A narrative review of prognosis prediction models for non-small cell lung cancer: what kind of predictors should be selected and how to improve models?
Introduction
Although the status of the most common cancers has changed, lung cancer remains the leading cause of cancer-related death in the world, with a mortality of 22% in male and 13.8% in female in 2018 (1-3). The 5-year relative survival rate for lung cancer is also poor and is estimated at 21% in 2021 for all stages combined (1). According to the WHO classification, lung cancer is histologically divided into two subtypes: non-small cell lung cancer (NSCLC) and small cell lung cancer (SCLC), with the former accounting for 85% and the latter for 15% (4). In recent years, therapy for NSCLC has progressed with the rise of targeted therapy and immune therapy, and personalized treatment. Emphasized by The American Joint Committee on Cancer, this therapeutic approach has increased the importance of prognostic prediction to assist clinicians in making the most effective treatment plan for patients (5,6). At present, the prognosis prediction for NSCLC is based on tumor, node, and metastasis (TNM) staging system (7), which stratifies patients to four stages according to clinical and pathological characteristics. However, the staging system is too broad to make a prognosis prediction for treatment guidance.
A clinical prediction model, also known as a “clinical prediction rules” or “risk scores”, is a tool incorporating multiple predictors to predict the risk of some event (8) which has been developed explosively in recent years and has been applied to the detection, diagnosis, and prognosis of NSCLC. The model contains two sub-types: the diagnostic model and the prognostic model. The diagnostic model focuses on estimating the risk of developing a disease based on the epidemiological and clinical features of patients. Some guidelines, such as the National Comprehensive Center Network (NCCN) guidelines and the United States Preventive Services Task Force (USPSTF) (9,10), have recommended or applied some risk prediction models to the detection of NSCLC. The prognostic model focuses on the risk of disease recurrence, death, disability, and complications in the current state of the disease. An increasing number of prognostic predictors have been found to have potential for clinical application, and numerous models have been developed and validated. Compared to the TNM staging system, a prognosis prediction model can improve the accuracy of and guide personalized therapy through the combination of multiple prognostic factors.
Since the 1980s, many large cohort studies (11-13) have been performed to develop and validate risk prediction models for the screening of NSCLC because of their high effectiveness compared to the NLST criteria (14). However, there is a lack of research on prognosis prediction models for NSCLC in large cohort studies, and the small-size sample of existing models may cause a reduction of persuasiveness.
Although there are many studies on NSCLC, there are few reviews on clinical prediction models of NSCLC, which focus on the methodology of model establishment. This paper focuses on the selection of predictors and the control of model bias to provide more ideas and suggestions for modelers. In this article, we reviewed existing lung cancer prediction models with an emphasis on those with utility for prognosis, analyzed the urgent problems to be solved in the field, and suggest novel approaches to the construction of models. The focus of this article is to inform readers of the kind of predictors which should be selected to build particular models at a time when greater attention is being paid to this method.
We present the following article in accordance with the Narrative Review reporting checklist (available at https://dx.doi.org/10.21037/atm-21-4733).
Methods
PubMed and Cochrane Library were searched using the items “NSCLC”, “prognostic model”, “prognosis prediction”, “survival prediction”, and “PROBAST” from 1 January 1980 to 5 May 2021. Reference lists from articles were reviewed and relevant articles were identified. Non-English language articles and abstracts were excluded.
Screening of predictors
Screening of the predictors is fundamental to the development of prediction models. The predictive value of several classic predictors, such as the TNM staging system, WHO-PS/ECOG-PS, and pathological classification has been widely validated (4,5,15,16). These traditional predictors have been included as the classification standard of prognosis stratification and treatment decision making by guidelines and expert consensus (17,18). Hence, we consider these factors should be routinely incorporated in the prognostic prediction models of NSCLC.
The screening, diagnosis, and treatment of cancers has been promoted to the molecular genetic stage, benefiting from the development of high-throughput techniques such as next-generation sequencing and microarray (19-21). The classification of lung cancer has been further sub-classified from pathological classification to molecular classification based on driver genes (4). The treatment of lung cancer has developed to a comprehensive treatment based on pathological classification, stage, and molecular classification. The extraordinary development and application of genomics and epigenomics is defining reproducible and scalable prediction models (22), and increasingly, modelers have incorporated gene-related biomarkers obtained from the aspect of genomics and epigenomics such as the expression of DNA/RNA, somatic mutations, and DNA methylation. Accordingly, we have conducted a special review and summary in this aspect. On the other hand, the progress of prediction models depends on the discovery of innovative predictors, and we have summarized some factors that have not been incorporated or have been rarely used in models which we believe may hold some prediction value.
Predictors that should be incorporated routinely
TNM staging system
The TNM staging system involves the combination of three important factors, and most prognostic models would take this system into consideration when screening for predictors. Although some models may only include one component, such as T stage or number of positive lymph node stations, almost all research involving univariate and multi-variate analysis in the field of NSCLC incorporates the TMN system (23-26).
Oberije et al. developed a survival prediction model with a dataset of 548 stage III NSCLC patients (23). After the predictor screening, they constructed a multi-variates model (MV model) consisting of age, gender, number of positive lymph node stations, gross tumor volume, and three other factors. Compared to making survival prediction with only TNM staging, the new MV model had better discrimination (C-statistic: 0.62 vs. 0.57).
She et al. developed a deep learning model with a training data of 12,912 samples which incorporated 127 predictors including the TNM staging system (26). At the same time, they developed a model only including TNM staging system, and the results showed that the performance of the deep learning model was better than the TNM model in C-statistics (0.739 vs. 0.706).
WHO-PS
WHO-PS is the Eastern Cooperative Oncology Group Performance Status Scale (ECOG-PS), which is mainly used to assess the functional status of cancer patients from the clinical manifestation of tumors, patient activity, and the proportion of time in bed. Although WHO-PS is a subjective factor that would, relatively speaking, cause a heterogeneous group, it is increasingly applied to prediction models of cancer (23,27).
Many researchers have suggested that WHO-PS 2 is a precise indicator of a bad prognosis (16,28), and for patients whose WHO-PS ≥3, guidelines recommend the best supportive treatment, which most researchers take into exclusion criteria (28,29).
Prelaj et al. (27) conducted a retrospective study of 154 patients with advanced NSCLC receiving immunotherapy to find prognostic predictors and develop an effective prognostic scoring system. The study highlighted the negative prognostic role for OS and PFS of WHO-PS. The researchers divided patients into an WHO-PS 0–1 group and WHO-PS 2 group. The results of the multi-variates analysis suggested that WHO-PS had the worst prognostic significance for OS (HR =4.85, CI: 2.87–8.20) and PFS (HR =2.20, CI: 1.46–3.131) among the five variables finally incorporated, indicating patients with a lower WHO-PS might be less likely to be benefit from immunotherapy, possibly due to their poorer immune function.
While WHO-PS may be a very expectant predictor, both before and after treatment, there are some defects in its application. Many studies have highlighted the subjectivity and variability of WHO-PS due to the evaluator (30-32), and the question of how to standardize its evaluation needs to be solved.
Pathological classification
The pathological classification of lung cancer has been increasingly detailed with the development and popularization of immunotherapy and targeted therapy. The 2015 WHO classification of lung tumors provides more information on improving outcomes in comparison to the 2004 version, and at present, almost all clinical prediction models of lung cancer incorporate pathological classification or use it as a grouping factor to make a subgroup analysis.
However, the influence of the proportion of various subtypes on prognosis, such as whether the proportion of micropapillary and solid pattern is correlated with prognosis, is of great concern (33). It has been widely shown that the proportion of micropapillary is an independent prognosis predictor for OS and was associated with poor outcome (34-36), and some studies demonstrated that the presence or absence of micropapillary pattern is a poor prognostic factor. In cases where the micropapillary proportion is only 1%, the prognosis of patients is worse than that of patients without micropapillary (37,38). This suggests that whether as a dichotomous variable or continuous variable, micropapillary pattern can be incorporated into models as a very good predictor. Additionally, ground-glass opacification (GGO) of lung and spread of tumor through alveolar spaces (STAS) are both potential predictors to be considered (39,40).
The histological classification of squamous cell carcinoma is less specific than that of adenocarcinoma (41). According to the new WHO pathological classification, the former is only classified into keratosis and non-keratosis, which is not significant for prognostic prediction. Therefore, more study of the histological classification of squamous cell carcinoma is required to determine its prognosis and treatment value.
Biomarker roles of gene-related information in lung cancer prognosis and their practical applications in models
Genomic biomarkers for NSCLC prognosis
Genomics has played a significant part in the prognosis and treatment management of patients with NSCLC by clarifying the role of driver genes and providing information on mutation and gene expression information (42-44). In NSCLC, any known somatic mutation is associated with a higher risk of disease recurrence than NSCLC with no mutations, regardless of the type and stage of lung cancer, although the mechanism by which this occurs remains unclear (45). Researchers have explored the prognosis prediction value of somatic mutations, and many studies have revealed the prognostic role of some individual mutations. For example, the mutation of EGFR (46,47), the mutation of TP53 (48), and the mutation of KRAS (49,50) have all been linked to a poor prognosis in NSCLC patients. Additionally, the mutation of EGFR, ALK, ROS1 and BRAF V600E have been defined as drive genes by NCCN guidelines, because of their significant roles in prognosis of NSCLC (9). In recent years, researchers have paid more attention to the prognosis value of co-mutations of multiple somatic mutations. For example, Jao and colleagues showed that multiple mutations were significantly associated with worse DFS but not OS, and multiple mutations with TP53 presented an additional risk to prognosis (51). In a different study, the same authors revealed the prognostic value of TP53 and EGFR co-mutations with data collected from 1,441 NSCLC patients (45). The results suggested that TP53 mutation is more frequent in EGFR mutated NSCLC patients compared to the EGFR wild types, and in patients with mutated EGFR, TP53 mutation is a negative prognostic factor, which highlighted the prognosis value of both co-mutations. However, some contradictions to these results have emerged, including those of the study by Labbé et al., which showed TP53 co-mutation in resected NSCLC patients with EGFR mutated had no significant association with OS nor PFS (52), although the small sample size may have skewed the results. Somatic mutation data are usually used in prediction models in the form of “0” and “1”, with “0” representing no mutation and “1” representing mutation. Most studies usually use gene mutation information to build models, such as EGFR [0/1], but more and more researchers begin to pay attention to the different effects of different mutation sites of the same gene, such as EGFR C. [2,573T >G] [0/1] (53,54).
