Development and validation of an early predictive nomogram for delayed cerebral ischemia after aneurysmal subarachnoid hemorrhage
Introduction
Aneurysmal subarachnoid hemorrhage (aSAH) is a serious form of hemorrhagic stroke with high rates of mortality and permanent disability for those who survive the initial hemorrhage (1). Cerebrovascular spasm (CVS) was once considered the major pathological cause of death or disability in aSAH patients until it was found that the occurrence of cerebral vasospasm and cerebral ischemia events was often inconsistent in clinical practice (2). In recent years, it has been widely accepted that early brain injury (EBI) is the main prognosticator of SAH.A series of ischemic events occurring after EBI are classified as delayed cerebral ischemia (DCI). Therefore, EBI and DCI, respectively, represent the characteristic pathophysiological changes after aSAH in the temporal category. At present, DCI is also considered a significant cause of poor functional outcome in aSAH patients (3). Recent reports suggest that approximately one-third of aSAH patients may develop DCI during hospitalization (4). However, clinical trials to date have been largely unsuccessful at preventing or treating DCI after SAH (5). On the one hand, this is related to the complex mechanism of DCI; on the other hand, it may also be attributed to a clinical failure to accurately predict and identify DCI. Therefore, early prediction and identification of DCI is important in improving the prognosis of patients with poor neurological function.
A number of predictors for DCI have been reported, including the World Federation of Neurological Surgeons (WFNS) grade, Hunt-Hess grade, modified Fisher grade, peripheral monocyte counts, white blood cell counts, and neutrophil/lymphocyte ratios (6-8). Among these, Hunt-Hess grade, WFNS grade, and modified Fisher grade are often used to establish predictive models (9,10). However, in the absence of more quantitative indicators, they do not distinguish the likelihood of DCI among patients at similar risk levels. In recent years, more clinical and laboratory indicators, as well as artificial intelligence methods, have been used to establish a prediction model for DCI, but prediction efficiency has not been significantly improved (11,12). For example, Liu et al. (11) established a predictive nomogram for DCI based on six preoperative risk factors (age >65 years, modified Fisher grade of 3–4, ruptured aneurysm in the anterior circulation, Hunt-Hess grade of 4–5, high blood pressure on admission, and plasma homocysteine level ≥10 μmol/L). In this study, several continuous variables were dichotomized. Furthermore, high blood pressure on admission and plasma homocysteine level ≥10 μmol/L has not been considered as risk factors for DCI in other studies. These might help explain the relatively low C index of the predictive nomogram. On the one hand, this paper demonstrates that nomogram is a feasible method to comprehensively utilize multiple variables to reflect the occurrence probability of DCI, and on the other hand, its relatively low C index indicates that further research is needed on the selection of included risk factors and modeling methods.
Platelets are essential for hemostasis and play an important role in various clotting-related diseases. The functional status of platelets often determines the progression and prognosis of disease. As microthrombi is one of the main causes of DCI and microthrombosis is affected by platelet number and functional state, DCI is inevitably affected by platelet changes. Prodan et al. (13) reported that an increase of coated platelets after aSAH was closely related to the occurrence of DCI and poor prognosis, indicating that platelet activation played an important role in the development of DCI. Mean platelet volume (MPV) is an indicator of function and activation of platelets, and elevated MPV is an independent risk factor for poor prognosis in patients with acute ischemic stroke (14,15). Ray et al. (16) reported that the MPV/platelet count (PLT) ratio after aSAH could predict DCI. Recently, it was reported that elevated MPV was closely related to DCI and poor prognosis in patients with aSAH (17). However, the predictive value of MPV for DCI after aSAH is still controversial (18). At the same time, there are no reports that include early platelet parameters into the comprehensive predictive model of DCI. The purpose of this study was to explore the relationship of early platelet parameters with the development of DCI and develop and validate a quantifiable early-stage predictive model based on platelet parameters. We present the following article in accordance with the TRIPOD reporting checklist (available at https://dx.doi.org/10.21037/atm-21-5200).
Methods
Participants
This study was a retrospective study of data from consecutive aSAH patients admitted within 24 hours after onset at the Affiliated Hospital of North Sichuan Medical College between August 2017 and September 2020. The following criteria were used for inclusion in this study: (I) adult patients with spontaneous SAH; (II) diagnosis based on cranial computed tomography (CT); (III) responsible aneurysm confirmed by CT angiography (CTA) or digital subtraction angiography (DSA); and (IV) admitted to hospital within 24 hours of onset. The exclusion criteria were as follows: (I) comorbidities that would interfere with the outcome assessment and follow-up, such as recent infectious diseases, autoimmune diseases, liver and kidney dysfunction, cardiovascular diseases, hematological disease, and central nervous system diseases, etc.; (II) history of previous use of anticoagulants or antiplatelet drugs; (III) preoperative or postoperative rebleeding; (IV) declined medical intervention; and (VI) insufficient clinical or follow-up data. Patients were managed by qualified neurosurgeons following current guidelines (19). In order to perform internal validation, we split the data into a training set (n=214) and validation set (n=71) based on admission time. We constructed the prediction model with the training set and validated it on the validation set. All procedures performed in this study involving human participants were in accordance with the Declaration of Helsinki (as revised in 2013). The Ethics Committees of the Affiliated Hospital of North Sichuan Medical College approved the study (No. 2021ER126-1). Since this study involved chart review with no risk to patients, patient consent was waived.
