Immunological perspective on the malignant progression of renal clear cell carcinoma
Introduction
Renal cell carcinoma (RCC) is the most common of all primary malignant kidney tumors in adults and includes the “classical” or “eosinophilic” variant of chromophobe RCC. Despite new targeted therapies having improved progression-free survival (PFS) and overall survival (OS) for patients with early-stage RCC, for those with stage IV disease, the 5-year survival rate remains below 10%, and 50% of patients survive for less than 1 year (1). In Europe, there are an estimated around seventy thousand new cases of clear cell renal cell carcinoma (ccRCC) and around thirty thousand related deaths each year (2,3). However, no reliable biomarkers to predict the prognosis of ccRCC have been discovered (4,5), nor have there been any major breakthroughs in treatment for the disease in the past 20 years (6).
Researchers have gradually come to recognize the key role of immunoregulation in cancer (7). Cancer immunotherapy has always been the main driving force of personalized medicine. Studies have shown that maintaining the balance between timely and effective immunity and a lasting immune response is essential for immune defense (8). This delicate balance is maintained through internal or external immune regulatory mechanisms. Internal control of immune cell activation is mediated through stimulation of effector cells and related inhibitory receptors, whereas external control is mediated through the secretion of cells or inhibitors. Tumors can exacerbate these immunosuppressive pathways, creating a tolerable microenvironment. The past few years have seen the emergence of immune checkpoint inhibitors (ICIs) which act by blocking the programmed cell death receptor 1 (PD-1) pathway. These ICIs can improve the OS of patients with metastatic renal cell carcinoma (mRCC) and metastatic urothelial carcinoma (9). Evidence suggests that immune regulatory mechanisms carry importance in tumorigenesis and cancer development. Along with the research related to tumor immunology, the characteristics of tumor microenvironment (TME) in ccRCC have been identified by researchers, which consists of cancer cells, endothelial cells, myofibroblasts, fibroblasts, tumor-infiltrating immune cells, and extracellular matrix (10). The characteristics of the composition of TILs in TME affect the effectiveness of tumor therapy. Among them, anti-tumor immunity is characterized by M1 macrophages and CD8+ T cells, while immune evasion is characterized by T-cell regulation (Tregs), T-cell regulation (Tregs) and M2-macrophages. Macrophages in tumor tissues are biased towards the M2 subtype (11). Owing to the development of high-throughput technologies, such as gene sequencing, researchers can quickly process large datasets to identify and analyze specific tumor markers (12).
The value of immune-related genes (IRGs) in the development of personalized immune assessment models to improve the prognosis of patients with non-small cell lung cancer ccRCC and thyroid cancer has already been described (13,14). However, the use of immune models to assess immune levels and sensitivity to immunotherapy in ccRCC patients and to predict lymph node and distant metastases has not been reported. Therefore, the main objective of this study was to investigate the potential clinical efficacy of IRGs in the prognostic and metastatic classification of ccRCC patients and the significance of IRGs as an indicator for assessing the therapeutic sensitivity of ICI therapy. We present the following article in accordance with the TRIPOD reporting checklist (available at https://dx.doi.org/10.21037/atm-21-4973).
Methods
Extraction of gene samples and clinical data
Figure 1 shows the experimental flowchart. First, a dataset containing transcriptome RNA sequencing data from 611 tissue samples including 539 primary ccRCC and 72 non-tumor tissue samples was downloaded from The Cancer Genome Atlas (TCGA). After standardization, the clinical data of ccRCC patients in the dataset were extracted and analyzed and cases which lacked complete data were deleted. ImmPort is a real-time immunology database containing a large number of IRGs that can be analyzed and studied. A list of IRGs was extracted from the ImmPort database for screening of target genes in ccRCC.The TIMER database provides 10,897 samples from 32 cancers and provides a wealth of data on tumor-infiltrating immune cells (TIICs), including CD4+T cells, B cells, CD8+ T cells, macrophages, dendritic cells, and neutrophils, and the immune cell infiltration in 539 primary ccRCC sample was estimated by the algorithm provided by this database. All procedures performed in this study involving human participants were in accordance with the Declaration of Helsinki (as revised in 2013). The study was approved by the ethical standards of Shanghai Tenth People’s Hospital (No. SHSY-IEC-4.1/19-120/01).
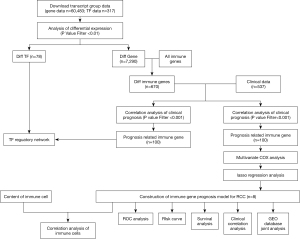
Screening of differential genes
Differentially expressed IRGs (DEIRGS) between ccRCC and non-tumor tissue samples were screened out using the edgeR package in R (http://bioconductor.org/package/EDGER/). After standardization of the original data, differential analysis was carried out based on error detection rate [false discovery rate (FDR) <0.05 and log2|fold change| >1], and DEIRGs were extracted. Subsequently, Gene Ontology (GO) and Kyoto Encyclopedia of Genes and Genomes (KEGG) analyses were carried out to explore the potential molecular pathways and physiological processes of the DEIRGs.
Establishment of a prognostic IRG signature
The DEIRGs were subjected to univariate Cox regression analysis using the survival package in R to obtain those key DEIRGS related to prognosis. The core prognostic DEIRGs were identified with the screening criterion set to P<0.01. After that, the Cox regression model with least absolute shrinkage and selection operator (LASSO) was used to reduce noise and redundant genes. The optimal values of the penalty parameter λ were determined through 10 cross-validations, and the risk model was constructed based on the results. The following signature formula was used: Risk score = ε1×expLncRNA1 + ε2×expLncRNA2 + … εn×expLncRNAn.
In this signature, ε represents the regression coefficient of LASSO Cox regression, and expLncRNA represents the expression levels of the core prognostic DEIRGs.
