Identification of a novel iron zinc finger protein 36 (ZFP36) for predicting the overall survival of osteosarcoma based on the Gene Expression Omnibus (GEO) database
Introduction
Osteosarcoma is a highly malignant primary tumor that originates from malignant mesenchymal cells (1), which has the characteristics of extensive tissue heterogeneity, high local invasiveness, rapid invasion and metastasis, and is more common in teenagers and children under 20 years old (2,3). The mortality rate of osteosarcoma is very high (4). The lesions are characterized by malignant spindle stromal cells producing bone-like tissues, which primarily occur in the metaphysis of long bones of limbs, most commonly in the distal femur region (5). Traditional treatment methods include surgical resection, radiotherapy, and chemotherapy, but the prognosis of patients has not improved significantly. At present, the 5-year survival rate of osteosarcoma patients in China is 37–77%. Although chemotherapy and surgical treatment can improve the 5-year survival rate of osteosarcoma patients by 60–70%, the 5-year survival rate of patients with tumor metastasis at the time of recurrence is less than 30% (6). For example, the average survival time of patients with lung metastasis is generally less than 1 year, and the survival rate is often less than 20% (7). Thus, improving the prognosis of osteosarcoma using markers that can positively and effectively predict the prognosis of osteosarcoma is crucial.
Ferroptosis is a new form of regulating cell death known as cell oxidative death, which is characterized by the production and accumulation of iron-dependent lipid reactive oxygen species (8). It has been reported that the interaction between ferroptosis and lipid metabolism plays an important role in tumor development, invasion, metastasis, drug resistance, and tumor immunity (9). In addition, among the various types of cancer cells with drug resistance, cancer cells with mesenchymal and dedifferentiated characteristics are more susceptible to ferroptosis (10,11). Recent studies have shown that overexpression of HMOX1 can increase the sensitivity of osteosarcoma cells to EF24. EF24, as a promoter of ferroptosis, can trigger ferroptosis of osteosarcoma cells by increasing the lipid peroxidation level, intracellular iron concentration, and reactive oxygen species (12). Lei et al. showed that the interaction between iron droop and immune system plays an important role in the occurrence and development of osteosarcoma, providing a new idea for the exploration of molecular mechanism and targeted therapy of osteosarcoma (13). Therefore, ferroptosis-related genes are expected to become new potential targets for osteosarcoma treatment.
Our research screened out differentially expressed genes (DEGs) related to osteosarcoma prognosis from the intersection of osteosarcoma chip data and ferroptosis-related gene datasets in the Gene Expression Omnibus (GEO) database. We then further discussed the effectiveness of the genes combined with the clinical data of osteosarcoma patients in our hospital, so as to provide more practical clinical reference significance for the timely screening patients with poor prognosis characteristics.
We present the following article in accordance with the TRIPOD reporting checklist (available at https://dx.doi.org/10.21037/atm-21-5086).
Methods
GEO data analysis
Two RNA expression datasets, GEO series 16088 (GSE16088) and GSE36001 (including tumor tissue and normal tissue), were downloaded from the GEO database (https://www.ncbi.nlm.nih.gov/geo/query/acc.cgi) using the GEOquery package, and the probes corresponding to multiple molecules were removed. When the probes corresponding to the same molecule were encountered, only probes with the largest signal values were kept.
Ferroptosis data analysis
The related ferroptosis dataset was downloaded from the ferroptosis database (http://www.zhounan.org/ferrdb), which contains 259 genes. The annotation of these genes revealed 108 driving genes, 69 suppressor genes, and 111 gene markers (14).
Selection of DEGs
DEGs between osteosarcoma and cancer-adjacent tissues were screened using limma package (3.42.2 version) in the GSE16088 and GSE36001 datasets. DEGs and ferroptosis-related genes were intersected to obtain DEGs related to ferroptosis. Next, based on the median expression quantity, ferroptosis-related DEGs were divided into high and low expression groups. Combined with their corresponding clinical survival data, survival analysis of ferroptosis-related DEGs was carried out in the TARGET database (https://ocg.cancer.gov/programs/target) using the Survival package, and ferroptosis-related genes related to prognosis were identified.