Another significant prognostic biomarker resulting from genomics analysis is tumor mutational burden (TMB), which is defined as the number of nonsynonymous mutations, especially in immunotherapy patients (55). TMB is a comprehensive manifestation of copy number alterations and somatic mutations. A study by Devarakonda et al. reported high TMB (>8) had a strong positive effect on the prognosis of patients with lung cancer after resection, and patients with low TMB (<4) were more likely to benefit from adjuvant chemotherapy (56). Additionally, the value of TMB as a powerful biomarker in immune checkpoint indicators therapy has been proposed (57-59). Nevertheless, the gold standards for TMB calculation are whole-genome sequencing or whole-exome sequencing, which are time-consuming and costly, and carry a high criterion for the quality and quantity of tissue samples. Recently, Tian and colleagues constructed a TMB estimation model with only 23 genes successfully correlated with whole-exome sequencing TMB (60), and the TMB estimated by the 23-gene panel was shown to have significant correlation with DFS and OS in patients with early-stage NSCLC. The calculation principle of TMB is very complicated and will not be described here (61,62). It is defined as the number of non-synonymous somatic variation per Mb region. The form applied to the model is its value.
Advances in liquid biopsy provide a significant opportunity for acquiring genomics information from circulating tumor DNA (ctDNA). Circulating cell-free DNA is a substance found in blood and body fluids. The amount of cfDNA in cancer patients is much higher than in healthy people, most of which is produced in tumor tissue where it is referred to as ctDNA. ctDNA enters the periphery with necrotic or apoptotic tumor cells and has been proposed as having a significant prognosis value in NSCLC (63,64). On the other hand, it contains the same information as tumor tissue, such as tumor-specific somatic alterations and gene expression (65), and can be accessed with minimally invasive methods and in real time (66). Wang et al. conducted a study to explore whether TMB estimated by ctDNA in blood is associated with clinical outcomes in NSCLC patients with immunotherapy (63) by constructing a cancer gene panel including 150 genes and named this NCC-GP150, to estimate blood TMB and measured tissue TMB by whole-exome sequencing. The results suggested that blood TMB estimated by a small set of genes in ctDNA had a stable correlation with tissue TMB measured by whole-exome sequencing and was associated with superior PFS. This indicated that the clinical limitations of genomics have been largely addressed by liquid biopsy.
Epigenetic biomarkers for NSCLC patients
The wide diversity of tumor mutations is a great challenge for researchers developing effective biomarkers for diagnosis or prognosis because of the large proportions of the genome needed to be examined to provide adequate sensitivity (67). Moreover, epigenetic alterations are a good substitute due to their better stability and homogeneousness in cancer (68). The epigenetic biomarkers most studied are DNA methylation, histone modifications, chromatin remodeling complexes, and long non-coding RNA (lncRNA) (22,69). The form of its application to the model is its expression quantity.
DNA methylation, the most common epigenetic alteration, is also the most widely studied. It has been proved that aberrant methylation in the promoter of tumor suppress gene (TSG) could eliminate its function and promote carcinogenesis (70,71). Although DNA methylation is thought to occur at the early stages of carcinogenesis, researchers have found that some specific genes are also methylated at different tumor stages (72). Many methylated TSG promoters have been shown to be associated with a worse prognosis of NSCLC, such as APC, STXBP6, and RASSF1 (73-76), while many detection panels based on DNA methylation biomarkers were also shown to be suitable for prognosis prediction (77-79). In addition, researchers have acquired DNA methylation information based on liquid biopsy. There is considerable evidence that gene methylation detection in serum, plasma, and sputum is an effective prognostic prediction tool (22,68), and Ooki et al. have demonstrated that the methylation of gene sequences in malignant pleural effusion and malignant ascites is also significant for the prognosis of tumors (77). Thus far, only a few studies with small samples have reported the prognostic and monitoring effect of ctDNA methylation in NSCLC, and most were performed in advanced patients. However, many studies have found that the level of gene methylation seems to be closely related to the prognosis of patients with NSCLC treated with neoadjuvant chemotherapy and surgery combined with radiotherapy (68).
lncRNAs are non-protein-coding RNA molecules composed of more than 200 nucleotides and are often expressed in a spatial, temporal, and tissue-specific pattern (80). In recent years, some meta-analysis and studies have shown that the aberrant expression of lncRNA is a significant prognostic biomarker in a variety of cancers including NSCLC (81). For example, linc00673 could influence the prognosis of NSCLC by regulating its proliferation, migration, invasion, and epithelial mesenchymal transition (82), and by promoting aerobic glycolysis (83). At the same time, some prognostic models based on lncRNAs have also been developed, including a seven-lncRNAs signature to predict OS for patients with early-stage NSCLC (84), and a four-lncRNAs signature to predict the prognosis of patients with NSCLC recently developed by a multicenter study in China (85). Around 3,000 lncRNAs have been discovered to have numerous biological functions in cell growth, differential, and disease progression (86) and more are expected to be identified as tumor prognostic biomarkers in the near future.
Similarly, histone modifications and chromatin remodeling could also provide valuable prognostic biomarkers for NSCLC. Some studies have shown that epigenetic changes involving multiple histones, especially H2A and H3, have great prognostic value for early NSCLC (86), while others have demonstrated that the low expression of BRM and BRG1 contained in two functionally complementary chromatin remodeling complexes was associated with a poor prognosis in NSCLC (87,88). However, to date, prediction models incorporating these two epigenetic modifications are few, perhaps because such modifications are hard to quantify.
The practical application of gene-related biomarkers in prognosis prediction models
Increasingly, evidence has shown that molecular biomarkers can greatly benefit prognostic prediction. Molecular changes in tumors occur when tumor sizes are small and hard to capture, so models that combine genetic and non-genetic factors will have better biological accuracy (79). Moreover, the increasing development of sequencing technology also provides a convenient condition for the application of gene information.
Most studies to date have preferred constructing a prediction model with data from a single omics. The genomics and epigenomics characteristics have been increasingly complex with the discovery of more driver genes and signal pathways, so as other omics (22,51). Compared with a single prognostic marker, integrating multiple prognostic markers into a simple prediction model might effectively improve the predictive value for prognosis. For example, when making a prognosis prediction based on somatic mutations, the risk must be more accurately stratified with an integration of multiple mutations compared to predicting with only a simple gene mutation. In addition to the mutation of gene level, the specific mutation sites could also be incorporated into models (51). Similarly, integrating different kinds of biomarkers to build predictive models should also make predictions more accurate, but may also involve more cost to patients.
Many researchers have commented on the issue of cost-effectiveness. Gray et al. (89) performed a meta-analysis on lung cancer risk prediction models and found that although the discrimination of models incorporating genetic information has improved, there has been little improvement in epidemiological models, which remain costly and time consuming. As there are no systematic reviews on lung cancer prognostic prediction models, we conducted a literature screening to compare the performance of those incorporating gene-related biomarkers with those that do not (Table 1). The details of the literature screening process are summarized in Figure 1, and show that although only some models were compared, it appears that incorporating gene-related biomarkers cannot significantly improve the performance of prediction models.
Table 1
Title | Reference | Gene-related | Training sample size | C-statistics in training set | Test sample size | C-statistics in validation set |
---|---|---|---|---|---|---|
The development and external validation of an overall survival nomogram in medically inoperable centrally located early-stage non-small cell lung carcinoma | Duijm et al. (90) | No | 220 | 0.640 | 92 | 0.620 |
A nomogram based on CT deep learning signature: a potential tool for the prediction of overall survival in resected non-small cell lung cancer patients | Lin et al. (91) | No | 231 | 0.800 | 77 | 0.723 |
Development and validation of a nomogram for preoperative prediction of lymph node metastasis in lung adenocarcinoma based on radiomics signature and deep learning signature | Ran et al. (92) | No | 200 | 0.820 | 60 | 0.861 |
A seven-gene signature with close immune correlation was identified for survival prediction of lung adenocarcinoma* | Zou et al. (93) | Yes | 499 | 0.781 | 180 | 0.659 |
Identification and validation of a tumor microenvironment-related gene signature for prognostic prediction in advanced- stage non-small-cell lung cancer*#^ | Zhang et al. (94) | Yes | 192 | 0.681 | 91 | 0.637 |
Development of an immune-related gene pairs signature for predicting clinical outcome in lung adenocarcinoma*# | Wu et al. (95) | Yes | 465 | 0.87 | 431 | 0.803 |
Identification of a 5-gene metabolic signature for predicting prognosis based on an integrated analysis of tumor microenvironment in lung adenocarcinoma | Yu et al. (96) | Yes | 535 | 0.767 | 442 | 0.685 |
A model of twenty-three metabolic-related genes predicting overall survival for lung adenocarcinoma*# | Zhao et al. (97) | Yes | 445 | 0.734 | 393 | 0.742 |
A prognostic nomogram combining immune-related gene signature and clinical factors predicts survival in patients with lung adenocarcinoma# | Song et al. (98) | Yes | 500 | 0.652 | 442 | 0.632 |
*, more than one external validation set was used, and the one with the largest sample size was compared; #, time-dependent ROC curves were made, and the ROC curve with the longest predicted survival time was compared; ^, several models were made according to the different end points of the study, and the model with OS as the end point was compared.
