Radiomic signatures based on multiparametric MR images for predicting Ki-67 index expression in medulloblastoma
Introduction
Medulloblastoma (MB), an embryonal tumor of the cerebellum, is the most common malignant brain tumor in childhood (WHO grade IV) (1). MB is suspected to originate from various discrete neuronal stem cells or progenitor cell populations in the early stages of life (1). Patients with MB will show symptoms and signs of high intracranial pressure and cerebellar dysfunction. Some patients have poor therapeutic effects and prognoses due to the tumor’s high heterogeneity and strong invasiveness.
The Ki-67 index is a clinically important proliferation marker used to classify various cancers (2,3). In general, overexpression of the Ki-67 index indicates rapid growth and the malignant transformation of cells, and a poor prognosis (4). The Ki-67 index can be regarded as a valuable independent prognostic biomarker for patients with MB. Patients with a Ki-67 index greater than 30% have worse overall survival (OS) and progression-free survival (PFS) than those with a Ki-67 index less than 30% (5). This finding suggests that the Ki-67 index should be incorporated into the prognostication of patients with MB. However, information on the Ki-67 index can only be obtained by invasive procedures, including surgery or biopsy, followed by immunohistochemical methods in the laboratory.
Magnetic resonance imaging (MRI) is formed by reconstructing the signal generated by the resonance of hydrogen nuclei in a magnetic field. It is a conventional non-invasive technique for evaluating MB. There are multiple parametric images in MRI, including T1 weighted image (T1WI), T2 weighted image (T2WI), diffusion weighted image (DWI), water suppression image (FLAIR) and so on. The signal performance of the same tissue on different weighted images is different, which results in a variety of different MRI images for the same patient. We analyze and integrate the patient’s multi-parameter images to obtain tumor information non-invasively. However, it is very difficult to judge the expression of Ki-67 index directly on the MRI images of MB patients, especially the result of diagnosis.
Radiomics is a novel high-throughput method that can noninvasively retrieve comprehensive information within, between, and around tumors (6,7); this method has been the subject of extensive medical research in recent years. Use machine learning methods to explore information that is invisible to the naked eye and transform it into quantitative research to predict the classification and prognosis of clinical patients. Radiomics can partially predict the expression of the Ki-67 index in certain tumors, including breast cancer, liver cancer, and low-grade glioma (8-11). Using radiomics to predict Ki-67 has gradually increased, especially in central nervous system tumors, but has not been used in MB (12). In MB, there were several studies that predict molecular typing, but these were only a classification for diagnosis.
This study aimed to predict and classify the expression level of the Ki-67 index through multiparameter MRI-based imaging omics research. The results will be helpful for clinicians, allowing them to noninvasively determine the expression of Ki-67 and effectively judge the prognosis of the disease. Intensive anti-tumor therapy should be given for patients with high prognosis risk, which has more important significance in clinical application. We present the following article in accordance with the STARD reporting checklist (available at https://dx.doi.org/10.21037/atm-21-5348).
Methods
All procedures performed in this study involving human participants were in accordance with the Declaration of Helsinki (as revised in 2013). The study was approved by the Medical Ethics Committee of Beijing Tiantan Hospital, Capital Medical University (No. YW2018-022-08). Since this study is a retrospective analysis and the patients have been anonymously processed, the patient's informed consent is not required.
Patient selection
We retrospectively collected the clinical information and MRI data of all patients diagnosed with MB who underwent surgical treatment in Beijing Tiantan Hospital from January 2016 to December 2019. The pathology complied with the pathological diagnosis of 2016 WHO “Central Nervous System Tumor Classification”.
The inclusion criteria were as follows: (I) patients with available pathology (after resection); (II) patients with preoperative MRI data (see Figures 1,2); (III) patients with proven expression of the Ki-67 index by immunohistochemistry; Ki-67 index greater than or equal to 30% is defined as high expression, and Ki-67 index less than 30% is defined as low expression (see Figures 3,4); (IV) patients aged less than 18 years. A total of 271 patients were screened, and 181 patients were excluded due to the following reasons: (I) patients lacking Ki-67 expression by immunohistochemistry (n=96); (II) patients lacking at least one of the following MRI sequences: T1-weighted images (T1W), T2-weighted images (T2W), and contrast-enhanced T1-weighted images (CE-T1W) (n=71); and (III) patients with MR images that had motion or other kinds of artifacts that may affect subsequent segmentation and analysis (n=14). Finally, 90 subjects met the requirements and were included in this study (22 cases of Ki-67 index ≤30 and 68 cases of Ki-67 index >30). Clinical information (age and sex) and tumor characteristics (location, pathological type, and molecular type) are summarized in Table 1.
