The potential prognostic values of the ADAMTS-like protein family: an integrative pan-cancer analysis
Introduction
In addition to providing structural integrity, the biomechanical properties of extracellular matrices (ECMs) are critical in regulating intercellular communication, cell migration, and function (1). Emerging evidence has indicated that ECM accumulation and remodeling are key events in diseases, such as fibrosis and cancer (2,3). ECM homeostasis is regulated by various secreted proteins, including proteases, modifying enzymes, cross-linkers, and protease inhibitors, and alterations in the activities of these regulators facilitate tumor cell proliferation and metastatic dissemination, which suggests that they are potential targets for cancer therapy (4). Among the ECM regulators, a disintegrin-like and metalloproteinase domain with thrombospondin type 1 motifs (ADAMTS) proteins are known to be involved in elastic fiber and microfibril formation, and ECM-associated signal transduction processes (5,6).
The superfamily of glycoproteins consists of 19 secreted ADAMTS proteases and 7 ADAMTS-like proteins (including ADAMTSL1-6 and papilin) in mammals, which are present in the ECM or associated with the cell surface (7). ADAMTS proteases are zinc metalloproteases characterized by protease domains at the N-terminus and ancillary domains containing 1 or more ADAMTS repeats, cysteine-rich modules, and spacer modules in the C-terminus (8). They are involved in a variety of biological processes, including procollagen maturation, and ECM proteolysis related to morphogenesis, angiogenesis, and cancer (9-11). ADAMTSL proteins show homology to ancillary domains of ADAMTS proteases, including thrombospondin type I repeats, a cysteine-rich domain, and an ADAMTS spacer, but lack the N-terminal protease domain and a proteolytically active motif within the ADAM spacer of ADAMTS proteases. Thus, the ADAMTSL proteins are not involved in proteolytic activity (5,12). ADAMTSL proteins appear to regulate ECM assembly and ADAMTS activity (7). Mutations in several ADAMTSL proteins, such as ADAMTSL1, ADAMTSL2, ADAMTSL3, ADAMTSL4, ADAMTSL5 and ADAMTSL6 (also known as THSD4), lead to connective tissue or eye disorders or thoracic aortic aneurysms by binding fibrillins and regulating the assembly, stability, and anchorage of microfibrils (13).
Recently, several studies have explored the role of ADAMTSL proteins in the initiation and progression of cancers. For example, ADAMTSL1 was deposited in the cell substratum and act in the extracellular matrix (14). ADAMTSL1 has been shown to regulate chondrosarcoma cell proliferation, and its germline variation is associated with the prognosis of young women following breast cancer treatment (14). ADAMTSL2 mutations led to geleophysic dysplasia, including stiff skin and cardiac anomalies (15). ADAMTSL3 and ADAMTSL5 suppress hepatocellular carcinoma proliferation and metastasis (16,17). In particular, ADAMTSL4 was localized to the lens equator and anchored microfibrils into the lens capsule (18). ADAMTSL4 is correlated with immune-related processes in glioblastoma (GBM) and represents the infiltration of immune cells and a complicated tumor microenvironment (TME) (19). Interestingly, ADAMTSL2 affects transforming growth factor beta (TGFβ) activity by binding to fibrillin-1 and latent TGFβ-binding protein-1 (15,20), and ADAMTSL4 interacts specifically with lysyl oxidases (21). Studies have identified two new members of ADAMTSL proteins such as ADAMTSL-6alpha and -6beta, which were association with fibrillin-1-rich microfibrils in elastic connective tissues (12). Furthermore, recent studies demonstrated that adamalysin family (consisting of ADAM, ADAMTS, ADAMTSL) were associated with TME by regulating the inflammatory response and the epithelial-mesenchymal transition in hepatocellular carcinoma (22). The host ADAM28-deficient was correlated with a decreased lung infiltration by CD8+ T lymphocytes, suggesting ADAM28 involved in T cell mobilization and TME regulation (23).
The comprehensive characteristics of ADAMTS were explored in various cancer types. On the one hand, different ADAMTS revealed variable biological functions. Although the mutations in ADAMTS10 and ADAMTS17 could cause lenticular myopia and spherophakia, ADAMTS17 mutations have been screened in patients with ‘Weill-Marchesani-like’ syndrome, suggesting different ADAMTS contributed different roles (24). In addition, a type of ADAMTSL played different roles in different diseases. Studies have showed that ADAMTSL-2 was associated with immune response and glucose metabolism in patients with coronary heart disease with congenital cold syndrome (25). Deletion of ADAMTSL-2 led to abnormal differentiation and proliferation of chondrocytes in dwarfism (20).
The recent study investigated the roles of ADAM-like protein family in hepatocellular carcinoma (HCC) (22). The over-expression of these ADAMs predicted poor prognosis in HCC. ADAM18, ADAM21 and ADAM32 were recently associated with HCC invasion (22,26). Conversity, low expression of ADAMTS5 and ADAMTS8 predicted poor prognosis and inhibited the progression of tumor in HCC cells (22,27,28). The balance of pro-tumor and anti-tumor roles of this family were critical processes in cancer progression (22,29).
Thus, the ADAMTSL protein family plays an important role in tumor development and progression. However, the involvement of ADAMTSLs in drug responses, subtypes of infiltrating immune cells, and the TME has received less attention, and studies systematically characterizing their functions in a cancer setting are urgently needed.
In this study, we investigated the pan-cancer effects and potential mechanisms of ADAMTSL proteins by integrating The Cancer Genome Atlas (TCGA) data, annotated knowledge resources and by performing a comprehensive proteomic analysis to identify and characterize the specific functions of ADAMTSL proteins in various cancers. We evaluated the expression and prognostic patterns of ADAMTSLs across 33 diverse tumors. Their associations with drug responses, subtypes of infiltrating immune cells, and the TME were also investigated. To study these genes in a non-small cell lung cancer (NSCLC) cohort, especially their roles in regulating the TME and immune checkpoint inhibitor (ICI) sensitivity, we analyzed the association between the expression of ADAMTSLs and the outcomes of NSCLC patients treated with ICIs from the Gene Expression Omnibus (GEO) repository (GSE135222). A proteomic analysis was also conducted to investigate the related proteins of THSD4 or PAPLN. The results of this systematic study will assist with ADAMTSL-related cancer research and targeted treatment.
We present the following article in accordance with the REMARK reporting checklist (available at https://dx.doi.org/10.21037/atm-21-4946).