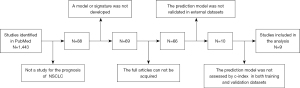
This may indicate the benefits of incorporating genetic information into prediction models should be evaluated from more aspects. Despite prognosis prediction models being different from diagnostic prediction models in function, the cost-effectiveness of each is an issue that requires common attention, and whether the added cost and time to obtain gene-related biomarkers is worthy requires further investigation (89). Some authors believe that with the progresses in science and technology, the detection technology of bionomics will be more convenient allowing more patients to benefit from it (79). Overall, the most fundamental role of the clinical prognosis prediction model is to allow clinicians and patients to understand the prognosis of patients simply, quickly, and effectively, and to guide treatment in a timely manner. Therefore, how to reduce the number of genes that need to be detected as much as possible while ensuring the improvement of accuracy is more important than the continuous development of new biomarkers (99).
In fact, there is a solution to this problem. More and more researchers have begun to select genes based on their function in order to reduce the number of genes. At present, more and more mechanisms have been found to be related to tumor genesis, development and prognosis, such as hypoxia (100,101), autophagy (102,103), ferroptosis (104,105), immune microenvironment (106) and so on. At the same time, more and more genes have been found to be associated with these mechanisms. Genes with similar functions have been collected by some researchers into a set of genes called a functional gene set, such as ferroptosis-related genes (107). Such models, based on specific functional gene sets, have practical applications, especially in drug use, and could provide clinicians with new ideas for treatment (103,108). For example, autophagy, has been found as a new way to treat cancer (109-111). It plays a role in the occurrence and development of tumors by virtue of various mechanisms. At the same time, various autophagy mechanisms can also inhibit cancer. On this basis, Zhang et al. (103) downloaded 210 autophagy-related genes from the Human Autophagy Database (HADb, https://autophagy.lu/clustering/index.html). Then 1496 lncRNAs were identified by a coexpression analysis. Finally, a 9 autophagy-related lncRNAs were screened to construct a prognostic model for NSCLC. According to this model, patients were divided into high-risk and low-risk groups. The significance of this model is that patients in the high-risk group may be more likely to receive autophagy-related therapy. Obviously, this model is more targeted from the selection of genes, which greatly reduces the amount of calculation needed to establish the model, and at the same time increases the practicality of the model, and puts forward more intuitive opinions on the treatment of diseases. Of course, the application of the model depends on the development of related drugs or treatments. Similarly, starting with a pathway, genes involved in an important pathway are selected to model the set of genes to be screened, which has the same effect with selecting the specific functional gene sets (111).
Potential predictors that were not included or were not examined in detail in the model
Marital status
Recently, Chen et al. recognized the prognostic role of marital status in lung cancer (112). In the model for predicting the prognosis of NSCLC with marital status, the HR of married patients versus unmarried patients was 0.914 (CI: 0.896–0.933, P<0.001) by univariate analysis and 0.869 (CI: 0.851–0.887, P<0.001) by multivariate analysis. A prognostic model of squamous cell carcinoma in the elderly carried out by Chen et al. (113) included marital status as one of the predictors, and the HR of unmarried versus married persons was 1.146 (CI: 1.103–1.190, P<0.001) by univariate analysis, and 1.042 (CI: 1.000–1.085, P=0.049) by multivariate analysis. While studies showing the effect of marital status on the prognosis of lung cancer patients date back to 2007 (114), those examining the relationship between marital status and prognosis in patients with NSCLC have yielded different results using data from different regions. Studies conducted in the United States have generally concluded that unmarried patients have a worse prognosis than those who are divorced or married, while studies in Japan have shown that divorced patients have a worse prognosis (115,116). Overall, unmarried patients have worse overall survival than those who had been married (married or divorced) (112-118). The differences in these results may indicate marriage is not a direct influence on tumor prognosis, which could result from several factors, such as sociodemographic differences in culture and economy between regions.
Chen et al. also noted that the current studies only recorded marital status at the time of diagnosis and did not record information on changes in marital status after diagnosis, which could lead to biased results (113). On the other hand, the present data used in most relevant studies we reviewed are from 10 years ago, and the data source is relatively single. Due to changes in social demographics, published conclusions may be different from the current situation, and it is difficult to translate the existing research conclusions into practical applications. Therefore, more new data are needed to carry out relevant studies.
Inflammation scores
The difficulty of obtaining extensive lung cancer tissue in many cases underscores the value of blood biomarkers (119). The role of inflammation in cancer progression is broadly accepted and inflammatory biomarkers are established prognostic predictors (120). However, using a single biomarker of inflammation to predict prognosis may be premature. Therefore, inflammation scores combined with multiple inflammatory biomarkers, such as NLR, PLR, ALI, and SII may provide greater accuracy (121-127). The examination indexes included in inflammation scores can be obtained in routine diagnostic tests, including blood routine and blood biochemical tests. These tests are convenient and cheap to implement and have great potential for clinical application.
Mandaliya et al. (127) collected data from 279 patients with advanced NSCLC to conduct unified evaluation of PLR, NLR, ALI, and LMR (110) for the first time. The prognostic effect of these factors was assessed by establishing their association with OS before and after treatment, and it was found that basal high PLR and NLR were associated with poor prognosis. However, while the study illustrated the relationship between these inflammatory scores and prognosis, it did not establish a quantitative relationship within a model.
Sandfeld-Paulsen et al. (128) evaluated five existing inflammatory scoring systems (NLR, PLR, GPS, optimization of three of them—CNG, ACBS) using a dataset of 275 people, with the systems optimized by including comorbidity, age, PS score, TNM stage, and smoking. After the model was established and tested, the order of C statistics from small to large was as follows: original inflammatory score model, TNM staging model, original inflammatory score +TNM+PS model. Among them, ACBS, which is a scoring system developed by the authors themselves and differs from CNG in that the rate is replaced with a parameter and globulin is included, had the best prognostic effect. Most patients with lung cancer have comorbidities that affect inflammatory factors (129), and their influence must be considered when using inflammatory cytokines as prognostic predictors, and Sandfeld-Paulsen et al. addressed this by building a multi-factor model. However, the limitation of that study is that the measurement of inflammatory factors was only conducted at one time point, and it may be necessary to measure them several times at different time points to more accurately and dynamically evaluate the treatment effect and patient prognosis.
Although many articles have revealed the relationship between various inflammatory scores and the prognosis of NSCLC, this indicator has rarely been included as a predictor in the prognosis prediction model of NSCLC. It is well known that inflammatory markers are easily affected by body states, such as complications, so the use of an inflammatory score alone for prognosis prediction may be unreliable. However, their role as a predictor in an appropriate model should be considered.
Radiomics features
Radiomics involves the study of quantitative features extracted from medical images such as CT, MRI, and PET, and has been used to assess the prognosis of cancer patients (130-133). The use of quantitative information on cancer phenotypic characteristics obtained from imaging to develop clinical predictive models is an important goal of radiomics (134). Existing radiomics studies have provided multiple predictors for the prognostic model of NSCLC. For example, it has been shown that the imaging features of CBCT (cone-beam CT) can be used to evaluate the therapeutic effect of lung cancer patients (135). CBCT is a clinical image promoted to use in recent years, and its image quality is lower than that of conventional CT. In this study, through a two-step calibration process, we showed the imaging features of CBCT and conventional CT had internal conversion, that is, the imaging features of CBCT could be used to predict the prognosis of patients with NSCLC instead of conventional CT. While this provided a subset of new factors for the prognostic model of NSCLC, the authors also put forward problems that need to be addressed. Compared with conventional CT, CBCT is more sensitive to artifacts, and more studies are needed to explore the influence of artifacts on CBCT radiomics. In addition, we believe that the excessive selection of predictors is also a problem. In this study, 149 features were included in the establishment of the model from 1,119 candidate features, which was a great obstacle to the clinical application of the model. Future studies should focus on screening out more representative features for model building.
There is another key advantage that radiomics features could provide is that it overcomes the problem of continuity of predictors, including of time and space continuity. It is wide known that tumors are a continuously envolving biological system, a continue predictor could greatly improve models. Profiting from the development of machine learning technology, radiomics features used for modeling have been developed from quantifiable features extracted manually from traditional images to imaging images without artificial definition (136-138). In 2019, Xu et al. (139) constructed a deep learning model from time-series CT-images to predict lung cancer treatment response to chemoradiation. The researchers included 179 stage III NSCLC patients treated with chemoradiation, and established a deep learning model using their pre- and post-treatment CT images at 1,3, and 6 months. The AUC of the model predicting 2-year overall-survival was 0.74 (P<0.05). The models stratified patients into low and high mortality risk-groups, significantly associated with overall survival (HR =6.16, 95% CI: 2.17–17.44, P<0.001). Regardless of the discrimination of the model, after all, AUC can be improved by increasing the sample size or changing the statistical analysis method. The key point of this study is that it solved the problem that the predictors in the prognostic prediction model are not capable of dynamic evaluation. Moreover, the change of input predictors from traditional numbers to two-dimensional images is a qualitative leap in the development of models.
The development of radiomics has promoted the development of personalized medicine. By the early identification of quantitative markers of treatment response, treatment can be adjusted in time (140). Currently, most predictive models characterized by imaging omics lack reproducibility and external validation, and cannot be applied in clinical practice (141). In addition, the standardization of imagomics features, including the standardization of images and workflow, should be a focus of development (134,140,142).
Development, validation, and assessment of models
Development
First, data is the basis on which models are built. Nowadays, more and more databases are being created and published, such as The Cancer Genome Atlas (TCGA), Gene Expression Omnibus (GEO). More and more researchers tend to use data from databases to build their own models. This has promoted the development of clinical prediction models. Data should be cleaned prior to application to modeling, including steps of quality control, standardization, and batch removal. Part of the database or datasets has completed this step of processing, researchers can directly use the data. Some researchers tend to combine multiple data sets to build models. Because different data sets have different data processing methods, different sources, and even different sequencing platforms, direct integration of them into a new data set leads to very large heterogeneity. Therefore, it is necessary to remove batch effects and standardize before integration. R packages “lumi”, “limma” and “beadarry” can be used for quality control and normalization, “sva” can be used for batch removal.