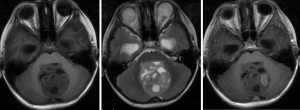
Table 1
Clinical characteristics | Total cases (%) | Ki-67 <30 (%) | Ki-67 >30 (%) | P |
---|---|---|---|---|
Case numbers | 90 | 22 (24.4) | 68 (75.6) | – |
Sex | 0.6697 | |||
Male | 48 (53.3) | 11 (50.0) | 37 (54.4) | |
Female | 42 (46.7) | 11 (50.0) | 30 (45.6) | |
Age | 0.2036 | |||
≤3 | 13 (14.4) | 5 (22.7) | 8 (11.8) | |
>3 | 77 (85.6) | 17 (77.3) | 60 (88.2) | |
Mean [SD] | 9.72 [7.47] | 8.95 [6.39] | 9.97 [7.81] | 0.581 |
Molecular type | 0.5759 | |||
G3 | 3 (6.5) | 0 (0.0) | 3 (9.1) | |
G4 | 21 (45.7) | 5 (41.7) | 15 (45.5) | |
SHH | 13 (14.0) | 3 (25.0) | 10 (30.3) | |
WNT | 9 (9.8) | 4 (33.3) | 5 (15.1) | |
Tumor location | 0.8947 | |||
The fourth ventricle | 49 (53.3) | 11 (50.0) | 37 (54.4) | |
Cerebellar hemisphere | 5 (5.4) | 1 (4.6) | 4 (5.9) | |
Cerebellar vermis | 17 (18.5) | 5 (22.7) | 11 (16.2) | |
Others | 21 (22.8) | 5 (22.7) | 16 (23.5) | |
Pathological type | 0.213 | |||
DN | 17 (19.8) | 5 (23.8) | 12 (19.0) | |
LC/A | 9 (10.5) | 0 (0.0) | 9 (14.3) | |
MBEN | 3 (3.5) | 0 (0.0) | 3 (4.8) | |
CMB | 57 (66.2) | 16 (76.9) | 39 (61.9) |
MR imaging acquisition
MR images were acquired on a 3T MR imaging system (Discovery 750; GE Healthcare, Milwaukee, Wisconsin) using a standard head coil. The imaging protocol for MB included the following sequences: (I) T2W [repetition time/echo time (TR/TE) =4,245/105 ms, field of view (FOV) =24 cm × 24 cm, matrix =192×192, NEX =1.5]; (II) T1W [TR/TE/TI (inversion time) =1,725/25/780 ms, FOV =24 cm × 24 cm, matrix =320×320, NEX =1]; and (III) CE-T1W acquired 2 min after intravenous administration of contrast agents (0.1 mmol/kg). The slice thickness was 5 mm with a 1.5-mm intersection space.
Image preprocessing and standardization
In our experiment, three sequences of T1W, CE-T1W, and T2W were used as test data. Two experienced radiologists (Dr. L and Dr. P, with more than 10 years of experience) manually delineated the volume of interest (VOI) on the T2W images. To save time, we used SPM12 to register T1W and T1WC to T2W. The MRI scanning system automatically calculated the co-registration among the different sequences (Figure 5).
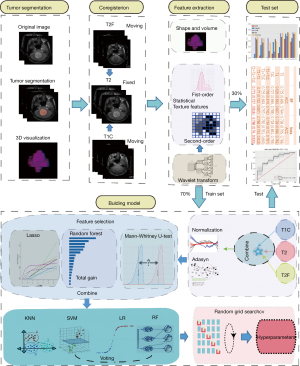
PyRadiomics (13), an open-source toolkit for feature extraction of medical images, standardized image intensity values. Each image was standardized as follows: the average and variance of the image pixels were first calculated, and the average was subtracted from each pixel value of the image and divided by the variance:
Feature extraction
We used PyRadiomics to perform feature extraction in the region of interest (VOI) of each image. We extracted 14 shape features. From nine types of images, 18 first-order statistical features and 73 texture features were extracted (an original image and eight images generated after wavelet change). The first-order statistical features described the changes in the gray level of the image (for example, moment, central moment, absolute moment, etc.). Compared with the first-order features, the second-order statistical texture features could provide the relative position among different gray levels in the image (all the low gray levels are together or cross into the high level). They mainly included 22 gray-level co-occurrence matrix (GLCM) features, 16 grayscale run-length matrices (GLRLM) features, 16 grayscale size area matrix (GLSZM) features, five adjacent grayscale tone difference matrix (GTDM) features, and 14 grayscale dependent matrix (GLDM) features (14,15). A total of 833 [14+(18+73)×9] dimensional features were extracted.