Gene Ontology (GO) and Kyoto Encyclopedia of Genes and Genomes (KEGG) enrichment analyses
Metascape (https://metascape.org/gp/index.html#/main/step1) was used for online functional analysis. Ferroptosis-related genes were added to Metascape for functional analysis and a protein-protein interaction (PPI) network diagram was constructed.
Clinical data
The clinical data of 60 osteosarcoma patients treated in Jiangyin Hospital Affiliated to Nanjing University of Chinese Medicine, Zhongda Hospital and Nanjing Drum Tower Hospital from January 2011 to January 2016 were selected. The inclusion criteria were as follows: (I) all patients were diagnosed as osteosarcoma for the first time and underwent surgery; (II) osteosarcoma was confirmed by histopathology after surgery; (III) patients with complete clinical and follow-up data; (IV) patients who had not undergone any other anti-tumor surgery before admission; and (V) patients with better compliance. The exclusion criteria were as follows: (I) patients with positive pathological resection margins after surgery; (II) patients complicated with other serious diseases, such as chronic obstructive pulmonary disease, heart failure, and severe diabetes; (III) patients who experienced serious complications during the perioperative period; and (IV) those who refused to follow up. In this study, 60 patients, aged 19–51 years, with an average age of (30.2±5.8) years, were included.
All procedures performed in this study involving human participants were in accordance with the Declaration of Helsinki (as revised in 2013). The study was approved by Jiangyin Hospital Affiliated to Nanjing University of Chinese Medicine (No. 2016010). Individual consent for this retrospective analysis was waived.
Tissue microarray construction and immunohistochemistry
The tissue specimens of 60 patients with osteosarcoma who were admitted into Jiangyin Hospital Affiliated to Nanjing University of Chinese Medicine, Zhongda Hospital and Nanjing Drum Tower Hospital from January 2011 to January 2016 were selected. The tissue microarray was constructed by the pathology department of these three hospitals. Sixty cases with osteosarcoma were stained with hematoxylin-eosin, and the most typical features were labeled at the fixed points under microscope. Each point array contained less than 160 points. Three μm-thick sections were cut from the receptor block and transferred to a glass slide using a tape transfer system for ultraviolet crosslinking. The ZFP36 antibody was purchased from Abgent (dilution, 1:100; Shanghai, China). Immunohistochemical results were scored based on the proportion of positive cells and the intensity of cell staining as follows: 0 points (negative), 1 point (≤25%), 2 points (25–50%), 3 points (51–75%), and 4 points (>75%), and the staining intensity was 0 (negative or no staining), 1 (weakly positive), 2 (moderately positive), and 3 (strongly positive). The value obtained by multiplying the two scores was the final score corresponding to each specimen. After calculating the arithmetic average of these scores, specimens with a score lower than 6 were finally defined as low ZFP36 expression.
Detection of mRNA encoding ZFP36 by reverse transcription quantitative polymerase chain reaction (qRT-PCR)
Intraoperatively, the tumor tissues and para-carcinoma tissues of patients were taken and frozen in liquid nitrogen tanks. One hundred mg of tumor tissues and normal tissues adjacent to the cancer were collected, which were milled into powder using the liquid nitrogen milling method, and then 1 mL Trizol lysis buffer (Shanghai Xitang Biotechnology Co., LTD) was added. Total RNA was extracted according to the manufacturer’s instructions. The following primers were used: 5'-AGT GAC AAA GTG ACT GCC CG-3' (285 bp, Tm 58 °C), 5'-GGG AGA GGG TTC ATT GCC TC-3' (19 bp, Tm 58 °C); and GAPDH was 5'-CAT GGG TGT GAA CCA TGA GAA GTA-3' (20 bp, Tm 60 °C), 5'-CAG TAG AGG CAG GGA TGA TGT TCT-3' (239 bp, Tm 60 °C) (15). The cDNA was obtained by reverse transcription of RNA using a reverse transcription kit (Shanghai Xitang Biotechnology Co., Ltd.), and real-time fluorescence quantitative PCR was performed on a fluorescence quantitative PCR instrument (Nanjing Ruiyuan Biotechnology Co., Ltd.). Finally, the relative mRNA expression of the target molecule was calculated using the 2−ΔΔCt method, and its expression situation in tumor tissues and para-carcinoma tissues was confirmed.
