Identification of m6A methyltransferase-related genes predicts prognosis and immune infiltrates in head and neck squamous cell carcinoma
Introduction
Head and neck squamous cell carcinoma (HNSCC) is a major global public health problem, with a high rate of recurrence and distant metastasis (1). It is a heterogeneous disease even though the tumors all originate in the epithelial cells of the mucosal linings of the upper airway and food passages (2). The onset of HNSC is hidden and the early clinical symptoms are not obvious, so patients are mostly in the middle and late stages of disease at the time of diagnosis. Studies have shown that the occurrence and metastasis of HNSCC is complex, involving multiple genes. Therefore, searching for genetic and molecular markers related to the occurrence and development of HNSCC will help to better evaluate its prognosis and find new treatment methods, thereby improving the survival rate of HNSCC patients. Meanwhile, it is very important to understand the tumor immune microenvironment of HNSCC and identify valuable biomarkers. One research has developed an immune-related genetic prognostic index (IRGPI), and after analyzing different molecular and immune characteristics. It is found that in the classification of HNSCC immune subtypes, IRGPI-high subtypes are higher than IRGPI-low subtypes. In the high subgroup of IRGPI, cytotoxic CD8 T cells, CD4 T cells and M1 macrophages are more enriched. B cells and M0 and M2 macrophages are more enriched in the IRGPI low subgroup.
The most common post-transcriptional modification in eukaryotic RNA is N6-methyladenosine (m6A) (3). This modification mainly occurs in the DRACH (D = A, G, U; R = A, G; H = A, C, U) in various RNAs with adenine in the sequence (4). As early as the 1970s, scientists discovered the m6A modification, which is significantly concentrated in the messenger RNA (mRNA) stop codon and 3' non-coding region (5), which can dynamically regulate biological processes such as cell differentiation. Although m6A modification has been discovered for more than 40 years, it is difficult to detect due to its poor stability and short life span (6). The current research found that m6A is a reversible dynamic process, and its function is jointly determined by the “Writer”, “Eraser”, and “Reader”. The “Writer” is also called methyltransferase complex, the known components of which include METL3 (7), METL14, and WTAP (8,9). The “Erase”, also called demethylase, including ALKBH5 (10) and FTO (11), can reverse methylation n. The functions of m6A are recognized by the m6A binding protein, which is the “reader”. The currently discovered m6A binding proteins include the YTH (YT521-B homology) domain protein family, which is YTHDF1, YTHDF2, YTHDF3 (12), YTHDC1 (13), and YTHDC2 (5) and the HNRNP family of heterogeneous nuclear proteins, namely HNRNPA2B1 and HNRNPC (14).
The evolutionarily conserved YTH domain contained in the YTH protein family can selectively recognize m6A (13). The family member YTHDF1 promotes the binding of target RNA to ribosomes by interacting with initiation factors, and enhancing mRNA translation and protein synthesis (15). Additionally, YTHDF2 reduces mRNA stability and accelerates mRNA degradation by recruiting CCR4-NOT complexes (12,16). The effect of YTHDF3 is bidirectional. It can not only interact with YTHDF1 to enhance RNA translation but also combine with YTHDF2 to promote RNA degradation (17). A function of YTHDC1 is to affect the splicing and output of RNA, and YTHDC2 can promote the translation efficiency of target RNA (18).
More and more evidences show that m6A mRNA methylation is closely related to the occurrence and development of tumors, and m6A modification has different biological functions in different types of tumors. Glioblastoma stem cells (GSCs) are a kind of stem cells with strong invasive ability. In recent years, studies have found that GBM is closely related to the level of m6A increases and the induced differentiated of m6A moCs. Inhibiting the expression of METTL3 or METTL14 can reduce m6A levels, enhance the growth and self-renewal of GSCs in vitro, and promote the ability of GSCs to form tumors in vivo. In addition, METL3 is significantly up-regulated in human hepatocellular carcinoma cells. Overexpression of METL3 can promote HCC proliferation and migration, and significantly enhance HCC tumor formation. Suppressor of cytokine signaling 2 (SOCS2) is a downstream target of METTL3 and also a tumor suppressor. METTL3 reduces the stability of SOCS2 mRNA through the m6A-YTHDF2-dependent pathway to promote the occurrence and development of HCC. Studies have shown that m6A methylation-related genes are involved in the occurrence and development of many diseases, but there is very little research regarding their role in in HNSCC. The purpose of this study was to explore the effect of m6A methylation-related genes on the prognosis of HNSCC and their relationships with tumor immune infiltration. We present the following article in accordance with the REMARK reporting checklist (available at https://dx.doi.org/10.21037/atm-21-4712).