Feature selection
Before the training started, a set of best features was selected for each MR sequence according to the training queue. We used three methods to select the features: (I) hypothesis testing idea using the Mann-Whitney U test, which retained the characteristics that were different between the two categories (P<0.05); (II) information theory using random forest (RF) plots to select features as potential predictors according to the Gini gain of the variable (16); and (III) class separability using LASSO (least absolute shrinkage and selection operator) (17) and adjusting the regular term according to the average AUC in cross-validation. The value of the coefficient lambda and the features with nonzero weights were selected as the best feature group.
To select the most critical features, reduce the redundancy between features and the complexity of the calculations, and increase the stability of the model, we used a combination of three methods to overcome the limitations of a single method and achieved better results.
Feature extraction scheme Plan A: I + II; Plan B: I + III; Plan C: I + II + III.
Classifier modeling
Given that the hypothesis space is unknown, we could not predict which algorithm’s mapping rules were more in line with the corresponding relationship between the input space and the output space in advance. To improve the accuracy of the prediction, we chose four classification models: logistic regression (logistic), RF, K nearest neighbor (KNN), support vector machine (SVM), and a soft voting ensemble model (RF, SVM). A total of five classic models were used for prediction.
We used adaptive oversampling method 16 to generate a small number of samples on the training set to eliminate sample imbalance and overfitting effects. A fivefold cross-validation method combined with a random grid search to select the parameter with the highest average AUC was used as the model parameter. We evaluated the effectiveness of the five models in the test set (ACC, AUC). The averages of 10 random segments and their 95% confidence intervals were used to evaluate the model’s final performance and reduce the random influence of single segmentation.
In addition, we combined the best features of each sequence into a set of multiparameter MRI features to train the classifier and conducted experiments on all possible combinations of the three sequences. The entire experimental process is shown in Figure 5.
Statistical analysis
The chi-square test was performed using SAS (v.9.4) to analyze whether there were statistically significant differences in clinical and tumor information between Ki-67 index high and low groups. Fisher’s exact test were used in the analysis of contingency tables where sample sizes were small. The Mann-Whitney U-test and LASSO were performed using SciPy and Sklearn for feature selection. The level of confidence for all the statistical analyses mentioned above was kept at 95% and results with P<0.05 were significant.
Results
Data description
MRI images of patients with MB were collected from Tiantan Hospital during the past 4 years. MRI images of 90 patients were used in this experiment. The images were divided into two categories by a threshold of 30 for the Ki-67 value. Patients whose Ki-67 value was higher than 30% belonged to the same class, while the remaining patients were in the other class. Sixty-eight images had Ki-67 values greater than 30%, and 22 images had Ki-67 values less than 30%. This experiment randomly selected 30 processed images as training samples and 60 processed images as testing samples.
Clinical characteristics of the study cohort
No significant differences were found in sex, age, tumor location, pathological type, or molecular type between patients with Ki-67 greater than 30% and those with Ki-67 less than 30% (Table 1).