The establishment of new models relies on the proposal of innovative predictors. After potential predictors are identified, they are further screened with univariate and multi-variates analysis as the candidate variables. In fact, after determining the predictors to be screened, the researchers generally did not include all of this information, but selected variables that were significantly different between the experimental and control groups. For example, in the prognostic prediction models of NSCLC with gene expression, the researchers will first compare the gene expression in cancer tissue with that in normal tissue, the basic way is that the two samples are tested with Student’s t test, and then select the genes with significant differences in expression in the two groups to be included in the next screening, such as those whose adj.P value is less than 0.05 and whose absolute value of logFC (log2FoldChange) is greater than 1. In fact, this method of screening for variables that vary significantly between the experimental and control groups is universal when there are many predictors to screen for, not just for gene-related models. For example, Nair et al. (143) have carried out a study that using machine learning techniques to predict EGFR mutations in NSCLC by radiogenomics. Before feature screening and model building, 326 imaging omics features were analyzed by linear discriminant analysis (LDA) to screen out those more important features and rank them by importance. Although the author did not specify how many features were screened out by this step, the author also clearly indicated that this step could greatly reduce the possibility of overfitting the model. In fact, this approach is similar to difference analysis. In this study, 326 imaging features of patients with EGFR mutated and patients with EGFR wild-type were analyzed, among which adj. P<0.05 were screened out, and the features with greater changes were sorted according to the absolute value of logFC to enter the next stage of screening. This approach has the same effect as the LDA method adopted by the author. After that, univariate analysis is used to primarily screen out the predictors which have influence on the outcome, and multi-variates analysis can eliminate the bias caused by other confounding factors by stepwise regression or The Least Absolute Shrinkage and Selection operator (LASSO). According to different types of predictors, clinical prediction models can also be divided into epidemiological models, clinical models, and biomarker models (89). There are many ways to develop models, such as logistic regression to dichotomous outcomes and COX regression to time-event outcomes (144). Prognosis prediction models are used to evaluate the survival probabilities in future years, so COX regression is used widely. In recent years, machine learning has been applied to the development of clinical prediction models (145,146), and in a background of the rapid development of high-throughput sequencing, more models are incorporating gene-related information for analysis. Machine learning can effectively analyze large samples of biomedical data containing genomic and genetic information and elucidate the complex biological mechanisms involved (146). Researchers should choose the most appropriate way to develop a model according to the difference of the outcome variables.
Validation
When a regression model is developed and fitted on a particular data set, the model must fit the random changes within the data set. However, when the model is applied to other data, its fitting performance may not be satisfactory due to the unique internal random variation of the data set (147). Therefore, models must be validated by internal validation and external validation. Internal validation is mainly used for the correction of overfitting and optimism by cross-validation and optimism-corrected bootstrapping (11). However, external validation that tests the model with different data from the original is more convincing. It is also necessary to assess the accuracy of models. Tammemägi et al. (147) found that internal validation tends to underestimate the loss of predictive performance observed when the model is applied to new data. However, in reviewing existing prognostic models, every model has its own external validation dataset, and there is no unified dataset for external validation of existing models to create a rigorous evaluation environment, which means that comparison is difficult. Additionally, the independent external validation of biomarker models and clinical models is inadequate, which could be due to the high cost and disinterest of volunteers.
Assessment
After the development and validation of models, some critical indexes should be used to assess them, and when this does not take place, serious bias may occur (148). The main components of model assessment are discrimination and calibration. Discrimination refers to the ability to distinguish case from control, which is most widely represented by an area under the receiver operator characteristic curve (AUC) and concordance or c-statistic, and the two are numerically equal. However, discrimination can only evaluate the ability to classify correctly, and does not reflect the ability of a model to predict individual probabilities accurately. To correct this, calibration is introduced. Calibration has been measured by goodness-of-fit tests (149), and the Hosmer-Lemershow statistic has been used for logistic regression. However, this method has been criticized for its instability of P value (150). The most widely applied method for assessing calibration is plotting-observed probabilities versus model-predicted probabilities. Some modelers also use the Brier score, which is a proper scoring rule that is affected by both discrimination and calibration (151). However, differentiation and calibration alone can only reflect the accuracy of model prediction and cannot directly reflect whether patients can benefit from it in clinical practice. Decision curve analysis (DCA) integrates the preferences of patients and decision makers into the curve, directly showing the extent to which patients can benefit from a decision, which meets the needs of clinical application and is an effective supplement to an ROC (146,152).
The performance of models developed by different methods with the same data set will not be the same, and no method is consistently better than others. This leads to a status quo, in that modelers may report the best indexes alternatively, which increases the difficulty for comparison, and calls for a unified assessment rule to be identified.
While development, validation, and assessment are indispensable in clinical prediction model research, most currently published models are unsatisfactory in their validation and evaluation. As the focus of this study is not on the methodology of the model, we have provided a brief introduction only, and more details on the development, validation, and assessment of clinical prediction models are discussed in Harrell et al. (153).
Visualization of models
Generally, the regression formula should be reported in a logistic or COX regression model to allow validation by other researchers. Some modelers have also transformed the formula to a risk score to make it easier to understand and practice. For example, we have developed a survival prediction model for NSCLC and reported a risk score formula:
Age: represented age of diagnosis; stage: I/II =1, stage III/IV =2; EGFR, PIK3CA, TP53: mutation =1, no mutation =0 (53).
Visual depictions such as nomograms, graphical score charts, and website/mobile apps convey research results in an accessible manner. In this study, we used a nomogram (Figure 2) to assign a score to each patient on the corresponding axis of variation, and the sum of these numbers could determine the location on the total points axis. This carries the advantage of displaying multiple time points which, along with continuous and categorized predictors can also be presented on the one interface. However, nomograms can appear complex at first sight and require an explanation as to how they should be used (148). In addition, the result can be inaccurate due to the low pointers number for predictors resulting in personal equation during observation. A dynamic nomogram can be plotted by the R package “DynNom” (154), which is a web calculator, offering a high-precision and convenient method which may gradually replace the basic nomogram. In addition, this evaluation method, which relies on the network and mobile devices, can be easily embedded into the patient's diagnosis and treatment system to monitor their condition anytime and anywhere.
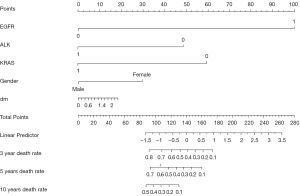
The format depends on user and environment conditions. Guides to presenting clinical prediction models for use in clinical settings provide key information for modelers when selecting visualization form (155). However, there is a restriction in that the guideline is only appropriate for traditional models using logistic or COX regression. As machine learning is increasingly used, so are some new forms of visualization, and a standard which is similar to the guide to presenting clinical prediction models should be formulated and applied.
Bias assessment based on PROBAST
PROBAST is a prediction model of bias assessment tool for assessing the risk of bias (ROB) and has applicability to review prediction models (156). It consists of four domains: participants, predictors, outcome, and analysis, and includes twenty specific items facilitating quality control (157). The assessment tool was designed for the systematic review of prediction model studies and has been applied in many areas including breast cancer, kidney cancer, lymphocytic leukaemia, and oropharyngeal cancer (158-161). We assessed the bias of the nine studies mentioned above using PROBAST and the results are shown in Table 2. While the applicability to review was not assessed because a systematic review question was not set, the results of the overall judgement are strict. The prediction models identified as “low ROB” were set when low ROB was seen in all domains, and a “high ROB” was reached if at least one domain had high ROB. While the overall judgement about concerns regarding applicability was the same, a prediction model was not validated in an external dataset, the study was judged as high ROB even if all domains had low ROB. Therefore, we excluded the studies without external validation.
Table 2
Study | ROB | Applicability | Overall | ||||||||
---|---|---|---|---|---|---|---|---|---|---|---|
Participants | Predictors | Outcome | Analysis | Participants | Predictors | Outcome | ROB | Applicability | |||
The development and external validation of an overall survival nomogram in medically inoperable centrally located early-stage non-small cell lung carcinoma | – | – | – | + | + | ||||||
A nomogram based on CT deep learning signature: a potential tool for the prediction of overall survival in resected non-small cell lung cancer patients | – | – | – | + | + | ||||||
Development and validation of a nomogram for preoperative prediction of lymph node metastasis in lung adenocarcinoma based on radiomics signature and deep learning signature | – | – | – | + | + | ||||||
A seven-gene signature with close immune correlation was identified for survival prediction of lung adenocarcinoma | ? | – | – | + | + | ||||||
Identification and validation of a tumor microenvironment-related gene signature for prognostic prediction in advanced-stage non-small-cell lung cancer | – | – | – | + | + | ||||||
Development of an immune-related gene pairs signature for predicting clinical outcome in lung adenocarcinoma | ? | – | – | + | + | ||||||
Identification of a 5-gene metabolic signature for predicting prognosis based on an integrated analysis of tumor microenvironment in lung adenocarcinoma | ? | – | – | + | + | ||||||
A model of twenty-three metabolic-related genes predicting overall survival for lung adenocarcinoma | – | – | – | + | + | ||||||
A prognostic nomogram combining immune-related gene signature and clinical factors predicts survival in patients with lung adenocarcinoma | ? | – | – | + | + |
+, low ROB/low concern regarding applicability; –, high ROB/high concern regarding applicability; ?, unclear ROB/unclear concern regarding applicability. ROB, risk of bias.
From the results, all included studies had a “high ROB” in the overall judgement. The main ROB was due to the domain “analysis”, and the domain “participants” was unclear because many researchers did not note the inclusion and exclusion criteria of subjects.