Follow-up
Follow-up was conducted every 3 months in the first 2 years, and every 6 months thereafter. Telephone follow-up was the main method, and outpatient appointments were conducted when necessary. The deadline for follow-up was January 2021. The observational index was as follows: overall survival (OS) was defined as the time from diagnosis of the disease to death from any cause or the end of follow-up; and progression-free survival (PFS) was defined as the progression of disease from the beginning of treatment to any follow-up project. At the end of follow-up, the survival data and loss of follow-up were entered into the statistical analysis as the final deadline.
Statistical methods
The software R (version 3.6.3) was used for statistical analysis and visualization. The GEOquery package (version 2.54.1) (16) was used for data download; the Limma package (version 3.42.2) (17) was used for variance analysis; the UMAP package (version 0.2.7.0) for was used for UMAP analysis; and the Ggplot 2 package (version 3.3.3) and ComplexHeatmap package (version 2.2.0) (18) were used to visualize the heat map.
The Chi-square test was used to compare and analyze the clinicopathological conditions in the two groups, and the t-test and multiple hypothesis test were used to analyze the quantitative data. The Kaplan-Meier method was used to evaluate the survival of patients, and the log rank statistical method was used to test the significance. The Cox proportional risk regression model was then used to identify the prognostic significance of the independent prognostic factors for osteosarcoma patients, and on this basis, a prediction model was subsequently constructed using R language to draw the line diagram. P<0.05 signified that the difference was statistically significant.
Results
Heat map of osteosarcoma-related genes in the GSE16088 and GSE36001 datasets
Through differential gene analysis, 5,005 maladjusted genes were obtained from the GEO: GSE16088 dataset, of which 2,719 genes were up-regulated and 2,286 genes were down-regulated, and 754 maladjusted genes were obtained from the GEO: GSE36001 dataset, of which 252 genes showed up-regulation and 502 genes showed down-regulation (Figure 1).
Selection of DEGs
The GSE16088 and GSE36001 datasets, and ferroptosis datasets were used to construct a Venn diagram for intersection, and DEGs were screened out. By showing the distribution of gene expression differences between normal tissues and tumor tissues by Volcano plot (Figure 2), it was found that ZFP36 is a down-regulated gene in the GSE16088 and GSE36001 datasets (Figure 3). Next, ferroptosis DEGs were divided into high and low expression groups. Combined with their corresponding clinical survival data, the survival analysis of ferroptosis DEGs was carried out in the TARGET database (https://ocg.cancer.gov/programs/target), and the Kaplan-Meier survival curve was drawn. We observed that only the different expressions of ATF4 and ZFP36 in TF, ASNS, PCK2, ATF4, and ZFP36 were related to the prognosis of osteosarcoma (Figure 4). Based on previous studies, it was then determined that ZFP36 has not been studied in osteosarcoma patients, and thus, we selected ZFP36 as the molecule to be studied.
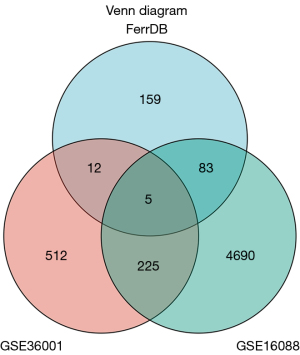
GO and KEGG enrichment analyses
Metascape was used for online functional analysis. The ferroptosis-related genes were added to Metascape for functional analysis, and a PPI network diagram was constructed. The first 20 most likely related signal pathways and the corresponding PPI network diagram were constructed (Figure 5).
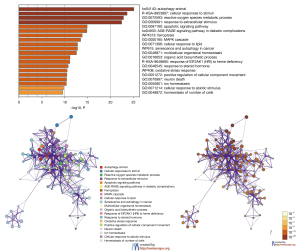
Expression of ZFP36 in immunohistochemistry
Immunohistochemical results indicated that ZFP36 was expressed in both tumor and para-carcinoma tissues of osteosarcoma, and the expression of ZFP36 in para-carcinoma tissues was higher than that in tumor tissues (Figure 6).