Methods
The TCGA-HNSCC cohort data collection
The RNA-seq data (Fragments Per Kilobase per Million (FPKM)) of the HNSCC dataset, corresponding clinical information, and somatic mutation data were obtained from TCGA GDC (https://portal.gdc.cancer.gov/). The RNA sequencing data (FPKM values) were then transformed to transcripts per million reads (TPM) and normalized into log2 (TPM +1). Subsequently, data analysis was performed in R language (R version 3.6.3; https://cran.r-project.org/bin/windows/base/old/3.6.3/). The study was conducted in accordance with the Declaration of Helsinki (as revised in 2013).
Tumor-infiltrating immune cells corresponding to TCGA-HNSCC cohort
The Immune Cell Abundance Identifier (ImmuCellAI) is an online tool to estimate the abundance of 24 immune cells from gene expression dataset, in which the 24 immune cells are comprised of 18 T-cell subtypes and 6 other immune cells: B cell, natural killer (NK) cell, monocyte cell, macrophage cell, neutrophil cell, and dendritic cell (DC) (http://bioinfo.life.hust.edu.cn/web/ImmuCellAI/) (19). The infiltrating data of tumor-infiltrating immune cells (TIICs) corresponding to TCGA-HNSCC samples were downloaded from the ImmuCellAI website.
Construction of the m6A methylation-related gene signature
The least absolute shrinkage and selection operator (LASSO)-Cox regression model was performed for variable screening and complexity adjustment to screen potential genes to establish the m6A methylation-related gene signature as previously described. The penalty value parameter was determined after 10-fold cross-validation using the “glmnet” package (20). Finally, a formula was developed using the gene signature constructed above. Risk score = expression of a gene (YTHDC2) × corresponding coefficient (−0.297) + expression of a gene (HNRNPA2B1) × corresponding coefficient (−0.752) + expression of gene (RBM15B) × corresponding coefficient (−0.332) (21,22). Patients of the TCGA-HNSCC cohort were divided into high and low-risk score groups.
Gene set enrichment analysis
To assess the potential differences in biological functions between the high- and low-risk score subgroups, gene set enrichment analysis (GSEA) software (https://www.gsea-msigdb.org/gsea/login.jsp) was used based on the hallmarks gene set (“h.all.v7.0.symbols.gmt”) as previously described (22).
Additional bioinformatic and statistical analysis
We used R software (version 3.6.3, https://www.r-project.org/) to perform analyses and plot graphs. Wilcoxon’s rank-sum test was used to analyze the parameters of different groups. Correlations between the levels of m6A methylation-related genes in HNSCC were also analyzed using Spearman correlation. A consensus cluster consisting of 20 m6A methylation-related genes was constructed by using the “ConsensusClusterPlus” package. The ImmuneScore was developed by using the “ESTIMATE” packages. The “survival” package was used to perform survival analysis (https://CRAN.R-project.org/package=survival). The best critical value was determined using the R packages (“survival” and “survminer”) and a double-sided log-rank test. Kaplan-Meier survival curves and log-rank tests were used to compare the differences in survival between high- and low-risk score groups. The R package “survivalROC” was used to plot the time-dependent receiver operating characteristic (ROC) curve to determine the prognostic value of the signature with OS in HNSCC. Visualization of analysis results was performed using the R packages ggplot2 (http://ggplot2.org). A P value of <0.05 was considered statistically significant.
