Analysis of the reasons for screening failure in phase I clinical trials in China: a retrospective study of the clinical trials screening process
Introduction
Phase I clinical trials represent the first stage of drug testing in humans. Their primary purpose is to evaluate the safety, tolerability, and pharmacokinetic parameters of a drug for human use. The progress and completion of phase I clinical trials directly affect the recommended phase II dose (RP2D) and can even affect new drug marketing. All trial stakeholders are actively developing better solutions to improve the efficiency of clinical trials and reduce the risk of failure (1). A large-scale survey showed that in the United States, the overall probability of success of a drug from the time of entering phase I clinical trials to obtaining Food and Drug Administration (FDA) approval was only 13.8%; that is, nearly 90% of drugs entering the clinical trial stage do not ultimately obtain regulatory approval (2).
With the introduction of favorable policies, such as encouraging drug innovation in China, the research and development of innovative drugs has entered a strategic period. Considering China’s large population and drug consumption market, multinational pharmaceutical companies have focused their attention on China, rendering it one of the world’s most important clinical trial bases. However, the accumulation of many innovative drugs and generic drugs on the market puts tremendous pressure on the approval process, the institutions conducting the trials, and auxiliary organizations (e.g., CRO, SMO) (3,4). Between 2009 and 2018, the China National Medical Products Administration (NMPA) published a series of regulatory reform measures to overcome delays in drug approval, encourage innovation, and strengthen the standardization of drug research and development (5). From the perspective of the number of clinical trials carried out in China each year, the average annual growth rate during that period was approximately 33%, with the number increasing sharply in 2016, reaching 113% of that in 2015. This increase was due to the substantial increase in bioequivalence trials in China following the official start of consistency evaluations of generic drugs in 2016. Among the types of trials, early-stage exploratory phase I trials accounted for the largest percentage, with an annual growth rate of approximately 15%, followed by phase III and phase II trials (although these had 10% and 6% annual decreases, respectively), with the proportions of bioequivalence trials and phase IV trials being the lowest (5,6). The US FDA has approved a total of 378 innovative drugs and 27 biosimilar drugs in the past 10 years [2010–2019]. The development of novel drugs also reflects a future in which drug development companies and institutions will meet new challenges and continue to provide patients with innovative, urgently needed drugs (7,8).
The coronavirus disease 2019 (COVID-19) pandemic has brought clinical trials to a standstill in most countries. The Medidata database was used to investigate and analyze 4,600 clinical trials with an “active status” at 182,227 research sites worldwide. The results showed that only China, South Korea, and Italy experienced less slowdown in the number of subjects enrolled in trials, while the number of subjects enrolled in trials in other countries declined dramatically; for example, in India and the United Kingdom the reduction was as high as 96% and 98%, respectively (9).
In the context of numerous ongoing or upcoming phase I clinical trials and the COVID-19 pandemic, improving the number and quality of recruited subjects (10), eliminating screening problems, and promoting the normal progress of trials will be major challenges for trial institutions. However, for some institutions and researchers, the development of specific standards in the clinical trial process seems to be arbitrary or is inherited from the inclusion and exclusion criteria of other trials. The more stringent protocol standards narrow the scope of healthy subjects included in phase I clinical trials, resulting in ineffective recruitment and seriously affecting overall clinical trial schedules and resource consumption (1,2,11). In the literature analyzing the reasons for the screening failure of many subjects in clinical trials, most reports are related to phase I clinical trials involving cancer patients, and studies on the screening of healthy subjects in phase I clinical trials are scarce. We used historical data which based on the 12 clinical trials completed by our center involving healthy subjects with similar inclusion and exclusion criteria, and the screening records of 1,640 healthy subjects. We found that 1008 healthy subjects failed in screening, with a failure rate of up to 61.5%. Abnormal laboratory test results (43.25%), abnormal vital sign examination results (11.81%), withdrawal of informed consent (10.02%), abnormal height/weight examination results (8.33%), and abnormal electrocardiogram (ECG) examination results (5.66%) accounted for 79.07% of the screening failures. The remaining 20.93% of screening failures were attributed to the subject-screening network database, abnormal consultation results, multiple reasons, abnormal imaging examination results, concomitant medication, abnormal smoking history results, principal investigator decisions, abnormal physical examination results, abnormal drug urinalysis results, and intervening medical issues. Facing the problem of screening failure, we need to analyze the main reasons for screening failure in the screening process of healthy subjects in phase I clinical trials and seek coping strategies, so as to improve the success rate of recruitment. Firstly, we find out the main causes of screening failure through Pareto Diagram. Secondly, the causal diagram (fishbone diagram) - 4M1E analysis method is used to find out the influencing factors leading to the failure of screening. Finally, formulate targeted coping strategies. These strategies have been used in recruitment and have good practical application value.
We present the following article in accordance with the MDAR reporting checklist (available at https://dx.doi.org/10.21037/atm-21-5010).
Methods
Data sources
The screening records of 1,640 healthy subjects in phase I clinical trials at the First Affiliated Hospital of Bengbu Medical College (public hospital) were collected between April 2019 and July 2020.