Classification results and analysis
Based on the different combinations of the three feature selection methods, namely, I, Mann-Whitney U-test, II, RF, and III, LASSO, we designed three schemes:
- Plan A (I + II): (see Table 2, Figure 6)
Table 2
The prediction performances of all models in plan A (95% confidence interval)Model Performance T2W T2W + T1C T1W + T1C T2W + T1W T2W + T1W + T1C Logistic ACC 0.586 (0.536, 0.636) 0.691 (0.648, 0.734) 0.646 (0.615, 0.678) 0.764 (0.72, 0.809) 0.725 (0.693, 0.757) AUC 0.61 (0.538, 0.681) 0.682 (0.639, 0.726) 0.657 (0.585, 0.73) 0.784 (0.735, 0.834) 0.752 (0.703, 0.8) KNN ACC 0.554 (0.476, 0.631) 0.588 (0.532, 0.644 0.593 (0.569, 0.616) 0.718 (0.663, 0.773) 0.693 (0.644, 0.742) AUC 0.569 (0.48, 0.658) 0.646 (0.595, 0.698) 0.552 (0.506, 0.599) 0.683 (0.613, 0.752) 0.662 (0.604, 0.72) SVM ACC 0.729 (0.674, 0.784) 0.606 (0.519, 0.693) 0.768 (0.723, 0.812) 0.743 (0.713, 0.773) 0.775 (0.745, 0.805) AUC 0.606 (0.506, 0.706) 0.739 (0.7, 0.779) 0.652 (0.594, 0.71) 0.757 (0.697, 0.818) 0.754 (0.678, 0.83) RF ACC 0.714 (0.669, 0.76) 0.716 (0.668, 0.765) 0.736 (0.687, 0.784) 0.777 (0.738, 0.815) 0.761 (0.735, 0.786) AUC 0.654 (0.586, 0.722) 0.77 (0.738, 0.801) 0.722 (0.665, 0.78) 0.808 (0.769, 0.848) 0.74 (0.687, 0.793) Vote ACC 0.743 (0.705, 0.78) 0.697 (0.632, 0.761) 0.771 (0.727, 0.816) 0.768 (0.742, 0.794) 0.775 (0.755, 0.795) AUC 0.66 (0.586, 0.734) 0.761 (0.724, 0.797) 0.712 (0.65, 0.775) 0.804 (0.773, 0.836) 0.752 (0.7, 0.803) ACC, accuracy; AUC, area under curve.
RF showed the best performance, reaching the highest average accuracy rate of 0.777 (0.738, 0.815) and AUC of 0.808 (0.769, 0.848) on the T2W + T1W sequence. The performance of the classification model and SVM after the integration of the two strong classifiers was slightly lower than that of the RF, and both reached the highest average accuracy on the T2W + T1W + T1WC sequence: ACC: 0.775 (0.755, 0.795), AUC: 0.752 (0.7, 0.803) and ACC: 0.775 (0.745, 0.805) AUC: 0.754 (0.687, 0.83). Compared to the other sequences, the T2W sequence had better effects on different classifications.
- Plan B (I + III): (see Table 3, Figure 7)
Table 3
The prediction performances of all models in plan B (95% confidence interval)Model Performance T2W T2W + T1C T1W + T1C T2W + T1W T2W + T1W + T1C Logistic ACC 0.571 (0.509, 0.634) 0.654 (0.608, 0.699) 0.668 (0.619, 0.716) 0.707 (0.658, 0.757) 0.711 (0.672, 0.749) AUC 0.576 (0.501, 0.65) 0.671 (0.596, 0.747) 0.684 (0.629, 0.738) 0.756 (0.709, 0.804) 0.713 (0.648, 0.778) KNN ACC 0.6 (0.538, 0.662) 0.557 (0.506, 0.608) 0.65 (0.591, 0.709) 0.607 (0.561, 0.653) 0.582 (0.518, 0.646) AUC 0.58 (0.515, 0.644) 0.543 (0.479, 0.606) 0.581 (0.509, 0.652) 0.605 (0.552, 0.657) 0.574 (0.496, 0.652) RF ACC 0.732 (0.699, 0.765) 0.705 (0.659, 0.752) 0.748 (0.721, 0.776) 0.782 (0.741, 0.823) 0.773 (0.718, 0.828) AUC 0.642 (0.583, 0.702) 0.62 (0.567, 0.672) 0.654 (0.577, 0.731) 0.733 (0.667, 0.799) 0.668 (0.59, 0.746) Vote ACC 0.732 (0.709, 0.756) 0.757 (0.721, 0.793) 0.786 (0.766, 0.806) 0.786 (0.758, 0.814) 0.789 (0.763, 0.816) AUC 0.639 (0.585, 0.692) 0.61 (0.554, 0.665) 0.649 (0.57, 0.728) 0.717 (0.657, 0.777) 0.659 (0.583, 0.735) ACC, accuracy; AUC, area under curve.
