Association between serum iron status and primary liver cancer risk: a Mendelian randomization analysis
Introduction
Primary liver cancer (PLC) is a commonly diagnosed gastrointestinal carcinoma. According to the recently published global statistics, PLC was the sixth most commonly diagnosed cancer and the third leading cause of cancer death worldwide in 2020, with approximately 906,000 new cases and 830,000 deaths (1). Incidence of PLC had shown a decreasing trend in many high-risk countries in Asia, including China, South Korea, and the Philippines over the last 3 decades (2); however, an unfavorable increasing trend was noted in some developed countries where PLC was less diagnosed (2). Owing to the poor prognosis in clinical practice, PLC imposes a heavy disease burden on human health and warrants higher primacy in future schemes of disease prevention.
The malignancy of PLC is deemed to be multi-etiological and involves many risk factors (3,4). For example, previous studies have reported that iron status, which is measured in clinical practice as serum iron, ferritin, transferrin, and transferrin saturation, is associated with PLC risk (5-7). Mechanisms whereby iron may act in carcinogenesis are induction of oxidative stress, facilitation of tumor growth, and modification of the immune system (8). However, the links between serum status and PLC development from observational studies might be biased by underlying confounders and might only reflect an indirect association through other factors (i.e., aging, alcohol consumption, and insulin resistance) (8). Whether iron status plays a role in PLC development remains inconclusive. To address this need, herein, we conducted a 2-sample Mendelian randomization (MR) analysis to determine the causal link between biomarkers for iron status and PLC. The MR is an approach using genetic variants as instrumental variables for assessing causal relationships from observational data and has been widely used to infer the relationship between exposures and outcomes (9-12). We present the following article in accordance with the STROBE reporting checklist (available at https://dx.doi.org/10.21037/atm-21-4608).
Methods
Summary statistics of serum iron status and PLC
We retrieved the genetic data of serum iron status from the online platform of Integrative Epidemiology Unit (IEU) open genome-wide association study (GWAS) project (https://gwas.mrcieu.ac.uk/) with searching codes of “ieu-a-1049”, “ieu-a-1050”, “ieu-a-1051”, and “ieu-a-1052” for serum iron, ferritin, transferrin saturation, and transferrin, respectively. The genetic data of serum iron status were derived from a GWAS consisting of 48,972 European individuals (13). In this GWAS, discovery samples consisted of summary data on genome-wide allelic associations between SNP genotypes and iron markers from 23,986 participants of European ancestry gathered from 11 cohorts in 9 participating centers. Replication samples to confirm suggestive and significant associations were obtained from up to 24,986 participants of European ancestry in 8 additional cohorts. Genome-wide association tests, genotype imputation, and associated quality control procedures were performed in each cohort separately (13). The association between genotyped and imputed SNPs and each iron phenotype was performed using an additive model for allelic effects, on the standardized residuals of the phenotype after adjusting for age, principal component scores, and other study specific covariates, for each gender separately (13).
The genetic data of PLC were retrieved from the online database of FinnGen (https://r4.finngen.fi/), which is a public-private partnership project combining genotype data from Finnish biobanks and digital health record data from Finnish health registries (14). In GWAS of PLC, 266 PLC cases and 176,633 controls were included. Individuals with ambiguous gender, high genotype missingness (>5%), excess heterozygosity, and non-Finnish ancestry were excluded. Variants with high missingness (>2%), low Hardy-Weinberg equilibrium test P value (<10-6), and minor allele count <3 were excluded. The study was conducted in accordance with the Declaration of Helsinki (as revised in 2013).
Selection of genetic instruments
We extracted SNPs showing a significant association with serum iron status at the conventional GWAS threshold (P<5×10-8). We performed a clumping process based on the linkage disequilibrium (LD) estimates from the European samples in 1,000 genomes project. Herein, the LD threshold was set as 0.1 and the window size was 10,000 kb. Among those pairs of SNPs that had LD estimate >0.1, we only retained the SNP with the lower P value. The SNPs with a minor allele frequency <1% were removed. We then retrieved the genetic statistics of the selected SNPs from the PLC GWAS summary data. For SNPs that were absent in the PLC GWAS, we alternatively retrieved data of SNP proxy that had LD estimate >0.8 with the requested SNP.