Outcomes
Patients were categorized into a DCI group and non-DCI group based on whether DCI occurred during hospitalization. DCI was defined as the detection of any new focal neurological impairment or when there was a minimum 2-point decrease in the Glasgow Coma Scale. The impairment had to last for at least 1 hour, not be apparent immediately after aneurysm occlusion, and not be attributed to other causes through clinical assessment or laboratory tests (20). DCI was identified by two independent reviewers. Any disagreement was resolved through cooperative discussion. A blind method was not adopted.
Clinical and laboratory variables
Patient baseline information was collected, including age, gender, medical history, clinical status on admission (Hunt-Hess grade and WFNS grade), modified Fisher grade, location of aneurysms, number of aneurysms, and treatment method. Medical history included hypertension, diabetes mellitus, previous stroke, Warfarin use, smoking, and drinking. Results of the immediate laboratory tests at admission were also collected, including white blood cell (WBC), red blood cell (RBC), hemoglobin (HGB), PLT, MPV, platelet distribution width (PDW), and plateletcrit (PCT). All enrolled patients underwent whole blood cell test with the same hematology analyzer (BC-6800 Mindray, China). For interpretation purposes, parameters were divided as follows: Hunt-Hess grade as “low grade” (grades 1–3)or “high grade” (grades 4–5), WFNS grade as “low grade” (grades 1–3) or “high grade” (grades 4–5), aneurysm location as “anterior circulation” or “posterior circulation”, number of aneurysms as “single” or “multiple”, and treatment method as “coiling” or “clipping”. All of the above parameters were recorded by investigators with no knowledge of the patient’s outcome.
Sample size
Since the results of platelet parameter detection are affected by detection methods, equipment, and timing, we collected all available data with similar detection conditions to maximize the power and generalizability of the results. Because we excluded all cases with missing information, this is a complete case analysis.
Statistical analysis
Statistical analysis was performed using IBM SPSS Statistics (version 22.0, Chicago, IL, USA) and the R programming language (version 3.4.1, Vienna, Austria). Continuous variables are expressed as mean ± standard deviation, and categorical variables are presented as numbers and percentages. Important baseline data, reported variables with independent predictive value, and blood cell analysis parameters were extracted from the database as possible predictors. Due to the different definitions of DCI used in previous studies, the value of predictors in the existing literature was limited (20). Therefore, we performed a univariate analysis of all possible predictors before modeling to screen appropriate predictors. Pearson’s chi-squared test, independent-samples t-test, and linear correlation test were performed in univariate analysis to determine differences in parameters between two groups. If P value was <0.05, statistical significance was assumed. Factors found to be significant (P<0.05) in univariate analysis were included in the subsequent multivariate logistic regression with backward stepwise selection to identify the independent risk variables associated with DCI and develop the predictive model.
We developed a graphical and quantitative rating predictive nomogram that allowed for simultaneous consideration of multiple variables including the established staging system. The established nomogram was validated internally in different cases from the same database. We also assessed internal validity with a bootstrapping procedure for a realistic estimate of the performance of the nomogram in similar future patients. The discriminative ability of the nomogram was summarized by receiver operating characteristic curve (ROC). The calibration curve was used to analyze the agreement between the nomogram and the ideal observation. Calibration plots on the slope of the 45-degree line were considered an excellent model. Decision curve analysis was conducted to determine the clinical value of the nomogram.
