The role of autoantibody detection in the diagnosis and staging of lung cancer
Introduction
Lung cancer (LC) is the leading cause of cancer death worldwide, and its morbidity and mortality rank first in China. The average 5-year survival rate of LC is less than 20% (1). The main type of LC is non-small cell lung cancer (NSCLC, accounting for approximately 85%), which includes two main subtypes: lung adenocarcinoma (ADC) and lung squamous cell carcinoma (SCC) (2,3). Also, small cell lung cancer (SCLC) and adeno-squamous carcinoma (ASC) are two frequently found subtypes. Tobacco smoking is still the major contributing factor (estimated to cause approximately 90% of all cases) (4). Given the poor survival rate, early detection of LC is a promising avenue to decrease lung mortality. Although serum tumor biomarkers, such as circulating tumor cells (CTCs), blood routine factors, and circulating tumor DNA, have a certain diagnostic role, efficient tools for early monitoring of LC are still limited.
Notably, in the early stages of tumorigenesis, the humoral immune response in the host can be activated, and tumor-associated antigens (TAAs) can be detected in the serum of tumor patients, as well as the corresponding autoantibodies. TAAs are key components of signal pathways in the evolution of tumors, participate in the cellular signal transduction process, and regulate cell proliferation and apoptosis. The expression of TAA stimulates the immune response and produces tumor-associated autoantibodies. Therefore, in theory, the detection of tumor autoantibodies may provide satisfactory sensitivity and specificity for LC screening. Furthermore, the combined detection of different types of tumor autoantibodies may help to improve the accuracy of LC diagnosis and provide new ideas for clinical screening. Published studies have shown that serum TAAs recognition can be used in LC screening, clinical course monitoring, and recurrence prediction (5-7).
Previously, the clinical value of seven autoantibodies (p53, PGP9.5, SOX2, GAGE7, GBU4-5, MAGEA1, and CAGE) has been surveyed in our pilot observation and other published studies (8-13). The sensitivity of the seven autoantibodies specific for Europeans (P53, c-myc, HER-2, NYESO1, CAGE, MUC1, and GBU4-5) is 76% and the specificity is 92% (13). And the China Food and Drug Administration (CFDA) have specifically selected seven autoantibodies suitable for Chinese people (14). P53 can be detected in lung cancer patients as autoantibody produced by the tumor suppressor genes. The MAGE-A1 belongs to the cancer/testicular antigen of the X chromosome cluster and is expressed in a variety of tumors. GAGE is found in a variety of cancers, most common in melanoma and LC. CAGE is associated with the cell cycle and plays a role in cell growth and proliferation. GBU4-5 is immunogenicity, cancer-specific, and may provide potential targets for the cancer diagnosis. PGP9.5 increases deubiquitylation of cyclin, which may lead to uncontrolled growth of tumor cells. SOX2 is expressed in LC, breast cancer, ovarian cancer, and it is associated with metastasis, tumor stemness and drug resistance. The clear role of autoantibody in the LC development is not fully understood. Generally, these autoantibodies were produced by B cells or T cells, and they may trigger immune responses during LC development (5,6,15). Herein, we aimed to further investigate the role of these autoantibodies in the diagnosis and staging of LC. More specifically, this work analyzed the association between the plasma levels of autoantibodies and the onset, stages, and subtypes of LC. Meanwhile, regression analysis in LC prediction (onset and stages) was also conducted. Our work can add some novel idea and new knowledge autoantibody detection in the diagnosis and staging of lung cancer. For example, published studies used the 7 autoantibodies to distinguish lung cancer and benign lung disease (16,17), we further investigated their association with LC subtypes and stages, and we reported the regression analysis of LC diagnosis, subtypes, and stages using the 7 autoantibodies and demographic features. We present the following article in accordance with the STARD reporting checklist (available at https://dx.doi.org/10.21037/atm-21-5357).
Methods
Subjects
All procedures performed in this study involving human participants were in accordance with the Declaration of Helsinki (as revised in 2013). The study was approved by the ethics committee of the Fourth Hospital of Hebei Medical University (No. 2021KY265). Individual consent for this retrospective analysis was waived. We included a total of 135 individuals who were admitted to the Fourth Hospital of Hebei Medical University from November 2016 to February 2017. The patients were divided into a LC group and a control group according to the final diagnosis. The control group included individuals with benign lung disease as well as healthy subjects. The inclusion criteria of the LC cancer group were as follows: (I) patients aged 18–86 years old; (II) patients with clearly diagnosed LC by pathology (histopathology or cytopathology), as well as documented information about the LC histological type; and (III) patients without tumors of other organs. The inclusion criteria of the control group were as follows: (I) patients aged 18–86 years old; (II) those with lung malignant tumors were excluded by computed tomography (CT) or auxiliary examinations, or cases in which no abnormalities in chest CT examination were found; and (III) patients without tumors of other organs. For all included individuals, the key demographic data (such as gender and age) were recorded. Also, 4 mL of venous blood was collected from all subjects, and after centrifugation (2,000 r/min for 10 min) the serum was acquired and stored at −20 °C before use.