Statistical analysis
We first tested the horizontal pleiotropy using Mendelian Randomization Pleiotropy RESidual Sum and Outlier (MR-PRESSO) global test and removed the outliers (i.e., SNPs with P<0.05) if the horizontal pleiotropy was presented. Next, we tested the between-SNP heterogeneity using the inverse variance weighting (IVW) method. The Cochran’s Q statistic was used to measure the heterogeneity. In the main analysis, IVW method with fixed-effect was applied if no between-SNP heterogeneity was detected, otherwise IVW method with random-effect was used. We also conducted a set of sensitivity analyses using MR-Egger regression, weighted median, and weighted mode methods. The MR-Egger regression is based on the INstrument Strength Independent of Direct Effect (InSIDE) assumption and consists of 3 parts: (I) a test for directional pleiotropy; (II) a test for a causal effect; and (III) an estimate of the causal effect (15). The analyses of the 4 MR methods were carried out using the TwoSampleMR package in R program (version 3.6.3; https://www.r-project.org/). We chose the main MR method as follows (16): (I) if no directional pleiotropy was detected, use IVW; (II) if directional pleiotropy was detected in MR-Egger regression, use MR-Egger; and (III) if directional pleiotropy and heterogeneity were both detected, use weighted median. Given the potential correlations between biomarkers of iron status, we also conducted a multivariate MR analysis.
Results
Quality control for genetic instruments
In this analysis, we included 22, 5, 36, and 41 independent genetic variants in MR analysis for serum iron, ferritin, transferrin saturation, and transferrin, respectively (Table 1). We did not detect horizontal pleiotropy for each biomarker of iron status in the MR-PRESSO global test (P value was 0.258, 0.354, 0.289, and 0.758 for serum iron, ferritin, transferrin saturation, and transferrin, respectively). Additionally, no evidence of between-SNP heterogeneity and directional pleiotropy was detected by the Cochran’s Q test and MR-Egger regression for serum iron, ferritin, and transferrin (Table 2). For transferrin saturation, although no heterogeneity was detected, the directional pleiotropy was significant (P value for intercept of MR-Egger regression =0.033). As a result, we reported MR estimates of IVW method for serum iron, ferritin, and transferrin, whereas estimates of MR-Egger regression for transferrin saturation.
Table 1
Rs ID | Chromosome | Position | Genes | Effect allele | Other allele | Beta | Se | P value |
---|---|---|---|---|---|---|---|---|
Serum iron | ||||||||
rs10434845 | 6 | 25582757 | LRRC16A | G | C | 0.1047 | 0.0101 | 3.77E-25 |
rs11756569 | 6 | 26323026 | NA | T | A | 0.0748 | 0.0134 | 2.28E-08 |
rs12216125 | 6 | 25997458 | TRIM38, HIST1H1A | T | C | 0.1535 | 0.0103 | 1.18E-50 |
rs13209646 | 6 | 28600128 | NA | A | T | 0.097 | 0.0147 | 4.01E-11 |
rs1525892 | 3 | 133484712 | TF, SRPRB | A | G | 0.0736 | 0.0104 | 1.65E-12 |
rs1800562 | 6 | 26093141 | HFE, HIST1H4C, HIST1H1T | A | G | 0.3724 | 0.02 | 3.96E-77 |
rs1927695 | 6 | 25378113 | LRRC16A | G | A | 0.1066 | 0.0136 | 4.08E-15 |