Immune-related gene sets were downloaded from http://software.broadinstitute.org/gsea/downloads and used in Gene Set Enrichment Analysis (GSEA) 3.0 [normalized enrichment score (NES) >1.5 and FDR <0.05].
Survival analysis
Death was used as the endpoint in this study. Clinical data were obtained from the TCGA database. The log2 (normalized value +1) data format was used for survival analysis. Prognosis-related IRGs (PRDEIRG) were screened out by univariate Cox analysis using the survival package in R.
Construction of the potential regulatory networks of PRDEIRGs and transcription factors (TFs)
To further explore the clinical value of the screened PRDEIRGs, our next focus was to explore the regulatory network of the PRDEIRGs and their upstream TFs. The Cistrome Cancer database is a reliable data source that integrates cancer genome data from TCGA with more than 23,000 ChIP-Seq and chromatin data. We download 317 TFs from Cistrome Cancer database and screened out those which were differentially expressed between ccRCC and non-tumor tissues. The correlations between the differentially expressed TFs and the previously screened PRDEIRGs were analyzed (the screening criteria were correlation score >|0.4| and composite score >|0.6|) and the resulting TF regulatory network was visualized using Cytoscape software (version 3.6.1).
Further analysis of the clinical significance of the IRG prognostic model
The prognosis-related IRGs were firstly screened by Unicox analysis. After the elimination of overfitting by lasso regression analysis, 8 prognosis-related IRGs were obtained. A prognostic model of IRGs was established based on the lasso coefficient. According to the median risk score of all the clinical samples from TCGA, patients were divided into high-risk and low-risk groups. Subsequently, the prognostic value of model was further evaluated using the two groups of samples.
Cell culture
The human normal kidney epithelial cell line HK-2 and ccRCC cell lines (786-O, Caki-2, and 89C-A1) were supplied by the Cell Bank of the Chinese Academy of Sciences. The human ccRCC cell lines were cultured in RPMI-1640 (Gibco; Thermo Fisher Scientific, Inc.) containing 10% fetal bovine serum (Gibco; Thermo Fisher Scientific, Inc.) and 1% penicillin/streptomycin. The HK-2 cell line was cultured in Dulbecco’s Modified Eagle Medium (DMEM; ScienCell Research Laboratory) containing 10% fetal bovine serum (Gibco; Thermo Fisher Scientific, Inc.). All cells were cultured at 37 °C and 5% CO2.
Quantitative reverse transcription PCR
TRIzol® reagent (Thermo Fisher Scientific, Inc.) was used to isolate total cellular RNA in line with the manufacturer’s instructions. SYBR® Green PCR Master Mix (Thermo Fisher Scientific, Inc.) and the 7900HT Fast Real-Time PCR System were used to perform quantitative PCR (qPCR). The cycling conditions were as follows: 95 °C for 10 minutes, 95 °C for 10 minutes, followed by 40 cycles at 95 °C for 10 seconds and 60 °C for 1 minute. Relative mRNA levels were normalized using GAPDH as a control. The 2−ΔΔCq method was applied for analysis of the results.
Nomogram development and validation
Univariate and multivariate Cox regression analyses were performed to predict the impact of the IRGPS and other clinicopathological features on prognosis and metastasis in patients with ccRCC. Subsequently, Nomograms were created to predict prognosis and metastasis, and these Nomograms were validated by consistency indices (C-index) and calibration plots using the rms package v5.1 in R (https://cran.r-project.org/web/packages/rms/index.html).
Statistical analysis
The prognostic performance of the IRG signature was evaluated through comparison of the sensitivity and specificity of receiver operating characteristic (ROC) curves. The area under the ROC curve of the risk score was calculated using the survival ROC package in R, and the area of the risks core was further compared with those of other clinical features related to prognosis, including stage, age, and T stage, to verify the reliability of the risk score as a prognostic factor. Principal component analysis (PCA) was performed to profile expression patterns of grouped samples. Differences between clinical parameters were tested by independent t tests. A P value of less than 0.05 was considered indicative of statistical significance. The Wilcoxon test was used to compare differences in the relative number of TIICs and the expression levels of DEIRGs between the low-risk score and high-risk score groups.
Results
Identification of DEIRGs
The edgeR algorithm was used to extract 7,290 differentially expressed genes including 5,454 which were upregulated and 1,836 which were downregulated (Figure 2A,2B). After screening the IRGs, we obtained 670 DEIRGs including 554 upregulated and 116 downregulated genes (Figure 2C,2D). Subsequently, the DEGs were used for gene enrichment analysis. GO analysis revealed that “signal transduction”, “plasma membrane”, and “cytokine activity” were the most common biological process, cellular component, and molecular function terms, respectively (Figure 3A). KEGG analysis shows that the DEGS were most commonly enriched in cytokine-cytokine receptor interaction (Figure 3B). At the same time, the DEIRGs were used for gene enrichment analysis. GO analysis revealed “immune response”, “plasma membrane”, and “antigen-binding” to be the most common biological process, cellular component, and molecular function terms, respectively (Figure 3C). KEGG analysis shows that the DEIRGs were most commonly enriched in cytokine-cytokine receptor interaction (Figure 3D).
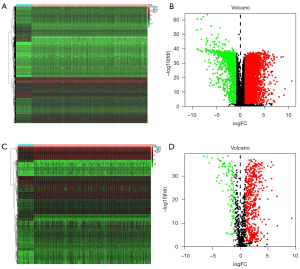
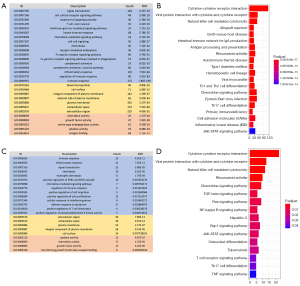
Identification of prognosis-associated DEIRGs
By combining clinical data, we screened out DEIRGs that were significantly correlated with clinical outcomes (P<0.05) and then obtained 100 prognosis-related DEIRGS (PRDEIRGs). As shown in the hazard ratio forest plot in Figure 4, most of the PRDEIRGs in the ccRCC samples were upregulated which act as promoters of cancer development (Figure 4).