The designers of PROBAST have broadened its scope of application to allow it to be used as a tool or yardstick for critically evaluating the original studies of predictive models. The development team of PROBAST recommend that researchers should refer to it to avoid the ROB from study design and datasets at the commencement of a study and should assess the applicability of statistics methods according to PROBAST during the study. After the study is completed, scholars or experts independent of the model development team should be invited to evaluate the methodological quality of the model to control the bias of the study (157).
Conclusions
The wide application of next-generation sequencing technology provides a variety of predictive factors for clinical prediction models. However, it seems that gene-related biomarkers cannot obviously improve the performance of models. An urgent solution as to how to simplify the required biomarkers while ensuring stability is required. The TNM staging system, WHO-PS, and pathological classification should be incorporated into all models, and the existing models should be validated in a large external dataset to make a meaningful comparison. Moreover, the current prognosis prediction model of NSCLC is at a high ROB, and promoting the application of PROBAST may improve this situation. In addition, a systematic review of the prognosis prediction model research of NSCLC is required.
Acknowledgments
Funding: Natural funding project of Tianjin Science and Technology Bureau (No. 20JCYBJC01350).
Footnote
Reporting Checklist: The authors have completed the Narrative Review reporting checklist. Available at https://dx.doi.org/10.21037/atm-21-4733
Conflicts of Interest: All authors have completed the ICMJE uniform disclosure form (available at https://dx.doi.org/10.21037/atm-21-4733). The authors have no conflicts of interest to declare.
Ethical Statement: The authors are accountable for all aspects of the work in ensuring that questions related to the accuracy or integrity of any part of the work are appropriately investigated and resolved.
Open Access Statement: This is an Open Access article distributed in accordance with the Creative Commons Attribution-NonCommercial-NoDerivs 4.0 International License (CC BY-NC-ND 4.0), which permits the non-commercial replication and distribution of the article with the strict proviso that no changes or edits are made and the original work is properly cited (including links to both the formal publication through the relevant DOI and the license). See: https://creativecommons.org/licenses/by-nc-nd/4.0/.
References
- Siegel RL, Miller KD, Fuchs HE, et al. Cancer Statistics, 2021. CA Cancer J Clin 2021;71:7-33. [Crossref] [PubMed]
- Ferlay J, Colombet M, Soerjomataram I, et al. Estimating the global cancer incidence and mortality in 2018: GLOBOCAN sources and methods. Int J Cancer 2019;144:1941-53. [Crossref] [PubMed]
- Schwartz AG, Cote ML. Epidemiology of Lung Cancer. Adv Exp Med Biol 2016;893:21-41. [Crossref] [PubMed]
- Travis WD, Brambilla E, Burke AP, et al. Introduction to The 2015 World Health Organization Classification of Tumors of the Lung, Pleura, Thymus, and Heart. J Thorac Oncol 2015;10:1240-2. [Crossref] [PubMed]
- Thakur MK, Gadgeel SM. Predictive and Prognostic Biomarkers in Non-Small Cell Lung Cancer. Semin Respir Crit Care Med 2016;37:760-70. [Crossref] [PubMed]
- Amin MB, Greene FL, Edge SB, et al. The Eighth Edition AJCC Cancer Staging Manual: Continuing to build a bridge from a population-based to a more "personalized" approach to cancer staging. CA Cancer J Clin 2017;67:93-9.
- Detterbeck FC, Boffa DJ, Kim AW, et al. The Eighth Edition Lung Cancer Stage Classification. Chest 2017;151:193-203.
- Au EH, Francis A, Bernier-Jean A, et al. Prediction modeling-part 1: regression modeling. Kidney Int 2020;97:877-84. [Crossref] [PubMed]
- Wood DE, Kazerooni EA, Baum SL, et al. Lung Cancer Screening, Version 3.2018, NCCN Clinical Practice Guidelines in Oncology. J Natl Compr Canc Netw 2018;16:412-41. [Crossref] [PubMed]
- Jaklitsch MT, Jacobson FL, Austin JH, et al. The American Association for Thoracic Surgery guidelines for lung cancer screening using low-dose computed tomography scans for lung cancer survivors and other high-risk groups. J Thorac Cardiovasc Surg 2012;144:33-8. [Crossref] [PubMed]
- Muller DC, Johansson M, Brennan P. Lung Cancer Risk Prediction Model Incorporating Lung Function: Development and Validation in the UK Biobank Prospective Cohort Study. J Clin Oncol 2017;35:861-9. [Crossref] [PubMed]
- Marcus MW, Chen Y, Raji OY, et al. LLPi: Liverpool Lung Project Risk Prediction Model for Lung Cancer Incidence. Cancer Prev Res (Phila) 2015;8:570-5. [Crossref] [PubMed]
- Tammemagi CM, Pinsky PF, Caporaso NE, et al. Lung cancer risk prediction: Prostate, Lung, Colorectal And Ovarian Cancer Screening Trial models and validation. J Natl Cancer Inst 2011;103:1058-68. [Crossref] [PubMed]
- National Lung Screening Trial Research Team. The National Lung Screening Trial: overview and study design. Radiology 2011;258:243-53. [Crossref] [PubMed]
- Brundage MD, Davies D, Mackillop WJ. Prognostic factors in non-small cell lung cancer: a decade of progress. Chest 2002;122:1037-57. [Crossref] [PubMed]
- Chen VW, Ruiz BA, Hsieh MC, et al. Analysis of stage and clinical/prognostic factors for lung cancer from SEER registries: AJCC staging and collaborative stage data collection system. Cancer 2014;120:3781-92. [Crossref] [PubMed]
- Ettinger DS, Wood DE, Aisner DL, et al. NCCN Guidelines Insights: Non-Small Cell Lung Cancer, Version 2.2021. J Natl Compr Canc Netw 2021;19:254-66. [Crossref] [PubMed]
- Park K, Vansteenkiste J, Lee KH, et al. Pan-Asian adapted ESMO Clinical Practice Guidelines for the management of patients with locally-advanced unresectable non-small-cell lung cancer: a KSMO-ESMO initiative endorsed by CSCO, ISMPO, JSMO, MOS, SSO and TOS. Ann Oncol 2020;31:191-201. [Crossref] [PubMed]
- Devarakonda S, Masood A, Govindan R. Next-Generation Sequencing of Lung Cancers: Lessons Learned and Future Directions. Hematol Oncol Clin North Am 2017;31:1-12. [Crossref] [PubMed]
- Li Y, Gu J, Xu F, et al. Transcriptomic and functional network features of lung squamous cell carcinoma through integrative analysis of GEO and TCGA data. Sci Rep 2018;8:15834. [Crossref] [PubMed]
- Kim IA, Hur JY, Kim HJ, et al. Targeted Next-Generation Sequencing Analysis for Recurrence in Early-Stage Lung Adenocarcinoma. Ann Surg Oncol 2021;28:3983-93. [Crossref] [PubMed]
- Duruisseaux M, Esteller M. Lung cancer epigenetics: From knowledge to applications. Semin Cancer Biol 2018;51:116-28. [Crossref] [PubMed]
- Oberije C, De Ruysscher D, Houben R, et al. A Validated Prediction Model for Overall Survival From Stage III Non-Small Cell Lung Cancer: Toward Survival Prediction for Individual Patients. Int J Radiat Oncol Biol Phys 2015;92:935-44. [Crossref] [PubMed]
- Wang Y, Qu X, Kam NW, et al. An inflammation-related nomogram for predicting the survival of patients with non-small cell lung cancer after pulmonary lobectomy. BMC Cancer 2018;18:692. [Crossref] [PubMed]
- Wang H, Zhao J, Zhang M, et al. The combination of plasma fibrinogen and neutrophil lymphocyte ratio (F-NLR) is a predictive factor in patients with resectable non small cell lung cancer. J Cell Physiol 2018;233:4216-24. [Crossref] [PubMed]
- She Y, Jin Z, Wu J, et al. Development and Validation of a Deep Learning Model for Non-Small Cell Lung Cancer Survival. JAMA Netw Open 2020;3:e205842 [Crossref] [PubMed]
- Prelaj A, Rebuzzi SE, Pizzutilo P, et al. EPSILoN: A Prognostic Score Using Clinical and Blood Biomarkers in Advanced Non-Small-cell Lung Cancer Treated With Immunotherapy. Clin Lung Cancer 2020;21:365-377.e5. [Crossref] [PubMed]