Results
Patient characteristics
We downloaded RNAseq and clinical data in HNSCC tumor and normal tissues from the TCGA dataset. After excluding cases with insufficient OS time, or lack of data, our study included a total of 502 patients (368 males and 134 females). The median age of the participants was 61 years. Clinical data were collected including age, gender, grade, race, clinical stage, history of smoking and alcohol, tumor, node, metastasis (TNM) stage, and pathological grade (Table S1).
Expression of m6A methylation-related genes in HNSC
To understand the expression pattern of m6A methylation-related genes between HNSCC tumors and normal tissues, we constructed a gene expression heat map of m6A methylation-related genes based on the TCGA-HNSCC cohort. Compared with adjacent normal tissues, 18 genes in tumor tissues showed significant upregulation, including IGF2BP2, IGF2BP1, IGF2BP3, VIRMA, YTHDF1, YTHDF2, YTHDF3, ZC3H13, METTL14, ALKBH5, METTL3, RBMX, WTAP, YTHDC1, FTO, HNRNPC, HNRNPA2B1, and RBM15 (Figure 1A) (P<0.05). Pearson correlation analysis showed that all 20 genes were positively correlated. Among them, VIRMA and YTHDF3 had the highest correlation, with a correlation coefficient of 0.86 (Figure 1B).
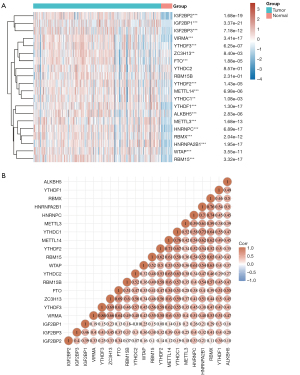
The ImmuneScore in differentially expressed m6A methylation-related genes level in HNSCC
The ImmuneScores were calculated through the ESTIMATE evaluation method, and the immune score in differentially expressed m6A methylation-related gene groups were analyzed (Figure 2, Figure S1). As shown in Figure 2A, patients with high expression of YTHDC1 had lower immune scores than those with low YTHDC1. In addition, 9 m6A methylation-related genes were observed to be negatively correlated with immune score, including IGF2BP1, IGF2BP3, YTHDF1, VIRMA, METTL3, HNRNPA2B1, ALKBH5, IGF2BP2, and HNRNPC (P<0.05). Immune score showed no differences in 8 differentially expressed m6A methylation-related genes groups (Figure S1) (P>0.05).
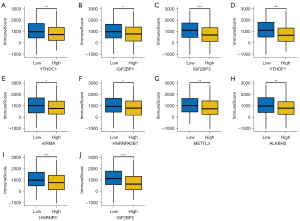
The prognostic values of m6A methylation-related genes in HNSCC
We next performed overall survival (OS) analysis for m6A methylation-related genes in HNSCC. The HNSCC patients with higher expression of HNRNPA2B1, HNRNPC, METTL3, IGFBP2, IGFBP1, and IGFBP3 had a poorer prognosis; however, those with a higher expression of YTHDC1 and YTHDC2 had a better prognosis (Figure 3). Considering the possibility of non-tumor death during follow-up, we analyzed the relationship between m6A methylation-related genes expression and disease-specific survival (DSS) in HNSCC. For DSS, increased expression of YTHDC2 was correlated with better clinical outcomes in HNSCC patients (Figure 4). Increased expression of IGFBP2, METTL3, HNRNPA2B1, and HNRNPC genes were correlated with poor clinical outcomes in HNSCC patients. Taken together, these data suggested that partially m6A methylation-related genes could be used as a biomarker predicting unfavorable prognosis in HNSCC patients.
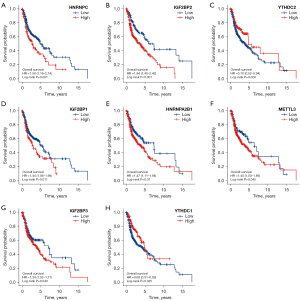
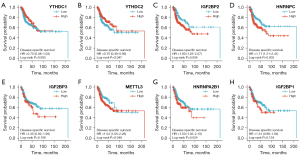
Consensus clustering identified 3e clusters of patients with HNSCC
Based on the expression levels of 20 m6A methylation-related genes, we divided 502 tumor samples into 3 different subgroups (Cluster1, Cluster2, and Cluster3) (Figure 5A,5B). The tracking plot for k=2 to k=6 is demonstrated in Figure 5C. The OS varied significantly between 3 clusters (Figure 5D; P=0.027). Clusters 1 (median, 6.1 years) and 2 (median, 6.1 years) had the highest and lowest hazard ratios, respectively.