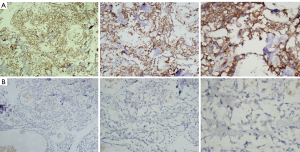
mRNA expression of ZFP36 in osteosarcoma
Taking tumor tissues and para-carcinoma tissues of patients as controls, the mRNA encoding ZFP36 was detected by reverse transcription quantitative PCR. It was also found that ZFP36 was expressed in both tumor tissues and para-carcinoma tissues of osteosarcoma, and the expression in para-carcinoma tissues was higher than that in tumor tissues (Figure 7).
Comparison of OS and PFS in high and low expression groups of ZFP36
In the final study, 60 patients were included, among which 23 patients died (mortality rate: 38.33%), and 37 patients survived (survival rate: 61.67%). The median PFS was 32.5 months, and the median OS was 77 months. The OS and PFS of the high ZFP36 expression group were significantly better than those of the low ZFP36 expression group (P<0.05) (Figure 8).
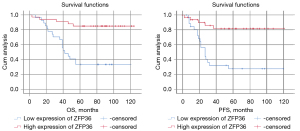
Relationship between high and low expression of ZFP36 and clinicopathological data
There were significant differences in age, tumor location, Enneking stage, and TSGF between the high and low ZFP36 expression groups (P<0.05) (Table 1).
Table 1
Variable | Total (n=60) | Low ZFP36 expression (n=27) | High ZFP36 expression (n=33) | P |
---|---|---|---|---|
Age (years old) | ||||
≤30 | 39 | 13 | 26 | 0.013* |
>30 | 21 | 14 | 7 | |
Gender | ||||
Male | 32 | 16 | 16 | 0.405 |
Female | 28 | 11 | 17 | |
Tumor size (cm) | ||||
≤8 | 29 | 10 | 19 | 0.113 |
>8 | 31 | 17 | 14 | |
Tumor site | ||||
Femur/tibia | 48 | 18 | 30 | 0.020* |
Other regions | 12 | 9 | 3 | |
Pathological fracture | ||||
No | 50 | 19 | 31 | 0.639 |
Yes | 10 | 8 | 2 | |
Distant metastasis | ||||
No | 56 | 24 | 32 | 0.212 |
Yes | 4 | 3 | 1 | |
ALP (IU/L) | ||||
Elevated | 17 | 11 | 6 | 0.054 |
Normal | 43 | 16 | 27 | |
Enneking staging | ||||
I–IIa | 37 | 12 | 25 | 0.013* |
IIb–III | 23 | 15 | 8 | |
TSGF (IU/mL) | ||||
Elevated | 25 | 16 | 9 | 0.012* |
Normal | 35 | 11 | 24 |
*, P<0.05, statistically significant difference. ALP, alkaline phosphatase; TSGF, tumor specific growth factor.
Single-factor analysis results
Univariate analysis showed that tumor location, pathological fracture, distant metastasis, alkaline phosphatase, and ZFP36 expression were the factors affecting OS (P<0.05) (Table 2).
Table 2
Variable | HR | 95% CI | P |
---|---|---|---|
Age (years) | |||
≤30 | 0.508 | 0.223–1.156 | 0.106 |
>30 | 1 | ||
Gender | |||
Male | 1.453 | 0.636–3.318 | 0.376 |
Female | 1 | ||
Tumor size (cm) | |||
≤8 | 0.581 | 0.246–1.374 | 0.216 |
>8 | 1 | ||
Tumor site | |||
Femur/tibia | 0.381 | 0.166–0.873 | 0.022* |
Other regions | 1 | ||
Pathological fracture | |||
No | 0.183 | 0.076–0.438 | 0.000* |
Yes | 1 | ||
Distant metastasis | |||
No | 0.235 | 0.077–0.718 | 0.011* |
Yes | 1 | ||
ALP (IU/L) | |||
Rise | 0.341 | 0.149–0.780 | 0.011* |
Normal | 1 | ||
Enneking by stages | |||
I–IIa | 0.479 | 0.209–1.098 | 0.082 |
IIb–III | 1 | ||
TSGF (IU/mL) | |||
Rise | 0.451 | 0.194–1.046 | 0.064 |
Normal | 1 | ||
ZFP36 expression | |||
Low expression | 6.197 | 2.286–16.798 | 0.000* |
High expression | 1 |
*, P<0.05, statistically significant difference. OS, overall survival; ALP, alkaline phosphatase; TSGF, tumor specific growth factor.