Study methods
Project inclusion criteria
This study examined all phase I clinical trial projects conducted at our institution and developed the following inclusion and exclusion criteria: (I) Phase I clinical trials that enrolled only healthy subjects were included, and phase I clinical trials that enrolled patients or phase II, III, or IV clinical trials were excluded; (II) clinical trials with no special restrictions on sex were included; otherwise, the trials were excluded; (III) clinical trials with no special restrictions regarding the sex ratio of the successfully enrolled group were included; otherwise, the trials were excluded; (IV) clinical trials with no special restrictions on age were included, and trials with special restrictions (such as middle-aged and older people) were excluded; and (V) clinical trials with no special restrictions on study drugs were included, and trials with special restrictions (such as radiopharmaceuticals) were excluded.
Protocol inclusion criteria
Inclusion criteria
The inclusion criteria were as follows: (I) male or female subjects aged 18 years or older; (II) a body mass index [(BMI) = body weight (kg)/height2 (m2)] within the range of 19–26 kg/m2, with male and female subjects weighing no less than 50 and 45 kg, respectively; (III) normal physical examination, vital signs, or abnormal results without clinical significance; (IV) subjects (including male subjects) who reported no plans to become pregnant in the next 6 months and were voluntarily using effective non-pharmacological contraception; and (V) subjects who voluntarily participated and signed an informed consent form (ICF), with the informed consent process conducted in compliance with the Good Clinical Practice (GCP) guidelines.
Exclusion criteria
The exclusion criteria were as follows: (I) people with an allergic constitution (such as those allergic to two or more drugs and food) and people with allergic symptoms such as bronchial asthma, rash, and urticaria; (II) people with severe renal dysfunction; (III) people with dysphagia or with a history of gastrointestinal disease that could affect drug absorption; (IV) people who had taken any prescription drugs, non-prescription drugs, vitamin products, or herbal medicines within 28 days before the test, especially any drugs that changed liver enzyme activity; (V) people who had a special diet and/or exercise factors that could affect drug absorption, distribution, metabolism, and excretion during the test, including but not limited to eating special foods (including dragon fruit, mango, grapefruit, chocolate, and/or a diet containing caffeine or xanthine) within 48 hours before the test that could affect drug metabolism; (VI) people with positive hepatitis B surface antigen (HBSAG), hepatitis C virus antibody (HVC-Ab), human immunodeficiency virus (HIV) antibody, or treponema pallidum antibody (TPAB) test results; (VII) people with positive pregnancy test results; (VIII) people with abnormal physical examinations, vital signs, complete blood count (CBC), routine urine test, blood biochemistry, coagulation function test, and ECG examination results; (IX) people who had a history of drug abuse within the past 5 years, had used drugs within 3 months before the test, or had a positive urine drug screening result; (X) pregnant or breastfeeding women; (XI) people who had participated in other clinical trials within the past 3 months or had lost more than 400 mL of blood within the last 3 months (such as blood donation but excluding menstrual blood loss); (XII) special lifestyles: people who smoked regularly (≥5 cigarettes/day) and those who could not abstain from smoking during the trial; a history of alcohol abuse (drinking 14 units of alcohol per week: 1 unit =25 mL of spirits with more than 40% alcohol, 85 mL of wine, or 285 mL of beer); previous long-term consumption of any food or beverage containing caffeine or xanthine (e.g., coffee, tea, cocoa-based beverages, caffeine-containing soda, and chocolate, alone or as an ingredient; more than 8 cups per day, 1 cup =250 mL); (XIII) people who could not comply with the study protocol; (XIV) people who could not tolerate venipuncture or had difficulty during blood collection; and (XV) other factors, such as people considered unsuitable by the investigators.
Screening process
The screening process for day 1 (D1) was as follows: (I) each subject’s identification (ID) information was verified, followed by the subject’s registration; (II) information was publicly provided to groups of potential subjects—the involved physician explained the entire trial process so that subjects could fully understand the requirements and consider whether to participate in the trial; (III) the subjects and study physicians signed ICFs individually; (IV) the external network (subject-screening database) was consulted to determine whether the subject had been involved in another clinical trial within the past 3 months; (V) demographic data were collected; (VI) height, weight, and vital sign measurements, consultations, and the physical examination screening were performed; and (VII) subjects who failed the screening were informed of relevant precautions and were signed out.
The screening process for day 2 (D2) was as follows: (I) qualified subjects who passed the screening on D1 checked in and underwent laboratory tests and ECG examinations; (II) imaging examinations were completed, and subjects signed out after completing the relevant examinations; (III) after the physician evaluated the relevant examination results, subjects were assessed for qualification; (IV) concomitant medications were documented; (V) the list of qualified subjects was confirmed; and (VI) qualified subjects were informed by telephone follow-up.
Data collection and processing
Data collection
The data collected included screening number, sex, age, ethnicity, location of residence, occupation, marital status, whether screening was successful, reason for screening failure, screening date, screening failure date, and sample types.