rs198855 | 6 | 26103398 | HFE, HIST1H4C, HIST1H1T | T | A | −0.0752 | 0.0112 | 1.88E-11 |
rs2074504 | 6 | 30530245 | GNL1, PRR3, ABCF1 | C | T | −0.063 | 0.0111 | 1.57E-08 |
rs2160907 | 22 | 37435900 | TST, MPST, KCTD17 | G | A | 0.1225 | 0.0141 | 3.76E-18 |
rs2744257 | 6 | 25267748 | LOC101928663, LRRC16A | C | T | −0.0679 | 0.0115 | 3.10E-09 |
rs407934 | 6 | 25504562 | LRRC16A | T | C | 0.0744 | 0.0103 | 4.80E-13 |
rs4712955 | 6 | 25684279 | SCGN | G | A | 0.068 | 0.0106 | 1.56E-10 |
rs518700 | 6 | 25889553 | SLC17A3 | A | T | 0.0707 | 0.0108 | 6.46E-11 |
rs5756492 | 22 | 37424991 | TST, MPST | A | G | 0.0817 | 0.011 | 1.08E-13 |
rs7286184 | 22 | 37478775 | KCTD17, TMPRSS6 | G | A | −0.129 | 0.0124 | 1.74E-25 |
rs806794 | 6 | 26200677 | HIST1H2BE, HIST1H4D, HIST1H3D, HIST1H2AD, | G | A | −0.0691 | 0.0112 | 7.34E-10 |
rs855791 | 22 | 37462936 | HIST1H2BF, HIST1H4E, HIST1H2BG, HIST1H2AE | G | A | 0.1868 | 0.0101 | 4.31E-77 |
rs916213 | 22 | 37397550 | KCTD17, TMPRSS6 | C | T | 0.0789 | 0.01 | 2.20E-15 |
rs926331 | 22 | 37510072 | TEX33, TST, MPST | T | C | 0.0756 | 0.0119 | 2.01E-10 |
rs9358858 | 6 | 25446308 | TMPRSS6, IL2RB | T | G | 0.0807 | 0.0111 | 3.30E-13 |
rs9358928 | 6 | 26341030 | LRRC16A | T | C | 0.0953 | 0.0108 | 1.31E-18 |
Ferritin | ||||||||
rs12216125 | 6 | 25997458 | TRIM38, HIST1H1A | T | C | 0.0687 | 0.0097 | 1.20E-12 |
rs12693541 | 2 | 190418690 | SLC40A1 | T | C | −0.106 | 0.014 | 4.18E-14 |
rs1800562 | 6 | 26093141 | HFE, HIST1H4C, HIST1H1T | A | G | 0.211 | 0.0187 | 1.42E-29 |
rs2413450 | 22 | 37470224 | KCTD17, TMPRSS6 | C | T | 0.0559 | 0.0095 | 3.57E-09 |
rs368243 | 17 | 56708979 | TEX14 | C | T | −0.0512 | 0.0093 | 3.80E-08 |
Transferrin saturation | ||||||||
rs1015811 | 6 | 28448086 | NA | G | A | 0.0833 | 0.0117 | 1.03E-12 |
rs10434845 | 6 | 25582757 | LRRC16A | G | C | 0.1414 | 0.0101 | 3.16E-44 |
rs10946813 | 6 | 26345141 | NA | A | G | −0.0772 | 0.0104 | 8.90E-14 |
rs12196939 | 6 | 27787990 | HIST1H2BL, HIST1H2AI, HIST1H3H, HIST1H2AJ, HIST1H2BM, HIST1H4J, HIST1H4K, HIST1H2AK, HIST1H2BN | A | G | −0.0916 | 0.0133 | 6.76E-12 |
rs12216125 | 6 | 25997458 | TRIM38, HIST1H1A | T | C | 0.2047 | 0.0103 | 2.29E-87 |
rs1233333 | 6 | 29795421 | HLA-G | A | G | 0.1063 | 0.014 | 3.24E-14 |
rs13209646 | 6 | 28600128 | NA | A | T | 0.1599 | 0.0148 | 3.36E-27 |
rs13215804 | 6 | 28415572 | ZSCAN23 | G | A | 0.0617 | 0.0108 | 1.04E-08 |
rs1736919 | 6 | 29697517 | HLA-F, HLA-F-AS1 | G | A | −0.0732 | 0.0115 | 1.93E-10 |
rs1799852 | 3 | 133475722 | TF | T | C | 0.1458 | 0.0183 | 1.51E-15 |
rs1800562 | 6 | 26093141 | HFE, HIST1H4C, HIST1H1T | A | G | 0.5772 | 0.0203 | 1.52E-178 |
rs1927695 | 6 | 25378113 | LRRC16A | G | A | 0.1577 | 0.0137 | 8.34E-31 |
rs198839 | 6 | 26112620 | HFE, HIST1H4C, HIST1H1T, HIST1H2BC, HIST1H2AC | G | T | −0.0889 | 0.0112 | 2.64E-15 |
rs2097775 | 6 | 30354303 | NA | T | A | −0.1087 | 0.014 | 8.12E-15 |
rs2160907 | 22 | 37435900 | TST, MPST, KCTD17 | G | A | 0.1203 | 0.0141 | 1.86E-17 |
rs221834 | 7 | 100343175 | ZAN | G | C | 0.1226 | 0.0205 | 2.38E-09 |
rs2393915 | 6 | 27080460 | HIST1H2BJ | C | A | −0.0556 | 0.01 | 2.78E-08 |
rs2744258 | 6 | 25268014 | LOC101928663, LRRC16A | C | A | −0.0924 | 0.0115 | 1.03E-15 |