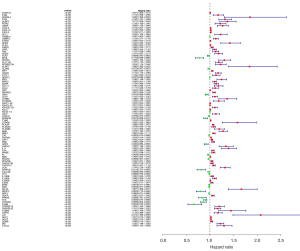
Construction of a TF and IRG regulatory network
To investigate the molecular mechanisms of the IRGs, we investigated the relationships between the IRGs and their upstream TFs. We identified 60 differentially expressed TFs between the normal and tumor tissues, and subsequently, the correlation between these TFs and disease prognosis and IRG was analyzed (Figure 5A,5B). Finally, a complete adjustment network between the selected TFs and IRGs was established (Figure 5C).
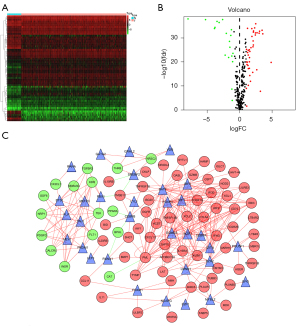
Construction of the IRG prognostic model
After ruling out the possibility of overfitting the selected genes, we established an IRG prognostic signature (IRGPS) for ccRCC based on the LASSO coefficient (Table 1, Figure 6A,6B). The following formula was used for the model: {(LTB4Rexpression level × 0.00162610575685915) + (AGERexpression level × 0.00687887880688249) + (PLAURexpression level × 0.0127930955874741) + (BMPlexpression level × 0.00197358886843568) + (MDKexpression level × 0.000382733494608676) + [PDGFDexpression level × (−0.000701610101642847)]} + (UCNexpression level × 0.0549516438186964) + (BIDexpression level × 0.0408935254267024). A total of 491 patients were included in the model and were divided into two groups: the high risk score (n=245) and low risk score (n=246) groups (Table 2). Survival analysis of the two groups suggested that the risk score could be an important tool for differentiating the OS of patients with ccRCC (Figure 6C). Additionally, we incorporated age, T stage, and other prognostic factors into the ROC analysis. The results showed that the reliability of the risk score as a predictor of survival was similar to or better than that of prognostic factors such as age or T-stage, which have previously been shown to be meaningful (Figure 6D).
Table 1
Gene | Coef |
---|---|
LTB4R | 0.001626106 |
AGER | 0.006878879 |
PLAUR | 0.012793096 |
BMP1 | 0.001973589 |
MDK | 0.000382733 |
PDGFD | -0.00070161 |
UCN | 0.054951644 |
BID | 0.040893525 |
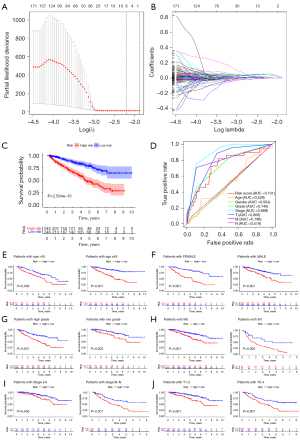
Table 2
ID | Futime | Fustat | LTB4R | AGER | PLAUR | BMP1 | MDK | PDGFD | UCN | BID | Risk Score | Risk |
---|---|---|---|---|---|---|---|---|---|---|---|---|
TCGA-BP-4987 | 3.079452055 | 0 | 5.841982 | 3.79131 | 1.370556 | 6.816226 | 5.958857 | 37.80334 | 1.219929 | 5.433927 | 1.393157438 | Low |
TCGA-B0-4839 | 4.490410959 | 1 | 2.115604 | 0.7539008 | 2.573832 | 4.863871 | 25.32093 | 29.26457 | 1.091857 | 6.729957 | 1.455751997 | Low |
TCGA-CW-6097 | 1.564383562 | 1 | 0.7891749 | 0.9286199 | 6.054266 | 10.75601 | 41.93875 | 11.71599 | 0.2799337 | 4.575919 | 1.372579032 | Low |
TCGA-BP-4163 | 7.778082192 | 0 | 1.712064 | 3.904903 | 2.943969 | 5.359348 | 76.89066 | 17.05063 | 0.8208398 | 5.614195 | 1.447794606 | Low |
TCGA-B0-5690 | 6.597260274 | 0 | 2.837976 | 2.46101 | 5.429501 | 9.164681 | 7.186415 | 74.36796 | 0.9442045 | 7.360424 | 1.510655589 | High |
TCGA-B0-5094 | 0.912328767 | 1 | 5.343834 | 4.873171 | 2.633175 | 10.09645 | 9.211124 | 7.052344 | 2.772821 | 6.239584 | 1.651908625 | High |
TCGA-DV-5575 | 2.756164384 | 0 | 2.250361 | 0.9014864 | 4.08454 | 5.645169 | 22.84387 | 30.83229 | 0.3964205 | 5.42895 | 1.355485137 | Low |
TCGA-B0-4828 | 0.84109589 | 1 | 5.074542 | 8.215924 | 1.797358 | 12.57838 | 11.39704 | 9.600267 | 3.905391 | 4.627119 | 1.67203201 | High |
TCGA-BP-4164 | 2.717808219 | 1 | 2.382375 | 3.651075 | 2.29374 | 3.194051 | 15.06219 | 33.45911 | 1.725434 | 3.062005 | 1.305985912 | Low |
Subsequently, we performed survival analysis after grouping the patients with ccRCC by different clinical characteristics (Figure 6E-6J). The results showed that the IRGPS had significant ability to stratify the prognosis of patients with ccRCC in different subgroups (P<0.01).