The following 14 categories were used to classify screening failure: (I) abnormal external network screening results (including ID verification and duplicates); (II) abnormal vital sign results (including body temperature, blood pressure, breathing, and pulse); (III) abnormal smoking history results; (IV) abnormal drug urinalysis results; (V) abnormal consultation results (including current disease history, previous disease history, family disease history, previous treatment regimen, history of food/drug allergy, history of smoking and alcohol, history of blood donation, menstrual history, special dietary requirements, and unprotected sexual behavior); (VI) concomitant medication; (VII) abnormal physical examination results; (VIII) abnormal laboratory test results (including blood biochemistry, complete blood count, routine urine, immune screening, blood pregnancy test, and coagulation function test); (IX) abnormal ECG examination results; (X) abnormal imaging examination results; (XI) withdrawal of informed consent; (XII) abnormal height/weight examination results (including BMI and body mass); (XIII) principal investigator (PI) decisions (including compliance and blood collection); and (XIV) intervening medical issues. Considering the potential crossover/duplication of reasons for screening failure, we added the following category: multiple reasons. All procedures performed in this study involving human participants were in accordance with the Declaration of Helsinki (as revised in 2013). The study was approved by institutional ethics board of the First Affiliated Hospital of Bengbu Medical College (No. 2021KY035) and informed consent was taken from all the patients.
Data processing
We summarized the collected data and converted them into an appropriate format. In particular, we converted “residence location” into longitude and latitude. We used the longitude and latitude of the clinical trial institution as the reference point to calculate the distance from other prefecture-level cities to the clinical trial institution using two methods (the Python library’s geopy using third-party geo-coders and the distance calculation formula). The difference in the distances generated by the two methods was calculated. To reduce the error, the average value of the straight-line distance obtained by the two methods was used; the error was approximately 5 km or less, as determined by distance measurements using a map of Baidu. “Screening number”, “age”, and “location of residence” after conversion were all raw integer data, while “sex”, “ethnicity” (Han or not), “marital status”, “successful screening” (yes or no), and “sample types” (blood sample, urine sample) were all the original binary data; “reason for screening failure” and “occupation” were first used to determine the number of categories and then underwent binary conversion into binary data.
Statistical analysis
Using the converted information, we calculated the influencing factors related to the screening failure rate. This specific method was used to reflect the proportions for categorical data (discrete data) and conduct controlled studies between subjects who failed screening and those who passed screening (continuous data). The reasons for screening failure were statistically analyzed (χ2 test), and correlation studies were conducted to explore the main factors associated with screening failure.
Results
Demographic characteristics
A total of 12 projects that used healthy subjects in phase I clinical trials met the criteria. Screening for the first project started in April 2019 and screening for the 12th project ended in July 2020. Ultimately, data from 1,640 healthy subjects were collected; the characteristics of the subjects are summarized in Table 1. Among them, 632 (38.5%) subjects passed screening, and 1,008 (61.5%) failed screening. In terms of the sex ratio, 1,133 males (69.1%) and 507 females (30.9%) were included, and among these subjects, 721 males (71.5%) and 287 females (28.5%) failed screening. In terms of ethnicity, a total of eight ethnic groups were noted: Hui [6], Tibetan [4], Manchu [3], Korean [1], Dai [1], Lahu [1], and Uygur [1]; the remainder were Han.
Table 1
Characteristics | Screening (n=1,640) | P value | |||||
---|---|---|---|---|---|---|---|
Failure, n (%) | Success, n (%) | ||||||
Number of people | 1,008 (61.50) | 632 (38.50) | – | ||||
Sex | 0.007* | ||||||
Male | 721 (63.64) | 412 (36.36) | |||||
Female | 287 (56.61) | 220 (43.39) | |||||
Age (years) | 0.002* | ||||||
18–30 | 541 (60.58) | 352 (39.42) | |||||
31–45 | 309 (58.63) | 218 (41.37) | |||||
46–571 | 158 (71.82) | 62 (28.18) | |||||
Ethnicity | 0.822 | ||||||
Han | 998 (61.49) | 625 (38.51) | |||||
Minorities | 10 (58.82) | 7 (41.18) | |||||
Occupation | 0.1693 | ||||||
Worker | 204 (59.65) | 138 (40.35) | |||||
National civil servant | 3 (60.00) | 2 (40.00) | |||||
Farmer | 27 (60.00) | 18 (40.00) | |||||
Student | 132 (56.90) | 100 (43.10) | |||||
Professional and technical personnel | 23 (69.70) | 10 (30.30) | |||||
Freelancer | 230 (57.64) | 169 (42.36) | |||||
Unemployed | 23 (82.14) | 5 (17.86) | |||||
Other | 319 (62.67) | 190 (37.33) | |||||
Missing2 | 47 | 0 | |||||
Distance between residence location and central institution (km) | 0.491 | ||||||
<100 | 345 (64.13) | 193 (35.87) | |||||
100–200 | 294 (58.57) | 208 (41.43) | |||||
200–500 | 239 (61.60) | 149 (38.40) | |||||
500–1,000 | 67 (60.91) | 43 (39.09) | |||||
>1,000 | 63 (61.76) | 39 (38.24) |
1, the oldest recorded age in this study was 57 years; 2, missing indicates people who failed external network screening and voluntarily withdrew (withdrawal of informed consent) or did not provide occupational information; 3, excluding missing occupation information, this signifies whether a significant correlation exists between occupation and screening failure. *, the application of chi-square test, with P value <0.05 as the reference standard.