rs301397 | 6 | 25464492 | LRRC16A | C | T | 0.0724 | 0.0109 | 2.86E-11 |
rs3129157 | 6 | 29141743 | OR2J2 | G | A | −0.1548 | 0.0281 | 3.46E-08 |
rs3804111 | 6 | 25602926 | LRRC16A | G | T | −0.063 | 0.0099 | 2.13E-10 |
rs407934 | 6 | 25504562 | LRRC16A | T | C | 0.108 | 0.0103 | 1.24E-25 |
rs4712955 | 6 | 25684279 | SCGN | G | A | 0.1003 | 0.0106 | 4.05E-21 |
rs4712972 | 6 | 25772047 | SLC17A4, SLC17A1 | G | A | 0.1093 | 0.0149 | 2.57E-13 |
rs518700 | 6 | 25889553 | SLC17A3 | A | T | 0.0951 | 0.0109 | 1.94E-18 |
rs5756492 | 22 | 37424991 | TST, MPST | A | G | 0.0809 | 0.011 | 2.02E-13 |
rs6939576 | 6 | 28669315 | NA | A | G | −0.0685 | 0.011 | 5.63E-10 |
rs7286184 | 22 | 37478775 | KCTD17, TMPRSS6 | G | A | −0.1344 | 0.0124 | 2.05E-27 |
rs7759489 | 6 | 24950599 | FAM65B | T | C | 0.0767 | 0.0137 | 2.20E-08 |
rs7762821 | 6 | 25335094 | LRRC16A | G | C | 0.0834 | 0.0119 | 2.19E-12 |
rs7773004 | 6 | 26267755 | HIST1H3F, HIST1H2BH, HIST1H3G, HIST1H2BI, HIST1H4H | G | A | −0.1172 | 0.01 | 4.93E-32 |
rs8177272 | 3 | 133482870 | TF | A | G | −0.097 | 0.0106 | 5.52E-20 |
rs855791 | 22 | 37462936 | KCTD17, TMPRSS6 | G | A | 0.1921 | 0.0101 | 3.50E-80 |
rs916213 | 22 | 37397550 | TEX33, TST, MPST | C | T | 0.0806 | 0.01 | 8.94E-16 |
rs926331 | 22 | 37510072 | TMPRSS6, IL2RB | T | C | 0.0724 | 0.012 | 1.43E-09 |
rs9358858 | 6 | 25446308 | LRRC16A | T | G | 0.119 | 0.0112 | 1.70E-26 |
Transferrin | ||||||||
rs10434845 | 6 | 25582757 | LRRC16A | G | C | −0.1101 | 0.0104 | 2.74E-26 |
rs10456324 | 6 | 25600968 | LRRC16A | A | G | 0.0574 | 0.0102 | 1.87E-08 |
rs1051772 | 3 | 133327457 | CDV3, TOPBP1 | C | T | −0.1196 | 0.017 | 2.25E-12 |
rs10946813 | 6 | 26345141 | NA | A | G | 0.0667 | 0.0106 | 2.81E-10 |
rs1105877 | 3 | 133561095 | RAB6B | C | T | −0.0865 | 0.0124 | 3.00E-12 |
rs1107413 | 3 | 133524717 | SRPRB, RAB6B | C | G | −0.161 | 0.0116 | 9.74E-44 |
rs11757000 | 6 | 28484869 | GPX6, GPX5 | C | T | −0.161 | 0.0148 | 2.14E-27 |
rs11963067 | 6 | 28560071 | ZBED9 | T | A | 0.085 | 0.0137 | 6.27E-10 |
rs1233333 | 6 | 29795421 | HLA-G | A | G | −0.1105 | 0.0144 | 2.01E-14 |
rs129128 | 6 | 26125342 | HIST1H1T, HIST1H2BC, HIST1H2AC | T | C | 0.1155 | 0.0143 | 7.42E-16 |
rs13080154 | 3 | 133475722 | NA | C | T | 0.1855 | 0.0108 | 9.98E-66 |
rs1410440 | 6 | 25415730 | LRRC16A | A | C | −0.0579 | 0.0104 | 2.93E-08 |
rs1444598 | 3 | 133553985 | NA | C | T | 0.1091 | 0.0104 | 8.24E-26 |
rs1444600 | 3 | 133599961 | CDV3 | T | C | 0.1365 | 0.0105 | 2.64E-38 |
rs1495741 | 8 | 18272881 | NAT2 | A | G | 0.0825 | 0.0122 | 1.57E-11 |
rs174577 | 11 | 61604814 | FADS2 | A | C | 0.0684 | 0.0107 | 1.90E-10 |
rs1799852 | 3 | 133476852 | TF | T | C | −0.3777 | 0.0191 | 1.72E-87 |
rs1800562 | 6 | 26093141 | HFE, HIST1H4C, HIST1H1T | A | G | −0.5496 | 0.0208 | 1.26E-153 |
rs2032447 | 6 | 26044369 | HIST1H4B, HIST1H3B, HIST1H2AB, HIST1H2BB, HIST1H3C, HIST1H1C | G | A | −0.0952 | 0.0108 | 1.07E-18 |
rs2393915 | 6 | 27080460 | HIST1H2BJ | C | A | 0.0822 | 0.0102 | 8.02E-16 |
rs2690097 | 6 | 25346742 | LRRC16A | C | T | −0.0741 | 0.012 | 5.69E-10 |
rs2692670 | 3 | 190378750 | SRPRB, RAB6B | C | T | −0.1663 | 0.013 | 1.06E-37 |
rs2715630 | 3 | 133699575 | RAB6B | T | C | 0.083 | 0.0147 | 1.61E-08 |