Clinical significance of the IRG model
Correlation analysis of the IRGPS risk score and clinical characteristics revealed that risk score was strongly correlated with T stage, M stage, N stage, sex, and stage (Figure 7A-7F). The results revealed that the IRGPS risk score was closely associated with the malignant progression of ccRCC and that the probability of a high risk score was greater in men than in women. The GSEA results of IRGPS showed that the set of genes related to immune system processes and immune responses were mainly enriched in the high-risk subgroup (Figure 7G,7H). Subsequent GO and KEGG analysis results suggested that the enrichment results in the high-risk subgroup were mainly involved in the regulation of the immune system (including positive regulation of activated T cell proliferation, B cell-mediated immune regulation, regulation of cd4-positive αβ cell activation, regulation of interleukin-1β production and regulation of T cell migration), renal cell carcinoma, IgA production in the intestinal immune network, primary immunodeficiency, auto immune thyroid disease and the p53 signaling pathway. (Figure 7I,7J).
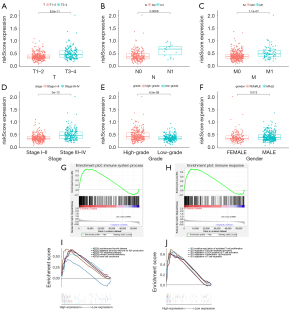
Validation of IRGPS for correlation with the immune system
The bar graph in Figure 8A shows the proportion of TIICs in the 539 ccRCC samples. The box plot in Figure 8B shows the level of immune cell infiltration in the high and low risk score subgroups. The results show that activated dendritic cells, CD8+ T cells, macrophages, mast cells, helper T cells, T follicular helper cells, tumor-infiltrating lymphocytes (TIL), and Tregs differed significantly between the two groups (P<0.05). The box plot in Figure 8C indicates that there were significant differences in the activation of tumor-related immune responses, such as, Antigen-presenting cells co-stimulation, activation of immune checkpoint, cytolytic activity, activation of human leukocyte antigen, Inflammation-promoting, parainflammation, T cell co-stimulation, T cell co-inhibition, and type I and type 2 interferon response, between the high-risk and low-risk groups (P<0.001). The TIDE algorithm was applied to validate the effect of the IRGPS on immunotherapeutic response in the TCGA-KIRC cohort. The ICI response results for each patient are shown in https://cdn.amegroups.cn/static/public/atm-21-4973-1.xls. The results show that IRGPS-low risk group had a significantly lower ICI response rate than the IRGPS-high-risk group (χ2 test, P<0.001; Figure 8D).
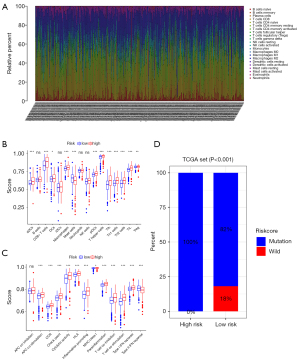
Verification of multiple databases
To further validate the reliability of the prognostic model, the genetic and clinical information of 91 patients with ccRCC from the GEO database was assessed using the same model and divided into two groups (Table 3). Survival analysis revealed a significant difference in survival time between the two groups (Figure 9A,9B). We next used the Oncomine database to search and analyze the expression of LTB4R, AGER, PLAUR, BMP1, MDK, PDGFD, UCN and BID and performed an χ2 test on the results of the three completed experimental studies. The results confirm that these genes were differentially expressed between the ccRCC tissues and normal tissues (P<0.001) (Figure 9C-9J).
Table 3
ID | Futime | Fustat | AGER | LTB4R | PLAUR | BMP1 | MDK | PDGFD | UCN | BID | RiskScore | Risk |
---|---|---|---|---|---|---|---|---|---|---|---|---|
DO47213 | 5.021917808 | 1 | 0.649058088 | 1.863723939 | 2.414612343 | 6.062902773 | 2.112220464 | 43.10360379 | 0.290694048 | 3.574457713 | 0.183047336 | Low |
DO47228 | 2.767123288 | 2 | 1.521765302 | 1.91660072 | 1.790784183 | 6.021359008 | 23.46798232 | 28.51212062 | 0.342735473 | 4.329498419 | 0.233225985 | Low |
DO46834 | 5.419178082 | 2 | 0.58407147 | 0.511539719 | 2.381064809 | 1.790919659 | 5.228666698 | 8.129596418 | 0.942487803 | 4.15915485 | 0.257012989 | Low |
DO47192 | 3.81369863 | 2 | 6.488441348 | 3.302337099 | 2.502805021 | 3.234641949 | 8.466375538 | 0.038682203 | 2.467778576 | 0.913033074 | 0.26456422 | Low |
DO46945 | 5.265753425 | 1 | 1.659009977 | 2.074482528 | 3.113904274 | 4.323923825 | 7.363930535 | 53.41095315 | 1.246109522 | 4.250659186 | 0.270779395 | Low |
DO47076 | 5.112328767 | 1 | 2.59220804 | 3.457047503 | 2.241605466 | 5.776804094 | 2.587257299 | 36.62719949 | 0.869178263 | 4.815966643 | 0.283513546 | Low |
DO46909 | 5.42739726 | 1 | 1.192748765 | 1.312247972 | 2.022179251 | 1.982656121 | 6.409782165 | 41.18857075 | 0.38951874 | 6.098022552 | 0.284434368 | Low |
DO46844 | 5.745205479 | 1 | 1.491945645 | 1.621494361 | 2.690756716 | 2.456977542 | 7.717768117 | 52.16131061 | 0.297543915 | 6.307217114 | 0.292782915 | Low |
DO46988 | 4.594520548 | 2 | 3.275029256 | 5.151889607 | 1.842292656 | 5.573109499 | 1.492700409 | 45.69700874 | 1.392091776 | 4.48457703 | 0.293853487 | Low |
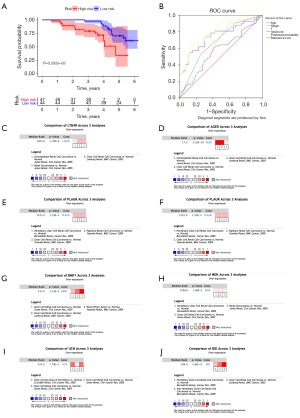
Validation of the expression of prognostic tumor microenvironment–related genes in vitro
Quantitative reverse transcription PCR was used to investigate the expression levels of the eight immune-related prognostic genes in three ccRCC cell lines (786-O, Caki-2, and 89C-A1) and one normal kidney epithelial cell line (HK-2). The results showed that LTB4R, AGER, PLAUR, BMP1, PDGFD, and UCN were significantly upregulated in ccRCC cells (Figure 10).