The characteristics of subjects who failed the screening were compared. Male subjects were more likely to fail screening than female subjects (721/1,133 vs. 287/507, respectively, P=0.007), and subjects aged 46–57 years were more likely to fail screening than those aged 18–30 or 31–45 years (158/220 vs. 541/893 vs. 309/527, respectively, P=0.002). No significant correlation was found between the distance from the subject’s residence to the clinical trial institution and screening failure (P=0.491) (Table 1).
Based on age as continuous data, the youngest age was 18 years, the oldest age was 57 years, and the average age was 31.59±9.88 years. A trend analysis was performed based on the association between age and the screening failure rate (Figure 1). No trend correlation was found between those aged 18–45 years and the screening failure rate, but a fluctuation in a bounding box indicated a positive correlation between those aged over 45 years and the screening failure rate. Based on the age distribution and the number of subjects participating in the screening (Figure 2), subjects aged 18–35 years constituted the main population in phase I clinical trials.
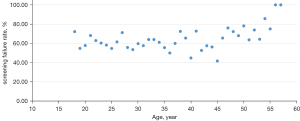
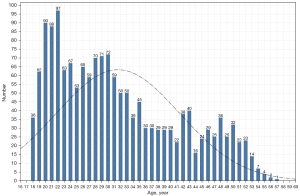
Based on “residence location”, we correlated the number of screened subjects with their provinces and cities and depicted the population distribution of screened subjects using a heat map (Figure 3). The results showed that the distribution of the subjects in the provinces adjacent to the central institution was relatively dense and that the distribution of the subjects in distant provinces was relatively sparse.
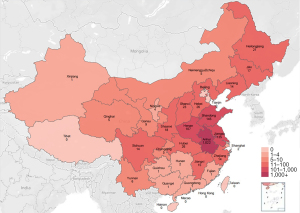
The primary causes of screening failure
We classified and summarized the reasons for screening failure and found that the most common reasons for screening failure were laboratory test results, vital sign examination results, withdrawal of informed consent, height/weight examination results, and ECG examination results (Figure 4). Notably, the first five screening failure reasons accounted for 79.07% of the total screening failures, while screening failure reasons such as external network screening, consultation results, multiple reasons, imaging examination results, concomitant medication, smoking history results, PI decisions, physical examination results, drug urinalysis results, and intervening medical issues accounted for only 20.93% of the total screening failures.
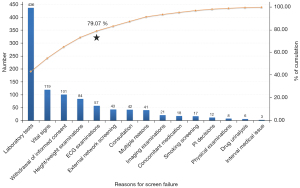
Abnormal laboratory test results were the most important factors, accounting for 43.25% of screening failures. Among subjects with abnormal laboratory test results, 391 (75.8%) had abnormal blood indicators, and 125 (24.2%) had abnormal urine indicators. The top five abnormal blood indicators were uric acid, direct bilirubin, hepatitis B core antibody, total bilirubin, and white blood cell (WBC). The top five abnormal urine indicators were urinary WBC count, urine occult blood, urinary red blood cells (RBCs), urinary protein, and bacteria in the urine sediment (Table 2). Vital signs included blood pressure, pulse, respiration, and body temperature. The reasons for vital sign screening failure are summarized in Table 3. Height/weight examinations included BMI and body mass. The reasons for height/weight screening failures are summarized in Table 4. Regarding ECG examination results, early repolarization syndrome, incomplete right bundle branch block, first-degree atrioventricular block, and T wave changes were the most common indicators of ECG abnormalities.
Table 2
Blood samples (n=391) | Urine samples (n=125) | |||||
---|---|---|---|---|---|---|
Item1 | Case2 | % of total (total =636) | Item1 | Case2 | % of total (total =184) | |
Uric acid | 68 | 10.69 | Urinary WBC | 58 | 31.52 | |
Direct bilirubin | 66 | 10.38 | Urine occult blood | 34 | 18.48 | |
Hepatitis B core antibody | 52 | 8.18 | Urinary RBC | 31 | 16.85 | |
Total bilirubin | 51 | 8.02 | Urinary protein | 28 | 15.22 | |
WBC count | 48 | 7.55 | Bacteria in urine sediment | 9 | 4.89 | |
Triglycerides | 34 | 5.35 | Urinary bilirubin | 6 | 3.26 | |
Indirect bilirubin | 33 | 5.19 | Urinary mucus | 2 | 1.09 | |
Hepatitis B antibody | 24 | 3.77 | Urinary glucose | 2 | 1.09 | |
Alanine aminotransferase | 22 | 3.46 | Urinary ketones | 2 | 1.09 | |
Neutrophil count | 22 | 3.46 | Nitrite | 2 | 1.09 |
1, only the top 10 blood/urine laboratory indicator abnormalities are shown; 2, abnormalities may overlap or be duplicated. WBC, white blood cell.