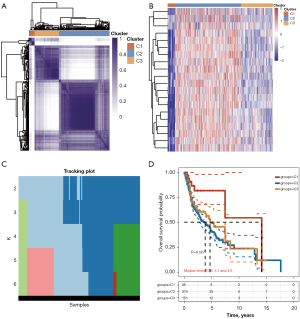
Construction of the 3-m6A methylation-related genes-based classifier
We used a LASSO Cox regression model to build a superior prognostic classifier, which selected 3 m6A methylation-related genes from 4 m6A methylation-related genes (IGF2BP2, YTHDC2, HNRNPA2B1, and RBM15B) identified in the TCGA-HNSCC cohort: YTHDC2, HNRNPA2B1, and RBM15B (Figure 6A,6B). Using the LASSO Cox regression models, we calculated a risk score for each patient based on their individual expression levels of the 3 m6A methylation-related genes, where risk score = (−0.297 × status of YTHDC2) + (−0.752 × status of HNRNPA2B1) + (−0.332 × status of RBM15B). As shown in Figure 6C, compared with low-risk patients, high-risk patients had significantly worse OS (P<0.05). The time-dependent ROC curve showed that the area under the curve (AUC) of the 3-, 5-, and 8-year OS in the cohort were 0.62, 0.603, and 0.643, respectively (Figure 6D). Thus, the 3-m6A methylation-related genes-based classifier performed well in predicting OS in HNSCC patients.
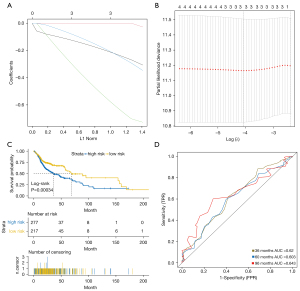
Immune characteristics of 3-m6A methylation-related genes-based classifier subgroups
Wilcoxon test was used to analyze the composition of immune cells in 3-m6A methylation-related genes-based classifier subgroups. We found that B cells, CD4 T cells, CD8 T cells, iTreg, NK, Tc, Tcm, Tex, Tfh, Tgd, Th1 cells, Th2 cells, and Tr1 cells were more abundant in the low-risk score subgroup, while CD8 naive cells, CD4 naive cells, DC, monocytes, neutrophils, natural killer T cells (NKT), and Th17 cells were more abundant in the high risk-score subgroup (Figure 7).
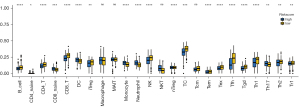
Molecular characteristics of patients in the high- and low-risk score subgroup
We used GSEA software to find the difference between the high-risk score subgroup and the low-risk score subgroup in the Hallmark pathway. As shown in Figure 8A,8B, in the high-risk group, the first 5 pathways include EPITHELIAL_MESENCHYMAL_TRANSITION, MTORC1_SIGNALING, MYC_TARGETS_V1, MYC_TARGETS_V2, and MYOGENESIS. The first 5 pathways in the low-risk group are ALLOGRAFT_REJECTION, COMPLEMENT, IL6_JAK_STAT3_SIGNALING, INTERFERON_ALPHA_RESPONSE, and INTERFERON_GAMMA_RESPONSE. We further analyzed the gene mutations in the high-risk group and the low-risk group and identified the top 10 genes with the highest mutation rate (Figure 8C,8D). Among them, the mutation rates of TP53, TIN, and FAT were greater than 20% in both the high-risk group and the low-risk group of HNSCC patients. In addition, TP53 mutations were the most abundant in the high-risk group and were significantly more common than in the low-risk group (80% vs. 52%).