Results
Characteristics of participants
Data of 865 aSAH patients were obtained from the inpatient management system. After strict screening based on the inclusion and exclusion criteria, a total of 285 patients, including 84 males (29.5%) and 201 females (70.5%), were enrolled in the current study (Figure 1). The mean age was 60.0±10.8 years and 157 patients (55.1%) had a history of hypertension. A history of smoking, alcohol consumption, and diabetes was found in 54 (18.95%), 39 (13.7%), and 6 patients (2.1%), respectively. The number of patients with low Hunt-Hess grade and high Hunt-Hess grade were 68 (23.9%) and 217 (76.1%), respectively. The WFNS grade was high in 203 (71.2%) and low in 82 patients (28.8%). The distribution of modified Fisher grade in all patients is as follow: 61 (21.4%), 66 (23.2%), 63 (22.1%), and 95 patients (33.3%) with grade I, grade II, grade III, and grade IV, respectively. Anterior circulation aneurysms ruptured in 269 cases (94.4%), and 60 patients (21.1%) had multiple aneurysms. A total of 133 patients (46.7%) received endovascular coiling and 152 (53.3%) received surgical clipping. The mean WBC, RBC, HGB, PLT, MPV, PCT, and PDW were (12.9±4.5)×109/L, (4.3±0.6)×1012/L, 128.3±16 g/L, (178.3±50.6)×109/L, 11.6±1.7 fl, 0.2±0.05 ng/mL, and 16.2%±1.4%, respectively. Sixty-six (23.16%) of the patients exhibited DCI during hospitalization and were included in the DCI group while the others were enrolled into the non-DCI group. The clinical characteristics of DCI patients and non-DCI patients are presented and compared in Table 1.
Table 1
Characteristics | Overall (n=285) | DCI (n=66) | Non-DCI (n=219) | P |
---|---|---|---|---|
Age (y) | 60.0±10.8 | 61.0±11.1 | 59.8±10.7 | 0.409a |
Sex, n (%) | 0.889b | |||
Male | 84 (29.5) | 19 (28.8) | 65 (29.7) | |
Female | 201 (70.5) | 47 (71.2) | 154 (70.3) | |
Smoking, n (%) | 0.210b | |||
Yes | 54 (18.9) | 16 (24.2) | 38 (17.4) | |
No | 231 (81.1) | 50 (75.8) | 181 (82.6) | |
Drinking, n (%) | 0.990b | |||
Yes | 39 (13.7) | 9 (13.6) | 30 (13.7) | |
No | 246 (86.3) | 57 (86.4) | 189 (86.3) | |
Diabetes, n (%) | 0.703b | |||
Yes | 6 (2.1) | 1 (1.5) | 5 (2.3) | |
No | 279 (97.9) | 65 (98.5) | 214 (97.7) | |
Hypertension, n (%) | 0.456b | |||
Yes | 157 (55.1) | 39 (59.1) | 118 (53.9) | |
No | 128 (44.9) | 27 (40.9) | 101 (46.1) | |
Hunt-Hess grade, n (%) | 0.676c | |||
Low grade | 68 (23.9) | 16 (24.2) | 52 (23.7) | |
High grade | 217 (76.1) | 50 (75.8) | 167 (76.3) | |
WFNS grade, n (%) | 0.851c | |||
High grade | 203 (71.2) | 47 (71.2) | 156 (71.2) | |
Low grade | 82 (28.8) | 19 (28.8) | 63 (28.8) | |
Modified Fisher grade, n (%) | 0.011c* | |||
I | 61 (21.4) | 9 (13.6) | 52 (23.8) | |
II | 66 (23.2) | 11 (16.7) | 55 (25.1) | |
III | 63 (22.1) | 18 (27.3) | 45 (20.5) | |
IV | 95 (33.3) | 28 (42.4) | 67 (30.6) | |
Location, n (%) | 0.267b | |||
Anterior circulation | 269 (94.4) | 64 (97.0) | 205 (93.6) | |
Posterior circulation | 16 (5.6) | 2 (3.0) | 14 (6.4) | |
Number of aneurysms, n (%) | 0.157b | |||
Single | 225 (78.9) | 48 (72.7) | 177 (80.8) | |
Multiple | 60 (21.1) | 18 (27.3) | 42 (19.2) | |
Surgery, n (%) | 0.117b | |||
Clipping | 152 (53.3) | 40 (60.6) | 112 (51.1) | |
Coiling | 133 (46.7) | 26 (39.4) | 107 (48.9) | |
WBC (109/L) | 12.9±4.5 | 13.2±4.5 | 12.8±4.5 | 0.467a |
RBC (1012/L) | 4.3±0.6 | 4.2±0.5 | 4.4±0.6 | 0.039a* |
HGB (g/L) | 128.3±16 | 127.0±16.1 | 128.7±16.0 | 0.450a |
PLT (109/L) | 178.3±50.6 | 168.1±44.8 | 181.4±51.9 | 0.041a |
MPV (fl) | 11.6±1.7 | 12.7±1.5 | 11.3±1.6 | <0.001a* |
PCT (ng/mL) | 0.2±0.05 | 0.2±0.1 | 0.2±0.1 | 0.737a |
PDW (%) | 16.2±1.4 | 16.6±1.3 | 16.1±1.4 | 0.012a* |
*, P<0.05; a, independent-samples t-test; b, Pearson’s chi-squared test; c, linear correlation test. DCI, delayed cerebral ischemia; WFNS, World Federation of Neurological Surgeons; WBC, white blood cell; RBC, red blood cell; HGB, hemoglobin; PLT, platelet count; MPV, mean platelet volume; PCT, plateletcrit; PDW, platelet distribution width.