Table 3
Vital sign | Number of screening failures | Percentage of total screening failures (%) | Percentage of vital sign examinations (%) |
---|---|---|---|
Blood pressure | 97 | 9.62 | 81.51 |
Pulse | 19 | 1.88 | 15.97 |
Body temperature | 3 | 0.30 | 2.52 |
Respiration | 0 | 0.00 | 0.00 |
Total | 119 | 11.81 | 100.00 |
Table 4
Height/weight | Number of screening failures | Percentage of total screening failures (%) | Percentage of height/weight examinations (%) |
---|---|---|---|
BMI | 75 | 7.44 | 89.29 |
Body mass | 9 | 0.89 | 10.71 |
Total | 84 | 8.33 | 100.00 |
BMI, body mass index.
Combined screening failure refers to the crossover or duplication of reasons for screening failure, including abnormalities in laboratory test results and ECG examination results; abnormalities in laboratory test results and imaging examination results; abnormalities in laboratory test results, ECG examination results, and imaging examination results; and concomitant medication and abnormal consultation results. The reasons for combined screening failure are provided in Table 5.
Table 5
Multiple reasons for screening failure | Case | Percentage of total screening failures (%) | Percentage of combined screening failures (%) |
---|---|---|---|
Abnormal laboratory test and ECG examination results (n=30) | |||
Abnormal blood biochemistry and ECG examination results | 14 | 1.39 | 34.15 |
Abnormal CBC and ECG examination results | 5 | 0.50 | 12.19 |
Abnormal routine urine and ECG examination results | 5 | 0.50 | 12.19 |
Abnormal coagulation function and ECG examination results | 1 | 0.10 | 2.44 |
Abnormal blood pregnancy test and ECG examination results | 1 | 0.10 | 2.44 |
Abnormal blood biochemistry, immune screening, and ECG examination results | 1 | 0.10 | 2.44 |
Abnormal blood biochemistry, routine urine, and ECG examination results | 1 | 0.10 | 2.44 |
Abnormal blood biochemistry, CBC, routine urine, and ECG examination results | 1 | 0.10 | 2.44 |
Abnormal CBC, immune screening, routine urine, and ECG examination results | 1 | 0.10 | 2.44 |
Abnormal laboratory test and imaging examination results (n=8) | |||
Abnormal blood biochemistry and B-ultrasound examination results | 5 | 0.50 | 12.19 |
Abnormal CBC and B-ultrasound examination results | 3 | 0.30 | 7.32 |
Abnormal laboratory test, ECG examination, and imaging examination results (n=1) | |||
Abnormal blood biochemistry, color Doppler echocardiography, and ECG examination results | 1 | 0.10 | 2.44 |
Concomitant medication and abnormal consultation (n=2) | |||
Concomitant medication, smoking, and alcohol history | 1 | 0.10 | 2.44 |
Concomitant medication, special food requirements, and previous disease history | 1 | 0.10 | 2.44 |
Total | 41 | 4.07 | 100.00 |
Discussion
During the screening process for phase I clinical trials, the screening failure rate directly affects the progress of trials and resource allocation for trials. Efficient screening can ensure the smooth progression of phase I clinical trials. In China, many healthy subjects are eligible to participate in phase I clinical trials, and highly selective protocols can be applied in the screening process. Therefore, protocol development involves demanding requirements and high screening failure rates. Screening failure is a serious problem that must be properly addressed by various clinical trial institutions. For all clinical trials, researchers try to find ways to address the issue of screening failure.
Meanwhile, drug type is indeed one of the factors affecting the screening of phase I clinical trials, because different drug types may have different inclusion and exclusion criteria, but for healthy subjects, their health level should be consistent or can reach a certain health level. In other words, there should be a minimum standard for the health level of healthy subjects in the screening stage of clinical trials. One of our research contents is to try to determine the reasons for the screening failure of healthy subjects in phase I clinical trials on the premise of the minimum standard. If a drug trial has strict or special inclusion and exclusion criteria, the trial may be selected from the group of healthy subjects who meet the minimum standard.