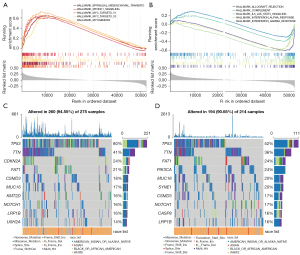
Discussion
The occurrence and metastasis of HNSCC is a complex process involving multiple genes. Therefore, searching for genes and molecular markers related to HNSCC will help us better evaluate the prognosis of HNSCC patients. There is only one study that has reported a correlation between m6A and HNSCC, in which METTL3-mediated inhibition of ZNF750 promoted the progression of NPC. Previous studies have shown that METTL3 can promote apoptosis of a variety of tumor cells, such as breast cancer and gastric cancer. Therefore, in order to predict the prognosis of HNSCC patients, we used TCGA to establish 20 gene characteristics. The expression of all genes was positively correlated, and 18 m6A methylation-related genes were significantly up-regulated in HNSCC (including IGF2BP2, IGF2BP1, IGF2BP3, VIRMA, YTHDF1, YTHDF2, YTHDF3, ZC3H13, METTL14, ALKBH5, METTL3, RBMX, WTAP, YTHDC1, FTO, HNRNPC, HNRNPA2B1, and RBM15) (P<0.05). In addition, we also analyzed the relationship between these 18 m6A methylation-related genes and immune scores. The study showed that 10 m6A methylation-related genes were negatively correlated with immune scores (including YTHDC1, IGF2BP1, IGF2BP3, YTHDF1, VIRMA, METTL3, HNRNPA2B1, ALKBH5, IGF2BP2, and HNRNPC) (P<0.05). Next, the Gene Expression Profiling Interactive Analysis (GEPIA) database was used to analyze the most differential survival genes of HNSCC. The differential expression of the remaining eight m6A methylation-related genes did not differ from the immune score (P>0.05). Our research also showed that YTHDC2 and YTHDC1 can be used as protective genes because their high expression suggests a better prognosis, while the IGFBP2, METTL3, HNRNPA2B1, and HNRNPC genes are associated with and indicative of a poor prognosis. Our results show that the expression levels of YTHDC1 and YTHDC2 are positively correlated with the prognosis of HNSCC and are expected to be prognostic biomarkers of HNSCC. These findings indicate m6A modulators can play a potential role in the treatment of HNSCC.
According to the expression levels of 20 m6A methylation-related genes, we used R’s ConsensusClusterPlus package to group the data into 3 subgroups. The overall analysis showed the highest and lowest risk ratios of subgroup 1 and subgroup 2 stages, indicating that the dynamic time is related to the comprehensive expression level of m6A methylation-related genes.
The LASSO algorithm simultaneously analyzes all independent variables and selects the most influential variable (21). According to LASSO Cox analysis, 3 m6A methylation-related genes (YTHDC2, HNRNPA2B1, and RBM15B) were selected from the 4 m6A methylation-related genes (IGF2BP2, YTHDC2, HNRNPA2B1, and RBM15B) identified in the TCGA-HNSCC cohort, and calculating their risk-scores, it was shown that YTHDC2, HNRNPA2B1, and RBM15B high-risk patients had significantly worse OS (P<0.05). The results indicate that m6A methylation-related genes can independently predict the prognosis of HNSCC patients. Studies have shown that YTHDC2 can specifically recognize and bind m6A through the YTH sequence, promote mRNA translation and affect mRNA stability (4). The YTHDC2 gene is essential for the maintenance of meiosis and the production of germ cells (23-25), and is involved in the occurrence and development of a variety of diseases, including autism, pancreatic cancer, colon adenocarcinoma, liver cancer, and lung adenocarcinoma (26-30). The HNRNPA2B1 gene was highly expressed in a variety of human cancers, such as prostate cancer (31), pancreatic cancer (32), and hepatocellular carcinoma (33). It has been reported that RBM15B participates in the evolution of a variety of solid tumors, such as when Zhang et al. (34) found that RBM15B has at least 2 mutated possible carcinogenic sites in primary epithelial ovarian cancer through next-generation high-throughput sequencing. Shahriyari et al. (35) found that it was highly positively correlated with the expression of RBM15B. The BRCA1 associated protein-1 (BPA1) is a prognostic gene for uveal melanoma and breast cancer. In addition, RBM15B is also associated with an increased risk of malignant mesothelioma and renal clear cell carcinoma (26,36,37). This is consistent with our research results.