- Dall'Olio FG, Maggio I, Massucci M, et al. ECOG performance status ≥2 as a prognostic factor in patients with advanced non small cell lung cancer treated with immune checkpoint inhibitors-A systematic review and meta-analysis of real world data. Lung Cancer 2020;145:95-104. [Crossref] [PubMed]
- Spigel DR, McCleod M, Jotte RM, et al. Safety, Efficacy, and Patient-Reported Health-Related Quality of Life and Symptom Burden with Nivolumab in Patients with Advanced Non-Small Cell Lung Cancer, Including Patients Aged 70 Years or Older or with Poor Performance Status (CheckMate 153). J Thorac Oncol 2019;14:1628-39. [Crossref] [PubMed]
- Azam F, Latif MF, Farooq A, et al. Performance Status Assessment by Using ECOG (Eastern Cooperative Oncology Group) Score for Cancer Patients by Oncology Healthcare Professionals. Case Rep Oncol 2019;12:728-36. [Crossref] [PubMed]
- Young J, Badgery-Parker T, Dobbins T, et al. Comparison of ECOG/WHO performance status and ASA score as a measure of functional status. J Pain Symptom Manage 2015;49:258-64. [Crossref] [PubMed]
- Friedlaender A, Banna GL, Buffoni L, et al. Poor-Performance Status Assessment of Patients with Non-small Cell Lung Cancer Remains Vague and Blurred in the Immunotherapy Era. Curr Oncol Rep 2019;21:107. [Crossref] [PubMed]
- Beasley MB, Dembitzer FR, Flores RM. Surgical pathology of early stage non-small cell lung carcinoma. Ann Transl Med 2016;4:238. [Crossref] [PubMed]
- Watanabe M, Yokose T, Tetsukan W, et al. Micropapillary components in a lung adenocarcinoma predict stump recurrence 8 years after resection: a case report. Lung Cancer 2013;80:230-3. [Crossref] [PubMed]
- Cha MJ, Lee HY, Lee KS, et al. Micropapillary and solid subtypes of invasive lung adenocarcinoma: clinical predictors of histopathology and outcome. J Thorac Cardiovasc Surg 2014;147:921-928.e2. [Crossref] [PubMed]
- Watanabe K, Sakamaki K, Ito H, et al. Impact of the micropapillary component on the timing of recurrence in patients with resected lung adenocarcinoma. Eur J Cardiothorac Surg 2020;58:1010-8. [Crossref] [PubMed]
- Sica G, Yoshizawa A, Sima CS, et al. A grading system of lung adenocarcinomas based on histologic pattern is predictive of disease recurrence in stage I tumors. Am J Surg Pathol 2010;34:1155-62. [Crossref] [PubMed]
- Ohe M, Yokose T, Sakuma Y, et al. Stromal micropapillary component as a novel unfavorable prognostic factor of lung adenocarcinoma. Diagn Pathol 2012;7:3. [Crossref] [PubMed]
- Miyoshi T, Aokage K, Katsumata S, et al. Ground-Glass Opacity Is a Strong Prognosticator for Pathologic Stage IA Lung Adenocarcinoma. Ann Thorac Surg 2019;108:249-55. [Crossref] [PubMed]
- Mino-Kenudson M. Significance of tumor spread through air spaces (STAS) in lung cancer from the pathologist perspective. Transl Lung Cancer Res 2020;9:847-59. [Crossref] [PubMed]
- Travis WD. Lung Cancer Pathology: Current Concepts. Clin Chest Med 2020;41:67-85. [Crossref] [PubMed]
- Parikh AR. Lung Cancer Genomics. Acta Med Acad 2019;48:78-83. [Crossref] [PubMed]
- Mascaux C, Tsao MS, Hirsch FR. Genomic Testing in Lung Cancer: Past, Present, and Future. J Natl Compr Canc Netw 2018;16:323-34. [Crossref] [PubMed]
- Shukla S, Evans JR, Malik R, et al. Development of a RNA-Seq Based Prognostic Signature in Lung Adenocarcinoma. J Natl Cancer Inst 2017;109:djw200 [Crossref] [PubMed]
- Jiao XD, Qin BD, You P, et al. The prognostic value of TP53 and its correlation with EGFR mutation in advanced non-small cell lung cancer, an analysis based on cBioPortal data base. Lung Cancer 2018;123:70-5. [Crossref] [PubMed]
- Zhang Z, Wang T, Zhang J, et al. Prognostic value of epidermal growth factor receptor mutations in resected non-small cell lung cancer: a systematic review with meta-analysis. PLoS One 2014;9:e106053 [Crossref] [PubMed]
- Izar B, Sequist L, Lee M, et al. The impact of EGFR mutation status on outcomes in patients with resected stage I non-small cell lung cancers. Ann Thorac Surg 2013;96:962-8. [Crossref] [PubMed]
- Xu F, Lin H, He P, et al. A TP53-associated gene signature for prediction of prognosis and therapeutic responses in lung squamous cell carcinoma. Oncoimmunology 2020;9:1731943 [Crossref] [PubMed]
- Rulli E, Marabese M, Torri V, et al. Value of KRAS as prognostic or predictive marker in NSCLC: results from the TAILOR trial. Ann Oncol 2015;26:2079-84. [Crossref] [PubMed]
- Pan W, Yang Y, Zhu H, et al. KRAS mutation is a weak, but valid predictor for poor prognosis and treatment outcomes in NSCLC: A meta-analysis of 41 studies. Oncotarget 2016;7:8373-88. [Crossref] [PubMed]
- Jao K, Tomasini P, Kamel-Reid S, et al. The prognostic effect of single and multiple cancer-related somatic mutations in resected non-small-cell lung cancer. Lung Cancer 2018;123:22-9. [Crossref] [PubMed]
- Labbé C, Cabanero M, Korpanty GJ, et al. Prognostic and predictive effects of TP53 co-mutation in patients with EGFR-mutated non-small cell lung cancer (NSCLC). Lung Cancer 2017;111:23-9. [Crossref] [PubMed]
- Zhang W, Lin X, Li X, et al. Survival prediction model for non-small cell lung cancer based on somatic mutations. J Gene Med 2020;22:e3206 [Crossref] [PubMed]
- Bertl J, Guo Q, Juul M, et al. A site specific model and analysis of the neutral somatic mutation rate in whole-genome cancer data. BMC Bioinformatics 2018;19:147. [Crossref] [PubMed]
- Steuer CE, Ramalingam SS. Tumor Mutation Burden: Leading Immunotherapy to the Era of Precision Medicine? J Clin Oncol 2018;36:631-2. [Crossref] [PubMed]
- Devarakonda S, Rotolo F, Tsao MS, et al. Tumor Mutation Burden as a Biomarker in Resected Non-Small-Cell Lung Cancer. J Clin Oncol 2018;36:2995-3006. [Crossref] [PubMed]
- Rizvi NA, Hellmann MD, Snyder A, et al. Cancer immunology. Mutational landscape determines sensitivity to PD-1 blockade in non-small cell lung cancer. Science 2015;348:124-8. [Crossref] [PubMed]
- Alborelli I, Leonards K, Rothschild SI, et al. Tumor mutational burden assessed by targeted NGS predicts clinical benefit from immune checkpoint inhibitors in non-small cell lung cancer. J Pathol 2020;250:19-29. [Crossref] [PubMed]
- Wang Z, Duan J, Cai S, et al. Assessment of Blood Tumor Mutational Burden as a Potential Biomarker for Immunotherapy in Patients With Non-Small Cell Lung Cancer With Use of a Next-Generation Sequencing Cancer Gene Panel. JAMA Oncol 2019;5:696-702. [Crossref] [PubMed]
- Tian Y, Xu J, Chu Q, et al. A novel tumor mutational burden estimation model as a predictive and prognostic biomarker in NSCLC patients. BMC Med 2020;18:232. [Crossref] [PubMed]
- Aladwani M, Lophatananon A, Ollier W, et al. Prediction models for prostate cancer to be used in the primary care setting: a systematic review. BMJ Open 2020;10:e034661 [Crossref] [PubMed]
- Bertl J, Guo Q, Juul M, et al. A site specific model and analysis of the neutral somatic mutation rate in whole-genome cancer data. BMC Bioinformatics 2018;19:147. [Crossref] [PubMed]
- Wang Z, Duan J, Cai S, et al. Assessment of Blood Tumor Mutational Burden as a Potential Biomarker for Immunotherapy in Patients With Non-Small Cell Lung Cancer With Use of a Next-Generation Sequencing Cancer Gene Panel. JAMA Oncol 2019;5:696-702. [Crossref] [PubMed]
- Raja R, Kuziora M, Brohawn PZ, et al. Early Reduction in ctDNA Predicts Survival in Patients with Lung and Bladder Cancer Treated with Durvalumab. Clin Cancer Res 2018;24:6212-22. [Crossref] [PubMed]
- Nie K, Jia Y, Zhang X. Cell-free circulating tumor DNA in plasma/serum of non-small cell lung cancer. Tumour Biol 2015;36:7-19. [Crossref] [PubMed]
- Chen M, Zhao H. Next-generation sequencing in liquid biopsy: cancer screening and early detection. Hum Genomics 2019;13:34. [Crossref] [PubMed]