Challenges of screening criteria for clinical trials
This study found that abnormal laboratory indicators were the main factor leading to healthy subject screening failure, which is consistent with the results reported by Mckane et al., Tiu et al., and Park et al. (11-13). However, the most common abnormal laboratory indicator was increased serum uric acid, which can have many causes but is primarily caused by purine metabolism disorders. Serum uric acid is generally believed to be affected by region, ethnicity, age, body weight, dietary habits, and genetic factors. The main reason for increased serum uric acid in healthy people is poor dietary habits, such as the consumption of beer, carbonated beverages, barbecued food, and seafood, which are favorite foods among young people. Short-term diets including these types of food have been shown to cause an increase in serum uric acid (male >420 µmol/L or female >350 µmol/L) (14). If a diet-related transient increase in serum uric acid is suspected, reassessment of serum uric acid levels on different days at the time of screening may reduce the screening failure rate. Additionally, in the urine laboratory test data, we found that an increased urinary WBC count was the most important reason for screening failure. If the subject has no urinary tract infection symptoms, such as frequent urination, urgent urination, or dysuria, and no laboratory indicator abnormalities suggestive of acute infection, such as increased WBCs in the blood or increased C-reactive protein (CRP), high urinary WBCs may be caused by contamination of the urine by the external genital area during the process of urine specimen collection. For example, first-pass urine is more likely to be contaminated by residual bacteria in the foreskin or perineum, resulting in a high number of urinary WBCs. If the protocol allows cleaning of the external genitalia or perineum, repeating the routine urine test may reduce the screening failure rate. In addition, in a study with healthy elderly subjects in South Korea, the reference values for some exclusion criteria were slightly modified by 10–20%, and the final results indicated nonsignificant changes (13). Therefore, in addition to strictly complying with the inclusion/exclusion criteria of the trial protocol, subjects with indicator levels beyond the normal reference ranges can be included in trials in the absence of clinical significance when the clinician determines the MDL of each indicator. Limiting results to reference ranges is not necessary (1,2).
The data in this study showed that most ECG examination abnormalities were early repolarization syndrome, incomplete right bundle branch block, first-degree atrioventricular block, and T wave changes, which can be caused by electrophysiological phenomena, cardiovascular drug effects, insufficient blood supply to the heart, fatigue, emotional changes, and circadian changes (15) and can be either pathological or physiological in nature; when these abnormalities are encountered in healthy people without any clinical symptoms, no treatment is needed. To avoid excluding potentially suitable subjects, clinical diagnosis physicians should conduct comprehensive clinical assessments based on the subject’s previous disease history, family history, medication history, clinical manifestations, and retesting. Therefore, the inclusion criteria of some trials may be too strict in terms of ECG examination results. However, with the current lack of sufficient evidence, expanding the inclusion criteria of clinical trials is very challenging. Based on existing data alone, the parties involved in trials cannot reach a consensus on the abolition of specific criteria or the expansion of inclusion criteria (2).
The reasons for screening failure—vital signs
In addition, abnormal vital signs, primarily abnormal blood pressure, were found to be a secondary factor leading to healthy subject screening failure. Reviewing the screening data of relevant subjects, all subjects denied a personal or family history of hypertension. The possible influencing factors of abnormal blood pressure include travel fatigue, poor work and rest habits, occupation-related (night shift) fatigue, a suboptimal health status, or manifestations of prehypertension (16). Additionally, the possible influencing factors of abnormal pulse include mental stress factors and exercise in nonprofessional athletes. Therefore, regarding recruitment information (approved by the ethics committee), trial institutions are obliged to remind subjects to maintain a good schedule and a relaxed attitude before participating in the screening examination, which will help to improve the screening success rate.
The reasons for screening failure—withdrawal of informed consent
Withdrawal of informed consent is also one of the most common screening failure factors. Through face-to-face interviews with subjects, we learned that the factors influencing withdrawal of informed consent include the purpose of the trial, the trial process and timing, the nature of the drug, possible risks, possible discomfort during the trial, rights and obligations, and financial compensation not being fully disclosed; some subjects participate in trials only for free health examinations; and some are prevented from participating by intervention from family members or friends. For subjects who participate in clinical trials for the first time, the possibility of withdrawal is very high (17,18). In 2013, an online survey of 5,701 respondents conducted by the Center for Information and Study on Clinical Research Participation (CISCRP) showed that 69% of the respondents from the Asia-Pacific region believed that ICFs were difficult to understand, while only 12% of the respondents from North America considered ICFs to be difficult to understand. A simulation study evaluating consent decisions in India showed that patients generally had a poor understanding of the ICF and only 30% provided consent (19). Therefore, adequate notification is an indispensable and important step, and subjects should be given sufficient time to consider and discuss their participation in trials with their family members. Additionally, investigators need to objectively inform potential subjects of the possible risks, rights, and obligations of the clinical trial and provide information regarding the primary function and requirements of investigational drugs and follow-up requirements, thereby reducing the occurrence of the withdrawal of informed consent.
Influencing factors of screening failure
Sex differences are also one of the factors that affect the success of screening (20,21). The results showed that sex was significantly correlated with screening failure. The screening failure rate for males was higher than that for females. For males, a poor lifestyle (smoking and drinking) is one reason leading to the high screening failure rate. For women, normal physiological changes associated with menstruation, lactation, and reproduction status will lead to screening failure (21). In phase I clinical trials, we found that sponsors (not the trial protocol) deliberately requested the recruitment of more male subjects than female subjects. The general male to female ratio was 2:1, which surprised us. Under the premise that study protocols have no special requirements regarding sex, project sponsors should not have an explicit sex orientation and increasing the number of female subjects may help improve the healthy subject screening success rate.