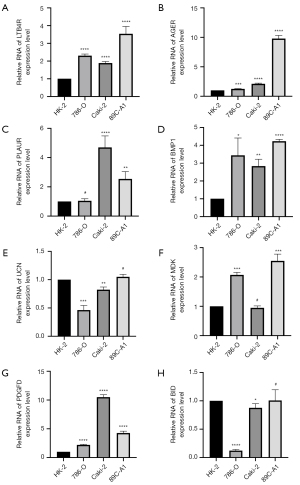
Establishment and validation of Nomograms to predict survival based on the IRGPS
In the analysis of the 539 ccRCC samples in the TCGA-KIRC cohort, clinical characteristics were screened by univariate and multifactorial Cox regression, and Nomograms to predict OS at 1, 3, and 5 years were created based on the IRGPS risk score, age, class, and stage (Figure 11A,11B). The Nomogram had a C index of 0.79±0.18 (mean ± standard error), which indicated that the actual OS of the sample was generally consistent with the predicted OS (Figure 11C). Finally, the calibration plots showed the Nomogram to have good predictive utility in the prediction of ccRCC prognosis (Figure 11D,11E).
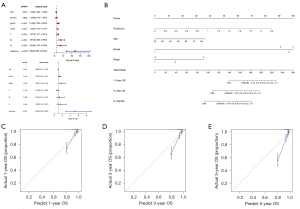
Establishment and validation of Nomograms to predict metastasis based on the IRGPS
In the analysis of the 539 ccRCC samples in the TCGA-KIRC cohort, clinical characteristics were screened by univariate and multifactorial Cox regression, and Nomograms to predict distant metastasis were created based on the IRGPS risk score, grade, and T stage (Figure 12A,12B). The C-index of the Nomograms was 0.865±0.03 (mean ± standard error), which indicated that the actual rate of distant metastasis within the sample was generally consistent with the predicted results. The calibration plots also showed consistency between the Nomogram-predicted and actual distant metastasis (Figure 12C) Similarly, after screening, the IRGPS risk score, grade, T stage, and stage were used to construct Nomograms to predict lymph node metastasis (Figure 12D,12E). The C-index of the Nomograms was 0.96±0.02 (mean ± standard error), which indicated the validity of this Nomograms. The calibration plots showed the lymph node metastasis-related Nomograms to have good predictive power (Figure 12F).
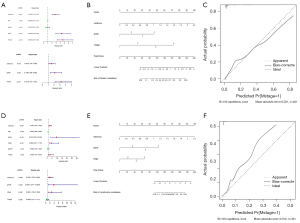
Discussion
RCC is one of the most common tumors of the urinary tract and is insensitive to radiation and chemotherapy. ccRCC is the most common subtype of RCC (70–80%). A large number of evidence demonstrates that alterations in gene and protein levels are closely related to the development of ccRCC and patient prognosis. Zhang et al. (15) demonstrated that p-CREB1 protein levels were significantly higher in tumor tissues than in adjacent normal tissues and increased progressively from normal to tumor fractions and was an independent prognostic biomarker for ccRCC patients. Heyliger et al. (16) found that KRAB-ZNF protein expression levels were significantly downregulated in clear cell carcinoma by analyzing the data obtained. In addition, ZNF433 had different expression levels at different stages and grades of ccRCC and correlated with tumor metastasis and patient prognosis; Su et al. (17) found that YTHDF2 expression was significantly correlated with the abundance of immune cells and affected patients’ OS and DFS. Currently, molecularly targeted drugs (tyrosine kinase inhibitors; e.g., pazopanib, sunitinib) are the most preferred treatment option for patients with advanced RCC, but a highly adaptive, active, and heterogeneous TME can have an impact on treatment efficacy and even drug resistance situation (18). TME consists of tumor cell components and surrounding non-tumor components (e.g., vascular endothelial cells, mesenchymal stem cells, adipocytes, fibroblasts, cytokines, lymphocytes, macrophages, etc.) that provide the necessary structure, environment, and barriers for tumor cell development and progression (19). Also, TME contains many biochemical components (e.g., cytokines, growth factors, etc.) These components together constitute a highly complex and dynamic heterogeneous network that is important for tumor growth and invasion (20). A large number of studies have now shown that immunosuppression of TME and low immunogenicity of tumor cells are negatively correlated with the efficacy of antitumor drugs and may promote the occurrence of immune escape of tumor cells (21). Currently, IRG is considered to be closely related to the tumor microenvironment and tumor development. Although significant progress has been made in the study of IRGs in tumorigenesis, progression, and immunotherapy, systematic analysis and molecular mechanism studies of the prognostic impact of IRGs on ccRCC are still lacking. In this study, we performed a comprehensive analysis of IRGs in ccRCC to better understand their prognostic significance and molecular characteristics. We obtained a large number of clinical samples from TGCA, which ensured the accuracy of the results. From these ccRCC samples, we screened eight IRGs that were significantly associated with the onset and progression of ccRCC by bioinformatics. by further analysis of the findings, we found that these IRGs could serve as potential clinical biomarkers for ccRCC and guide personalized treatment.