The classification model integrated by two strong classifiers showed the best performance, reaching the highest average accuracy rate of 0.789 (0.763, 0.816) and AUC of 0.659 (0.583, 0.735) on the T2W + T1W + T1WC sequence. Performance was relatively stable, and the accuracy rate was higher than 70% in the seven sequence combinations. Following a RF, the average accuracy rate reached 0.782 (0.741, 0.823), and the AUC reached 0.733 (0.667, 0.799) in the T2W + T1W sequence. The results of the nearest neighbor and logistic regression models are not ideal, especially the nearest neighbor algorithm.
- Plan C (I + II + III): (see Table 4, Figure 8)
Table 4
The prediction performances of all models in plan C (95% confidence interval)Model Performance T2W T2W + T1C T1W + T1C T2W + T1W T2W + T1W + T1C Logistic ACC 0.593 (0.528, 0.658) 0.646 (0.602, 0.691) 0.696 (0.633, 0.76) 0.707 (0.666, 0.748) 0.7 (0.664, 0.736) AUC 0.576 (0.512, 0.641) 0.671 (0.607, 0.735) 0.681 (0.636, 0.726) 0.737 (0.677, 0.798) 0.731 (0.661, 0.801) KNN ACC 0.589 (0.529, 0.65) 0.532 (0.477, 0.587) 0.664 (0.618, 0.71) 0.632 (0.594, 0.67) 0.596 (0.538, 0.655) AUC 0.56 (0.499, 0.62) 0.544 (0.501, 0.587) 0.624 (0.557, 0.69) 0.612 (0.56, 0.664) 0.579 (0.508, 0.65) RF ACC 0.725 (0.686, 0.764) 0.709 (0.676, 0.742) 0.725 (0.693, 0.757) 0.77 (0.732, 0.807) 0.771 (0.727, 0.816) AUC 0.648 (0.594, 0.703) 0.63 (0.565, 0.695) 0.658 (0.576, 0.74) 0.71 (0.638, 0.782) 0.697 (0.614, 0.78) Vote ACC 0.736 (0.705, 0.766) 0.754 (0.72, 0.787) 0.782 (0.761, 0.803) 0.786 (0.753, 0.818) 0.796 (0.76, 0.833) AUC 0.636 (0.585, 0.688) 0.622 (0.559, 0.686) 0.661 (0.571, 0.75) 0.704 (0.632, 0.777) 0.689 (0.615, 0.763) ACC, accuracy; AUC, area under curve.
After combining the key features of the different sequences, the performance of the three sequences was greatly improved in the integrated classification model [ACC: 0.796 (0.76, 0.833), AUC: 0.689 (0.615, 0.763)]. The average accuracy rate was close to 80%, and the average AUC exceeded 70%. Furthermore, the performance was stable, and the accuracy rate was higher than 70% in multiple sequence combinations.
After the combination of features, the performance of the other models improved to varying degrees. The average accuracy of the RF was 0.771 (0.727, 0.816), and the AUC was 0.697 (0.614, 0.78).
After combining the key characteristics of the different sequences, the combined effect of the three sequences was greatly enhanced in the ensemble model [ACC: 0.796 (0.76, 0.833), AUC: 0.689 (0.615, 0.763)]. The average accuracy rate was close to 80%, and the average AUC exceeded 70% (Figures 9,10).
Discussion
Ki-67 index is a nuclear antigen related to proliferating cells. Its function is closely related to mitosis and is indispensable in cell proliferation. At present, clinical immunohistochemistry techniques are used to detect the expression of Ki-67 protein, and its positive staining indicates that cancer cells are proliferating actively. It can be used to evaluate the differentiation status of MB cells at the molecular biology level. Compared with traditional pathological classification, this indicator is more objective and easier to implement.
Several studies have confirmed that high expression of the Ki-67 index in tumors suggests a poor prognosis (18,19). At present, mature studies have confirmed that the expression level of Ki-67 can objectively reflect the proliferation speed and malignant degree of brain tumors. Current studies have proven that Ki-67 has a clear guiding significance for the prognosis of MB (20). However, among tumors of the central nervous system, most studies (6,21) that predicted Ki-67 by radiomics studied gliomas; few scholars have attempted to predict Ki-67 expression in MB. In addition, few studies on MB (22,23) have implemented predictions of molecular typing. Our research aimed to predict the expression level of Ki-67 in MB through machine learning methods to provide a noninvasive way to predict the outcomes of patients.