Multi-factor analysis results
Cox multivariate analysis showed that distant metastasis and ZFP36 were independent risk factors for tumor progression (P=0.021 and P=0.006, respectively) (Table 3).
Table 3
Variable | HR | 95% CI | P |
---|---|---|---|
Distant metastasis | |||
No | 2.968 | 1.182–7.453 | 0.021* |
Yes | 1 | ||
ZFP 36 expression | |||
Low expression | 0.226 | 0.078–0.655 | 0.006* |
High expression | 1 |
*, P<0.05, statistically significant difference. OS, overall survival.
Using R language to draw nomogram and build prediction model
In internal validation, the C-index of the nomogram was 0.7211 (95% CI: 0.6308–0.8115), and the prediction model had certain accuracy (Figure 9).
Discussion
Owing to the easy metastasis and high invasiveness of osteosarcoma, metastasis is detected at the first clinical visit in 10–20% of osteosarcoma patients (19). The most common metastatic site of osteosarcoma is the lung, and the recurrence rate of osteosarcoma patients with lung metastasis is as high as 80% (20,21), which seriously threatens their survival and prognosis (22). Therefore, for patients with osteosarcoma, especially after surgery, timely screening of those patients with adverse prognostic characteristics or development of targeted drugs with therapeutic significance is crucial.
Iron death is a newly discovered form of cell death, which mainly depends on iron-mediated oxidative damage and subsequent cell membrane damage, and is closely related to a variety of diseases, tumors, and injuries (23-25). In contrast to classical apoptosis, there is no cell shrinkage and chromatin agglutination in the process of iron death, but there will be mitochondrial shrinkage and increased lipid peroxidation. Traditional apoptosis, autophagy, and apoptosis inhibitors cannot inhibit the process of iron death, but iron ion chelators can inhibit this process, indicating that iron death is an iron ion-dependent process (26). In the process of tumorigenesis, iron death plays a dual role in promoting and inhibiting tumor progression. This depends on the release of damage-associated molecular patterns (DAMPS) in the tumor microenvironment and activation of the immune response induced by iron death injury. Therefore, iron death-related genes are expected to become a new potential target for the treatment of osteosarcoma.
Our study primarily selected two RNA expression datasets, GSE16088 and GSE36001, which contained osteosarcoma tumor tissues and normal tissues from the GEO database. We then took the intersection of these two datasets with the current iron death-related gene dataset to construct Wayne diagram in order to screen five iron death-related genes (TF, ASNs, pck2, ATF4, and ZFP36) in osteosarcoma genes. The survival package was subsequently used to analyze the survival of iron death DEGs in the target database. It was found that only the different expressions of ATF4 and ZFP36 were related to the prognosis of osteosarcoma. There have been numerous related studies on ATF4 in osteosarcoma, such as chemosensitivity (27), ubiquitination induced cell death (28), participating in endoplasmic reticulum stress to inhibit the growth of osteosarcoma (29), etc. However, there is no relevant study on ZFP36 in osteosarcoma. Therefore, we selected the ZFP36 molecule for further research. At the same time, we also found that ZFP36 is a down-regulated gene in the GSE16088 and GSE36001 datasets, so it may also be related to the inhibition of iron death.