rs3094124 | 6 | 30711805 | TUBB, FLOT1, IER3 | G | C | 0.1537 | 0.0178 | 5.25E-18 |
rs3094188 | 6 | 31142245 | CCHCR1, TCF19, POU5F1, PSORS1C3 | A | C | 0.0592 | 0.0106 | 2.04E-08 |
rs3811658 | 3 | 133476852 | TF | T | C | 0.3883 | 0.0109 | 1.00E-200 |
rs407934 | 6 | 25504562 | LRRC16A | T | C | −0.0895 | 0.0106 | 3.33E-17 |
rs4711080 | 6 | 25189192 | NA | C | A | 0.0674 | 0.0113 | 2.74E-09 |
rs4712955 | 6 | 25684279 | SCGN | G | A | −0.0898 | 0.0108 | 1.17E-16 |
rs5009711 | 6 | 25333090 | LRRC16A | T | C | −0.1468 | 0.0144 | 2.06E-24 |
rs6923367 | 6 | 25745852 | HIST1H2AA, HIST1H2BA, HIST1H2APS1, SLC17A4 | T | A | −0.3798 | 0.021 | 3.09E-73 |
rs722086 | 6 | 25428954 | LRRC16A | T | C | −0.0886 | 0.0134 | 3.96E-11 |
rs744653 | 2 | 133492471 | NA | T | C | 0.0916 | 0.0144 | 2.00E-10 |
rs7646392 | 3 | 195827205 | SLCO2A1 | T | C | −0.0615 | 0.0105 | 4.49E-09 |
rs7762537 | 6 | 25334967 | LRRC16A | C | A | −0.0936 | 0.0122 | 1.42E-14 |
rs9268633 | 6 | 32406473 | HLA-DRA | G | A | 0.0717 | 0.0128 | 2.31E-08 |
rs9358858 | 6 | 25446308 | LRRC16A | T | G | −0.1108 | 0.0115 | 3.99E-22 |
rs9358928 | 6 | 26341030 | NA | T | C | −0.109 | 0.0111 | 9.07E-23 |
rs9405124 | 6 | 29368813 | OR12D2 | G | A | −0.0792 | 0.0111 | 1.19E-12 |
rs9824452 | 3 | 1.33E+08 | TF, SRPRB | A | G | −0.3321 | 0.0149 | 1.28E-110 |
rs9990333 | 3 | 1.96E+08 | TFRC | T | C | −0.067 | 0.0101 | 3.01E-11 |
The nearest genes were detected within a flanking distance of 20 kb. NA, not available.
Table 2
Variables | Iron | Ferritin | Transferrin saturation | Transferrin |
---|---|---|---|---|
Inverse variance weighted | ||||
OR (95% CI) | 0.71 (0.45, 1.11) | 0.56 (0.16, 2.04) | 0.91 (0.66, 1.26) | 0.91 (0.72, 1.15) |
Q statistics (P value) | 16.24 (0.436) | 1.02 (0.796) | 29.70 (0.429) | 28.40 (0.738) |
MR-Egger | ||||
OR (95% CI) | 0.53 (0.18, 1.57) | 1.09 (0.05, 22.18) | 0.45 (0.22, 0.90) | 1.26 (0.85, 1.87) |
Q statistics (P value) | 15.89 (0.389) | 0.795 (0.672) | 24.65 (0.647) | 24.61 (0.853) |
Intercept (P value) | 0.034 (0.574) | −0.062 (0.681) | 0.093 (0.033) | −0.058 (0.060) |
Weighted median | ||||
OR (95% CI) | 0.66 (0.35, 1.26) | 0.62 (0.09, 4.10) | 0.80 (0.49, 1.28) | 1.05 (0.76, 1.45) |
Weighted mode | ||||
OR (95% CI) | 0.68 (0.37, 1.24) | 0.58 (0.14, 2.40) | 0.80 (0.51, 1.26) | 1.05 (0.75, 1.47) |
MR, Mendelian randomization; OR, odds ratio; CI, confidence interval.
Univariate MR analysis
The estimated effect sizes of the SNPs on both the exposures (serum iron, ferritin, transferrin saturation, and transferrin) and outcome (PLC) are displayed in scatter plots (Figure 1). According to the MR analysis, we estimated that there was no causal link between serum iron [odds ratio (OR) =0.71; 95% confidence interval (CI): 0.45 to 1.11], ferritin (OR =0.56, 95% CI: 0.16 to 2.04), and transferrin (OR =0.91, 95% CI: 0.72 to 1.15) and PLC risk (Table 2). The findings were consistent with that of sensitivity analyses by another 3 MR methods. By contrast, we found a significant causal relationship between transferrin saturation and PLC risk (OR =0.45, 95% CI: 0.22 to 0.90), although this link was not significant according to methods other than MR-Egger regression.