Methods
Data sets used
The pan-cancer data from TCGA, including messenger ribonucleic acid (mRNA) sequence data (RNA SeqV2 RSEM), clinical prognostic data, stemness scores, and immune subtypes in the Xena Browser (https://xenabrowser.net/datapages/), were downloaded. Exairesis samples of primary tumors from patients who had not received preoperative neoadjuvant therapy were used as tumor samples from TCGA. In the inter-tumor/pan-tumor analysis, gene expression was normalized to TATA-box binding protein (TBP). Thirty-three cancer types were included in the analysis; their abbreviations are list in the Figures 1,2. Specifically, a total of 11057 samples were used, and the sample size for each cancer type ranged from 45 (for CHOL) to more than 1,000 cases (BRCA). Eighteen cancer types were selected to examine whether the expression of ADAMTSLs was dysregulated in tumor tissue compared to normal tissue, for which more than 5 adjacent normal samples were available. All tumor samples were used to investigate the association between the expression of ADAMTSLs and patients’ overall survival (OS) (30).
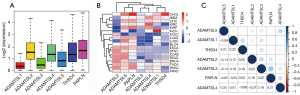
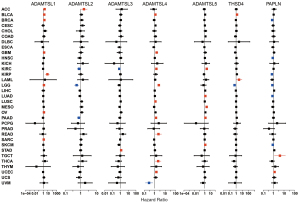
The GEO GSE135222 data set was selected to examine correlations between ADAMTSL expression levels and ICI treatment sensitivity in patients with NSCLC. This cohort comprised 27 patients with advanced NSCLC who had been treated with anti-programmed cell death protein 1/programmed death-ligand 1 (PD-1/PD-L1) inhibitors and had detailed mRNA expression data available. Kaplan-Meier curves were generated, X-tile software was used to cut off the high and low expression of genes in the survival analysis (31), and univariate survival distributions were compared using the log-rank test.
The National Cancer Institute (NCI)-60 database (https://discover.nci.nih.gov/cellminer/) was used to investigate the relationship between the expression of ADAMTSLs in cancer cells and responses to drug treatment. The NCI-60 database contains data on 60 different cancer cell lines from a total of 9 different tumors. The baseline gene expressions of the 60 different cancer cell lines were obtained from GSE32474. The z scores for cell sensitivity (GI50) for 262 drugs and expression levels of ADAMTSL mRNAs were also retrieved. The study was conducted in accordance with the Declaration of Helsinki (as revised in 2013).
TME analyses
The stromal and immune scores, which reflect the levels of infiltrating stromal cells and immune cells in tumors, and the estimated score, which describes tumor purity, were evaluated using the algorithm, ESTIMATE (32). The analysis was based on mRNA expression profiles from TCGA (http://bioinformatics.mdanderson.org/estimate/). The expression of ADAMTSLs in 6 immune cell subtypes that were defined by integrating major immunogenomics methods to measure immune cell infiltrates in the TME were evaluated (33). Two stemness indices, including RNA single strand (RNAss) based on mRNA expression and DNA single strand (DNAss) based on methylation data from publicly available non-tumor and tumor data sets, were used to measure tumor stemness features (34).
Proteomic analysis of patients with LUAD
Primary tumor tissues resected from 18 LUAD samples were collected at The Second Affiliated Hospital of Chongqing Medical University. The samples were quickly frozen in liquid nitrogen after resection, then stored in a refrigerator at –80 °C until they were used in the proteomic analysis. Informed consent was obtained from all subjects involved in the study. All human experiments were approved by the Ethics Committee of our hospital.
The proteomes were analyzed using data-independent acquisition (DIA) quantitation. Briefly, the samples were lysed and sonicated in SDT buffer (2% SDS, 100 mM DTT, 100 mM Tris-HCL, PH 7.6). The protein concentration of the supernatant was measured using a Bicinchoninic Acid Protein Assay Kit (P0012, Beyotime). Filter-aided sample preparation digestion was conducted as previously reported (34). In total, 200 µg of proteins for each sample were digested, and the resulting peptides were collected.
Peptides from all samples were fractionated by RP (reversed phase) chromatography using high-performance liquid chromatography (Agilent 1260 infinity II). The mixture of peptide was loaded onto a XBridge Peptide BEH C18 Column (130 Å, 5 µm, 4.6 mm × 100 mm), which was diluted with Buffer A (10 mM HCOONH4 and 5% ACN, pH 10.0). The flow rate was 1 mL/min with a linear gradient of 5–45% Buffer B (10 mM HCOONH4 and 85% ACN, pH 10.0) within 40 min. The temperature of the column was maintained at 30°C, and 36 fractions were collected every 1 min from the 5th min, and dried by vacuum centrifugation. For spectral library generation, the peptides were re-dissolved in 0.1% formic acid (Buffer A) and analyzed using online nano-liquid chromatograph-mass spectrometer (LC-MS)/mass spectrometer (MS) on Q Exactive™ HF-X coupled to an EASY-nLC 1200 system (Thermo Fisher Scientific, MA, USA). 2 µL of peptides was loaded on an analytical column (Thermo Fisher Scientific, Acclaim PepMap RSLC 50 µm × 15 cm, nano viper, P/N164943). The column temperature was maintained at 55 °C, and the charge mode was positive. The sample was separated at a flow rate of 300 nL/min with the following gradients: 0–5 min, 1% B (B: 0.1% formic acid, 80% ACN); 5–95 min, 1–28% B; 95–110 min, 28–38% B; 110–115 min, 38–100% B; 115–120 min, 100% B. The electrospray voltage of 2 kV versus the inlet of the mass spectrometer was used. The parameters of the mass spectrometer were as follows: (I) MS: scan range (m/z) =350–1,500; Automatic Gain Control (AGC) target =3e6; resolution =60,000; maximum injection time =30 ms; dynamic exclusion =30 s; include charge states =2–7; (II) HCD-MS/MS: isolation window =1.6 m/z; AGC target =1e5; resolution =15,000; maximum injection time =45 ms; collision energy =28. It was automatically switched between the MS and MS/MS mode. The raw data of data-dependent acquisition were analyzed using Spectronaut Pulsar X (version 12, Biognosys AG, Switzerland). Spectronaut was set up to search the UniProt Homo sapiens_20367_20200226 database. A Q value [false discovery rate (FDR)] cutoff of 1% was applied at the precursor, protein, and peptide levels.
