification leading to metastasis of non-small cell lung cancer. Mol Ther Oncolytics 2021;21:367-76. [Crossref] [PubMed]
- Zhao T, Wang J, Wu Y, et al. Increased m6A modification of RNA methylation related to the inhibition of demethylase FTO contributes to MEHP-induced Leydig cell injury☆. Environ Pollut 2021;268:115627 [Crossref] [PubMed]
- Yang S, Wei J, Cui YH, et al. m6A mRNA demethylase FTO regulates melanoma tumorigenicity and response to anti-PD-1 blockade. Nat Commun 2019;10:2782. [Crossref] [PubMed]
- Zeng C, Huang W, Li Y, et al. Roles of METTL3 in cancer: mechanisms and therapeutic targeting. J Hematol Oncol 2020;13:117. [Crossref] [PubMed]
- Xia T, Wu X, Cao M, et al. The RNA m6A methyltransferase METTL3 promotes pancreatic cancer cell proliferation and invasion. Pathol Res Pract 2019;215:152666 [Crossref] [PubMed]
- Du M, Zhang Y, Mao Y, et al. MiR-33a suppresses proliferation of NSCLC cells via targeting METTL3 mRNA. Biochem Biophys Res Commun 2017;482:582-9. [Crossref] [PubMed]
- Zhou J, Wang J, Hong B, et al. Gene signatures and prognostic values of m6A regulators in clear cell renal cell carcinoma - a retrospective study using TCGA database. Aging (Albany NY) 2019;11:1633-47. [Crossref] [PubMed]
- Zhang QJ, Luan JC, Song LB, et al. m6A RNA methylation regulators correlate with malignant progression and have potential predictive values in clear cell renal cell carcinoma. Exp Cell Res 2020;392:112015 [Crossref] [PubMed]
- Chen H, Gao S, Liu W, et al. RNA N6-methyladenosine methyltransferase METTL3 facilitates colorectal cancer by activating the m6A-GLUT1-mTORC1 axis and is a therapeutic target. Gastroenterology 2021;160:1284-300.e16. [Crossref] [PubMed]
- Xie S, Wu Z, Qi Y, et al. The metastasizing mechanisms of lung cancer: Recent advances and therapeutic challenges. Biomed Pharmacother 2021;138:111450 [Crossref] [PubMed]
- Han X, Li F, Fang Z, et al. Transdifferentiation of lung adenocarcinoma in mice with Lkb1 deficiency to squamous cell carcinoma. Nat Commun 2014;5:3261. [Crossref] [PubMed]
- Hou S, Zhou S, Qin Z, et al. Evidence, mechanism, and clinical relevance of the transdifferentiation from lung adenocarcinoma to squamous cell carcinoma. Am J Pathol 2017;187:954-62. [Crossref] [PubMed]
- Sun F, Yang X, Jin Y, et al. Bioinformatics analyses of the differences between lung adenocarcinoma and squamous cell carcinoma using The Cancer Genome Atlas expression data. Mol Med Rep 2017;16:609-16. [Crossref] [PubMed]
- Anusewicz D, Orzechowska M, Bednarek AK. Lung squamous cell carcinoma and lung adenocarcinoma differential gene expression regulation through pathways of Notch, Hedgehog, Wnt, and ErbB signalling. Sci Rep 2020;10:21128. [Crossref] [PubMed]
- Dong A, Wang ZW, Ni N, et al. Similarity and difference of pathogenesis among lung cancer subtypes suggested by expression profile data. Pathol Res Pract 2021;220:153365 [Crossref] [PubMed]
- Zengin T, Önal-Süzek T. Comprehensive profiling of genomic and transcriptomic differences between risk groups of lung adenocarcinoma and lung squamous cell carcinoma. J Pers Med 2021;11:154. [Crossref] [PubMed]
- Zhang YQ, Yuan Y, Zhang J, et al. Evaluation of the roles and regulatory mechanisms of PD-1 target molecules in NSCLC progression. Ann Transl Med 2021;9:1168. [Crossref] [PubMed]
(English Language Editor: L. Huleatt)