- Warton K, Samimi G. Methylation of cell-free circulating DNA in the diagnosis of cancer. Front Mol Biosci 2015;2:13. [Crossref] [PubMed]
- Constâncio V, Nunes SP, Henrique R, et al. DNA Methylation-Based Testing in Liquid Biopsies as Detection and Prognostic Biomarkers for the Four Major Cancer Types. Cells 2020;9:624. [Crossref] [PubMed]
- Nebbioso A, Tambaro FP, Dell'Aversana C, et al. Cancer epigenetics: Moving forward. PLoS Genet 2018;14:e1007362 [Crossref] [PubMed]
- Belinsky SA. Gene-promoter hypermethylation as a biomarker in lung cancer. Nat Rev Cancer 2004;4:707-17. [Crossref] [PubMed]
- Heyn H, Esteller M. DNA methylation profiling in the clinic: applications and challenges. Nat Rev Genet 2012;13:679-92. [Crossref] [PubMed]
- Kulis M, Esteller M. DNA methylation and cancer. Adv Genet 2010;70:27-56. [Crossref] [PubMed]
- Lenka G, Tsai MH, Lin HC, et al. Identification of Methylation-Driven, Differentially Expressed STXBP6 as a Novel Biomarker in Lung Adenocarcinoma. Sci Rep 2017;7:42573. [Crossref] [PubMed]
- Buckingham L, Penfield Faber L, Kim A, et al. PTEN, RASSF1 and DAPK site-specific hypermethylation and outcome in surgically treated stage I and II nonsmall cell lung cancer patients. Int J Cancer 2010;126:1630-9. [PubMed]
- Costa-Pinheiro P, Montezuma D, Henrique R, et al. Diagnostic and prognostic epigenetic biomarkers in cancer. Epigenomics 2015;7:1003-15. [Crossref] [PubMed]
- Feng H, Zhang Z, Qing X, et al. Promoter methylation of APC and RAR-β genes as prognostic markers in non-small cell lung cancer (NSCLC). Exp Mol Pathol 2016;100:109-13. [Crossref] [PubMed]
- Ooki A, Maleki Z, Tsay JJ, et al. A Panel of Novel Detection and Prognostic Methylated DNA Markers in Primary Non-Small Cell Lung Cancer and Serum DNA. Clin Cancer Res 2017;23:7141-52. [Crossref] [PubMed]
- Wang Y, Wang Y, Wang Y, et al. Identification of prognostic signature of non-small cell lung cancer based on TCGA methylation data. Sci Rep 2020;10:8575. [Crossref] [PubMed]
- Zhang R, Chen C, Dong X, et al. Independent Validation of Early-Stage Non-Small Cell Lung Cancer Prognostic Scores Incorporating Epigenetic and Transcriptional Biomarkers With Gene-Gene Interactions and Main Effects. Chest 2020;158:808-19. [Crossref] [PubMed]
- Derrien T, Johnson R, Bussotti G, et al. The GENCODE v7 catalog of human long noncoding RNAs: analysis of their gene structure, evolution, and expression. Genome Res 2012;22:1775-89. [Crossref] [PubMed]
- Wang M, Ma X, Zhu C, et al. The prognostic value of long non coding RNAs in non small cell lung cancer: A meta-analysis. Oncotarget 2016;7:81292-304. [Crossref] [PubMed]
- Lu W, Zhang H, Niu Y, et al. Long non-coding RNA linc00673 regulated non-small cell lung cancer proliferation, migration, invasion and epithelial mesenchymal transition by sponging miR-150-5p. Mol Cancer 2017;16:118. [Crossref] [PubMed]
- Hua Q, Jin M, Mi B, et al. LINC01123, a c-Myc-activated long non-coding RNA, promotes proliferation and aerobic glycolysis of non-small cell lung cancer through miR-199a-5p/c-Myc axis. J Hematol Oncol 2019;12:91. [Crossref] [PubMed]
- Lin T, Fu Y, Zhang X, et al. A seven-long noncoding RNA signature predicts overall survival for patients with early stage non-small cell lung cancer. Aging (Albany NY) 2018;10:2356-66. [Crossref] [PubMed]
- Khalil AM, Guttman M, Huarte M, et al. Many human large intergenic noncoding RNAs associate with chromatin-modifying complexes and affect gene expression. Proc Natl Acad Sci U S A 2009;106:11667-72. [Crossref] [PubMed]
- Barlési F, Giaccone G, Gallegos-Ruiz MI, et al. Global histone modifications predict prognosis of resected non small-cell lung cancer. J Clin Oncol 2007;25:4358-64. [Crossref] [PubMed]
- Fukuoka J, Fujii T, Shih JH, et al. Chromatin remodeling factors and BRM/BRG1 expression as prognostic indicators in non-small cell lung cancer. Clin Cancer Res 2004;10:4314-24. [Crossref] [PubMed]
- Song S, Nguyen V, Schrank T, et al. Loss of SWI/SNF Chromatin Remodeling Alters NRF2 Signaling in Non-Small Cell Lung Carcinoma. Mol Cancer Res 2020;18:1777-88. [Crossref] [PubMed]
- Gray EP, Teare MD, Stevens J, et al. Risk Prediction Models for Lung Cancer: A Systematic Review. Clin Lung Cancer 2016;17:95-106. [Crossref] [PubMed]
- Duijm M, Oomen-de Hoop E, van Voort van der Zyp N, et al. The development and external validation of an overall survival nomogram in medically inoperable centrally located early-stage non-small cell lung carcinoma. Radiother Oncol 2021;156:223-30. [Crossref] [PubMed]
- Lin T, Mai J, Yan M, et al. A Nomogram Based on CT Deep Learning Signature: A Potential Tool for the Prediction of Overall Survival in Resected Non-Small Cell Lung Cancer Patients. Cancer Manag Res 2021;13:2897-906. [Crossref] [PubMed]
- Ran J, Cao R, Cai J, et al. Development and Validation of a Nomogram for Preoperative Prediction of Lymph Node Metastasis in Lung Adenocarcinoma Based on Radiomics Signature and Deep Learning Signature. Front Oncol 2021;11:585942 [Crossref] [PubMed]
- Zou X, Hu Z, Huang C, et al. A Seven-Gene Signature with Close Immune Correlation Was Identified for Survival Prediction of Lung Adenocarcinoma. Med Sci Monit 2020;26:e924269 [Crossref] [PubMed]
- Zhang X, Shi X, Zhao H, et al. Identification and Validation of a Tumor Microenvironment-Related Gene Signature for Prognostic Prediction in Advanced-Stage Non-Small-Cell Lung Cancer. Biomed Res Int 2021;2021:8864436 [PubMed]
- Wu C, Hu Q, Ma D. Development of an immune-related gene pairs signature for predicting clinical outcome in lung adenocarcinoma. Sci Rep 2021;11:3611. [Crossref] [PubMed]
- Yu X, Zhang X, Zhang Y. Identification of a 5-Gene Metabolic Signature for Predicting Prognosis Based on an Integrated Analysis of Tumor Microenvironment in Lung Adenocarcinoma. J Oncol 2020;2020:5310793 [Crossref] [PubMed]
- Zhao Z, He B, Cai Q, et al. A model of twenty-three metabolic-related genes predicting overall survival for lung adenocarcinoma. PeerJ 2020;8:e10008 [Crossref] [PubMed]
- Song C, Guo Z, Yu D, et al. A Prognostic Nomogram Combining Immune-Related Gene Signature and Clinical Factors Predicts Survival in Patients With Lung Adenocarcinoma. Front Oncol 2020;10:1300. [Crossref] [PubMed]
- Berdasco M, Esteller M. Clinical epigenetics: seizing opportunities for translation. Nat Rev Genet 2019;20:109-27. [Crossref] [PubMed]
- Saliakoura M, Rossi Sebastiano M, Pozzato C, et al. PLCγ1 suppression promotes the adaptation of KRAS-mutant lung adenocarcinomas to hypoxia. Nat Cell Biol 2020;22:1382-95. [Crossref] [PubMed]
- Salem A, Asselin MC, Reymen B, et al. Targeting Hypoxia to Improve Non-Small Cell Lung Cancer Outcome. J Natl Cancer Inst 2018; [Crossref] [PubMed]
- Gremke N, Polo P, Dort A, et al. mTOR-mediated cancer drug resistance suppresses autophagy and generates a druggable metabolic vulnerability. Nat Commun 2020;11:4684. [Crossref] [PubMed]
- Zhang X, Cao Y, Chen L. Construction of a prognostic signature of autophagy-related lncRNAs in non-small-cell lung cancer. BMC Cancer 2021;21:921. [Crossref] [PubMed]
- Gao X, Tang M, Tian S, et al. A ferroptosis-related gene signature predicts overall survival in patients with lung adenocarcinoma. Future Oncol 2021;17:1533-44. [Crossref] [PubMed]
- Zhang A, Yang J, Ma C, et al. Development and Validation of a Robust Ferroptosis-Related Prognostic Signature in Lung Adenocarcinoma. Front Cell Dev Biol 2021;9:616271 [Crossref] [PubMed]
- Wang J, Gong M, Xiong Z, et al. Immune-related prognostic genes signatures in the tumor microenvironment of sarcoma. Math Biosci Eng 2021;18:2243-57. [Crossref] [PubMed]
- Zhou N, Bao J. FerrDb: a manually curated resource for regulators and markers of ferroptosis and ferroptosis-disease associations. Database (Oxford) 2020;2020:baaa021.