Studies have shown that changes of IRGs such as platelet-derived growth factor D (PDGF-D) may enhance invasion and metastasis in several human malignancies. For instance, studies have indicated that overexpressing PDGF-D in SN12- cells promoted the growth, angiogenesis, and metastasis of human renal cell carcinoma in an orthotopic severe combined immunodeficient (SCID) mouse model. Xu et al. reported that PDGF-D overproduction in SN12-C cells increased the proliferation and migration of mural cells in vitro and improved perivascular cell coverage in vivo (22). The present study sought to investigate the relationships between IRGs and the tumor microenvironment, and their clinical significance. Functional enrichment analysis of the screened IRGs showed that these genes were mainly involved in immune response. The advent of ICI immunotherapy has led to significant changes in the treatment landscape for several solid malignancies. In particular, drugs targeting the programmed death 1 (PD-1) and cytotoxic T-lymphocyte-associated antigen (CTLA-4) pathways can achieve considerable clinical efficacy (23). This further confirms that the IRGs we identified are meaningful.
To further elucidate the regulatory mechanisms of the selected genes, we constructed a TF-IRG regulatory network to explore potentially clinically valuable molecular mechanisms. In the future, this network can guide further verification and analysis of upstream and downstream TFs and specific regulatory mechanisms of target IRGs. A regulatory relationship between MYBL2 and MDK, which are closely related to growth factors, has been suggested; these types of relationships should guide future research (24).
An IRGPS including eight prognostic IRGs that were differentially expressed between ccRCC and normal tissues was established through a combination of Cox regression analysis, LASSO Cox regression analysis, and survival analysis. The IRGPS showed a significant ability to stratify the OS of patients with ccRCC, and the prognosis of the low-risk group was significantly better than that of the high-risk group. These results suggest that the IRGPS can effectively predict the prognosis of patients with ccRCC. By studying genome-wide DNA methylation, Wang et al. constructed a model using six methylation-driven genes to predict OS in bladder cancer (25). Chen et al. explored prognostic TME-related genes and identify key regulators for TME of ccRCC (26). Many other studies have focused on the effects of single genes or proteins on the prognosis of patients with ccRCC (27). In contrast to previous research directions, the present study focused on eight IRGs that were significantly associated with the prognosis of ccRCC. The IRGPS can be used not only as a prognostic indicator, but also to evaluate the immune status of patients and provide personalized treatment plans. Also, the results of the correlation analysis based on patient clinical characteristics suggested that the IRGPS was closely related to the malignant progression of ccRCC, which also provided direction for the next step of constructing a metastasis-related Nomogram. Follow-up gene function and pathway enrichment analyses pf the IRGPS showed that dysregulation of the expression of these eight prognostic IRGs was closely associated with immune system disorders. Functional and pathway enrichment analyses of the high risk score subgroup suggested that the functions of these genes mainly included the regulation of immune cells involved in specific immunity. The main pathways included the renal cancer, P53 signaling, and immune disease pathways. Importantly, p53, a well-studied oncogene, is mutated or missing in almost half of the cancers, which provides a direction for subsequent exploration of the molecular mechanisms underlying the occurrence of ccRCC and the search for corresponding therapeutic targets (28).
The activation of immune system and TIIC infiltration play an important role in the development of immunotherapeutic strategies (29,30). By assessing the status of TIICS and the immune system in ccRCC samples, we found that IRGPS risk score was correlated with multiple TIICs, being positively correlated with macrophages, CD8+ T cells, T follicular helper cells, and Tregs and negatively correlated with mast cells. Sheng et al. found that samples from patients with ccRCC could be divided into two subgroups: a subgroup with a better prognosis characterized by a higher proportion of mast cell infiltration and a group with a worse prognosis characterized by severe immune infiltration and the presence of immunosuppression (21). The present study provides a side-by-side explanation of why the IRGPS high-risk group has a poorer prognosis than the low-risk group. Furthermore, the IRGPS was found to be significantly positively correlated with the activation of the immune system, representing a more active immune microenvironment in patients in the IRPGS high-risk group. This active change may stem from enhanced tumor immune escape, which in turn elevates the activity of the immune system through negative feedback regulation. Subsequent results of immunotherapy responsiveness evaluation suggested that the high-risk group had higher immune activity and responded more effectively to immunotherapy than the low-risk group. Therefore, the IRGPS has the potential to be an important basis for developing individualized immunotherapy plans for patients with ccRCC; however, the mechanisms involved need to be further explored. It is now commonly believed that IRG regulates TME and affects immune cell differentiation and ccRCC progression. The mechanism of the role of TME in tumor treatment and tumor escape is still under investigation. Although immunotherapy has become an important component of tumor treatment, the impact of TME on immunotherapy and related mechanisms still need to be further explored. With the development of targeted therapeutic agents targeting various TME components or immune genes, strategies combining them with TKI or ICI will likely be the future direction of cancer therapy.
The prognostic significance of the IRGPS was further validated in a sample of 91 ccRCC cases from the GEO database. The results of cellular assays and Oncomine database analysis further demonstrated that the eight IRGs were differentially highly expressed in ccRCC.