Understanding the status of the tumor microenvironment (TME) can help find new ways to treat HNSCC or change TME to improve the effectiveness of immunotherapy. We divided the 3 m6A methylation-related genes into high- and low-risk groups and analyzed the composition of immune cells between the 2 subgroups. The results showed that B cells, cytotoxic CD8 T cells, and CD4 T cells were more abundant in the low-risk subgroup, while inflammatory cells such as neutrophils, monocytes, and DC cells were more common in the high-risk subgroup. A large number of studies have shown that dense infiltration of T cells, especially cytotoxic CD8 T cells, indicates a good prognosis (38-40). While tumors stimulate neutrophils to promote tumor migration, invasion, and metastasis (41), acute and chronic inflammation are conducive to tumor growth and the development of aggressive phenotypes. Our research results support these conclusions. In addition, GSEA showed that the high-risk subgroup of m6A methylation-related genes has immunosuppressive effects and is related to high TP53 mutations, while the low-risk subgroup is related to active immunity and low TP53 mutations.
Our research has several limitations. First of all, the sample size of this study is small, and it only focuses on the mRNA sequencing data from TCGA. Future work is necessary to further improve the sample size of HNSCC patients and further explore other public databases to obtain more verification from independent cohorts to confirm the results. Secondly, our conclusion lacks certain clinical practice, so more clinical studies are needed to confirm whether m6A-related genes can be used as new targets for HNSCC treatment. In conclusion, our study has determined the risk characteristics of m6A methylation-related genes, which can be independently used to predict the prognosis of HNSCC patients and is related to immune infiltration, providing a new treatment strategy for HNSCC patients.
Acknowledgments
Funding: None.
Footnote
Reporting Checklist: The authors have completed the REMARK reporting checklist. Available at https://dx.doi.org/10.21037/atm-21-4712
Conflicts of Interest: All authors have completed the ICMJE uniform disclosure form (available at https://dx.doi.org/10.21037/atm-21-4712). The authors have no conflicts of interest to declare.
Ethical Statement: The authors are accountable for all aspects of the work in ensuring that questions related to the accuracy or integrity of any part of the work are appropriately investigated and resolved. The study was conducted in accordance with the Declaration of Helsinki (as revised in 2013).
Open Access Statement: This is an Open Access article distributed in accordance with the Creative Commons Attribution-NonCommercial-NoDerivs 4.0 International License (CC BY-NC-ND 4.0), which permits the non-commercial replication and distribution of the article with the strict proviso that no changes or edits are made and the original work is properly cited (including links to both the formal publication through the relevant DOI and the license). See: https://creativecommons.org/licenses/by-nc-nd/4.0/.
References
- Sung H, Ferlay J, Siegel RL, et al. Global Cancer Statistics 2020: GLOBOCAN Estimates of Incidence and Mortality Worldwide for 36 Cancers in 185 Countries. CA Cancer J Clin 2021;71:209-49. [Crossref] [PubMed]
- Leemans CR, Snijders PJF, Brakenhoff RH. The molecular landscape of head and neck cancer. Nat Rev Cancer 2018;18:269-82. [Crossref] [PubMed]