Detection of autoantibodies
In this study, seven autoantibody detection kits (ELISA method) produced by Hangzhou Kaibao Biotechnology Co., Ltd. (Hangzhou, China) were utilized. The ELISA process was performed in strict accordance with the official manual. When all steps had been performed, the OD450 value was recorded by a microplate reader.
Statistical analysis
Categorical data were described by percentages, and numeric variables were expressed as mean ± standard deviation (SD). The differences in values derived from categorical variables were compared using the Chi-squared test or Fisher’s exact test. The Student’s t-test or Mann-Whitney U test was applied for comparison between two groups of continuous variables; and one-way ANOVA was used for three or four groups. Logistic regression was used for prediction of LC, LC subtypes, and stages. A P value <0.05 was considered statistically significant. The area under the receiver operating characteristic (ROC) curve (AUC) was used to assess the predictive performance of each regression.
Results
The clinical characteristics of enrolled subjects
The clinical characteristics of enrolled subjects are presented in Table 1. A total of 135 patients were included. Among them, there were 80 (59.3%) males and 55 (40.7%) females. The age range was 35–80 years old, with an average age of 59.14±11.91 years. At least 40% of all individuals had a history of smoking. There were 47 (34.8%) normal cases and 88 (65.2%) LC cases. For the histopathological subtypes of the LC cohort, most cases (54, 61.4%) were ADC patients, followed by 18 (20.5%) cases with SCC, seven (8.0%) cases with SCLC, two (2.3%) cases with ASC, and three cases with other types. Also, among all LC patients, there were 53 (60.2%) stages I–II (early stage) cases, five (5.7%) III-a cases, two (2.3%) III-b cases, and 28 (31.8%) IV cases.
Table 1
Characteristics | Subtypes | Number | % | Mean ± SD |
---|---|---|---|---|
Total cases | 135 | 100 | ||
Gender | Male | 80 | 59.3 | |
Female | 55 | 40.7 | ||
Age | 135 | 59.14±11.91 | ||
Smoking | Unknown | 6 | 4.4 | |
No | 75 | 55.6 | ||
Yes | 54 | 40.0 | ||
Lung cancer diagnosis | Normal | 47 | 34.8 | |
Lung cancer | 88 | 65.2 | ||
Lung cancer subtypes | ADC | 54 | 61.4 | |
SCC | 18 | 20.5 | ||
ASC | 2 | 2.3 | ||
SCLC | 7 | 8.0 | ||
Others | 3 | 3.4 | ||
Undetermined | 4 | 4.5 | ||
Stages | I–II | 53 | 60.2 | |
III-a | 5 | 5.7 | ||
III-b | 2 | 2.3 | ||
IV | 28 | 31.8 |
ADC, adenocarcinoma; SCC, squamous cell carcinoma; ASC, adenosquamous carcinoma; SCLC, small cell lung carcinoma.
The change of autoantibody levels along with LC onset, subtypes, and stages
Through autoantibody detection, no adverse events were observed. Among the seven types of autoantibodies, only P53 and GBU4-5 had a significant increase in the LC group (P<0.01) (Table 2). No changes were found regarding the other autoantibodies. For LC patients, we further observed different autoantibody levels among the various subtypes. Only ADC, SCC, and SCLC had more than three samples, and thus, other types were not used for comparison. The P53 autoantibody was markedly different between the groups (P<0.05); the P53 autoantibody levels in the SCC subgroup were two times higher than those of ADC, while those of the SCLC subgroup were two times higher than those of SCC (Table 3). Meanwhile, the GBU4-5 level was significantly higher in the SCLC patients compared to those with ADC. Taken together, the P53 and GBU4-5 autoantibodies may be potential indicators of LC, and their range may be useful in distinguishing the subtypes.
Table 2
Autoantibody | Control | Lung cancer | t | P |
---|---|---|---|---|
P53 | 4.16±11.77 | 15.90±37.97 | 2.671 | 0.009 |
PGP9.5 | 8.55±29.21 | 15.06±41.05 | 0.965 | 0.336 |
SOX2 | 4.23±12.19 | 7.84±19.35 | 1.325 | 0.188 |
GAGE7 | 5.88±16.03 | 8.37±23.24 | 0.654 | 0.514 |
GBU4-5 | 1.01±1.42 | 4.91±11.06 | 3.256 | 0.002 |
MAGEA1 | 3.84±15.88 | 10.59±26.41 | 1.852 | 0.066 |
CAGE | 5.22±17.84 | 8.49±29.48 | 0.693 | 0.489 |
Table 3
Autoantibody | ADC | SCC | SCLC | F | P |
---|---|---|---|---|---|
P53 | 9.88±28.19 | 23.10±46.83 | 51.28±70.80* | 4.033 | 0.022 |
PGP9.5 | 8.38±30.21 | 23.70±49.66 | 32.48±76.82 | 1.750 | 0.181 |
SOX2 | 6.52±17.04 | 4.35±8.92 | 14.11±28.62 | 0.850 | 0.431 |
GAGE7 | 7.99±24.01 | 13.85±29.70 | 4.67±7.87 | 0.505 | 0.606 |
GBU4-5 | 3.53±10.91 | 4.29±9.29 | 12.28±12.26* | 2.080 | 0.132 |
MAGEA1 | 10.97±27.15 | 13.29±28.55 | 0.80±1.02* | 0.582 | 0.561 |
CAGE | 8.32±31.32 | 4.14±6.61 | 23.70±57.35 | 1.027 | 0.363 |
*, P<0.05 vs. ADC. ADC, adenocarcinoma; SCC, squamous cell carcinoma; SCLC, small cell lung carcinoma.