To further explore the clinical role of IRGPS, we combined IRGPS with other clinical factors to create Nomograms to predict prognosis and metastasis in ccRCC patients. Subsequent validation results showed that the Nomogram was effective in predicting prognosis and metastasis of ccRCC.
Of course, the current research has limitations. First, the immune system is a dynamic and complex system, and simple transcriptome analysis only reflects certain aspects of an individual's immune status and is not comprehensive. Second, the molecular regulatory mechanisms of the IRGs has not been further confirmed in vivo. Third, the prognosis of ccRCC is affected by many factors. The prognostic indicators used in this study did not exclude the effects of other factors such as metabolism and infection, and we only addressed the role of the immune system. Actually there are similarities between this study and the previous publication by Gao et al. (31). We all focused on exploring the effect of IRGs on ccRCC, but this study still has many differences and innovations compared to the latter. Firstly, the present study established a more demanding filtering condition. Second, the eight-gene based signature created in this study adopts more variables than the latter, is relatively more stable, and performs better in its ability to predict prognosis (cf. AUC). Third, we explored the signature's relevance to the immune system more comprehensively than the latter, adding an inquiry about the degree of activation of the immune system and sensitivity to ICI treatment. Fourth, compared to the latter, we added cellular level studies, verifying that there were significant differences in transcript levels of target genes in the three tumor cell lines and normal epithelial cells. Most importantly, this study combined with other common ccRCC clinical traits to produce a Nomogram applied to the prediction of tumor prognosis and metastasis, an approach that enables better clinical application of IRGPS.
The IRG prognostic model constructed in this study provides a tool for assessing the immune status of patients, developing individualized treatment plans, and predicting prognosis and metastasis in ccRCC. However, further research is necessary. For example, study of the specific relationships between IRGs, downstream proteins, and the metabolic processes they control will allow the dynamic and complete tumor immune response system to be described.
Conclusions
In conclusion, our study explored the malignant progression of ccRCC and its associated mechanisms from an immunological perspective. Our IRGPS based on eight OS-related immune genes was found to be strongly associated with the prognosis and metastasis of ccRCC, and could assess the immune status of patients with ccRCC and predict their sensitivity to immunotherapy. Biofunctional and pathway analyses provided direction for further mechanistic analysis. The Nomogram built based on the IRGPS is a useful tool for predicting prognosis, distant metastasis, and lymph node metastasis in patients with ccRCC.
Acknowledgments
We would like to thank Peng Zhong, Jiaxin Zhang, and Cheng Li for their contributions to the preliminary planning of this research.
Funding: This work was supported in part by a grant from the Shanghai Municipal Health Commission Fund (202040179); Shanghai Science Committee Foundation #19411967700; National Natural Science Genetic Committee, Youth Project, 8160246; Shanghai Science and Technology Commission, Pujiang Talent Program, 20PJ1412400; Shanghai Science and Technology Commission, Shanghai Natural Science Foundation, Surface Project, 20ZR1443000.
Footnote
Reporting Checklist: The authors have completed the TRIPOD reporting checklist. Available at https://dx.doi.org/10.21037/atm-21-4973
Conflicts of Interest: All authors have completed the ICMJE uniform disclosure form (available at https://dx.doi.org/10.21037/atm-21-4973). The authors have no conflicts of interest to declare.
Ethical Statement: The authors are accountable for all aspects of the work in ensuring that questions related to the accuracy or integrity of any part of the work are appropriately investigated and resolved. All procedures performed in this study involving human participants were in accordance with the Declaration of Helsinki (as revised in 2013). The study was approved by the ethical standards of Shanghai Tenth People’s Hospital (No. SHSY-IEC-4.1/19-120/01).
Open Access Statement: This is an Open Access article distributed in accordance with the Creative Commons Attribution-NonCommercial-NoDerivs 4.0 International License (CC BY-NC-ND 4.0), which permits the non-commercial replication and distribution of the article with the strict proviso that no changes or edits are made and the original work is properly cited (including links to both the formal publication through the relevant DOI and the license). See: https://creativecommons.org/licenses/by-nc-nd/4.0/.