- Batista PJ. The RNA Modification N6-methyladenosine and Its Implications in Human Disease. Genomics Proteomics Bioinformatics 2017;15:154-63. [Crossref] [PubMed]
- Hsu PJ, Zhu Y, Ma H, et al. Ythdc2 is an N6-methyladenosine binding protein that regulates mammalian spermatogenesis. Cell Res 2017;27:1115-27. [Crossref] [PubMed]
- Dominissini D, Moshitch-Moshkovitz S, Schwartz S, et al. Topology of the human and mouse m6A RNA methylomes revealed by m6A-seq. Nature 2012;485:201-6. [Crossref] [PubMed]
- Desrosiers R, Friderici K, Rottman F. Identification of methylated nucleosides in messenger RNA from Novikoff hepatoma cells. Proc Natl Acad Sci U S A 1974;71:3971-5. [Crossref] [PubMed]
- Bokar JA, Shambaugh ME, Polayes D, et al. Purification and cDNA cloning of the AdoMet-binding subunit of the human mRNA (N6-adenosine)-methyltransferase. RNA 1997;3:1233-47. [PubMed]
- Ping XL, Sun BF, Wang L, et al. Mammalian WTAP is a regulatory subunit of the RNA N6-methyladenosine methyltransferase. Cell Res 2014;24:177-89. [Crossref] [PubMed]
- Liu J, Yue Y, Han D, et al. A METTL3-METTL14 complex mediates mammalian nuclear RNA N6-adenosine methylation. Nat Chem Biol 2014;10:93-5. [Crossref] [PubMed]
- Zheng G, Dahl JA, Niu Y, et al. ALKBH5 is a mammalian RNA demethylase that impacts RNA metabolism and mouse fertility. Mol Cell 2013;49:18-29. [Crossref] [PubMed]
- Jia G, Fu Y, Zhao X, et al. N6-methyladenosine in nuclear RNA is a major substrate of the obesity-associated FTO. Nat Chem Biol 2011;7:885-7. [Crossref] [PubMed]
- Wang X, Lu Z, Gomez A, et al. N6-methyladenosine-dependent regulation of messenger RNA stability. Nature 2014;505:117-20. [Crossref] [PubMed]
- Xu C, Wang X, Liu K, et al. Structural basis for selective binding of m6A RNA by the YTHDC1 YTH domain. Nat Chem Biol 2014;10:927-9. [Crossref] [PubMed]
- Liu N, Dai Q, Zheng G, et al. N(6)-methyladenosine-dependent RNA structural switches regulate RNA-protein interactions. Nature 2015;518:560-4. [Crossref] [PubMed]
- Lotan TL, Torres A, Zhang M, et al. Somatic molecular subtyping of prostate tumors from HOXB13 G84E carriers. Oncotarget 2017;8:22772-82. [Crossref] [PubMed]
- Du H, Zhao Y, He J, et al. YTHDF2 destabilizes m(6)A-containing RNA through direct recruitment of the CCR4-NOT deadenylase complex. Nat Commun 2016;7:12626. [Crossref] [PubMed]
- Wang X, Zhao BS, Roundtree IA, et al. N(6)-methyladenosine Modulates Messenger RNA Translation Efficiency. Cell 2015;161:1388-99. [Crossref] [PubMed]
- Li X, Li J, Cai Y, et al. Hyperglycaemia-induced miR-301a promotes cell proliferation by repressing p21 and Smad4 in prostate cancer. Cancer Lett 2018;418:211-20. [Crossref] [PubMed]
- Miao YR, Zhang Q, Lei Q, et al. ImmuCellAI: A Unique Method for Comprehensive T-Cell Subsets Abundance Prediction and its Application in Cancer Immunotherapy. Adv Sci (Weinh) 2020;7:1902880 [Crossref] [PubMed]
- Shahraki HR, Salehi A, Zare N. Survival Prognostic Factors of Male Breast Cancer in Southern Iran: a LASSO-Cox Regression Approach. Asian Pac J Cancer Prev 2015;16:6773-7. [Crossref] [PubMed]
- Friedman J, Hastie T, Tibshirani R. Regularization Paths for Generalized Linear Models via Coordinate Descent. J Stat Softw 2010;33:1-22. [Crossref] [PubMed]
- Dai X, Jiang W, Ma L, et al. A metabolism-related gene signature for predicting the prognosis and therapeutic responses in patients with hepatocellular carcinoma. Ann Transl Med 2021;9:500. [Crossref] [PubMed]
- Soh YQS, Mikedis MM, Kojima M, et al. Meioc maintains an extended meiotic prophase I in mice. PLoS Genet 2017;13:e1006704 [Crossref] [PubMed]