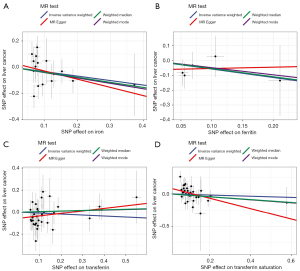
Multivariate MR analysis
In the multivariable MR analysis, a total of 76 genetic variants were included. No evidence of between-SNP heterogeneity (Q statistics =66.10, P=0.610) and directional pleiotropy (intercept of MR-Egger regression =−0.011, P=0.748) was detected (Table 3). The IVW method estimated that there was no significantly causal relationship between the four biomarkers of iron status and PLC risk, which were concordant with estimates from the other three methods (Table 3).
Table 3
Variables | Iron | Ferritin | Transferrin saturation | Transferrin |
---|---|---|---|---|
Inverse variance weighted | ||||
OR (95% CI) | 1.22 (0.91, 1.64) | 1.00 (0.63, 1.57) | 1.14 (0.77, 1.67) | 0.94 (0.85, 1.03) |
Q statistics (P value) | 66.10 (0.610) | |||
MR-Egger | ||||
OR (95% CI) | 1.28 (0.78, 2.08) | 1.00 (0.60, 1.68) | 1.17 (0.96, 1.41) | 0.94 (0.81, 1.10) |
Q statistics (P value) | 60.82 (0.758) | |||
Intercept (P value) | −0.011 (0.748) | |||
Weighted median | ||||
OR (95% CI) | 0.99 (0.64, 1.55) | 1.00 (0.50, 1.98) | 1.01 (0.74, 1.39) | 0.92 (0.75, 1.12) |
Lasso method | ||||
OR (95% CI) | 1.15 (0.85, 1.56) | 0.99 (0.59, 1.66) | 1.16 (0.96, 1.41) | 0.94 (0.81, 1.10) |
MR, Mendelian randomization; OR, odds ratio; CI, confidence interval; Lasso, least absolute shrinkage and selection operator.
Discussion
In this 2-sample MR study, we estimated the causal link between serum status and PLC risk based on genetic summary data from two previously large-scale GWASs. According to the estimates of MR analyses, we found there was no significant causal relationship between serum iron, ferritin, and transferrin and risk of PLC development. We detected a significant causal effect of transferrin saturation on PLC, although this effect was not significant in multivariable MR analysis. Our findings provide novel insights into the association of serum iron status with PLC genesis.
Links between serum iron status and PLC risk have been reported in previous epidemiological and experimental studies (17,18). A recent meta-analysis reported an association between high serum ferritin and PLC risk (HR =1.49, 95% CI: 1.13 to 1.96) and high serum iron and PLC risk (HR =2.47, 95% CI: 1.31 to 4.63) (5). The previous results obtained from observational studies were not in line with our findings. The potential explanations for this inconsistence might be as follows. First, previous studies involved small numbers of studies and sample size, leading to wide confidence intervals for the association estimates. Additionally, estimates from observational studies might be subject to the inherent defects of residual confounding and reverse causality (19). Findings obtained from experimental studies were not conclusive regarding the direct carcinogenic effect of iron status on the liver, whereas indirect pathways were revealed between iron and PLC such as promoting oxidative stress, cell death, and compensatory proliferation (20). In this regard, the abnormal iron status might be a result of liver carcinogenesis. The causal relationship between iron status and PLC development is debatable and needs further investigations.
In a recent 2-sample MR analysis, Yuan et al. reported that genetically predicted iron status was positively associated with liver cancer (21). However, only 3 SNPs were used to serve as genetic instruments of iron status in this study, and thus may have led to a weak instrumental variable bias. On the contrary, in our study, we used all SNPs that reached the GWAS significance threshold as the genetic instruments after quality-control processes. Different to results of Yuan et al., we found no causal relationship between serum iron, ferritin, and transferrin and risk of PLC. This inconsistency might be attributed to the following reasons: (I) different genetic instrumental tools used; and (II) summary data of PLC GWAS were derived from different populations: Yuan et al. retrieved from genetic data from UK Biobank, in which the number of PLC cases included in GWAS was less than that of FinnGen (https://pan.ukbb.broadinstitute.org/phenotypes/index.html). Since the GWAS results were largely dependent on sample size and ratio of case-control (22), we speculate that the variations in genetic data of PLC resulted in the aforementioned inconsistence. Future studies based on a more robust GWAS are therefore warranted.