- Salem A, Asselin MC, Reymen B, et al. Targeting Hypoxia to Improve Non-Small Cell Lung Cancer Outcome. J Natl Cancer Inst 2018; [Crossref] [PubMed]
- Cochrane CR, Vaghjiani V, Szczepny A, et al. Trp53 and Rb1 regulate autophagy and ligand-dependent Hedgehog signaling. J Clin Invest 2020;130:4006-18. [Crossref] [PubMed]
- Shen T, Cai LD, Liu YH, et al. Ube2v1-mediated ubiquitination and degradation of Sirt1 promotes metastasis of colorectal cancer by epigenetically suppressing autophagy. J Hematol Oncol 2018;11:95. [Crossref] [PubMed]
- He L, Chen J, Xu F, et al. Prognostic Implication of a Metabolism-Associated Gene Signature in Lung Adenocarcinoma. Mol Ther Oncolytics 2020;19:265-77. [Crossref] [PubMed]
- Chen Z, Yin K, Zheng D, et al. Marital status independently predicts non-small cell lung cancer survival: a propensity-adjusted SEER database analysis. J Cancer Res Clin Oncol 2020;146:67-74. [Crossref] [PubMed]
- Chen S, Gao C, Du Q, et al. A prognostic model for elderly patients with squamous non-small cell lung cancer: a population-based study. J Transl Med 2020;18:436. [Crossref] [PubMed]
- Jatoi A, Novotny P, Cassivi S, et al. Does marital status impact survival and quality of life in patients with non-small cell lung cancer? Observations from the mayo clinic lung cancer cohort. Oncologist 2007;12:1456-63. [Crossref] [PubMed]
- Tannenbaum SL, Zhao W, Koru-Sengul T, et al. Marital status and its effect on lung cancer survival. Springerplus 2013;2:504. [Crossref] [PubMed]
- Saito-Nakaya K, Nakaya N, Akechi T, et al. Marital status and non-small cell lung cancer survival: the Lung Cancer Database Project in Japan. Psychooncology 2008;17:869-76. [Crossref] [PubMed]
- Lin G, Qi K, Liu B, et al. A nomogram prognostic model for large cell lung cancer: analysis from the Surveillance, Epidemiology and End Results Database. Transl Lung Cancer Res 2021;10:622-35. [Crossref] [PubMed]
- Liao Y, Wang X, Zhong P, et al. A nomogram for the prediction of overall survival in patients with stage II and III non-small cell lung cancer using a population-based study. Oncol Lett 2019;18:5905-16. [Crossref] [PubMed]
- Dehing-Oberije C, Aerts H, Yu S, et al. Development and validation of a prognostic model using blood biomarker information for prediction of survival of non-small-cell lung cancer patients treated with combined chemotherapy and radiation or radiotherapy alone (NCT00181519, NCT00573040, and NCT00572325). Int J Radiat Oncol Biol Phys 2011;81:360-8. [Crossref] [PubMed]
- McMillan DC. The systemic inflammation-based Glasgow Prognostic Score: a decade of experience in patients with cancer. Cancer Treat Rev 2013;39:534-40. [Crossref] [PubMed]
- Diem S, Schmid S, Krapf M, et al. Neutrophil-to-Lymphocyte ratio (NLR) and Platelet-to-Lymphocyte ratio (PLR) as prognostic markers in patients with non-small cell lung cancer (NSCLC) treated with nivolumab. Lung Cancer 2017;111:176-81. [Crossref] [PubMed]
- Templeton AJ, McNamara MG, Šeruga B, et al. Prognostic role of neutrophil-to-lymphocyte ratio in solid tumors: a systematic review and meta-analysis. J Natl Cancer Inst 2014;106:dju124 [Crossref] [PubMed]
- Templeton AJ, Ace O, McNamara MG, et al. Prognostic role of platelet to lymphocyte ratio in solid tumors: a systematic review and meta-analysis. Cancer Epidemiol Biomarkers Prev 2014;23:1204-12. [Crossref] [PubMed]
- Zhang H, Xia H, Zhang L, et al. Clinical significance of preoperative neutrophil-lymphocyte vs platelet-lymphocyte ratio in primary operable patients with non-small cell lung cancer. Am J Surg 2015;210:526-35. [Crossref] [PubMed]
- Yang R, Chang Q, Meng X, et al. Prognostic value of Systemic immune-inflammation index in cancer: A meta-analysis. J Cancer 2018;9:3295-302. [Crossref] [PubMed]
- Wang Y, Li Y, Chen P, et al. Prognostic value of the pretreatment systemic immune-inflammation index (SII) in patients with non-small cell lung cancer: a meta-analysis. Ann Transl Med 2019;7:433. [Crossref] [PubMed]
- Mandaliya H, Jones M, Oldmeadow C, et al. Prognostic biomarkers in stage IV non-small cell lung cancer (NSCLC): neutrophil to lymphocyte ratio (NLR), lymphocyte to monocyte ratio (LMR), platelet to lymphocyte ratio (PLR) and advanced lung cancer inflammation index (ALI). Transl Lung Cancer Res 2019;8:886-94. [Crossref] [PubMed]
- Sandfeld-Paulsen B, Meldgaard P, Sorensen BS, et al. The prognostic role of inflammation-scores on overall survival in lung cancer patients. Acta Oncol 2019;58:371-6. [Crossref] [PubMed]
- Sandfeld-Paulsen B, Meldgaard P, Aggerholm-Pedersen N. Comorbidity in Lung Cancer: A Prospective Cohort Study of Self-Reported versus Register-Based Comorbidity. J Thorac Oncol 2018;13:54-62. [Crossref] [PubMed]
- Gillies RJ, Kinahan PE, Hricak H. Radiomics: Images Are More than Pictures, They Are Data. Radiology 2016;278:563-77. [Crossref] [PubMed]
- Carvalho S, Leijenaar RT, Velazquez ER, et al. Prognostic value of metabolic metrics extracted from baseline positron emission tomography images in non-small cell lung cancer. Acta Oncol 2013;52:1398-404. [Crossref] [PubMed]
- Coroller TP, Grossmann P, Hou Y, et al. CT-based radiomic signature predicts distant metastasis in lung adenocarcinoma. Radiother Oncol 2015;114:345-50. [Crossref] [PubMed]
- Kumar V, Gu Y, Basu S, et al. Radiomics: the process and the challenges. Magn Reson Imaging 2012;30:1234-48. [Crossref] [PubMed]
- Chetan MR, Gleeson FV. Radiomics in predicting treatment response in non-small-cell lung cancer: current status, challenges and future perspectives. Eur Radiol 2021;31:1049-58. [Crossref] [PubMed]
- van Timmeren JE, Leijenaar RTH, van Elmpt W, et al. Survival prediction of non-small cell lung cancer patients using radiomics analyses of cone-beam CT images. Radiother Oncol 2017;123:363-9. [Crossref] [PubMed]
- Zhang X, Cao Y, Chen L. Construction of a prognostic signature of autophagy-related lncRNAs in non-small-cell lung cancer. BMC Cancer 2021;21:921. [Crossref] [PubMed]
- Gao X, Tang M, Tian S, et al. A ferroptosis-related gene signature predicts overall survival in patients with lung adenocarcinoma. Future Oncol 2021;17:1533-44. [Crossref] [PubMed]
- Zhang A, Yang J, Ma C, et al. Development and Validation of a Robust Ferroptosis-Related Prognostic Signature in Lung Adenocarcinoma. Front Cell Dev Biol 2021;9:616271 [Crossref] [PubMed]
- Xu Y, Hosny A, Zeleznik R, et al. Deep Learning Predicts Lung Cancer Treatment Response from Serial Medical Imaging. Clin Cancer Res 2019;25:3266-75. [Crossref] [PubMed]
- Shi L, He Y, Yuan Z, et al. Radiomics for Response and Outcome Assessment for Non-Small Cell Lung Cancer. Technol Cancer Res Treat 2018;17:1533033818782788 [Crossref] [PubMed]
- Sollini M, Antunovic L, Chiti A, et al. Towards clinical application of image mining: a systematic review on artificial intelligence and radiomics. Eur J Nucl Med Mol Imaging 2019;46:2656-72. [Crossref] [PubMed]
- Park JE, Kim D, Kim HS, et al. Quality of science and reporting of radiomics in oncologic studies: room for improvement according to radiomics quality score and TRIPOD statement. Eur Radiol 2020;30:523-36. [Crossref] [PubMed]
- Nair JKR, Saeed UA, McDougall CC, et al. Radiogenomic Models Using Machine Learning Techniques to Predict EGFR Mutations in Non-Small Cell Lung Cancer. Can Assoc Radiol J 2021;72:109-19. [Crossref] [PubMed]
- Steyerberg EW, Harrell FE Jr. Regression Modeling Strategies: With Applications, to Linear Models, Logistic and Ordinal Regression, and Survival Analysis, 2nd ed. Heidelberg: Springer, 2016;72(3).
- Goecks J, Jalili V, Heiser LM, et al. How Machine Learning Will Transform Biomedicine. Cell 2020;181:92-101. [Crossref] [PubMed]
- Li Y, Ge D, Gu J, et al. A large cohort study identifying a novel prognosis prediction model for lung adenocarcinoma through machine learning strategies. BMC Cancer 2019;19:886. [Crossref] [PubMed]
- Tammemägi MC. Application of risk prediction models to lung cancer screening: a review. J Thorac Imaging 2015;30:88-100. [Crossref] [PubMed]
- Collins GS, Reitsma JB, Altman DG, et al. Transparent reporting of a multivariable prediction model for individual prognosis or diagnosis (TRIPOD): the TRIPOD statement. BMJ 2015;350:g7594. [Crossref] [PubMed]
- Chen L, Sui Y, Song C, et al. The sum of standardized residuals: Goodness-of-fit test for binary response models. Stat Med 2018;37:1932-41. [Crossref] [PubMed]
- Nattino G, Pennell ML, Lemeshow S. Assessing the goodness of fit of logistic regression models in large samples: A modification of the Hosmer-Lemeshow test. Biometrics 2020;76:549-60. [Crossref] [PubMed]
- Deist TM, Dankers FJWM, Valdes G, et al. Machine learning algorithms for outcome prediction in (chemo)radiotherapy: An empirical comparison of classifiers. Med Phys 2018;45:3449-59. [Crossref] [PubMed]
- Vickers AJ, Elkin EB. Decision curve analysis: a novel method for evaluating prediction models. Med Decis Making 2006;26:565-74. [Crossref] [PubMed]
- Harrell FE Jr, Lee KL, Mark DB. Multivariable prognostic models: issues in developing models, evaluating assumptions and adequacy, and measuring and reducing errors. Stat Med 1996;15:361-87. [Crossref] [PubMed]
- Jalali A, Alvarez-Iglesias A, Roshan D, et al. Visualising statistical models using dynamic nomograms. PLoS One 2019;14:e0225253 [Crossref] [PubMed]
- Bonnett LJ, Snell KIE, Collins GS, et al. Guide to presenting clinical prediction models for use in clinical settings. BMJ 2019;365:l737. [Crossref] [PubMed]
- Wolff RF, Moons KGM, Riley RD, et al. PROBAST: A Tool to Assess the Risk of Bias and Applicability of Prediction Model Studies. Ann Intern Med 2019;170:51-8. [Crossref] [PubMed]
- Moons KGM, Wolff RF, Riley RD, et al. PROBAST: A Tool to Assess Risk of Bias and Applicability of Prediction Model Studies: Explanation and Elaboration. Ann Intern Med 2019;170:W1-W33. [Crossref] [PubMed]
- Kreuzberger N, Damen JA, Trivella M, et al. Prognostic models for newly-diagnosed chronic lymphocytic leukaemia in adults: a systematic review and meta-analysis. Cochrane Database Syst Rev 2020;7:CD012022 [PubMed]
- . Ontario Health (Quality) . Gene Expression Profiling Tests for Early-Stage Invasive Breast Cancer: A Health Technology Assessment. Ont Health Technol Assess Ser 2020;20:1-234.
- Palazón-Bru A, Mares-García E, López-Bru D, et al. A critical appraisal of the clinical applicability and risk of bias of the predictive models for mortality and recurrence in patients with oropharyngeal cancer: Systematic review. Head Neck 2020;42:763-73. [Crossref] [PubMed]
- Aladwani M, Lophatananon A, Ollier W, et al. Prediction models for prostate cancer to be used in the primary care setting: a systematic review. BMJ Open 2020;10:e034661 [Crossref] [PubMed]
(English Language Editor: B. Draper)