Interestingly, the MAGEA1 autoantibody was more enriched in ADC and SCC versus SCLC (P<0.05). Furthermore, we probed which autoantibodies may change along with stages, and found that antibodies against PGP9.5, SOX2, GBU4-5, and CAGE were associated with stages (P≤0.05 for PGP9.5 and CAGE; P<0.01 for SOX2 and GBU4-5). Their expressions were significantly higher in the advanced stage (IV) versus early stages (I–II) (Table 4). Therefore, these autoantibodies may be more efficient in screening advanced LC compared to the diagnosis of the entire LC cohort.
Table 4
Autoantibody | Stages | Level (U/mL) | F | P |
---|---|---|---|---|
PGP9.5 | I–II | 5.75±15.81 | 2.714 | 0.05 |
III-a | 32.48±30.03 | |||
III-b | 3.56±1.82 | |||
IV | 30.39±65.84* | |||
SOX2 | I–II | 3.26±8.70 | 4.17 | 0.008 |
III-a | 1.31±0.81 | |||
III-b | 3.75±5.07 | |||
IV | 17.96±30.05* | |||
GBU4-5 | I–II | 1.42±3.51 | 6.365 | 0.001 |
III-a | 4.59±6.21 | |||
III-b | 2.37±2.86 | |||
IV | 11.75±17.06* | |||
CAGE | I–II | 1.81±2.37 | 3.258 | 0.026 |
III-a | 4.54±3.95 | |||
III-b | 1.87±0.39 | |||
IV | 22.31±49.95* |
*, P<0.05 vs. I–II.
Regression analysis of LC diagnosis, subtypes, and stages
Next, we used above demographic factors and autoantibody levels to predict LC, subtypes, and stages. The results of the two-end logistic regression analysis for LC diagnosis are shown in Table 5, with age and smoking being more significant risk factors than autoantibodies. Using this regression, the outcome (for LC prediction) was named LCprediction, whose power was higher than that of single factors. When applying the variable LCprediction to distinguish LC, the AUC of the ROC curve was 0.743 (Figure 1). However, for each autoantibody, the predictive performance was poor.
Table 5
Factors | B | Wald | P | Exp (B) |
---|---|---|---|---|
Gender (female vs. male) | 0.627 | 1.381 | 0.24 | 1.872 |
Age | 0.035 | 3.98 | 0.046 | 1.036 |
Smokers (yes vs. no) | 1.246 | 5.073 | 0.024 | 3.477 |
p53 | 0.019 | 1.802 | 0.179 | 1.019 |
PGP9.5 | −0.006 | 0.523 | 0.47 | 0.994 |
SOX2 | −0.008 | 0.231 | 0.631 | 0.992 |
GAGE7 | −0.004 | 0.119 | 0.73 | 0.996 |
GBU4-5 | 0.114 | 2.877 | 0.09 | 1.121 |
Constant | −2.326 | 3.95 | 0.047 | 0.098 |
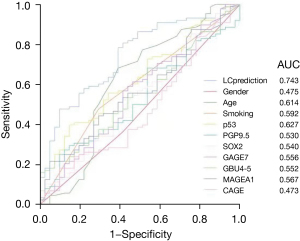
Next, multinomial logistic regression was conducted for subtype prediction (Table 6). Compared to the normal controls, only demographic factors (e.g., age and smoking) were found to be significant risk factors for SCC. Notably, for SCLC prediction, p53 and GBU4-5 autoantibodies were significant risk factors in the equation, which was consistent with Table 3, and suggests that autoantibodies may have unique advantages in the recognition of SCLC.