Risk factors for DCI
The results of univariate analysis revealed that the factors associated with DCI were the modified Fisher grade, RBC, MPV, PLT, and PDW (P<0.05). There was no significant difference in sex, age, smoking, drinking, diabetes, hypertension, Hunt-Hess grade, WFNS grade, aneurysm location, number of aneurysms, treatment, WBC, HGB, or PCT (P>0.05) (Table 1). Multivariate logistic regression analysis demonstrated that the independent risk factors for DCI were modified Fisher grade [odds ratio (OR) =1.354; 95% confidence interval (CI): 1.034–1.773; P=0.028] and MPV (OR =1.825; 95% CI: 1.429–2.331; P<0.001) (Table 2). Given that the predictive value of modified Fisher grade for DCI after aSAH has been verified many times, we only evaluated the predictive value of early MPV (11,15). ROC curve analysis demonstrated that MPV could serve as a predictor of DCI, with an area under the curve (AUC) of 0.740 (95% CI: 0.674–0.806; P<0.001, Figure 2). The value of 11.95 fl was selected as the best cut-off value for MPV to evaluate whether DCI occurs. For this cut-off value, MPV had a sensitivity of 67.1% and specificity of 74.2% (Figure 2).
Table 2
Characteristics | Univariate analysis | Multivariate analysis | |||
---|---|---|---|---|---|
OR (95% CI) | P | OR (95% CI) | P | ||
Modified Fisher grade (I/II/III/IV) | 1.380 (1.074–1.774) | 0.012* | 1.354 (1.034–1.773) | 0.028* | |
RBC (1012/L) | 0.568 (0.332–0.972) | 0.039* | 0.579 (0.313–1.072) | 0.082 | |
PLT (109/L) | 0.994 (0.989–1.000) | 0.042* | 1.005 (0.998–1.012) | 0.179 | |
MPV (fl) | 1.710 (1.412–2.072) | <0.001* | 1.825 (1.429–2.331) | <0.001* | |
PDW (%) | 1.341 (1.060–1.695) | 0.014* | 1.061 (0.831–1.355) | 0.635 |
*, P<0.05. OR, odds ratio; CI, confidence interval; RBC, red blood cell count; PLT, platelet count; MPV, mean platelet volume; PCT, plateletcrit; PDW, platelet distribution width.
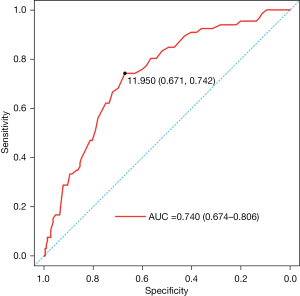
Development of nomogram
Five clinical features from the univariate analysis were used to establish the nomogram, including modified Fisher score, RBC, MPV, PDW, and PLT. The nomogram allowed for an estimation of the individual risk of DCI (Figure 3). A total number of points were calculated based on modified Fisher grade, MPV, PLT, PDW, and RBC. By projecting the total points to the lower total points scale, we were able to predict the probability of DCI and also reveal the contribution of each factor to the overall risk for DCI. For example, the probability of DCI is 80% for an aSAH patient with modified Fisher grade III, MPV 14 fl, PDW 18%, platelet 260×109/L, and RBC 4.0×1012/L. Calculations were made as follows: on the basis of the location of each factor in the nomogram, 20 points were given for “modified Fisher grade III”, 74 for “MPV =14 fl”, 18 for “PDW =18%”, 20 for “platelet =260×109/L”, and 28 for “RBC =4.0×1012/L”; a total score of 160 points was then obtained by adding these points together and; 160 points is equivalent to a probability of approximately 80% for DCI.
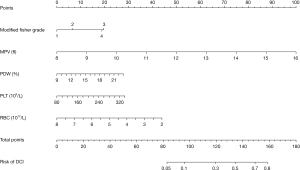
Validation of nomogram
The AUC of the nomogram in the training set and the validation set were 0.799 (95% CI: 0.737–0.861) and 0.783 (95% CI: 0.616–0.949), respectively, indicating that the nomogram had a good degree of differentiation (Figure 4). The calibration curve constructed by bootstrapping with 1,000 resamples showed that the nomogram had good calibration in both the training set and validation set (Figure 5). In the training set and validation set, decision curve analysis showed that the nomogram had good clinical application value and could assist in clinical decision making (Figure 6).