For the sample analysis, the peptides were analyzed using the above nano-LC-MS/MS system. The same parameters were used for LC separation, and the parameters for MS/MS were as follows: (I) MS: scan range (m/z) =350–1,500; AGC target =3e6; resolution =60,000; maximum injection time =50 ms; (II) HCD-MS/MS: AGC target =2e5; resolution =15,000; maximum injection time =45; collision energy =28. A DIA was performed with a variable isolation window, and the total cycle time was 3 s, and the window number was 42, each window overlapped 1 m/z.
Statistical analyses
The gene expression in all the tumors and between the tumor and normal tissues was compared using the linear mixed-effects models. The differences in gene expression across tumor types are shown in boxplots, and the differential expression compared to normal tissue is displayed in a heatmap. The correlations between levels of ADAMTSLs and patients’ OS were examined using univariate Cox proportional hazard regression models. Spearman’s or Pearson’s correlation coefficients were calculated to determine the associations between the expression of ADAMTSLs and the stromal score, immune score, ESTIMATE score, stemness scores, programmed cell death 1 ligand 2 (PDCD1LG2), programmed cell death 1 (PDCD1), cytotoxic T-lymphocyte associated protein 4 (CTLA4), lymphocyte activating 3 (LAG-3), hepatitis A virus cellular receptor 2 (HAVCR2) and CD274 molecule (CD274) expression, and drug sensitivity. The correlations between the expression of ADAMTSLs and subtypes of infiltrating immune cells and cancer subtypes were determined by an analysis of variance. All tests and plots were performed using R (R Core Team). The number of false positives method was used to adjust for multiple comparisons, except for survival studies, which adopted α=0.05 as the cutoff value.
Results
Expression of different ADAMTSL family members in various cancer types
We first conducted a pan-cancer analysis of gene expression across 33 diverse cancer types profiled in TCGA databases to understand the intrinsic expression patterns of ADAMTSL family members. The intra- and inter-tumor heterogeneity of all 7 ADAMTSL members was observed (see Figure 1A and Figure S1); ADAMTSL1 and ADAMTSL3 showed relatively low-expression levels, while ADAMTSL2, ADAMTSL4, THSD4, and PAPLN showed relatively high-expression levels (see Figure 1A). In terms of the expression of each ADAMTSL, striking heterogeneity of expression was observed across different cancer types; for example, KIRP, THCA, and KIRC displayed high levels of PAPLN, while KICH, LIHC, COAD, and READ displayed low levels of PAPLN expression (see Figure S1). Additionally, we compared gene expression in 18 different primary tumors from patients and their corresponding normal tissues samples for tumors for which at least 5 normal samples were available (see Figure 1B and Figure S1). As the heatmap shows, they were separated into 3 major clusters, among which 1 cluster included ADAMTSL5 and PAPLN, which were predominantly upregulated. Another cluster was further separated into 2 subclusters. 1 subcluster included ADAMTSL3 and ADAMTSL4, and the other subcluster included ADAMTSL1 and THSD4, which were predominantly downregulated, with a few exceptions. In addition, ADAMTSL2 showed mixed up- or downregulation in different cancer types. According to Spearman’s correlation tests results, the expression of ADAMTSLs were positively or negatively correlated with each other, and ADAMTSL3 and PAPLN pair showed the strongest correlation (r=0.32; P<0.0001) averaged across cancer types, which suggests that these 2 genes share some common features or functions (see Figure 1C and Table S1).
The expression of ADAMTSLs is associated with patient OS
Univariate Cox proportional hazard regression models were used to evaluate the association between the expression of ADAMTSLs and the OS of patients with each of the 33 cancer types. As the forest plots show (see Figure 2), the altered expression of ADAMTSLs was associated with patient survival (a significant association was defined as a P value <0.05 without adjustment for multiple comparisons). A general pattern was observed whereby an increase in gene expression was usually associated with a decrease in patient survival, where ADAMTSL1 predicted a poor prognosis of patients with ACC, BLCA, BRCA, GBM, KIRP, LGG, OV, and SARC, and ADAMTSL5 predicted a poor prognosis of patients with KIRC, LUAD, MESO, PAAD, and SKCM. Additionally, increased ADAMTSL4 expression was mainly associated with a survival disadvantage with BLCA, GBM, KIRC, LGG, LUSC, PAAD, READ, THCA, and UCEC, but predicted a survival advantage for patients with UVM. Conversely, PAPLN was primarily associated with longer OS and predicted an OS advantage for patients with BRCA, HNSC, KIRP, LGG, LUAD, and SKCM, but predicted an OS disadvantage for patients with TGCT and UCEC. The remaining ADAMTSLs were associated with either an OS advantage or disadvantage depending on the cancer type. For example, ADAMTSL2 was associated with shorter OS for patients with ACC but longer OS for patients with KIRC, LGG, and PAAD. THSD4 predicted worse outcomes for patients with BLCA and LAML, but favored survival for LGG. ADAMTSL3 predicted a poor prognosis for patients with STAD only, and predicted a better prognosis for patients with KIRC.
ADAMTSLs are associated with tumor stemness and chemosensitivity
Cancer stem cells (CSCs) are responsible for driving tumor growth, metastasis, and chemoresistance (35). Based on accumulating evidence, the ECM, 1 of the CSC niches, provides structural and biochemical support to regulate the self-renewal, proliferation, and differentiation of CSCs (36). The two stemness indices of RNAss and DNAss were developed using data compiled by TCGA. RNAss reflects gene expression, while DNAss reflects epigenetic features (37). In this study, the correlations between ADAMTSL expression and tumor stemness scores were explored. Across all cancer types, most ADAMTSLs were negatively correlated with the RNAss score to varying degrees (r ranging from –0.443 to –0.176; P<0.001), except ADAMTSL5. In relation the correlation with the DNAss score, ADAMTSL4 and ADAMTSL5 showed positive correlations, and other genes showed negative correlations, while all members showed negligible correlations (see Table S2). At the level of the individual cancer type (see Figure 3A,3B), among the significant correlations observed between the expression of ADAMTSLs with RNAss and DNAss, all ADAMTSL members were negatively correlated with RNAss, except for ADAMTSL5 in PAAD (r=0.21) and THSD4 in ACC (r=0.31) and LGG (r=0.38). Additionally, positive or negative correlations were observed with DNAss depending on the cancer type tested. Notably, the strongest associations with OV were observed for ADAMTSL1 (r=–0.79) and ADAMTSL5 (r=0.75). In thymoma, all family members, except ADAMTSL2, were positively correlated with DNAss but negatively correlated with RNAss. These contradictory results indicate that the 2 stemness indices may identify distinct cancer cell populations in different cancers characterized by different features or degrees of stemness (27).