We then used Metascape for GO and KEGG enrichment analyses, and obtained the top 20 most likely related signal pathways, including oxidative metabolism, apoptosis, iron death, organic anion transport, lipid metabolism, vascular endothelial growth factor A (VEGFRA)-VEGFR2, and organic homeostasis. Some studies have found that the presence of the RNA binding protein ZFP36 impairs epithelial mesenchymal transformation (EMT) and induces higher susceptibility of colon cancer to anoikis (30). Kröhler et al. (31) also found that the expression of ZFP36 was down-regulated in liver cancer tissues, which played an inhibitory role in the tumor by affecting liver lipid deposition and inflammation. Dong et al. (32) reported that ZFP36 can inhibit cell proliferation and increase cell death via an autophagy pathway in lung cancer cells. ZFP36 can also induce senescence of human papillomavirus-transformed cervical cancer cells by targeting E6-AP ubiquitin ligase (33). Therefore, through the enrichment analysis the results of GO and KEGG, combined with the existing research of ZFP36 in other tumors, we could further explore the specific mechanism.
Next, we detected the selected molecule ZFP36 by immunohistochemistry and PCR in osteosarcoma tissue samples. We found that ZFP36 was expressed in osteosarcoma tumor tissues and adjacent tissues, and the expression in adjacent tissues was higher than that in tumor tissues. Combined with the clinical data of 60 patients with osteosarcoma, the expression of ZFP36 was correlated with age, tumor site, Enneking stage and TSGF. Low expression of ZFP36 was more common in patients with age >30 years, lesions other than femur or tibia, Enneking stage IIb–III, and elevated TSGF. These results suggest that ZFP36 may be involved in the occurrence and development of osteosarcoma. On the other hand, it was found that OS and PFS in the high ZFP36 expression group were significantly better than those in the low ZFP36 expression group. This is consistent with the previous result that ZFP36 is down-regulated in the GSE16088 and GSE36001 datasets. In total, 23 of the 60 patients with osteosarcoma died, with a mortality rate of 38.33%. Also, a total of 37 patients survived, with a survival rate of 61.67%, a median PFS of 32.5 months, and a median OS of 77 months, which is consistent with the data of the current National Comprehensive Cancer Network (NCCN) treatment guidelines for osteosarcoma (34). Further Cox multivariate analysis showed that distant metastasis and ZFP36 were independent risk factors for tumor progression (P=0.021 and P=0.006, respectively). In the internal validation, the C-index of the nomogram was 0.7211 (95% CI: 0.6308–0.8115), and the prediction model we constructed has certain accuracy. Therefore, ZFP36 plays a certain role in predicting the prognosis of patients with osteosarcoma, providing a reference for clinical identification of ideal prognostic markers, and it is speculated that ZFP36 can be used as a new therapeutic target.
Conclusions
Although this study is a retrospective study of small samples, we screened the iron death-related gene ZFP36 of osteosarcoma by means of biological information analysis. At the same time, combined with the analysis of clinical data, such as immunohistochemistry, it was shown that ZFP36 could be used as a new predictive biomarker and a novel therapeutic target for osteosarcoma patients. In future, it is also necessary to conduct further multicenter, large sample prospective studies to clarify the exact mechanism of ZFP36 in osteosarcoma.
Acknowledgments
Funding: This work was supported by the National Natural Science Foundation of China (81871810 and 81702203), the Wuxi Health Committee Research Grants for Top Talent Support Program (2020), and the Nanjing University of Traditional Chinese Medicine Research Grant (XZR2020075).
Footnote
Reporting Checklist: The authors have completed the TRIPOD reporting checklist. Available at https://dx.doi.org/10.21037/atm-21-5086
Data Sharing Statement: Available at https://dx.doi.org/10.21037/atm-21-5086
Conflicts of Interest: All authors have completed the ICMJE uniform disclosure form (available at https://dx.doi.org/10.21037/atm-21-5086). The authors have no conflicts of interest to declare.
Ethical Statement: The authors are accountable for all aspects of the work in ensuring that questions related to the accuracy or integrity of any part of the work are appropriately investigated and resolved. All procedures performed in this study involving human participants were in accordance with the Declaration of Helsinki (as revised in 2013). The study was approved by Jiangyin Hospital Affiliated to Nanjing University of Chinese Medicine (No. 2016010). Individual consent for this retrospective analysis was waived.