- Bailey AS, Batista PJ, Gold RS, et al. The conserved RNA helicase YTHDC2 regulates the transition from proliferation to differentiation in the germline. Elife 2017;6:26116. [Crossref] [PubMed]
- Jain D, Puno MR, Meydan C, et al. ketu mutant mice uncover an essential meiotic function for the ancient RNA helicase YTHDC2. Elife 2018;7:30919. [Crossref] [PubMed]
- Fanale D, Iovanna JL, Calvo EL, et al. Germline copy number variation in the YTHDC2 gene: does it have a role in finding a novel potential molecular target involved in pancreatic adenocarcinoma susceptibility? Expert Opin Ther Targets 2014;18:841-50. [Crossref] [PubMed]
- Xu D, Shao J, Song H, et al. The YTH Domain Family of N6-Methyladenosine "Readers" in the Diagnosis and Prognosis of Colonic Adenocarcinoma. Biomed Res Int 2020;2020:9502560 [PubMed]
- Tanabe A, Tanikawa K, Tsunetomi M, et al. RNA helicase YTHDC2 promotes cancer metastasis via the enhancement of the efficiency by which HIF-1α mRNA is translated. Cancer Lett 2016;376:34-42. [Crossref] [PubMed]
- Ma L, Chen T, Zhang X, et al. The m6A reader YTHDC2 inhibits lung adenocarcinoma tumorigenesis by suppressing SLC7A11-dependent antioxidant function. Redox Biol 2021;38:101801 [Crossref] [PubMed]
- Li Y, Zheng JN, Wang EH, et al. The m6A reader protein YTHDC2 is a potential biomarker and associated with immune infiltration in head and neck squamous cell carcinoma. PeerJ 2020;8:e10385 [Crossref] [PubMed]
- Stockley J, Villasevil ME, Nixon C, et al. The RNA-binding protein hnRNPA2 regulates β-catenin protein expression and is overexpressed in prostate cancer. RNA Biol 2014;11:755-65. [Crossref] [PubMed]
- Siveke JT. The increasing diversity of KRAS signaling in pancreatic cancer. Gastroenterology 2014;147:736-9. [Crossref] [PubMed]
- Shilo A, Ben Hur V, Denichenko P, et al. Splicing factor hnRNP A2 activates the Ras-MAPK-ERK pathway by controlling A-Raf splicing in hepatocellular carcinoma development. RNA 2014;20:505-15. [Crossref] [PubMed]
- Zhang L, Luo M, Yang H, et al. Next-generation sequencing-based genomic profiling analysis reveals novel mutations for clinical diagnosis in Chinese primary epithelial ovarian cancer patients. J Ovarian Res 2019;12:19. [Crossref] [PubMed]
- Shahriyari L, Abdel-Rahman M, Cebulla C. BAP1 expression is prognostic in breast and uveal melanoma but not colon cancer and is highly positively correlated with RBM15B and USP19. PLoS One 2019;14:e0211507 [Crossref] [PubMed]
- Louie BH, Kurzrock R. BAP1: Not just a BRCA1-associated protein. Cancer Treat Rev 2020;90:102091 [Crossref] [PubMed]
- Abdel-Rahman MH, Pilarski R, Cebulla CM, et al. Germline BAP1 mutation predisposes to uveal melanoma, lung adenocarcinoma, meningioma, and other cancers. J Med Genet 2011;48:856-9. [Crossref] [PubMed]
- Bindea G, Mlecnik B, Tosolini M, et al. Spatiotemporal dynamics of intratumoral immune cells reveal the immune landscape in human cancer. Immunity 2013;39:782-95. [Crossref] [PubMed]
- Fridman WH, Zitvogel L, Sautès-Fridman C, et al. The immune contexture in cancer prognosis and treatment. Nat Rev Clin Oncol 2017;14:717-34. [Crossref] [PubMed]
- Gentles AJ, Newman AM, Liu CL, et al. The prognostic landscape of genes and infiltrating immune cells across human cancers. Nat Med 2015;21:938-45. [Crossref] [PubMed]
- Rapoport BL, Steel HC, Theron AJ, et al. Role of the Neutrophil in the Pathogenesis of Advanced Cancer and Impaired Responsiveness to Therapy. Molecules 2020;25:1618. [Crossref] [PubMed]
(English Language Editor: J. Jones)