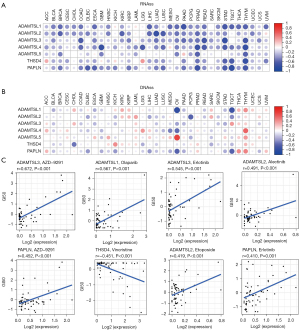
We next investigated ADAMTSL expression in NCI-60 cell lines and the correlations with the drug sensitivity scores (measured by Z scores) of over 200 chemotherapy drugs (38). A higher score indicates that the cell is more sensitive to drug treatment (see Figure 3C and Table S3). ADAMTSL members showed a strong correlation with drug responses (|r|≥0.4 and P<0.01); ADAMTSL1, ADAMTSL2, ADAMTSL3, and PAPLN were mainly correlated with increased sensitivity to a list of drugs, while ADAMTSL4, ADAMTSL5, and THSD4 were mainly correlated with increased drug resistance. Interestingly, ADAMTSL3 and PAPLN were positively correlated with cell sensitivity to erlotinib and AZD-9291 (osimertinib), which are 1st- or 3rd-generation tyrosine kinase inhibitors of epidermal growth factor receptor (EGFR-TKIs), respectively, for the treatment of NSCLC with EGFR mutations. Additionally, ADAMTSL2 was associated with sensitivity to alectinib, a treatment for NSCLC with anaplastic lymphoma kinase mutations. ADAMTSL1 was also positively correlated with sensitivity to olaparib, a treatment for advanced ovarian cancer with BRCA mutations. The sensitivity of cells to some other anticancer drugs, such as etoposide (a treatment for small cell lung cancer), afatinib (a treatment for locally advanced or metastatic NSCLC with EGFR mutations), lapatinib (a treatment for breast cancer with HER2 overexpression), and vincristine (a treatment for lymphoma) was also associated with the expression levels of ADAMTSL family members. Thus, the high expression of these aforementioned genes may indicate a better prognosis for patients receiving targeted therapy; however, further research needs to be conducted.
ADAMTSLs are associated with infiltrating immune subtypes and the TME in cancer
The TME, which consists of the ECM, infiltrating immune cells, connective tissue, and tumor vasculature, plays a pivotal role in tumor immune escape, and TME remodeling has emerged as a strategy to promote cancer treatment, such as PD-1/PD-L1-based immunotherapy (39). The ECM controls intratumor signaling, metabolism, oxygenation, transport mechanisms, and immunogenicity, and a loss of regulatory control of the ECM affects not only the malignancy and growth of the tumor but also its response to treatment (40). ADAMTSL proteins regulate ECM assembly and/or ADAMTS activity, which is primarily associated with proteolytic events in the ECM (7). Thus, the correlations between ADAMTSL levels and immune cell infiltrates in tumors, and their associations with several immune blockade molecules were tested to explore how each ADAMTSL family member is associated with the TME.
By integrating major immunogenomics methods to characterize the immune TME spanning multiple tumor types analyzed in TCGA, the following 6 immune subtypes were identified: wound healing (C1), interferon-gamma (IFN-γ) dominant (C2), inflammatory (C3), lymphocyte-depleted (C4), immunologically quiet (C5), and TGFβ-dominant (C6) (26). These subtypes have potential prognostic implications; for example, C3 and C5 indicate better OS prognoses, while C4 and C6 had the least favorable outcomes. We compared ADAMTSL expression among the 6 immune subtypes (see Figure 4A). The expression of different ADAMTSL genes exhibited distinct association patterns with different subtypes of infiltrating immune cells. Specifically, ADAMTSL1 and ADAMTSL4 showed higher expression in subtype C6, and ADAMTSL5 expression was increased in C1, C2, and C6, indicating that they may be predictors of a poor prognosis due to a higher proliferation rate and the enrichment of TGFβ. Conversely, PAPLN expression was significantly increased in C3 and decreased in C4, which was correlated with a longer OS. Additionally, ADAMTSL2 showed increased expression in C5. Further, the expression of ADAMTSL3 and THSD4 increased in subtypes C3 and C6; thus, their roles in predicting prognosis may differ. These results were consistent with those on the relationship between the expression of ADAMTSLs and patient outcomes (mentioned above).
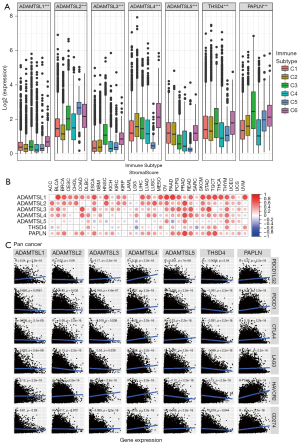
As ADAMTSLs can function as regulators in the course of ECM remodeling, which regulates major biological processes, such tumor growth and metastasis, we used the algorithm, ESTIMATE to further investigate the correlation between the expression of ADAMTSLs and stromal scores, immune scores and estimate scores, which reflect stromal cells and immunes cell infiltration in the TME, and tumor purity (see Figure 4B and Figure S2). The majority of cancer types demonstrated a significantly positive correlation between the stromal score and the expression of ADAMTSL1, ADAMTSL2, ADAMTSL3, ADAMTSL4, and PAPLN. Across the tested cancer types, ADAMTSL3 had the highest positive correlation (r=0.313), followed by ADAMTSL1 (r=0.294), and ADAMTSL4 (r=0.287) (see Table S2). More specifically, significant positive correlations between ADAMTSL1 and the stromal scores in BLCA (r=0.73), OV (r=0.72), and PAAD (r=0.79), and between PAPLN expression, and the stromal score in PRAD (r=0.73) were identified (P<0.0001). ADAMTSL5 and THSD4 showed either positive or negative correlations depending on the cancer type tested.