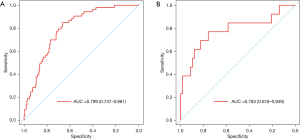
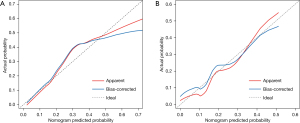
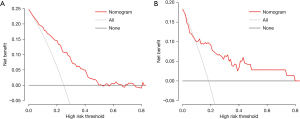
Discussion
In this study, we investigated the relationship between early-stage (within 24 hours of onset) platelet parameters and DCI in 285 aSAH patients from a single center. We then developed and internally validated a predictive nomogram for DCI. The main findings of this study included: (I) a higher level of MPV within 24 hours of onset was independently correlated with the occurrence of DCI after aSAH; (II) ROC curve analysis showed that MPV has an ability to predict the occurrence of DCI after aSAH, and the area under the curve was 0.74; and (III) a quantifiable nomogram based on early platelet parameters is more effective in predicting the occurrence of DCI after aSAH than MPV alone. The model had a high degree of differentiation and calibration as well as good clinical application value.
It has been reported that DCI occurs in approximately 30% of patients surviving the initial hemorrhage (21,22). In the present study, the incidence of DCI was 23.16% in 285 consecutive patients with aSAH, which was consistent with previous studies and indicated that our data was representative. As microthrombi are one of the main causes of DCI and microthrombosis is affected by platelet number and functional state, DCI is inevitably affected by platelet changes (23,24). Previous studies have shown that platelet parameters were associated with the occurrence of DCI and some platelet parameters were able to predict DCI independently or collectively (13-17,25). Our univariate analysis illustrated that PLT, MPV, and PDW were all related to DCI, which further proved that platelet parameters were closely related to DCI. However, in multivariate logistic regression analysis, only MPV was independently correlated with DCI, indicating that there was mutual influence among platelet parameters.
MPV is an index of platelet size that correlates with the function and activation of platelets and is associated with the clinical outcomes of myocardial infarction, acute ischemic stroke, and hemorrhagic stroke (14,15,26). Chen et al. (17,27) reported that within 9 days of SAH, patients with DCI had significantly higher MPV than those without DCI and that in DCI patients, MPV first increased and then decreased. In addition, MPV at 3–5 days was an independent predictor for DCI. These results indicated that MPV was associated with the development of DCI. However, since MPV shows a trend of dynamic change after aSAH, it is unclear how MPV can be effectively used to predict DCI. It is also possible that patients had undergone a clipping or embolization within 3–5 days, which may have affected the MPV level, or the patient may have developed DCI prior to 3 days after aSAH. Therefore, although MPV observed at 3–5 days had the best predictive effect, it was not the best time for predicting DCI. In order to identify, as early as possible, high-risk patients who may develop DCI after aSAH and implement effective interventions, it is important to evaluate the relationship between early platelet parameters and the occurrence of DCI. In this study, we only included patients admitted within 24 hours of onset in order to evaluate the relationship between early MPV and DCI more rigorously and to establish an early predictive model to predict DCI sooner. Our results confirmed that early elevation of MPV was an independent risk factor for DCI in aSAH patients, which is consistent with a previous report (27). With an appropriate cut-off value for MPV, we were able to predict whether patients were at high risk of DCI.
It has been reported that the value of MPV is affected by many factors, such as detection timing, detection instrument, and the anticoagulant contained in the blood tube (28). Additionally, increased MPV can be observed in cardiovascular diseases, cerebral stroke, respiratory diseases, chronic renal failure, intestine diseases, rheumatoid diseases, diabetes, and various cancers (29). For these reasons, there have been doubts among some researchers about the predictive value of MPV (18). It is important when evaluating the relationship between MPV and DCI that, as far as possible, the above factors are accounted for. In the present study, we enrolled aSAH patients who were admitted within 24 hours of onset at the same hospital. For acute hemorrhagic stroke, routine blood tests need to be completed within hour of admission, which ensured that the blood test time had little effect on the MPV value. In addition, we used the same blood cell analyzer to avoid the influence of different testing equipment on the results. Further, we excluded patients with the above-mentioned underlying diseases and those with a history of anticoagulation and antiplatelet use so as to avoid the influence of underlying diseases and drugs. For these reasons, the MPV data obtained in this study was considered reliable and the relationship between MPV and DCI objective. Although MPV changes dynamically after aSAH and is affected by surgery, drugs, and disease complications, MPV values detected immediately after admission is rarely affected by these factors. Therefore, while the results only reflected the relationship between MPV and DCI within 24 hours of the onset of aSAH, early MPV was a stable and reliable indicator for predicting DCI after aSAH.
Our results showed that MPV had a certain degree of differentiation in predicting DCI. However, MPV was only able to make a judgment on high or low level of risk, not provide a quantitative possibility of DCI. Further, as MPV is influenced by other factors, its clinical usefulness has limits. In order to comprehensively evaluate the influence of various factors on DCI, including MPV, a multifactor predictive model is needed.