Table 6
Outcome | Factors | Wald | P | Exp (B) |
---|---|---|---|---|
ADC | Age | 1.798 | 0.180 | 1.025 |
p53 | 0.827 | 0.363 | 1.016 | |
PGP9.5 | 1.510 | 0.219 | 0.970 | |
SOX2 | 0.327 | 0.567 | 0.990 | |
GAGE7 | 0.042 | 0.838 | 0.997 | |
GBU4-5 | 1.899 | 0.168 | 1.112 | |
MAGEA1 | 1.209 | 0.272 | 1.017 | |
CAGE | 0.020 | 0.888 | 0.998 | |
Gender (female vs. male) | 2.530 | 0.112 | 2.395 | |
Smokers (yes vs. no) | 1.123 | 0.289 | 1.889 | |
SCC | Age | 5.030 | 0.025 | 1.093 |
p53 | 1.701 | 0.192 | 1.025 | |
PGP9.5 | 1.201 | 0.273 | 1.037 | |
SOX2 | 0.040 | 0.842 | 0.992 | |
GAGE7 | 0.148 | 0.700 | 1.006 | |
GBU4-5 | 0.819 | 0.366 | 1.110 | |
MAGEA1 | 0.008 | 0.930 | 0.998 | |
CAGE | 2.452 | 0.117 | 0.900 | |
Gender (female vs. male) | 0.000 | 0.994 | <0.001 | |
Smokers (yes vs. no) | 6.020 | 0.014 | 0.1661 | |
ASC | Age | 0.032 | 0.858 | 0.012 |
p53 | 0.022 | 0.883 | <0.001 | |
PGP9.5 | 0.023 | 0.878 | <0.001 | |
SOX2 | 0.028 | 0.867 | 34.881 | |
GAGE7 | 0.002 | 0.966 | 0.306 | |
GBU4-5 | 0.024 | 0.876 | 23.748 | |
MAGEA1 | 0.000 | 0.998 | 0.216 | |
CAGE | 0.027 | 0.870 | 13.536 | |
Gender (female vs. male) | 0.000 | 0.993 | <0.001 | |
Smokers (yes vs. no) | 0.001 | 0.974 | <0.001 | |
SCLC | Age | 0.134 | 0.715 | 1.019 |
p53 | 5.542 | 0.019 | 1.054 | |
PGP9.5 | 0.143 | 0.706 | 0.984 | |
SOX2 | 0.978 | 0.323 | 0.949 | |
GAGE7 | 0.103 | 0.748 | 0.979 | |
GBU4-5 | 7.753 | 0.005 | 1.333 | |
MAGEA1 | 1.647 | 0.199 | 0.870 | |
CAGE | 0.123 | 0.726 | 0.986 | |
Gender (female vs. male) | 0.089 | 0.766 | 2.150 | |
Smokers (yes vs. no) | 2.019 | 0.155 | 20.629 | |
Others | Age | 0.508 | 0.476 | 1.077 |
p53 | 0.005 | 0.943 | 0.986 | |
PGP9.5 | 2.346 | 0.126 | 1.071 | |
SOX2 | 0.193 | 0.660 | 0.565 | |
GAGE7 | 0.274 | 0.601 | 0.868 | |
GBU4-5 | 1.402 | 0.236 | 1.225 | |
MAGEA1 | 0.102 | 0.750 | 0.814 | |
CAGE | 0.030 | 0.863 | 0.975 | |
Gender (female vs. male) | – | – | <0.001 | |
Smokers (yes vs. no) | 0.000 | 0.998 | <0.001 |
ADC, adenocarcinoma; SCC, squamous cell carcinoma; ASC, adenosquamous carcinoma; SCLC, small cell lung carcinoma.
Finally, the logistic regression regarding stages was analyzed. Multiple-stage prediction was performed by multinomial logistic regression using the levels of PGP9.5, SOX2, GBU4-5, and CAGE autoantibodies (Table 7), given that these four variables were associated with stage progression in the univariate analysis (Table 4). In this regression, GBU4-5 showed a special ability in indicating the stages. The outcomes of this regression can easily distinguish the early stages (I–II) and the advanced stage (IV); however, its power is still poor in recognition of the mid-stage (as well as the mid-advanced-stage) LC.
Table 7
Factors | B | Wald | P | Exp (B) |
---|---|---|---|---|
Age | 0.045 | 3.644 | 0.056 | 1.046 |
PGP9.5 | −0.001 | 0.012 | 0.914 | 0.999 |
SOX2 | 0.012 | 0.576 | 0.448 | 1.012 |
GBU4-5 | 0.118 | 8.232 | 0.004 | 1.125 |
CAGE | 0.019 | 2.684 | 0.101 | 1.019 |
Constant | −4.809 | 9.768 | 0.002 | 0.008 |
For warning the advanced LC, we performed the two-end logistic regression (Table 8). Similar to Table 7, the result showed that age and the GBU4-5 autoantibody perform exceptionally in the recognition of advanced LC. The prediction outcome of this regression (named Advprediction) was assessed using a ROC curve in conjunction with age, PGP9.5, SOX2, GBU4-5, and CAGE (Figure 2), which showed the highest AUC (0.798).