Recently, immunotherapy has revolutionized the management of patients with cancer. Thus, we also tested the correlation between the expression of ADAMTSLs and the immune blockade molecules Programmed Cell Death 1 Ligand 2 (PDCD1LG2) (PD-L2), Programmed Cell Death 1 (PDCD1) (PD-1), Cytotoxic T-Lymphocyte Associated Protein 4 (CTLA4), Lymphocyte Activating 3 (LAG-3), Hepatitis A Virus Cellular Receptor 2 (HAVCR2) (TIM-3), and CD274 Molecule (CD274) (PD-L1). ADAMTSL4 and PAPLN were positively correlated with the selected molecules (P<0.0001), while THSD showed negative correlations with PDCD1, CTLA4, LAG-3, HAVCR2, and CD274 (P<0.0001); however, no significant correlation with PDCD1LG2 was found (P=0.54; see Figure 4C and Table S4). ADAMTSLs is highly correlated with the TME and immune checkpoint molecules, which indicates that ADAMTSLs hold potential as targets and/or immune modulators for cancer therapy.
Expression of the ADAMTSL gene family in NSCLC
Lung cancer is the leading cause of cancer-related mortality worldwide, and among lung cancer cases, NSCLC exhibits the highest prevalence (80–85%) (41). LUAD (approximately 40–50% of cases) and LUSC (approximately 20–30% of cases) comprise the predominant histological subtypes of NSCLC. In recent years, ICI therapies have become a mainstay of first-line treatment for advanced/metastatic NSCLC without targetable genetic alterations. However, a significant proportion of patients experience disease progression. TME plays a vital role in ICI resistance by influencing extrinsic and/or intrinsic resistance pathways (42). The results of the pan-cancer analysis described above revealed that ADAMTSLs are associated with the TME, and we also detected their correlations in patients with NSCLC. In patients with LUAD, the levels of all the ADAMTSLs were positively correlated with the immune score, stromal score, and tumor purity score, except ADAMTSL5 and THSD4, which were not associated with the 3 scores, respectively (see Figure 5A). A similar result was observed in patients with LUSC, except that ADAMTSL5 was negatively associated with the 3 scores (see Figure 5B). ADAMTSL expression showed a significant correlation with the TME in NSCLC, and we presumed that the expression of these genes affects patients’ sensitivity to ICI therapies. In support of this hypothesis, we investigated mRNA expression data (RNA-Sequencing) from the GEO repository (GSE135222). Twenty-seven patients with advanced NSCLC who were treated with ICIs (anti-PD-1/PD-L1 antibodies) were included in this repository. We observed that patients with a low expression of THSD4 or PAPLN experienced significantly prolonged progression-free survival (PFS) compared to the corresponding high-expression groups, with P values of 0.0192 and 0.0023, respectively (see Figure 6A,6B). Most patients with THSD4 or PAPLN-high expression showed no durable benefits during treatment; however, most low-expression patients enjoyed durable clinical benefits. For other ADAMTSL family members, we did not detect any effect of gene expression by tumor cells on PFS.
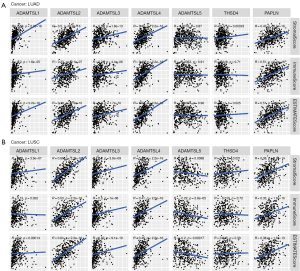
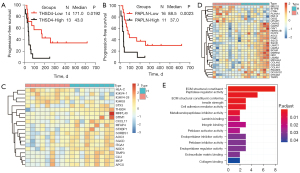
We collected 18 primary LUAD samples from treatment-naive Chinese patients to investigate how the expression of THSD4 or PAPLN affects the proteome and related proteins that may modulate ICI sensitivity. A HPLC/MS-based label-free quantification strategy was adopted. Proteomic measurements of all samples resulted in a total of 5,318 protein groups with a 1% FDR at the precursor, protein, and peptide levels, among which 2,270 were present in all 18 patients. Further, the significantly altered proteins in patients with THSD4 (or PAPLN) high or low expression were examined (Wilcoxon rank-sum test, fold change >1.0, P<0.05, identified in all patient samples). For THSD4, 20 differentially expressed proteins were observed between the THSD4-high and THSD4-low expression groups, among which 16 proteins were upregulated and 4 proteins were downregulated in the THSD4-high expression group (see Figure 6C and Figure S3A). For PAPLN, we identified 30 proteins that were significantly positively or negatively correlated with PAPLN expression, among which 23 were upregulated and 7 were downregulated (see Figure 6D and Figure S3B). An enrichment analyses of the altered proteins identified the GO function that had relatively strong correlations, which were mainly involved in ECM structural constituent, peptidase regulator activity, cell adhesion mediator activity, and metalloendopeptidase inhibitor activity (see Figure 6E). Some of the related proteins were involved in TME regulation or host immune response, such as integrin alpha 1 (ITGA1), TIMP Metallopeptidase Inhibitor 3 (TIMP3), and CXCL17, indicating that THSD4 or PAPLN may interact with these proteins directly or indirectly, thereby causing immunotherapy resistance.
Discussion
The human ADAMTSL superfamily appears to be relevant to ECM functions or cell-matrix interactions, and their functions first emerged from the analysis of spontaneous human mutations and genetically engineered cell lines (7,43). Recently, their roles in tumor growth and TME regulation have been increasingly recognized. In this study, we performed a comprehensive investigation of the pan-cancer effects of ADAMTSLs. The expression of ADAMTSLs showed high heterogeneity among different tumor types, and the dysregulated ADAMTSLs were generally associated with patients OS with 33 cancer types. For example, ADAMTSL1 expression was decreased in BLCA, BRCA, and KIRP, and ADAMTSL4 expression was decreased in BLCA, LUSC, READ, and UCEC (see Figure S1A,S1D), and their downregulation was also associated with OS disadvantages for patients with those types of cancers (see Figure 2). In a previous study, ADAMTSL4 combined with 12 other genes were established as risk score system to predict the prognosis of clear cell renal cell carcinoma (44), and another study found that a 4 ECM-related gene (including ADAMTSL4) signature might serve as a marker to evaluate the prognosis of esophageal squamous cell carcinoma (45). For these two types of cancer, our study showed that ADAMTSL4 alone was associated with KIRC but not ESCA; thus, it is meaningful to establish ADAMTSL-based prognostic models for other cancer types.