Previous models for predicting DCI were mostly based on clinical and imaging data, combined with some laboratory data, and the majority of results were presented in the form of risk classification (9,10,30). As a multifactor graphical predictive tool, the advantage of a nomogram is that it can provide quantifiable probability and also reflect the contribution of each included factor to the prediction of DCI. Given that this study involved different inclusion criteria and evaluation purposes, predictors from other studies had limited reference value. Therefore, we established a nomogram based on univariate analysis rather than referring to previous research. The AUC of our nomogram in both the training set (0.799; 95% CI: 0.737–0.861) and validation set (0.783; 95% CI: 0.616–0.949) were higher than MPV (0.740; 95% CI: 0.674–0.806). This indicated the nomogram had more robust discriminatory ability than MPV alone. The predicted and actual probabilities of DCI were compared in a calibration diagram. The calibration curve showed that the predicted probability of DCI was consistent with the actual probability. Finally, decision curve analysis of the training set and validation set showed that patients predicted to be at high risk for DCI would benefit from effective intervention. This indicated that the nomogram had good clinical application value and could assist in clinical decision making.
So far, the prevention and treatment of DCI remain a crucial and complex issue in the management of aSAH. Prevention of DCI mainly relies on administration of nimodipine and optimization of blood volume and cardiac function. To date, nimodipine is the only pharmacological treatment to reduce development of DCI (31). And it was suggested that nimodipine should be given to all aSAH patients for the duration of hospital stay (19). However, in the case of rational use of nimodipine, the incidence of DCI obtained in recent years is still not optimistic. For patients who already present with DCI, induced hypertension is a first-line therapy while hemodilution and hypervolemia have fallen out of favor (5,32). Other therapies such as hemoglobin optimization, endovascular therapy, and cardiac output augmentation have also been applied individually (33-35). However, the clinical application value of these therapies is still controversial due to many complications and inexact effects (36). Antiplatelet therapy is mainly used for aSAH patients after stent-assisted aneurysm embolization, and it has been reported that antiplatelet therapy can reduce the incidence of DCI (24). Therefore, the potential clinical significance of our results is not only that platelet parameters can be used to identify people at high risk of DCI, but also that appropriate antiplatelet therapy may have the potential to prevent the occurrence of DCI after aSAH.
Our study had some inevitable limitations. First, the retrospective nature may have led to unavoidable selection bias. Second, the definition of DCI, especially as applied to patients who were comatose or sedated, may have excluded some patients who developed DCI. Third, due to the limitations of the inclusion criteria, the established nomogram is not applicable to data collected over 24 hours after onset and patients with specific diseases. Finally, our nomogram needs to be validated externally in other databases due to the inclusion indicators and epidemiological differences.
Conclusions
In conclusion, the present study suggested that MPV could be an early predictor for DCI in aSAH patients. A nomogram based on early platelet parameters quantitatively predicted the individual risk of DCI of aSAH patients and could assist in clinical decision making.
Acknowledgments
The authors are thankful to the enrolled patients, who made data on aSAH available.
Funding: This work was supported by the Funds for Cooperation Project of Nanchong City and North Sichuan Medical College, Grant No. 20SXKT0317 (to LZ), National Major Scientific and Technological Special Project for Significant New Drugs Development, Grant Number: 2019ZX09301-147 (to LZ) and Key Project of Affiliated Hospital of North Sichuan Medical College, Grant No. 2020ZD018 (to LZ).
Footnote
Reporting Checklist: The authors have completed the TRIPOD reporting checklist. Available at https://dx.doi.org/10.21037/atm-21-5200
Data Sharing Statement: Available at https://dx.doi.org/10.21037/atm-21-5200
Conflicts of Interest: All authors have completed the ICMJE uniform disclosure form (available at https://dx.doi.org/10.21037/atm-21-5200). The authors have no conflicts of interest to declare.
Ethical Statement: The authors are accountable for all aspects of the work in ensuring that questions related to the accuracy or integrity of any part of the work are appropriately investigated and resolved. All procedures performed in this study involving human participants were in accordance with the Declaration of Helsinki (as revised in 2013). The Ethics Committees of the Affiliated Hospital of North Sichuan Medical College approved the study (No. 2021ER126-1). Since this study involved chart review with no risk to patients, patient consent was waived.
Open Access Statement: This is an Open Access article distributed in accordance with the Creative Commons Attribution-NonCommercial-NoDerivs 4.0 International License (CC BY-NC-ND 4.0), which permits the non-commercial replication and distribution of the article with the strict proviso that no changes or edits are made and the original work is properly cited (including links to both the formal publication through the relevant DOI and the license). See: https://creativecommons.org/licenses/by-nc-nd/4.0/.