Table 8
Factors | B | Wald | P |
---|---|---|---|
Age | 0.039 | 6.254 | 0.012 |
p53 | 0.011 | 1.949 | 0.163 |
PGP9.5 | −0.003 | 0.133 | 0.716 |
SOX2 | 0.003 | 0.040 | 0.841 |
GAGE7 | −0.008 | 0.801 | 0.371 |
GBU45 | 0.125 | 10.645 | 0.001 |
MAGEA1 | 0.009 | 0.894 | 0.344 |
CAGE | 0.005 | 0.228 | 0.633 |
Gender (female vs. male) | 0.116 | 0.061 | 0.804 |
Smokers (yes vs. no) | 0.895 | 3.855 | 0.050 |
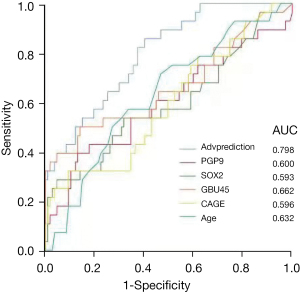
Discussion
Regarding LC screening, there have been increasing concerns about excessive inspection costs, accumulated radiation exposure, and false positive rates. Particular attention has been paid to the value of blood molecular markers. Blood markers can be used to assess the risk of tumors in the preliminary screening before CT examination. The common blood molecular and cellular indicators include blood routine indices, microRNA, circulating tumor cells, and ctDNAs. Preoperative blood markers can be prognostic factors for LC surgery, including plasma fibrinogen levels, serum C-reactive protein, hemoglobin concentration, and platelet count (18). With the help of peripheral blood markers, known prognostic factors can accurately predict the individualized survival probability of patients with NSCLC (19). Recently, it has been reported that peripheral blood markers are useful in the prediction of immune-related adverse effects in advanced NSCLC treated with PD -1 inhibitors (20).
Using autoantibody tests might enhance LC screening, especially when combined with imaging approaches (21). Moreover, serum S100B and S100B autoantibody levels may help to identify which LC patients have brain metastases (22). But it has been reported that autoantibody test has insufficient sensitivity for detecting LC at an equally early stage as with low-dose computed tomography screening (23). For LC, the presence of autoantibodies against tumor antigens can be detected in patient serum samples 5 years prior to LC diagnosis (24). Chapman et al. evaluated a panel of tumor-associated autoantibody profiles as an aid to other LC screening modalities, which included p53, c-myc, HER2, NY-ESO-1, CAGE, MUC1, and GBU4-5. These seven kinds of tumor antigens produced autoantibodies for detection. Elevated levels of autoantibodies have been observed to at least 1/7 antigens in 76% of all patients with LC plasma assessed, as well as 89% of node-negative patients, with a specificity of 92%. The sensitivity of the antibody level produced by the MUC1 antigen was the highest; moreover, the combined detection of these seven antibodies had a specificity of 92% and a sensitivity of 76% (25). Even after imaging, autoantibody blood tests can improve earlier diagnosis of LC, as shown by a recent randomized trial (21). In China, this panel of seven autoantibodies for LC was launched by Ren et al. (9).
Based on these studies, we employed the ELISA method in this study to assess the role of autoantibody markers, and some novel findings were obtained. The main positive results include the following: (I) P53 and GBU4-5 autoantibodies were significantly enriched in LC patients versus controls; (II) SCLC had particularly high levels of P53 and GBU4-5 autoantibodies, but a low MAGEA1 level; (III) four types of autoantibodies (PGP9.5, SOX2, GBU4-5, and CAGE) may change along with stages (especially highly expressed in the advanced stage); and (IV) the combination of demographic factors and these antibodies can be efficient in prediction of LC onset and LC stages. According to known articles, PGP9.5 is associated with the clinical stage of lung cancer (the positive rate of lung cancer was 44% in stage I, and 75% in stages II and III) (26). SOX2 was associated with sex and smoking history and was highly expressed in squamous cell carcinomas (27); but opposite conclusion also exists (some meta-analysis reported that SOX2 had nothing to do with gender and smoking history) (28). At the same time, it had a high expression in squamous cell carcinomas, which indicated better prognosis in different pathological types of lung cancer, suggesting that SOX2 has the potential to become a prognostic indicator (13). GAGE7 was reported to be unrelated to the LC pathological classification, but its expression in stages II and IIIa was significantly higher than that in stage I (29). The positive rate of CAGE varies between different sexes, and these differences should be excluded when using CAGE to diagnose lung cancer patients (13). Also, the positive rate of MAGEA1 was different between different sexes and pathological types. This suggested that MAGEA1 had the potential to function as a precise diagnosis autoantibody. The expression rate of MAGEA in lung adenocarcinoma is 46.66%, which is related to a lower 10-year survival rate and was a marker of poor prognosis (30).
In this study, the sensitivity of each autoantibody to LC screening was not high enough. Therefore, single-autoantibody tests cannot meet the requirements of large-scale inspection in clinical practice. In order to improve the detection sensitivity and efficiency, we combined the demographic information and autoantibodies to construct the predictive variables, whose AUCs could be as high as 0.798. Our results are similar to those of Ren et al., who reported an AUC of approximately 0.781 based on seven autoantibodies (9). However, in that study, the results showed that the serum concentrations of PGP9.5, SOX2, GBU4-5, MAGEA1, and CAGE antibodies were significantly higher in the LC group than the healthy controls (not only P53 and GBU4-5 autoantibodies as in our results), but the concentrations of p53 and GAGE7 in LC were similar to the controls. Furthermore, their significant findings were based on a large sample number (at least 2,308). The diagnostic efficiency of these seven autoantibodies in LC has been investigated other Chinese scholars, with similar logistic regression results to our findings; that is, a combination of the seven autoantibodies resulted in more reliable detection than any single autoantibody in early-stage LC [AUC: 0.764; 95% confidence interval (CI): 0.718–0.811] (11). Our conclusion is highly consistent with the above studies, and we believe that when combined with more data, such as imaging, other blood indicators, lifestyle habits, and past history information, the diagnosis of LC can be further improved.