CSCs promote cancer progression and the development of resistance to conventional anticancer approaches, such as chemotherapy, targeted therapy, and immunotherapy (35). The present study showed that ADAMTSL expression is associated with tumor stemness and drug sensitivity. ADAMTSL1, ADAMTSL2, ADAMTSL3, ADAMTSL5, and PAPLN had the highest correlations to a number of molecularly targeted drugs. Previous research has shown that targeting ADAMTSL5 in hepatocellular carcinoma cells interfered with tumorigenic properties, and that ADAMTSL5 acts upstream of several key oncogenic pathways, such as EGFR, MET, PDGFRβ, IGF1Rβ, and FGFR4. This phenotype confers sensitivity to several targeted drugs, including lenvatinib, sorafenib, and regorafenib (16). Together with the present results, we speculated that ADAMTSL family members were multifunctional and that the secreted ADAMTSLs act in a non-cell autonomous fashion, and as potential master regulators inside cells. Research has focused on deciphering the distinct molecular mechanisms underlying drug resistance. In this study, we showed the putative emerging role of ADAMTSLs in modulating targeted drug resistance and provided a new direction for studies on prognostic biomarkers.
By exploring the expression of ADAMTSLs in different subtypes of infiltrating immune cells in the TME, we found that ADAMTSL1, ADAMTSL3, ADAMTSL4, ADAMTSL5, and THSD4 were correlated with more aggressive immune cell subtypes (i.e., C1, C2, and C6), which indicated their association with a poor prognosis. Additionally, ADAMTSL3 and THSD4 were increased in subtype C3, and ADAMTSL2 and PAPLN showed the highest expression in subtypes C3 and C5, which are correlated with a better prognosis. ADAMTSLs are also associated with immune cell and stromal cell infiltrates to various degrees, and some immune blockade molecules. We further investigated their correlations with the TME in patients with NSCLC and their roles in the response to immunotherapy to confirm the functions of ADAMTSLs as proinflammatory and immune modulators. In patients with LUAD, ADAMTSL4 and PAPLN showed strong correlations with the immune score, stromal score, and tumor purity score (r ranging from 0.43 to 0.52; P<0.0001). In patients with LUSC, ADAMTSL2 showed the highest correlation with the TME (r ranging from 0.53 to 0.69; P<0.0001), followed by ADAMTSL4 and PAPLN. By analyzing the association between ADAMTSL expression and the outcomes of 27 advanced NSCLC patients who were treated with ICIs (anti-PD-1/PD-L1) from the GEO repository (GSE135222), we determined that THSD4 and PAPLN were negatively correlated with PFS, which suggests that they may serve as potential predictive biomarkers of the immunotherapy response.
The ICI resistance mechanism stems from the evolving interaction between tumor cells and the surrounding cell populations in the TME. Proteins are the functional executors of the cell; thus, we performed a proteomic analysis of samples from 18 patients with LUAD to identify THSD4- or PAPLN-related proteins that may influence ICI sensitivity. 20 and 30 proteins related to THSD4 and PAPLN were detected, respectively. A considerable portion of the altered proteins is related to the ECM, which suggests that these proteins may interact with each other to regulate the TME. Additionally, 4 proteins (i.e., ITGA1, SGCD, AOC3, and TIMP3) were positively related to both THSD4 and PAPLN. Some of these are well correlated with the clinical outcomes of cancer patients (46-48). Among them, TIMP3 (a tissue inhibitor of metalloproteinases) has been studied for its effects on regulating the pericellular proteolysis of a large number of cell surface proteins and matrices, generating effects on both cell signaling and tumor architecture (48). Additionally, ITGA1 provides dynamic cell-to-cell linkage and cell attachment to ECM components, which, in contrast to most other cell receptors, drives inside-out and outside-in signaling. In pancreatic cancer, the ITGA1-specific collagens expression is significantly correlated with poor patient prognosis (49). Additionally, ITGA1/collagen signaling promotes ALDH1-positive CSCs survival and cooperates with TGFβ to drive therapy resistance (49).
AOC3 encodes amine oxidase copper containing 3 (also known as VAP-1), which is an endothelial adhesion molecule expressed in tumor vascular endothelial cells and certain tumors. It contributes to the extravasation of neutrophils, lymphocytes, and macrophages to sites of inflammation, and is involved in immunosuppressive TME generation. The VAP-1-specific inhibitor U-V296 exerts synergistic antitumor effects with ICIs by increasing the number of IFN-γ-induced CD8 T cells (50). Notably, we found that some THSD4-related proteins were involved in the host immune response, such as HLA-C, NOD1, and CXCL17, among which CXCL17, a dendritic cell and monocyte chemoattractant, was found to be strongly correlated with immunomodulatory molecule B7-H4 expression in the TME (51). These results provide a resource to explore the possible mechanism by which THSD4 or PAPLN affects the efficacy of ICIs. Of course, the correlation between THSD4 (or PAPLN) expression and ICI responses revealed in the present study must be validated in the clinic.
Conclusions
In summary, our comprehensive pan-cancer analysis of the ADAMTSL family illustrates the characteristics of ADAMTSLs in various cancer types. Based on the present data, ADAMTSLs serve as prognostic factors for some cancer types. Further analysis indicated that ADAMTSLs may modulate drug responses and are frequently correlated with a number of molecularly targeted drugs and infiltrating immune cells subtypes in the TME. In the NSCLC cohort from the GEO repository (GSE135222), a comprehensive proteomic analysis of 18 cases of LUAD showed that THSD4 and PAPLN were potential biomarkers for the ICI response, and their related proteins were mainly ECM structural constituents. Additionally, some other THSD4-related proteins participated in the host immune response. This study lays a foundation for a comprehensive understanding of the role of ADAMTSLs in cancers and for the development of novel therapeutic approaches.
Acknowledgments
We gratefully acknowledge the assistance of BeiGene.
Funding: This research was funded by National NaturalScience Foundation of China (grant number 82002448 to X Zhang and 81802984 to Y Peng) and Chongqing Postdoctoral Science Foundation (grant number cstc2019jcyj-bshX0056 to X Zhang).
Footnote
Reporting Checklist: The authors have completed the REMARK reporting checklist. Available at https://dx.doi.org/10.21037/atm-21-4946
Conflicts of Interest: All authors have completed the ICMJE uniform disclosure form (available at https://dx.doi.org/10.21037/atm-21-4946). All authors reported that this research was funded by National Natural Science Foundation of China (grant number 82002448 to XZ and 81802984 to YP) and Chongqing Postdoctoral Science Foundation (grant number cstc2019jcyj-bshX0056 to XZ). The authors have no other conflicts of interest to declare.