References
- Ridwan S, Urbach H, Greschus S, et al. Health Care Costs of Spontaneous Aneurysmal Subarachnoid Hemorrhage for Rehabilitation, Home Care, and In-Hospital Treatment for the First Year. World Neurosurg 2017;97:495-500. [Crossref] [PubMed]
- Rowland MJ, Hadjipavlou G, Kelly M, et al. Delayed cerebral ischaemia after subarachnoid haemorrhage: looking beyond vasospasm. Br J Anaesth 2012;109:315-29. [Crossref] [PubMed]
- Geraghty JR, Testai FD. Delayed Cerebral Ischemia after Subarachnoid Hemorrhage: Beyond Vasospasm and Towards a Multifactorial Pathophysiology. Curr Atheroscler Rep 2017;19:50. [Crossref] [PubMed]
- Megjhani M, Terilli K, Weiss M, et al. Dynamic Detection of Delayed Cerebral Ischemia: A Study in 3 Centers. Stroke 2021;52:1370-9. [Crossref] [PubMed]
- Francoeur CL, Mayer SA. Management of delayed cerebral ischemia after subarachnoid hemorrhage. Crit Care 2016;20:277. [Crossref] [PubMed]
- de Oliveira Manoel AL, Jaja BN, Germans MR, et al. The VASOGRADE: A Simple Grading Scale for Prediction of Delayed Cerebral Ischemia After Subarachnoid Hemorrhage. Stroke 2015;46:1826-31. [Crossref] [PubMed]
- Mahta A, Azher AI, Moody S, et al. Association of Early White Blood Cell Trend with Outcomes in Aneurysmal Subarachnoid Hemorrhage. World Neurosurg 2021;151:e803-9. [Crossref] [PubMed]
- Al-Mufti F, Amuluru K, Damodara N, et al. Admission neutrophil-lymphocyte ratio predicts delayed cerebral ischemia following aneurysmal subarachnoid hemorrhage. J Neurointerv Surg 2019;11:1135-40. [Crossref] [PubMed]
- de Rooij NK, Greving JP, Rinkel GJ, et al. Early prediction of delayed cerebral ischemia after subarachnoid hemorrhage: development and validation of a practical risk chart. Stroke 2013;44:1288-94. [Crossref] [PubMed]
- Foreman PM, Chua MH, Harrigan MR, et al. External validation of the Practical Risk Chart for the prediction of delayed cerebral ischemia following aneurysmal subarachnoid hemorrhage. J Neurosurg 2017;126:1530-6. [Crossref] [PubMed]
- Liu H, Xu Q, Li A. Nomogram for predicting delayed cerebral ischemia after aneurysmal subarachnoid hemorrhage in the Chinese population. J Stroke Cerebrovasc Dis 2020;29:105005 [Crossref] [PubMed]
- de Jong G, Aquarius R, Sanaan B, et al. Prediction Models in Aneurysmal Subarachnoid Hemorrhage: Forecasting Clinical Outcome With Artificial Intelligence. Neurosurgery 2021;88:E427-34. [Crossref] [PubMed]
- Prodan CI, Vincent AS, Kirkpatrick AC, et al. Higher levels of coated-platelets are observed in patients with subarachnoid hemorrhage but lower levels are associated with increased mortality at 30 days. J Neurol Sci 2013;334:126-9. [Crossref] [PubMed]
- Varasteh-Ravan HR, Ali-Hasan-Al-Saegh S, Shokraneh S, et al. Relationship of admission mean platelet volume, platelet distribution width and white blood cells with ST resolution in patients with acute ST segment elevation myocardial infarction treated with streptokinase without history of previous cardiovascular surgery. Perspect Clin Res 2013;4:125-9. [Crossref] [PubMed]
- Staszewski J, Pogoda A, Data K, et al. The mean platelet volume on admission predicts unfavorable stroke outcomes in patients treated with IV thrombolysis. Clin Interv Aging 2019;14:493-503. [Crossref] [PubMed]
- Ray B, Tinsley L, Ford L, et al. Trends of Platelet Volume Index Predicts Delayed Cerebral Ischemia After Subarachnoid Hemorrhage. World Neurosurg 2018;111:e624-31. [Crossref] [PubMed]
- Chen L, Zhang Q. Dynamic Change in Mean Platelet Volume and Delayed Cerebral Ischemia After Aneurysmal Subarachnoid Hemorrhage. Front Neurol 2020;11:571735 [Crossref] [PubMed]
- Beyan C, Beyan E. Mean Platelet Volume may not be a Marker for Prognosis in Patients with Aneurysmal Subarachnoid Hemorrhage. J Stroke Cerebrovasc Dis 2021;30:105492 [Crossref] [PubMed]