No differences in clinical characteristics were found between the different expression groups of the Ki-67 index, indicating that the clinical characteristics of the patients could not completely distinguish the expression level of the Ki-67 index. Distinguishing between two types of patients with different expression levels through images is also a huge challenge for radiologists. The Ki-67 index is a marker that reflects the activity of cell proliferation. Analyzing and judging the growth, differentiation, and prognosis of MB is of great importance for clinical decision-making (24).
After analyzing the commonly used classifiers for brain tumors (25,26), we chose five models for classification: logistic regression (logistic), RF, KNN, SVM, and a soft voting integrated model (RF, SVM). The integrated classification model based on RF and SVM had the best predictive performance, which could be due to the combination of the advantages of the two models. In our research, T2W had the highest performance in predicting the expression level of Ki-67 in all sequences, and the acquired image features had a unique degree. The accuracy of the T1W sequence was slightly worse than that of the T2W sequence. In addition, we did not just identify a single sequence to predict the patient’s Ki-67, but also performed seven combinations of three sets of sequences.
We used selected combinations of the most valuable features selected from the different sequences to screen out the more meaningful features to predict Ki-67 expression levels. After the sequences were combined, the prediction performance of the five models was improved to varying degrees. The performance improvement in the logistic regression was particularly obvious. The image features of the different sequences could reflect the expression level of Ki-67, and we could superimpose the features of the different sequences to increase the level of discrimination.
Texture features are important in recognizing and classifying various brain tumor images (27-29). The key features we extracted and finally screened included many texture features, illustrating the importance of texture features in image prediction. If we can associate the different texture characteristics of the tumor with the patient’s internal pathways and prognosis, it will be helpful for the diagnosis and treatment of the patient in the future. In our research, a small part of the shape features appeared in a higher proportion of the final extracted features, including the original shape flatness and elongation. They describe the extent of image shape elongation, which applies to many shape classification tasks (30).
Our research extracted a large number of features from different sequences. Although they can completely describe the characteristics of tumors, there is a lot of redundant information. To reduce the influence of too much invalid information on the accuracy and stability of the model, we designed a combination of three ways to select variables: (I) U-test of hypothesis testing idea; (II) RF that eliminated invalid information through the angle of information gain; and (III) LASSO regression uses ROC as an evaluation criterion to reduce the impact of collinearity and correlation between variables by adding penalty factors.
The C plan U-test + RF + LASSO was relatively less used among the three combination schemes in other studies. In our research, the C scheme was more robust and had the highest performance. This finding might be because after adding the linear method (LASSO) and the nonlinear method (RF), the interference of irrelevant variables was eliminated from both angles simultaneously, making its efficiency the highest. This discovery also provided new ideas for subsequent related research.
Although KNN had some improvement after multi-sequence merging, it was still the worst of the models. SVM (RBF) and RF had relatively better effects, and the integrated model was the best. After combining multiple sequences, the effect of logistic regression was greatly improved, indicating that when the key information of the sample was sufficient, some simple linear classification models could also obtain better results.
Our work has some limitations. First, the differences between different devices might affect the results. Second, the sample size of this study was too small, leading to unsatisfactory results. In addition, the data were all from the Chinese population, and the extrapolation of the model needs to be verified and supplemented by adding populations from other regions. Therefore, we should conduct additional prospective multicenter studies to improve the performance of radiomics in detecting problems in tumors of the central nervous system. Nevertheless, the results are suitable for providing a reference for such related research, especially in gliomas with high heterogeneity. We can try to use this method to predict the expression of Ki-67 index to judge the prognosis of the patient. This may break through some of the limitations of existing glioma-related research, resulting in better performance.
In this study, based on the image features of the three sequences of T1W, T2W, and T1WC, three feature selection methods were used to construct the prediction model, which had good accuracy in predicting the expression of the Ki-67 index. Clinically, the proposed tool has important guiding significance for analysis and judging the growth, differentiation, and prognosis of MB.
Acknowledgments
Funding: This study has received funding from the Special Research Project of Capital Health Development 2016 (No. 2020-2-2048) and Clinical Major Specialty Projects of Beijing. The National Natural Science Foundation of Beijing (No. J200003) has also supported this study.