It has been reported that the serum P53 protein and P53 autoantibodies are associated with increased tumor risk and can be used as serological markers for early diagnosis of malignant tumors. Wu et al. reported that the positive rate of P53 protein in the tumor group was 4.22%, while that of the healthy control group was 0.34%. They also found that the positive rate of P53 autoantibodies in malignant tumor patients was 14.59% while and that of the control group was 1.02% [adjusted odds ratio (OR) =14.27; 95% CI: 6.75–30.16] (31).
Sasaki et al. observed the correlation between NSCLC and PGP9.5 expression, and found that the expression of PGP9.5 is correlated with T -status in NSCLC (32). PGP9.5 transcription was detected in 12.8% of tumor samples, and the expression ratio of T1–2 stages was 6/54 (11.1%); moreover, PGP9.5 was widely expressed in T3 and T4 stage. Therefore, PGP9.5 may be related to the invasion and development of NSCLC. Yanagawa et al. performed epigenetic studies and found that the positive rate of MAGE-A1 expression was 29.9%, the positive rate of MAGE-A3 expression was 38.8%, and the demethylation rates of MAGE-A/-A3 genes were 41.8% and 46.3, respectively. In particular, patients with MAGE expression have a poor prognosis. Their study strongly suggested that MAGE expression mediated by demethylation of MAGE promoters is associated with aggressive progression of NSCLC (33).
GBU4-5 is an ATP-binding RNA helicase, which plays an important role in the process of carcinogenesis. It is both tumor-specific and immunogenic in the process of cell differentiation, transposon methylation, and gene expression. CAGE (CAGE1 or CT95) belongs to the DEAD box helicase family. It is specifically expressed in malignant tumors and testicular tissues (which is why it is also called cancer/testis antigen). In normal individuals, the expression of cancer/testis antigens is largely restricted to the testis. In the case of cancer patients, the expression has also been frequently observed in tumoral cells, and cancer/testis antigens are considered to be promising targets for immunotherapy (34). CAGE expression is related to the cell cycle. Known diseases associated with CAGE1 include rectum ADC and supraglottis neoplasm. SOX2 is a well-known stem cell marker and an essential regulatory factor in tumorigenesis and metastasis (35). In LC, SOX2 signaling can promote cell proliferation, epithelial-mesenchymal transition, and metastasis (36). Regulation of SOX2 and stemness can strongly affect the development and resistance of LC (37). Moreover, it determines the LC cell fate and shapes the tumor immune microenvironment (38). In this study, we noticed that P53 and GBU4-5 are more useful in LC diagnosis, while PGP9.5, SOX2, GBU4-5, and CAGE were important in LC staging. In particular, GBU4-5 showed a high efficacy in the univariate analysis and multinomial logistic regression and is worthy of more attention in future mechanism research.
Yet, this study has some limitations. Firstly, the control group included patients with benign lung diseases (such as pneumonia, tuberculosis, chronic obstructive pulmonary disease, and inflammatory pseudotumor). Also, some patients have risk factors, such as elderly age and smoking; these are high-risk cases for LC and the potential development of LC cannot be absolutely excluded. That is, some subjects may be in the latent or initial stage, and the current medical examination could not spot the trivial lesions. Secondly, we obtained some conflicting results to known studies. For example, in Zhang et al.’s study, the concentration of GBU4-5 in the ADC group was significantly higher than that in the SCC group (39); however, in our results, the SCC group exhibited a slightly higher expression of GBU4-5.
In summary, our study confirmed the diagnostic value of tumor-associated autoantibodies. These may be useful as latent tumor markers to facilitate the detection of early LC. Single autoantibody testing is not yet sufficient in LC cancer screening, and the combined detection of autoantibodies can improve the sensitivity of detection compared with single antibody detection, especially for P53, PGP9.5, SOX2, GBU4-5, and CAGE autoantibodies.
Acknowledgments
Funding: None.
Footnote
Reporting Checklist: The authors have completed the STARD reporting checklist. Available at https://dx.doi.org/10.21037/atm-21-5357
Data Sharing Statement: Available at https://dx.doi.org/10.21037/atm-21-5357
Conflicts of Interest: All authors have completed the ICMJE uniform disclosure form (available at https://dx.doi.org/10.21037/atm-21-5357). The authors have no conflicts of interest to declare.