Ethical Statement: The authors are accountable for all aspects of the work in ensuring that questions related to the accuracy or integrity of any part of the work are appropriately investigated and resolved. The study was conducted in accordance with the Declaration of Helsinki (as revised in 2013).
Open Access Statement: This is an Open Access article distributed in accordance with the Creative Commons Attribution-NonCommercial-NoDerivs 4.0 International License (CC BY-NC-ND 4.0), which permits the non-commercial replication and distribution of the article with the strict proviso that no changes or edits are made and the original work is properly cited (including links to both the formal publication through the relevant DOI and the license). See: https://creativecommons.org/licenses/by-nc-nd/4.0/.
References
- Walma DAC, Yamada KM. The extracellular matrix in development. Development 2020;147:dev175596 [Crossref] [PubMed]
- Mohan V, Das A, Sagi I. Emerging roles of ECM remodeling processes in cancer. Semin Cancer Biol 2020;62:192-200. [Crossref] [PubMed]
- Taha IN, Naba A. Exploring the extracellular matrix in health and disease using proteomics. Essays Biochem 2019;63:417-32. [Crossref] [PubMed]
- Lorusso G, Rüegg C, Kuonen F. Targeting the Extra-Cellular Matrix-Tumor Cell Crosstalk for Anti-Cancer Therapy: Emerging Alternatives to Integrin Inhibitors. Front Oncol 2020;10:1231. [Crossref] [PubMed]
- Hubmacher D, Apte SS. ADAMTS proteins as modulators of microfibril formation and function. Matrix Biol 2015;47:34-43. [Crossref] [PubMed]
- Binder MJ, McCoombe S, Williams ED, et al. The extracellular matrix in cancer progression: Role of hyalectan proteoglycans and ADAMTS enzymes. Cancer Lett 2017;385:55-64. [Crossref] [PubMed]
- Dubail J, Apte SS. Insights on ADAMTS proteases and ADAMTS-like proteins from mammalian genetics. Matrix Biol 2015;44-46:24-37. [Crossref] [PubMed]
- Apte SS. A disintegrin-like and metalloprotease (reprolysin-type) with thrombospondin type 1 motif (ADAMTS) superfamily: functions and mechanisms. J Biol Chem 2009;284:31493-7. [Crossref] [PubMed]
- Mead TJ, Apte SS. ADAMTS proteins in human disorders. Matrix Biol 2018;71-72:225-39. [Crossref] [PubMed]
- Rienks M, Barallobre-Barreiro J, Mayr M. The Emerging Role of the ADAMTS Family in Vascular Diseases. Circ Res 2018;123:1279-81. [Crossref] [PubMed]
- Fontanil T, Mohamedi Y, Cobo T, et al. Novel Associations Within the Tumor Microenvironment: Fibulins Meet ADAMTSs. Front Oncol 2019;9:796. [Crossref] [PubMed]
- Tsutsui K, Manabe R, Yamada T, et al. ADAMTSL-6 is a novel extracellular matrix protein that binds to fibrillin-1 and promotes fibrillin-1 fibril formation. J Biol Chem 2010;285:4870-82. [Crossref] [PubMed]
- Hubmacher D, Taye N, Balic Z, et al. Limb- and tendon-specific Adamtsl2 deletion identifies a role for ADAMTSL2 in tendon growth in a mouse model for geleophysic dysplasia. Matrix Biol 2019;82:38-53. [Crossref] [PubMed]
- Hirohata S, Wang LW, Miyagi M, et al. Punctin, a novel ADAMTS-like molecule, ADAMTSL-1, in extracellular matrix. J Biol Chem 2002;277:12182-9. [Crossref] [PubMed]
- Le Goff C, Morice-Picard F, Dagoneau N, et al. ADAMTSL2 mutations in geleophysic dysplasia demonstrate a role for ADAMTS-like proteins in TGF-beta bioavailability regulation. Nat Genet 2008;40:1119-23. [Crossref] [PubMed]
- Arechederra M, Bazai SK, Abdouni A, et al. ADAMTSL5 is an epigenetically activated gene underlying tumorigenesis and drug resistance in hepatocellular carcinoma. J Hepatol 2021;74:893-906. [Crossref] [PubMed]
- Zhou X, Li R, Jing R, et al. Genome-wide CRISPR knockout screens identify ADAMTSL3 and PTEN genes as suppressors of HCC proliferation and metastasis, respectively. J Cancer Res Clin Oncol 2020;146:1509-21. [Crossref] [PubMed]
- Gabriel LA, Wang LW, Bader H, et al. ADAMTSL4, a secreted glycoprotein widely distributed in the eye, binds fibrillin-1 microfibrils and accelerates microfibril biogenesis. Invest Ophthalmol Vis Sci 2012;53:461-9. [Crossref] [PubMed]
- Zhao Z, Zhang KN, Chai RC, et al. ADAMTSL4, a Secreted Glycoprotein, Is a Novel Immune-Related Biomarker for Primary Glioblastoma Multiforme. Dis Markers 2019;2019:1802620 [Crossref] [PubMed]
- Delhon L, Mahaut C, Goudin N, et al. Impairment of chondrogenesis and microfibrillar network in Adamtsl2 deficiency. FASEB J 2019;33:2707-18. [Crossref] [PubMed]
- Aviram R, Zaffryar-Eilot S, Hubmacher D, et al. Interactions between lysyl oxidases and ADAMTS proteins suggest a novel crosstalk between two extracellular matrix families. Matrix Biol 2019;75-76:114-25. [Crossref] [PubMed]
- Théret N, Bouezzedine F, Azar F, et al. ADAM and ADAMTS Proteins, New Players in the Regulation of Hepatocellular Carcinoma Microenvironment. Cancers (Basel) 2021;13:1563. [Crossref] [PubMed]
- Gérard C, Hubeau C, Carnet O, et al. Microenvironment-derived ADAM28 prevents cancer dissemination. Oncotarget 2018;9:37185-99. [Crossref] [PubMed]
- Morales J, Al-Sharif L, Khalil DS, et al. Homozygous mutations in ADAMTS10 and ADAMTS17 cause lenticular myopia, ectopia lentis, glaucoma, spherophakia, and short stature. Am J Hum Genet 2009;85:558-68. [Crossref] [PubMed]