Footnote
Reporting Checklist: The authors have completed the STARD reporting checklist. Available at https://dx.doi.org/10.21037/atm-21-5348
Data Sharing Statement: Available at https://dx.doi.org/10.21037/atm-21-5348
Conflicts of Interest: All authors have completed the ICMJE uniform disclosure form (available at https://dx.doi.org/10.21037/atm-21-5348). The authors have no conflicts of interest to declare.
Ethical Statement: The authors are accountable for all aspects of the work in ensuring that questions related to the accuracy or integrity of any part of the work are appropriately investigated and resolved. All procedures performed in this study involving human participants were in accordance with the Declaration of Helsinki (as revised in 2013). The study was approved by the Medical Ethics Committee of Beijing Tiantan Hospital, Capital Medical University (No. YW2018-022-08). Since this study is a retrospective analysis and the patients have been anonymously processed, the patient’s informed consent is not required.
Open Access Statement: This is an Open Access article distributed in accordance with the Creative Commons Attribution-NonCommercial-NoDerivs 4.0 International License (CC BY-NC-ND 4.0), which permits the non-commercial replication and distribution of the article with the strict proviso that no changes or edits are made and the original work is properly cited (including links to both the formal publication through the relevant DOI and the license). See: https://creativecommons.org/licenses/by-nc-nd/4.0/.
References
- Sun Y, Liu G, Chen W, et al. Dosimetric comparisons of craniospinal axis irradiation using helical tomotherapy, volume-modulated arc therapy and intensity-modulated radiotherapy for medulloblastoma. Transl Cancer Res 2019;8:191-202. [Crossref]
- Gerdes J, Lemke H, Baisch H, et al. Cell cycle analysis of a cell proliferation-associated human nuclear antigen defined by the monoclonal antibody Ki-67. J Immunol 1984;133:1710-5. [PubMed]
- Sun X, Kaufman PD. Ki-67: more than a proliferation marker. Chromosoma 2018;127:175-86. [Crossref] [PubMed]
- Sharma V, Shoaib Y, Gupta LN, et al. P53 and Ki-67 Expression in Primary Pediatric Brain Tumors: Does it Correlate with Presentation, Histological Grade, and Outcome? Asian J Neurosurg 2018;13:1026-32. [Crossref] [PubMed]
- Zhao F, Zhang J, Li P, et al. Prognostic value of Ki-67 index in adult medulloblastoma after accounting for molecular subgroup: a retrospective clinical and molecular analysis. J Neurooncol 2018;139:333-40. [Crossref] [PubMed]
- Li Y, Qian Z, Xu K, et al. Radiomic features predict Ki-67 expression level and survival in lower grade gliomas. J Neurooncol 2017;135:317-24. [Crossref] [PubMed]
- Bai HX, Lee AM, Yang L, et al. Imaging genomics in cancer research: limitations and promises. Br J Radiol 2016;89:20151030 [Crossref] [PubMed]
- Wu H, Han X, Wang Z, et al. Prediction of the Ki-67 marker index in hepatocellular carcinoma based on CT radiomics features. Phys Med Biol 2020;65:235048 [Crossref] [PubMed]
- Caiazzo C, Di Micco R, Esposito E, et al. The role of MRI in predicting Ki-67 in breast cancer: preliminary results from a prospective study. Tumori 2018;104:438-43. [Crossref] [PubMed]
- Hu XX, Yang ZX, Liang HY, et al. Whole-tumor MRI histogram analyses of hepatocellular carcinoma: Correlations with Ki-67 labeling index. J Magn Reson Imaging 2017;46:383-92. [Crossref] [PubMed]
- Surov A, Clauser P, Chang YW, et al. Can diffusion-weighted imaging predict tumor grade and expression of Ki-67 in breast cancer? A multicenter analysis. Breast Cancer Res 2018;20:58. [Crossref] [PubMed]
- Sakai Y, Yang C, Kihira S, et al. MRI Radiomic Features to Predict IDH1 Mutation Status in Gliomas: A Machine Learning Approach using Gradient Tree Boosting. Int J Mol Sci 2020;21:8004. [Crossref] [PubMed]