A study conducted by Xuan Wu Hospital Affiliated with Capital Medical University showed that the screening failure rate for subjects was positively correlated with age (22). However, in our study on the causes of screening failure in healthy subjects in phase I clinical trials, we found that being aged between 18 and 45 years was not correlated with the screening failure rate, but the rate fluctuated among other age groups; being aged 45 years or older was positively associated with a higher screening failure rate. The possible reason for this result is that subjects aged between 18 and 30 years (screening failure rate: 60.58%) may not have fully understood the phase I clinical trial requirements, or relatives prevented their participation, resulting in a large number of withdrawals (23). Additionally, their poor lifestyle habits (sleep, diet) may have led to higher screening failure rates. Subjects between the ages of 31 and 45 years (screening failure rate, 58.63%) may have been affected by work or life stress, resulting in abnormal physiological indicators, suboptimal health status, or poor lifestyle habits (smoking and drinking), which in turn led to an increased screening failure rate (24,25). In our study, the comparison of the screening failure rates between the two age groups (60.58% and 58.63%) showed no significant difference in screening failure rates between subjects aged 18–30 years and those aged 31–45 years. However, the screening failure rate for subjects >45 years old (screening failure rate 71.82%) gradually increased with age, a finding that may be closely related to physiological changes and willingness to participate.
This study also performed a statistical analysis of the distance between the subject’s residence and the clinical trial institution. The distance was divided into five categories, based on the scope of the jurisdiction (e.g., county, city, or province) <100, 100–200, 200–500, 500–1,000, and >1,000 km. The results showed that the distance from the place of residence to the clinical trial institution (P=0.491) was not significantly correlated with screening failure. This finding is different from the results of a study conducted by a nonprofit organization in Arizona in 2013, which reported that subjects who lived farther away were more likely to fail screenings because of the potential burden of long-distance travel (11). We believe that an important factor leading to this conclusion is the difference in the subject population: the impact of distance on healthy subjects is not significant, but patients (especially cancer patients) may not be able to afford long-distance travel. Therefore, institutions should be obliged to remind subjects who travel long distances to get adequate rest, eat a healthy diet, and relax their minds to ensure trial screening success.
Finally, we believe that the suggestions to quickly complete the screening of healthy subjects are as follows: (I) objectively and reasonably formulate the entry and exclusion criteria of the project; (II) fully inform the subjects of the relevant rights and interests of participating in the clinical trial; (III) inform the subjects that they should have a light diet and maintain sufficient rest and relaxation before screening the clinical trial; (IV) give reasonable suggestions in terms of age and gender.
Conclusions
Our data were retrospectively collected from a single institution. Therefore, caution should be used when extending the results to other institutions. Although the universality of the current study is not wholly satisfactory, the data on the screening failure of phase I healthy subjects provide us with various factors that may play roles in future recruitment and screening. We cannot entirely eliminate the screening problem, but any strategy to reduce the screening failure rate is worthwhile (26), allowing researchers to not only control the time and costs but also continue to be motivated to carry out experiments. Therefore, strategies to reduce screening failure will benefit the subjects, researchers, and the entire scientific community.
Acknowledgments
Funding: This study was supported by the Key Scientific Research Project of the Department of Education of Anhui Province (No. SK2019A0200) and the 512 Talent Cultivation Program of Bengbu Medical College (No. 51201313). The funding source was not involved in the implementation of the study design or the analysis of the data.
Footnote
Reporting Checklist: The authors have completed the MDAR reporting checklist. Available at https://dx.doi.org/10.21037/atm-21-5010
Data Sharing Statement: Available at https://dx.doi.org/10.21037/atm-21-5010
Conflicts of Interest: All authors have completed the ICMJE uniform disclosure form (available at https://dx.doi.org/10.21037/atm-21-5010). The authors have no conflicts of interest to declare.
Ethical Statement: The authors are accountable for all aspects of the work in ensuring that questions related to the accuracy or integrity of any part of the work are appropriately investigated and resolved. All procedures performed in this study involving human participants were in accordance with the Declaration of Helsinki (as revised in 2013). The study was approved by institutional ethics board of the First Affiliated Hospital of Bengbu Medical College (No. 2021KY035) and informed consent was taken from all the patients.
Open Access Statement: This is an Open Access article distributed in accordance with the Creative Commons Attribution-NonCommercial-NoDerivs 4.0 International License (CC BY-NC-ND 4.0), which permits the non-commercial replication and distribution of the article with the strict proviso that no changes or edits are made and the original work is properly cited (including links to both the formal publication through the relevant DOI and the license). See: https://creativecommons.org/licenses/by-nc-nd/4.0/.