Ethical Statement: The authors are accountable for all aspects of the work in ensuring that questions related to the accuracy or integrity of any part of the work are appropriately investigated and resolved. All procedures performed in this study involving human participants were in accordance with the Declaration of Helsinki (as revised in 2013). The study was approved by the ethics committee of the Fourth Hospital of Hebei Medical University (No. 2021KY265). Individual consent for this retrospective analysis was waived.
Open Access Statement: This is an Open Access article distributed in accordance with the Creative Commons Attribution-NonCommercial-NoDerivs 4.0 International License (CC BY-NC-ND 4.0), which permits the non-commercial replication and distribution of the article with the strict proviso that no changes or edits are made and the original work is properly cited (including links to both the formal publication through the relevant DOI and the license). See: https://creativecommons.org/licenses/by-nc-nd/4.0/.
References
- Siegel RL, Miller KD, Jemal A. Cancer statistics, 2018. CA Cancer J Clin 2018;68:7-30. [Crossref] [PubMed]
- Molinier O, Goupil F, Debieuvre D, et al. Five-year survival and prognostic factors according to histology in 6101 non-small-cell lung cancer patients. Respir Med Res 2020;77:46-54. [Crossref] [PubMed]
- Butnor KJ. Controversies and challenges in the histologic subtyping of lung adenocarcinoma. Transl Lung Cancer Res 2020;9:839-46. [Crossref] [PubMed]
- Chapman CJ, Thorpe AJ, Murray A, et al. Immunobiomarkers in small cell lung cancer: potential early cancer signals. Clin Cancer Res 2011;17:1474-80. [Crossref] [PubMed]
- Mizukami M, Hanagiri T, Baba T, et al. Identification of tumor associated antigens recognized by IgG from tumor-infiltrating B cells of lung cancer: correlation between Ab titer of the patient's sera and the clinical course. Cancer Sci 2005;96:882-8. [Crossref] [PubMed]
- Safi S, Yamauchi Y, Rathinasamy A, et al. Functional T cells targeting tumor-associated antigens are predictive for recurrence-free survival of patients with radically operated non-small cell lung cancer. Oncoimmunology 2017;6:e1360458 [Crossref] [PubMed]
- Li S, Ma Y, Xiong Y, et al. Five tumor-associated autoantibodies expression levels in serum predict lung cancer and associate with poor outcome. Transl Cancer Res 2019;8:1364-73. [Crossref]
- Du Q, Yu R, Wang H, et al. Significance of tumor-associated autoantibodies in the early diagnosis of lung cancer. Clin Respir J 2018;12:2020-8. [Crossref] [PubMed]
- Ren S, Zhang S, Jiang T, et al. Early detection of lung cancer by using an autoantibody panel in Chinese population. Oncoimmunology 2018;7:e1384108 [Crossref] [PubMed]
- Yin-Yu M, Fu-Yi X, Fei-Bo W, et al. Performance Evaluation of an Enzyme-Linked Immunosorbent Assay for Seven Autoantibodies in Lung Cancer. Clin Lab 2019; [Crossref] [PubMed]
- Huang H, Luo W, Ni Y, et al. The diagnostic efficiency of seven autoantibodies in lung cancer. Eur J Cancer Prev 2020;29:315-20. [Crossref] [PubMed]
- Mu Y, Xie F, Sun T. Clinical value of seven autoantibodies combined detection in the diagnosis of lung cancer. J Clin Lab Anal 2020;34:e23349 [Crossref] [PubMed]
- Luo B, Mao G, Ma H, et al. The role of seven autoantibodies in lung cancer diagnosis. J Thorac Dis 2021;13:3660-8. [Crossref] [PubMed]
- Wang W, Zhuang R, Ma H, et al. The diagnostic value of a seven-autoantibody panel and a nomogram with a scoring table for predicting the risk of non-small-cell lung cancer. Cancer Sci 2020;111:1699-710. [Crossref] [PubMed]
- Farlow EC, Patel K, Basu S, et al. Development of a multiplexed tumor-associated autoantibody-based blood test for the detection of non-small cell lung cancer. Clin Cancer Res 2010;16:3452-62. [Crossref] [PubMed]
- Huo Y, Guo Z, Gao X, et al. Case study of an autoantibody panel for early detection of lung cancer and ground-glass nodules. J Cancer Res Clin Oncol 2020;146:3349-57. [Crossref] [PubMed]
- Solassol J, Maudelonde T, Mange A, et al. Clinical relevance of autoantibody detection in lung cancer. J Thorac Oncol 2011;6:955-62. [Crossref] [PubMed]
- Hamid UI, Al-Saudi R, Paul I, et al. Role of preoperative blood markers as prognostic factors for lung cancer surgery. Asian Cardiovasc Thorac Ann 2019;27:288-93. [Crossref] [PubMed]