- Van Griethuysen JJM, Fedorov A, Parmar C, et al. Computational Radiomics System to Decode the Radiographic Phenotype. Cancer Res 2017;77:e104-7. [Crossref] [PubMed]
- Park BE, Jang WS, Yoo SK. Texture Analysis of Supraspinatus Ultrasound Image for Computer Aided Diagnostic System. Healthc Inform Res 2016;22:299-304. [Crossref] [PubMed]
- Galloway M. Texture analysis using gray level run lengths. Computer Graphics & Image Processing 1975;4:172-9. [Crossref]
- Reif DM, Motsinger AA, McKinney BA, et al. Feature Selection using a Random Forests Classifier for the Integrated Analysis of Multiple Data Types. 2006 IEEE Symposium on Computational Intelligence and Bioinformatics and Computational Biology, 2006:1-8. doi:
10.1109/CIBCB.2006.330987 .10.1109/CIBCB.2006.330987 - Tibshirani R. Regression Shrinkage and Selection via the Lasso. Journal of the Royal Statistical Society. Series B (Methodological) 1996;58:267-88. [Crossref]
- Tagliafico AS, Bignotti B, Rossi F, et al. Breast cancer Ki-67 expression prediction by digital breast tomosynthesis radiomics features. Eur Radiol Exp 2019;3:36. [Crossref] [PubMed]
- Zhang QW, Gao YJ, Zhang RY, et al. Personalized CT-based radiomics nomogram preoperative predicting Ki-67 expression in gastrointestinal stromal tumors: a multicenter development and validation cohort. Clin Transl Med 2020;9:12. [Crossref] [PubMed]
- Moschovi M, Koultouki E, Stefanaki K, et al. Prognostic significance of angiogenesis in relation to Ki-67, p-53, p-27, and bcl-2 expression in embryonal tumors. Pediatr Neurosurg 2011;47:241-7. [Crossref] [PubMed]
- Li J, Liu S, Qin Y, et al. High-order radiomics features based on T2 FLAIR MRI predict multiple glioma immunohistochemical features: A more precise and personalized gliomas management. PLoS One 2020;15:e0227703 [Crossref] [PubMed]
- Iv M, Zhou M, Shpanskaya K, et al. MR Imaging-Based Radiomic Signatures of Distinct Molecular Subgroups of Medulloblastoma. AJNR Am J Neuroradiol 2019;40:154-61. [Crossref] [PubMed]
- Yan J, Liu L, Wang W, et al. Radiomic Features From Multi-Parameter MRI Combined With Clinical Parameters Predict Molecular Subgroups in Patients With Medulloblastoma. Front Oncol 2020;10:558162 [Crossref] [PubMed]
- Sengupta S, Chatterjee U, Banerjee U, et al. A study of histopathological spectrum and expression of Ki-67, TP53 in primary brain tumors of pediatric age group. Indian J Med Paediatr Oncol 2012;33:25-31. [Crossref] [PubMed]
- Sanghani P, Ang BT, King NKK, et al. Overall survival prediction in glioblastoma multiforme patients from volumetric, shape and texture features using machine learning. Surg Oncol 2018;27:709-14. [Crossref] [PubMed]
- Ortiz-Ramón R, Ruiz-España S, Mollá-Olmos E, et al. Glioblastomas and brain metastases differentiation following an MRI texture analysis-based radiomics approach. Phys Med 2020;76:44-54. [Crossref] [PubMed]
- Fetit AE, Novak J, Rodriguez D, et al. Radiomics in paediatric neuro-oncology: A multicentre study on MRI texture analysis. NMR Biomed 2018; [Crossref] [PubMed]
- Orphanidou-Vlachou E, Vlachos N, Davies NP, et al. Texture analysis of T1 - and T2 -weighted MR images and use of probabilistic neural network to discriminate posterior fossa tumours in children. NMR Biomed 2014;27:632-9. [Crossref] [PubMed]
- Yin HL, Li DB, Jiang Y, et al. High-throughput texture analysis in the distinction of single metastatic brain tumors from high-grade gliomas. Zhonghua Zhong Liu Za Zhi 2018;40:841-6. [PubMed]
- Stojmenovic M, Žunić J. Measuring Elongation from Shape Boundary. Journal of Mathematical Imaging and Vision 2008;30:73-85. [Crossref]
(English Language Editor: J. Chapnick)