References
- Liu R, Rizzo S, Whipple S, et al. Evaluating eligibility criteria of oncology trials using real-world data and AI. Nature 2021;592:629-33. [Crossref] [PubMed]
- Wong CH, Siah KW, Lo AW. Estimation of clinical trial success rates and related parameters. Biostatistics 2019;20:273-86. [Crossref] [PubMed]
- Gil-Extremera B, Jiménez-López P, Mediavilla-García JD. Clinical trials. A pending subject. Rev Clin Esp (Barc) 2018;218:137-41. [Crossref] [PubMed]
- Bentley C, Cressman S, van der Hoek K, et al. Conducting clinical trials-costs, impacts, and the value of clinical trials networks: A scoping review. Clin Trials 2019;16:183-93. [Crossref] [PubMed]
- Li N, Huang HY, Wu DW, et al. Changes in clinical trials of cancer drugs in mainland China over the decade 2009-18: a systematic review. Lancet Oncol 2019;20:e619-26. [Crossref] [PubMed]
- Chen W, Zheng R, Baade PD, et al. Cancer statistics in China, 2015. CA Cancer J Clin 2016;66:115-32. [Crossref] [PubMed]
- Brown DG, Wobst HJ. A Decade of FDA-Approved Drugs (2010-2019): Trends and Future Directions. J Med Chem 2021;64:2312-38. [Crossref] [PubMed]
- Eshera N, Itana H, Zhang L, et al. Demographics of clinical trials participants in pivotal clinical trials for new molecular entity drugs and biologics approved by FDA From 2010 to 2012. Am J Ther 2015;22:435-55. [Crossref] [PubMed]
- Asaad M, Habibullah NK, Butler CE. The Impact of COVID-19 on Clinical Trials. Ann Surg 2020;272:e222-3. [Crossref] [PubMed]
- Kearney A, Harman NL, Rosala-Hallas A, et al. Development of an online resource for recruitment research in clinical trials to organise and map current literature. Clin Trials 2018;15:533-42. [Crossref] [PubMed]
- Mckane A, Sima C, Ramanathan RK, et al. Determinants of patient screen failures in Phase 1 clinical trials. Invest New Drugs 2013;31:774-9. [Crossref] [PubMed]
- Tiu C, Loh Z, Gan CL, et al. Effect of Reasons for Screen Failure on Subsequent Treatment Outcomes in Cancer Patients Assessed for Clinical Trials. Oncology 2019;97:270-6. [Crossref] [PubMed]
- Park S, Oh DS, Yoo HR, et al. Age-adjusted reference values could be used for screening healthy older people in clinical trials. Maturitas 2013;74:383-5. [Crossref] [PubMed]
- . Guidelines for the diagnosis and treatment of hyperuricemia and gout in China (2019). Chinese Journal of Endocrinology and Metabolism 2020;1-13.
- Cai M. Objective influencing factors of ECG physical examination in healthy people. World Latest Medical Information 2019;78-9.
- Manton KJ, Gauld CS, White KM, et al. Qualitative study investigating the underlying motivations of healthy participants in phase I clinical trials. BMJ Open 2019;9:e024224 [Crossref] [PubMed]
- Raynor DK, Myers L, Blackwell K, et al. Clinical Trial Results Summary for Laypersons: A User Testing Study. Ther Innov Regul Sci 2018;52:606-28. [Crossref] [PubMed]
- Kadam RA, Borde SU, Madas SA, et al. Challenges in recruitment and retention of clinical trial subjects. Perspect Clin Res 2016;7:137-43. [Crossref] [PubMed]
- Mahajan P, Kulkarni A, Narayanswamy S, et al. Reasons why patients fail screening in Indian breast cancer trials. Perspect Clin Res 2015;6:190-3. [Crossref] [PubMed]
- Smeaton LM, Kacanek D, Mykhalchenko K, et al. Screening and Enrollment by Sex in Human Immunodeficiency Virus Clinical Trials in the United States. Clin Infect Dis 2020;71:1300-5. [Crossref] [PubMed]
- Jain N, Cottingham MD, Fisher JA. Disadvantaged, Outnumbered, and Discouraged: Women's Experiences as Healthy Volunteers in U.S. Phase I Trials. Crit Public Health 2020;30:141-52. [Crossref] [PubMed]
- Gong S, Wang C, Song Y, et al. Analysis of common screening failure causes in bioequivalence tests of chemical generics. Chinese Journal of Clinical Pharmacology and Therapeutics 2019;283-7.
- Ladia MAJ, Sison OT, Añonuevo CA, et al. Community-based recruitment for clinical trials poses the need for social and ethical considerations. J Clin Epidemiol 2018;102:78-86. [Crossref] [PubMed]
- Bompart F. Healthy Volunteers for Clinical Trials in Resource-Poor Settings: National Registries Can Address Ethical and Safety Concerns. Cambridge Quarterly of Healthcare Ethics 2018;134-43. [Crossref]
- Tan MH, Thomas M, MacEachern MP. Using registries to recruit subjects for clinical trials. Contemp Clin Trials 2015;41:31-8. [Crossref] [PubMed]
- Damen L, van Agt F, de Boo T, et al. Terminating clinical trials without sufficient subjects. J Med Ethics 2012;38:413-6. [Crossref] [PubMed]
(English Language Editor: D. Fitzgerald)