Open Access Statement: This is an Open Access article distributed in accordance with the Creative Commons Attribution-NonCommercial-NoDerivs 4.0 International License (CC BY-NC-ND 4.0), which permits the non-commercial replication and distribution of the article with the strict proviso that no changes or edits are made and the original work is properly cited (including links to both the formal publication through the relevant DOI and the license). See: https://creativecommons.org/licenses/by-nc-nd/4.0/.
References
- Narhari P, Haseeb A, Lee S, et al. Spontaneous Conventional Osteosarcoma Transformation of a Chondroblastoma: A Case Report and Literature Review. Indian J Orthop 2018;52:87-90. [Crossref] [PubMed]
- Flores RJ, Li Y, Yu A, et al. A systems biology approach reveals common metastatic pathways in osteosarcoma. BMC Syst Biol 2012;6:50. [Crossref] [PubMed]
- Harrison DJ, Geller DS, Gill JD, et al. Current and future therapeutic approaches for osteosarcoma. Expert Rev Anticancer Ther 2018;18:39-50. [Crossref] [PubMed]
- Smrke A, Anderson PM, Gulia A, et al. Future Directions in the Treatment of Osteosarcoma. Cells 2021;10:172. [Crossref] [PubMed]
- Poos K, Smida J, Nathrath M, et al. Structuring osteosarcoma knowledge: an osteosarcoma-gene association database based on literature mining and manual annotation. Database (Oxford), 2014.
- Sayles LC, Breese MR, Koehne AL, et al. Genome-Informed Targeted Therapy for Osteosarcoma. Cancer Discov 2019;9:46-63. [PubMed]
- Wang JY, Yang Y, Ma Y, et al. Potential regulatory role of lncRNA-miRNA-mRNA axis in osteosarcoma. Biomed Pharmacother 2020;121:109627 [Crossref] [PubMed]
- Dixon SJ, Lemberg KM, Lamprecht MR, et al. Ferroptosis: an iron-dependent form of nonapoptotic cell death. Cell 2012;149:1060-72. [Crossref] [PubMed]
- Stockwell BR, Jiang X. The Chemistry and Biology of Ferroptosis. Cell Chem Biol 2020;27:365-75. [Crossref] [PubMed]
- Hangauer MJ, Viswanathan VS, Ryan MJ, et al. Drug-tolerant persister cancer cells are vulnerable to GPX4 inhibition. Nature 2017;551:247-50. [Crossref] [PubMed]
- Viswanathan VS, Ryan MJ, Dhruv HD, et al. Dependency of a therapy-resistant state of cancer cells on a lipid peroxidase pathway. Nature 2017;547:453-7. [Crossref] [PubMed]
- Lin H, Chen X, Zhang C, et al. EF24 induces ferroptosis in osteosarcoma cells through HMOX1. Biomed Pharmacother 2021;136:111202 [Crossref] [PubMed]
- Lei T, Qian H, Lei P, et al. Ferroptosis-related gene signature associates with immunity and predicts prognosis accurately in patients with osteosarcoma. Cancer Sci 2021; Epub ahead of print. [Crossref] [PubMed]
- Zhou N, Bao J. FerrDb: a manually curated resource for regulators and markers of ferroptosis and ferroptosis-disease associations. Database (Oxford) 2020.