- Xie D, Allen MS, Marks R, et al. Nomogram prediction of overall survival for patients with non-small-cell lung cancer incorporating pretreatment peripheral blood markers. Eur J Cardiothorac Surg 2018;53:1214-22. [Crossref] [PubMed]
- Liu W, Liu Y, Ma F, et al. Peripheral Blood Markers Associated with Immune-Related Adverse Effects in Patients Who Had Advanced Non-Small Cell Lung Cancer Treated with PD-1 Inhibitors. Cancer Manag Res 2021;13:765-71. [Crossref] [PubMed]
- Sullivan FM, Mair FS, Anderson W, et al. Earlier diagnosis of lung cancer in a randomised trial of an autoantibody blood test followed by imaging. Eur Respir J 2021;57:2000670 [PubMed]
- Choi H, Puvenna V, Brennan C, et al. S100B and S100B autoantibody as biomarkers for early detection of brain metastases in lung cancer. Transl Lung Cancer Res 2016;5:413-9. [Crossref] [PubMed]
- González Maldonado S, Johnson T, Motsch E, et al. Can autoantibody tests enhance lung cancer screening?-an evaluation of EarlyCDT®-Lung in context of the German Lung Cancer Screening Intervention Trial (LUSI). Transl Lung Cancer Res 2021;10:233-42. [Crossref] [PubMed]
- Zhong L, Coe SP, Stromberg AJ, et al. Profiling tumor-associated antibodies for early detection of non-small cell lung cancer. J Thorac Oncol 2006;1:513-9. [Crossref] [PubMed]
- Chapman CJ, Murray A, McElveen JE, et al. Autoantibodies in lung cancer: possibilities for early detection and subsequent cure. Thorax 2008;63:228-33. [Crossref] [PubMed]
- Hibi K, Westra WH, Borges M, et al. PGP9.5 as a candidate tumor marker for non-small-cell lung cancer. Am J Pathol 1999;155:711-5. [Crossref] [PubMed]
- Li Q, Liu F, Zhang Y, et al. Association of SOX2 and Nestin DNA amplification and protein expression with clinical features and overall survival in non-small cell lung cancer: A systematic review and meta-analysis. Oncotarget 2016;7:34520-31. [Crossref] [PubMed]
- Chen Y, Huang Y, Huang Y, et al. The prognostic value of SOX2 expression in non-small cell lung cancer: a meta-analysis. PLoS One 2013;8:e71140 [Crossref] [PubMed]
- Gjerstorff MF, Pøhl M, Olsen KE, et al. Analysis of GAGE, NY-ESO-1 and SP17 cancer/testis antigen expression in early stage non-small cell lung carcinoma. BMC Cancer 2013;13:466. [Crossref] [PubMed]
- Sang M, Gu L, Yin D, et al. MAGE-A family expression is correlated with poor survival of patients with lung adenocarcinoma: a retrospective clinical study based on tissue microarray. J Clin Pathol 2017;70:533-40. [Crossref] [PubMed]
- Wu M, Mao C, Chen Q, et al. Serum p53 protein and anti-p53 antibodies are associated with increased cancer risk: a case-control study of 569 patients and 879 healthy controls. Mol Biol Rep 2010;37:339-43. [Crossref] [PubMed]
- Sasaki H, Yukiue H, Moriyama S, et al. Expression of the protein gene product 9.5, PGP9.5, is correlated with T-status in non-small cell lung cancer. Jpn J Clin Oncol 2001;31:532-5. [Crossref] [PubMed]
- Yanagawa N, Tamura G, Oizumi H, et al. MAGE expressions mediated by demethylation of MAGE promoters induce progression of non-small cell lung cancer. Anticancer Res 2011;31:171-5. [PubMed]
- Alsheimer M, Drewes T, Schütz W, et al. The cancer/testis antigen CAGE-1 is a component of the acrosome of spermatids and spermatozoa. Eur J Cell Biol 2005;84:445-52. [Crossref] [PubMed]
- Chaudhary S, Islam Z, Mishra V, et al. Sox2: A Regulatory Factor in Tumorigenesis and Metastasis. Curr Protein Pept Sci 2019;20:495-504. [Crossref] [PubMed]
- Wang K, Ji W, Yu Y, et al. FGFR1-ERK1/2-SOX2 axis promotes cell proliferation, epithelial-mesenchymal transition, and metastasis in FGFR1-amplified lung cancer. Oncogene 2018;37:5340-54. [Crossref] [PubMed]
- Schaal CM, Bora-Singhal N, Kumar DM, et al. Regulation of Sox2 and stemness by nicotine and electronic-cigarettes in non-small cell lung cancer. Mol Cancer 2018;17:149. [Crossref] [PubMed]
- Mollaoglu G, Jones A, Wait SJ, et al. The Lineage-Defining Transcription Factors SOX2 and NKX2-1 Determine Lung Cancer Cell Fate and Shape the Tumor Immune Microenvironment. Immunity 2018;49:764-779.e9. [Crossref] [PubMed]
- Zhang R, Ma L, Li W, et al. Diagnostic value of multiple tumor-associated autoantibodies in lung cancer. Onco Targets Ther 2019;12:457-69. [Crossref] [PubMed]
(English Language Editor: A. Kassem)