We also found a putative causal link between genetically predicted transferrin saturation and PLC risk. However, this link disappeared in the multivariable MR analysis. Our results suggested that there was no compelling evidence with respect to a direct role of iron status in PLC development, although establishment of this link had been attempted in previous experimental studies (18,23). Iron overload and iron deficiency are both related to significant abnormalities in immune function (24). The most mentioned mechanism by which excess iron may promote liver carcinogenesis is through DNA damage from the production of reactive oxygen species, especially hydroxyl radical (25). However, of note is that iron overload was also deemed to be a hepatic presentation of alcoholic liver diseases, which is a well-determined etiology for liver cancer. Alcoholic liver diseases are associated with significant oxidative stress as well as the hepatic accumulation of iron, a transition element also documented to initiate oxidative stress (26). Alcohol intake is therefore an important confounder to consider the observed correlations of iron status with PLC risk.
In our study, we included several genetic variants such as rs1800562 and rs198855 in HFE and rs855791 in TMPRSS6 that show a robust and consistent association with a systemic iron status. Mutations in HFE and TMPRSS6 were reported to be associated with hereditary hemochromatosis (HH) (27,28), which is a risk factor for the development of liver carcinoma (29). However, the population-attributable fraction of HH might be small due to HH being rarely diagnosed in general population. On the other hand, variants that directly cause HH were not always associated with elevated risk of liver cancer (30). In this regard, HH could only be termed as a risk factor rather than an etiology like viral hepatitis for liver cancer. In additional, liver iron metabolism signatures were related to survival, disease status, and prognosis in patients with hepatocellular carcinoma. These findings suggested an important role of iron in the development and progression of liver cancer.
The limitations of our study should be noted here. First, our results were based on genetic data from European populations, which limited the possibility of extrapolation to other populations. Second, the genetic summary data of PLC were derived from a GWAS with a small case size and an unbalanced case-control ratio, which might limit the statistical power and introduce variations into the MR estimates.
In summary, our study found that there might be no causal relationship between iron status and liver cancer risk. The previously observed links might be confounded by underlying factors and require further validation.
Acknowledgments
Funding: This work was financially supported by the National Natural Science Foundation of China (No. 81802983; No. 82072759), and Shanghai rising star program (No. 20QA1412000).
Footnote
Reporting Checklist: The authors have completed the STROBE reporting checklist. Available at https://dx.doi.org/10.21037/atm-21-4608
Conflicts of Interest: All authors have completed the ICMJE uniform disclosure form (available at https://dx.doi.org/10.21037/atm-21-4608). The authors have no conflicts of interest to declare.
Ethical Statement: The authors are accountable for all aspects of the work in ensuring that questions related to the accuracy or integrity of any part of the work are appropriately investigated and resolved. The study was conducted in accordance with the Declaration of Helsinki (as revised in 2013).
Open Access Statement: This is an Open Access article distributed in accordance with the Creative Commons Attribution-NonCommercial-NoDerivs 4.0 International License (CC BY-NC-ND 4.0), which permits the non-commercial replication and distribution of the article with the strict proviso that no changes or edits are made and the original work is properly cited (including links to both the formal publication through the relevant DOI and the license). See: https://creativecommons.org/licenses/by-nc-nd/4.0/.
References
- Sung H, Ferlay J, Siegel RL, et al. Global Cancer Statistics 2020: GLOBOCAN Estimates of Incidence and Mortality Worldwide for 36 Cancers in 185 Countries. CA Cancer J Clin 2021;71:209-49. [Crossref] [PubMed]
- Liu Z, Jiang Y, Yuan H, et al. The trends in incidence of primary liver cancer caused by specific etiologies: Results from the Global Burden of Disease Study 2016 and implications for liver cancer prevention. J Hepatol 2019;70:674-83. [Crossref] [PubMed]
- McGlynn KA, Petrick JL, El-Serag HB. Epidemiology of Hepatocellular Carcinoma. Hepatology 2021;73:4-13. [Crossref] [PubMed]