- Xie T, Guo J, Jiang Y, et al. Screening differentially expressed proteins of coronary heart disease with congenital cold syndrome based on tandem mass tag (TMT) technology. Bioengineered 2021;12:1338-50. [Crossref] [PubMed]
- Honda H, Takamura M, Yamagiwa S, et al. Overexpression of a disintegrin and metalloproteinase 21 is associated with motility, metastasis, and poor prognosis in hepatocellular carcinoma. Sci Rep 2017;7:15485. [Crossref] [PubMed]
- Li C, Xiong Y, Yang X, et al. Lost expression of ADAMTS5 protein associates with progression and poor prognosis of hepatocellular carcinoma. Drug Des Devel Ther 2015;9:1773-83. [Crossref] [PubMed]
- Zhao X, Yang C, Wu J, et al. ADAMTS8 targets ERK to suppress cell proliferation, invasion, and metastasis of hepatocellular carcinoma. Onco Targets Ther 2018;11:7569-78. [Crossref] [PubMed]
- Sun Y, Huang J, Yang Z. The roles of ADAMTS in angiogenesis and cancer. Tumour Biol 2015;36:4039-51. [Crossref] [PubMed]
- Zhang X, Shao S, Li L. Characterization of Class-3 Semaphorin Receptors, Neuropilins and Plexins, as Therapeutic Targets in a Pan-Cancer Study. Cancers (Basel) 2020;12:1816. [Crossref] [PubMed]
- Camp RL, Dolled-Filhart M, Rimm DL. X-tile: a new bio-informatics tool for biomarker assessment and outcome-based cut-point optimization. Clin Cancer Res 2004;10:7252-9. [Crossref] [PubMed]
- Yoshihara K, Shahmoradgoli M, Martínez E, et al. Inferring tumour purity and stromal and immune cell admixture from expression data. Nat Commun 2013;4:2612. [Crossref] [PubMed]
- Thorsson V, Gibbs DL, Brown SD, et al. The Immune Landscape of Cancer. Immunity 2018;48:812-830.e14. [Crossref] [PubMed]
- Wiśniewski JR, Zougman A, Nagaraj N, et al. Universal sample preparation method for proteome analysis. Nat Methods 2009;6:359-62. [Crossref] [PubMed]
- Zhang D, Tang DG, Rycaj K. Cancer stem cells: Regulation programs, immunological properties and immunotherapy. Semin Cancer Biol 2018;52:94-106. [Crossref] [PubMed]
- Nallanthighal S, Heiserman JP, Cheon DJ. The Role of the Extracellular Matrix in Cancer Stemness. Front Cell Dev Biol 2019;7:86. [Crossref] [PubMed]
- Malta TM, Sokolov A, Gentles AJ, et al. Machine Learning Identifies Stemness Features Associated with Oncogenic Dedifferentiation. Cell 2018;173:338-354.e15. [Crossref] [PubMed]
- Reinhold WC, Sunshine M, Liu H, et al. CellMiner: a web-based suite of genomic and pharmacologic tools to explore transcript and drug patterns in the NCI-60 cell line set. Cancer Res 2012;72:3499-511. [Crossref] [PubMed]
- Clift R, Souratha J, Garrovillo SA, et al. Remodeling the Tumor Microenvironment Sensitizes Breast Tumors to Anti-Programmed Death-Ligand 1 Immunotherapy. Cancer Res 2019;79:4149-59. [Crossref] [PubMed]
- Brassart-Pasco S, Brézillon S, Brassart B, et al. Tumor Microenvironment: Extracellular Matrix Alterations Influence Tumor Progression. Front Oncol 2020;10:397. [Crossref] [PubMed]
- Sung H, Ferlay J, Siegel RL, et al. Global Cancer Statistics 2020: GLOBOCAN Estimates of Incidence and Mortality Worldwide for 36 Cancers in 185 Countries. CA Cancer J Clin 2021;71:209-49. [Crossref] [PubMed]
- Horvath L, Thienpont B, Zhao L, et al. Overcoming immunotherapy resistance in non-small cell lung cancer (NSCLC) - novel approaches and future outlook. Mol Cancer 2020;19:141. [Crossref] [PubMed]
- Le Goff C, Cormier-Daire V. The ADAMTS(L) family and human genetic disorders. Hum Mol Genet 2011;20:R163-7. [Crossref] [PubMed]
- Zhang C, Wang F, Guo F, et al. A 13-gene risk score system and a nomogram survival model for predicting the prognosis of clear cell renal cell carcinoma. Urol Oncol 2020;38:74.e1-74.e11. [Crossref] [PubMed]
- Zhang H, Shi Q, Yang Z, et al. An Extracellular Matrix-Based Signature Associated With Immune Microenvironment Predicts the Prognosis and Therapeutic Responses of Patients With Oesophageal Squamous Cell Carcinoma. Front Mol Biosci 2021;8:598427 [Crossref] [PubMed]
- Boudjadi S, Bernatchez G, Sénicourt B, et al. Involvement of the Integrin α1β1 in the Progression of Colorectal Cancer. Cancers (Basel) 2017;9:96. [Crossref] [PubMed]
- Chang SJ, Tu HP, Lai YC, et al. Increased Vascular Adhesion Protein 1 (VAP-1) Levels are Associated with Alternative M2 Macrophage Activation and Poor Prognosis for Human Gliomas. Diagnostics (Basel) 2020;10:256. [Crossref] [PubMed]
- Jackson HW, Defamie V, Waterhouse P, et al. TIMPs: versatile extracellular regulators in cancer. Nat Rev Cancer 2017;17:38-53. [Crossref] [PubMed]
- Gharibi A, La Kim S, Molnar J, et al. ITGA1 is a pre-malignant biomarker that promotes therapy resistance and metastatic potential in pancreatic cancer. Sci Rep 2017;7:10060. [Crossref] [PubMed]
- Kinoshita T, Sayem MA, Yaguchi T, et al. Inhibition of vascular adhesion protein-1 enhances the anti-tumor effects of immune checkpoint inhibitors. Cancer Sci 2021;112:1390-401. [Crossref] [PubMed]
- MacGregor HL, Garcia-Batres C, Sayad A, et al. Tumor cell expression of B7-H4 correlates with higher frequencies of tumor-infiltrating APCs and higher CXCL17 expression in human epithelial ovarian cancer. Oncoimmunology 2019;8:e1665460 [Crossref] [PubMed]
(English Language Editor: L. Huleatt)