- Connolly ES Jr, Rabinstein AA, Carhuapoma JR, et al. Guidelines for the management of aneurysmal subarachnoid hemorrhage: a guideline for healthcare professionals from the American Heart Association/american Stroke Association. Stroke 2012;43:1711-37. [Crossref] [PubMed]
- Vergouwen MD, Vermeulen M, van Gijn J, et al. Definition of delayed cerebral ischemia after aneurysmal subarachnoid hemorrhage as an outcome event in clinical trials and observational studies: proposal of a multidisciplinary research group. Stroke 2010;41:2391-5. [Crossref] [PubMed]
- Lissak IA, Locascio JJ, Zafar SF, et al. Electroencephalography, Hospital Complications, and Longitudinal Outcomes After Subarachnoid Hemorrhage. Neurocrit Care 2021; Epub ahead of print. [Crossref] [PubMed]
- Suzuki H, Kanamaru H, Kawakita F, et al. Cerebrovascular pathophysiology of delayed cerebral ischemia after aneurysmal subarachnoid hemorrhage. Histol Histopathol 2021;36:143-58. [PubMed]
- Ohkuma H, Suzuki S, Kimura M, et al. Role of platelet function in symptomatic cerebral vasospasm following aneurysmal subarachnoid hemorrhage. Stroke 1991;22:854-9. [Crossref] [PubMed]
- Darkwah Oppong M, Gembruch O, Pierscianek D, et al. Post-treatment Antiplatelet Therapy Reduces Risk for Delayed Cerebral Ischemia due to Aneurysmal Subarachnoid Hemorrhage. Neurosurgery 2019;85:827-33. [Crossref] [PubMed]
- Ray B, Pandav VM, Mathews EA, et al. Coated-Platelet Trends Predict Short-Term Clinical OutcomeAfter Subarachnoid Hemorrhage. Transl Stroke Res 2018;9:459-70. [Crossref] [PubMed]
- Sadeghi F, Kovács S, Zsóri KS, et al. Platelet count and mean volume in acute stroke: a systematic review and meta-analysis. Platelets 2020;31:731-9. [Crossref] [PubMed]
- Chen L, Zhang Q. Increased Mean Platelet Volume is Associated with Poor Outcome in Patients with Aneurysmal Subarachnoid Hemorrhage. World Neurosurg 2020;137:e118-25. [Crossref] [PubMed]
- Noris P, Melazzini F, Balduini CL. New roles for mean platelet volume measurement in the clinical practice? Platelets 2016;27:607-12. [Crossref] [PubMed]
- Korniluk A, Koper-Lenkiewicz OM, Kamińska J, et al. Mean Platelet Volume (MPV): New Perspectives for an Old Marker in the Course and Prognosis of Inflammatory Conditions. Mediators Inflamm 2019;2019:9213074 [Crossref] [PubMed]
- Unda SR, Birnbaum J, Labagnara K, et al. Peripheral Monocytosis at Admission to Predict Cerebral Infarct and Poor Functional Outcomes in Subarachnoid Hemorrhage Patients. World Neurosurg 2020;138:e523-9. [Crossref] [PubMed]
- Allen GS, Ahn HS, Preziosi TJ, et al. Cerebral arterial spasm--a controlled trial of nimodipine in patients with subarachnoid hemorrhage. N Engl J Med 1983;308:619-24. [Crossref] [PubMed]
- Reynolds MR, Buckley RT, Indrakanti SS, et al. The safety of vasopressor-induced hypertension in subarachnoid hemorrhage patients with coexisting unruptured, unprotected intracranial aneurysms. J Neurosurg 2015;123:862-71. [Crossref] [PubMed]
- Kurtz P, Helbok R, Claassen J, et al. The Effect of Packed Red Blood Cell Transfusion on Cerebral Oxygenation and Metabolism After Subarachnoid Hemorrhage. Neurocrit Care 2016;24:118-21. [Crossref] [PubMed]
- Hollingworth M, Chen PR, Goddard AJ, et al. Results of an International Survey on the Investigation and Endovascular Management of Cerebral Vasospasm and Delayed Cerebral Ischemia. World Neurosurg 2015;83:1120-1126.e1. [Crossref] [PubMed]
- Kurtz P, Helbok R, Ko SB, et al. Fluid responsiveness and brain tissue oxygen augmentation after subarachnoid hemorrhage. Neurocrit Care 2014;20:247-54. [Crossref] [PubMed]
- Festic E, Rabinstein AA, Freeman WD, et al. Blood transfusion is an important predictor of hospital mortality among patients with aneurysmal subarachnoid hemorrhage. Neurocrit Care 2013;18:209-15. [Crossref] [PubMed]
(English Language Editor: A. Muijlwijk)