- Kulik L, El-Serag HB. Epidemiology and Management of Hepatocellular Carcinoma. Gastroenterology 2019;156:477-491.e1. [Crossref] [PubMed]
- Tran KT, Coleman HG, McCain RS, et al. Serum Biomarkers of Iron Status and Risk of Primary Liver Cancer: A Systematic Review and Meta-Analysis. Nutr Cancer 2019;71:1365-73. [Crossref] [PubMed]
- Bian Z, Hann HW, Ye Z, et al. Ferritin level prospectively predicts hepatocarcinogenesis in patients with chronic hepatitis B virus infection. Oncol Lett 2018;16:3499-508. [Crossref] [PubMed]
- Wen CP, Lee JH, Tai YP, et al. High serum iron is associated with increased cancer risk. Cancer Res 2014;74:6589-97. [Crossref] [PubMed]
- Deugnier Y. Iron and liver cancer. Alcohol 2003;30:145-50. [Crossref] [PubMed]
- Yavorska OO, Burgess S. MendelianRandomization: an R package for performing Mendelian randomization analyses using summarized data. Int J Epidemiol 2017;46:1734-9. [Crossref] [PubMed]
- Sekula P, Del Greco M F, Pattaro C, et al. Mendelian Randomization as an Approach to Assess Causality Using Observational Data. J Am Soc Nephrol 2016;27:3253-65. [Crossref] [PubMed]
- Li X, Meng X, Timofeeva M, et al. Serum uric acid levels and multiple health outcomes: umbrella review of evidence from observational studies, randomised controlled trials, and Mendelian randomisation studies. BMJ 2017;357:j2376. [Crossref] [PubMed]
- Telomeres Mendelian Randomization Collaboration. Association Between Telomere Length and Risk of Cancer and Non-Neoplastic Diseases: A Mendelian Randomization Study. JAMA Oncol 2017;3:636-51. [Crossref] [PubMed]
- Benyamin B, Esko T, Ried JS, et al. Novel loci affecting iron homeostasis and their effects in individuals at risk for hemochromatosis. Nat Commun 2014;5:4926. [Crossref] [PubMed]
- Locke AE, Steinberg KM, Chiang CWK, et al. Exome sequencing of Finnish isolates enhances rare-variant association power. Nature 2019;572:323-8. [Crossref] [PubMed]
- Burgess S, Thompson SG. Interpreting findings from Mendelian randomization using the MR-Egger method. Eur J Epidemiol 2017;32:377-89. [Crossref] [PubMed]
- Dong SS, Zhang K, Guo Y, et al. Phenome-wide investigation of the causal associations between childhood BMI and adult trait outcomes: a two-sample Mendelian randomization study. Genome Med 2021;13:48. [Crossref] [PubMed]
- Ellervik C, Tybjaerg-Hansen A, Nordestgaard BG. Risk of cancer by transferrin saturation levels and haemochromatosis genotype: population-based study and meta-analysis. J Intern Med 2012;271:51-63. [Crossref] [PubMed]
- Muto Y, Moroishi T, Ichihara K, et al. Disruption of FBXL5-mediated cellular iron homeostasis promotes liver carcinogenesis. J Exp Med 2019;216:950-65. [Crossref] [PubMed]
- Murphy N, Carreras-Torres R, Song M, et al. Circulating Levels of Insulin-like Growth Factor 1 and Insulin-like Growth Factor Binding Protein 3 Associate With Risk of Colorectal Cancer Based on Serologic and Mendelian Randomization Analyses. Gastroenterology 2020;158:1300-1312.e20. [Crossref] [PubMed]
- Fu Y, Chung FL. Oxidative stress and hepatocarcinogenesis. Hepatoma Res 2018;4:39. [Crossref] [PubMed]
- Yuan S, Carter P, Vithayathil M, et al. Iron Status and Cancer Risk in UK Biobank: A Two-Sample Mendelian Randomization Study. Nutrients 2020;12:526. [Crossref] [PubMed]
- Jiang W, Yu W. Erratum to: Power estimation and sample size determination for replication studies of genome-wide association studies. BMC Genomics 2017;18:73. [Crossref] [PubMed]
- Sikorska K, Bernat A, Wroblewska A. Molecular pathogenesis and clinical consequences of iron overload in liver cirrhosis. Hepatobiliary Pancreat Dis Int 2016;15:461-79. [Crossref] [PubMed]
- McCord JM. Iron, free radicals, and oxidative injury. J Nutr 2004;134:3171S-2S. [Crossref] [PubMed]
- Seitz HK, Stickel F. Risk factors and mechanisms of hepatocarcinogenesis with special emphasis on alcohol and oxidative stress. Biol Chem 2006;387:349-60. [Crossref] [PubMed]
- Petersen DR. Alcohol, iron-associated oxidative stress, and cancer. Alcohol 2005;35:243-9. [Crossref] [PubMed]
- Guo S, Casu C, Gardenghi S, et al. Reducing TMPRSS6 ameliorates hemochromatosis and β-thalassemia in mice. J Clin Invest 2013;123:1531-41. [Crossref] [PubMed]
- Valenti L, Fracanzani AL, Rametta R, et al. Effect of the A736V TMPRSS6 polymorphism on the penetrance and clinical expression of hereditary hemochromatosis. J Hepatol 2012;57:1319-25. [Crossref] [PubMed]
- Elmberg M, Hultcrantz R, Ekbom A, et al. Cancer risk in patients with hereditary hemochromatosis and in their first-degree relatives. Gastroenterology 2003;125:1733-41. [Crossref] [PubMed]
- Atkins JL, Pilling LC, Masoli JAH, et al. Association of Hemochromatosis HFE p.C282Y Homozygosity With Hepatic Malignancy. JAMA 2020;324:2048-57. [Crossref] [PubMed]