References
- Ismailov Z, Rasa A, Bandere K, et al. A Case of Stage IV Chromophobe Renal Cell Carcinoma Treated with the Oncolytic ECHO-7 Virus, Rigvir®. Am J Case Rep 2019;20:48-52. [Crossref] [PubMed]
- Ferlay J, Steliarova-Foucher E, Lortet-Tieulent J, et al. Cancer incidence and mortality patterns in Europe: estimates for 40 countries in 2012. Eur J Cancer 2013;49:1374-403. [Crossref] [PubMed]
- Bray F, Ren JS, Masuyer E, et al. Global estimates of cancer prevalence for 27 sites in the adult population in 2008. Int J Cancer 2013;132:1133-45. [Crossref] [PubMed]
- Zhu J, Armstrong AJ, Friedlander TW, et al. Biomarkers of immunotherapy in urothelial and renal cell carcinoma: PD-L1, tumor mutational burden, and beyond. J Immunother Cancer 2018;6:4. [Crossref] [PubMed]
- Zhang T, Zhu J, George DJ, et al. Metastatic clear cell renal cell carcinoma: Circulating biomarkers to guide antiangiogenic and immune therapies. Urol Oncol 2016;34:510-8. [Crossref] [PubMed]
- Wiechno P, Kucharz J, Sadowska M, et al. Contemporary treatment of metastatic renal cell carcinoma. Med Oncol 2018;35:156. [Crossref] [PubMed]
- Lalani AA, McGregor BA, Albiges L, et al. Systemic Treatment of Metastatic Clear Cell Renal Cell Carcinoma in 2018: Current Paradigms, Use of Immunotherapy, and Future Directions. Eur Urol 2019;75:100-10. [Crossref] [PubMed]
- Oldham KA, Parsonage G, Bhatt RI, et al. T lymphocyte recruitment into renal cell carcinoma tissue: a role for chemokine receptors CXCR3, CXCR6, CCR5, and CCR6. Eur Urol 2012;61:385-94. [Crossref] [PubMed]
- Lavoie JM, Bidnur S, Black PC, et al. Expanding Immunotherapy Options for Bladder Cancer: Commentary on: Pembrolizumab as Second-Line Therapy for Advanced Urothelial Carcinoma. Urology 2017;106:1-2. [Crossref] [PubMed]
- Hui L, Chen Y. Tumor microenvironment: Sanctuary of the devil. Cancer Lett 2015;368:7-13. [Crossref] [PubMed]
- Cervantes-Villagrana RD, Albores-García D, Cervantes-Villagrana AR, et al. Tumor-induced neurogenesis and immune evasion as targets of innovative anti-cancer therapies. Signal Transduct Target Ther 2020;5:99. [Crossref] [PubMed]
- Li S, Xu W. Mining TCGA database for screening and identification of hub genes in kidney renal clear cell carcinoma microenvironment. J Cell Biochem 2019; Epub ahead of print. [Crossref] [PubMed]
- Lin P, Guo YN, Shi L, et al. Development of a prognostic index based on an immunogenomic landscape analysis of papillary thyroid cancer. Aging (Albany NY) 2019;11:480-500. [Crossref] [PubMed]
- Wan B, Liu B, Huang Y, et al. Prognostic value of immune-related genes in clear cell renal cell carcinoma. Aging (Albany NY) 2019;11:11474-89. [Crossref] [PubMed]
- Zhang Z, Guan B, Li Y, et al. Increased phosphorylated CREB1 protein correlates with poor prognosis in clear cell renal cell carcinoma. Transl Androl Urol 2021;10:3348-57. [Crossref] [PubMed]
- Heyliger SO, Soliman KFA, Saulsbury MD, et al. The Identification of Zinc-Finger Protein 433 as a Possible Prognostic Biomarker for Clear-Cell Renal Cell Carcinoma. Biomolecules 2021;11:1193. [Crossref] [PubMed]
- Su G, Liu T, Han X, et al. YTHDF2 is a Potential Biomarker and Associated with Immune Infiltration in Kidney Renal Clear Cell Carcinoma. Front Pharmacol 2021;12:709548 [Crossref] [PubMed]
- Heidegger I, Pircher A, Pichler R. Targeting the Tumor Microenvironment in Renal Cell Cancer Biology and Therapy. Front Oncol 2019;9:490. [Crossref] [PubMed]
- Kou Y, Ji L, Wang H, et al. Connexin 43 upregulation by dioscin inhibits melanoma progression via suppressing malignancy and inducing M1 polarization. Int J Cancer 2017;141:1690-703. [Crossref] [PubMed]
- Giraldo NA, Sanchez-Salas R, Peske JD, et al. The clinical role of the TME in solid cancer. Br J Cancer 2019;120:45-53. [Crossref] [PubMed]
- Sheng X, Parmentier JH, Tucci J, et al. Adipocytes Sequester and Metabolize the Chemotherapeutic Daunorubicin. Mol Cancer Res 2017;15:1704-13. [Crossref] [PubMed]
- Xu L, Tong R, Cochran DM, et al. Blocking platelet-derived growth factor-D/platelet-derived growth factor receptor beta signaling inhibits human renal cell carcinoma progression in an orthotopic mouse model. Cancer Res 2005;65:5711-9. [Crossref] [PubMed]
- Atkins MB, Clark JI, Quinn DI. Immune checkpoint inhibitors in advanced renal cell carcinoma: experience to date and future directions. Ann Oncol 2017;28:1484-94. [Crossref] [PubMed]
- Han S, Shin H, Lee JK, et al. Secretome analysis of patient-derived GBM tumor spheres identifies midkine as a potent therapeutic target. Exp Mol Med 2019;51:1-11. [Crossref] [PubMed]
- Wang L, Shi J, Huang Y, et al. A six-gene prognostic model predicts overall survival in bladder cancer patients. Cancer Cell Int 2019;19:229. [Crossref] [PubMed]
- Chen B, Chen W, Jin J, et al. Data Mining of Prognostic Microenvironment-Related Genes in Clear Cell Renal Cell Carcinoma: A Study with TCGA Database. Dis Markers 2019;2019:8901649 [Crossref] [PubMed]
- Zhang H, Wei P, Lv W, et al. MELK is Upregulated in Advanced Clear Cell Renal Cell Carcinoma and Promotes Disease Progression by Phosphorylating PRAS40. Cell Transplant 2019;28:37S-50S. [Crossref] [PubMed]
- Huang J. Current developments of targeting the p53 signaling pathway for cancer treatment. Pharmacol Ther 2021;220:107720 [Crossref] [PubMed]
- Sjödahl G, Lövgren K, Lauss M, et al. Infiltration of CD3+ and CD68+ cells in bladder cancer is subtype specific and affects the outcome of patients with muscle-invasive tumors. Urol Oncol 2014;32:791-7. [Crossref] [PubMed]
- Necchi A, Madison R, Raggi D, et al. Comprehensive Assessment of Immuno-oncology Biomarkers in Adenocarcinoma, Urothelial Carcinoma, and Squamous-cell Carcinoma of the Bladder. Eur Urol 2020;77:548-56. [Crossref] [PubMed]
- Gao X, Yang J, Chen Y. Identification of a four immune-related genes signature based on an immunogenomic landscape analysis of clear cell renal cell carcinoma. J Cell Physiol 2020;235:9834-50. [Crossref] [PubMed]