- Davis S, Meltzer PS. GEOquery: a bridge between the Gene Expression Omnibus (GEO) and BioConductor. Bioinformatics 2007;23:1846-7. [Crossref] [PubMed]
- Zhu JG, Yuan DB, Chen WH, et al. Prognostic value of ZFP36 and SOCS3 expressions in human prostate cancer. Clin Transl Oncol 2016;18:782-91. [Crossref] [PubMed]
- Diboun I, Wernisch L, Orengo CA, et al. Microarray analysis after RNA amplification can detect pronounced differences in gene expression using limma. BMC Genomics 2006;7:252. [Crossref] [PubMed]
- Gu Z, Eils R, Schlesner M. Complex heatmaps reveal patterns and correlations in multidimensional genomic data. Bioinformatics 2016;32:2847-9. [Crossref] [PubMed]
- Fujiwara T, Oda M, Yoshida A, et al. Atypical manifestation of lung metastasis 17 years after initial diagnosis of low-grade central osteosarcoma. J Orthop Sci 2017;22:357-61. [Crossref] [PubMed]
- Khanna C. Novel targets with potential therapeutic applications in osteosarcoma. Curr Oncol Rep 2008;10:350-8. [Crossref] [PubMed]
- Wang C, Jing J, Cheng L. Emerging roles of non-coding RNAs in the pathogenesis, diagnosis and prognosis of osteosarcoma. Invest New Drugs 2018;36:1116-32. [Crossref] [PubMed]
- Mei J, Zhu XZ, Wang ZY, et al. Functional outcomes and quality of life in patients with osteosarcoma treated with amputation versus limb-salvage surgery: a systematic review and meta-analysis. Arch Orthop Trauma Surg 2014;134:1507-16. [Crossref] [PubMed]
- Louandre C, Ezzoukhry Z, Godin C, et al. Iron-dependent cell death of hepatocellular carcinoma cells exposed to sorafenib. Int J Cancer 2013;133:1732-42. [Crossref] [PubMed]
- Zhao B, Li X, Wang Y, et al. Iron-dependent cell death as executioner of cancer stem cells. J Exp Clin Cancer Res 2018;37:79. [Crossref] [PubMed]
- Kiessling MK, Klemke CD, Kaminski MM, et al. Inhibition of constitutively activated nuclear factor-kappaB induces reactive oxygen species- and iron-dependent cell death in cutaneous T-cell lymphoma. Cancer Res 2009;69:2365-74. [Crossref] [PubMed]
- Yang WS, Stockwell BR. Synthetic lethal screening identifies compounds activating iron-dependent, nonapoptotic cell death in oncogenic-RAS-harboring cancer cells. Chem Biol 2008;15:234-45. [Crossref] [PubMed]
- Luo J, Xia Y, Yin Y, et al. ATF4 destabilizes RET through nonclassical GRP78 inhibition to enhance chemosensitivity to bortezomib in human osteosarcoma. Theranostics 2019;9:6334-53. [Crossref] [PubMed]
- Luo J, Xia Y, Luo J, et al. GRP78 inhibition enhances ATF4-induced cell death by the deubiquitination and stabilization of CHOP in human osteosarcoma. Cancer Lett 2017;410:112-23. [Crossref] [PubMed]
- Zhao A, Zhang Z, Zhou Y, et al. β-Elemonic acid inhibits the growth of human Osteosarcoma through endoplasmic reticulum (ER) stress-mediated PERK/eIF2α/ATF4/CHOP activation and Wnt/β-catenin signal suppression. Phytomedicine 2020;69:153183 [Crossref] [PubMed]
- Montorsi L, Guizzetti F, Alecci C, et al. Loss of ZFP36 expression in colorectal cancer correlates to wnt/ ß-catenin activity and enhances epithelial-to-mesenchymal transition through upregulation of ZEB1, SOX9 and MACC1. Oncotarget 2016;7:59144-57. [Crossref] [PubMed]
- Kröhler T, Kessler SM, Hosseini K, et al. The mRNA-binding Protein TTP/ZFP36 in Hepatocarcinogenesis and Hepatocellular Carcinoma. Cancers (Basel) 2019;11:1754. [Crossref] [PubMed]
- Dong F, Li C, Wang P, et al. The RNA binding protein tristetraprolin down-regulates autophagy in lung adenocarcinoma cells. Exp Cell Res 2018;367:89-96. [Crossref] [PubMed]
- Sanduja S, Kaza V, Dixon DA. The mRNA decay factor tristetraprolin (TTP) induces senescence in human papillomavirus-transformed cervical cancer cells by targeting E6-AP ubiquitin ligase. Aging (Albany NY) 2009;1:803-17. [Crossref] [PubMed]
- Niu XH. Interpretation of 2020 NCCN Clinical Practice Guidelines in Oncology-Bone Cancer. Zhonghua Wai Ke Za Zhi 2020;58:430-4. [PubMed]
(English Language Editor: A. Kassem)