Integrated analysis of immune infiltration in esophageal carcinoma as prognostic biomarkers
Introduction
Esophageal cancer (EC) is one of the most fatal and aggressive malignancies among digestive cancers and is the sixth most common cause of cancer-related death (1). There are 2 types of EC: adenocarcinoma and squamous cell carcinoma (2). Esophageal squamous cell carcinoma (ESCC) comprises more than 90% of EC patients worldwide (3). Despite progress in screening, diagnosis, and curative resection, it has remained a major global cause of tumor-associated death with unsatisfactory clinical results (4). Surgical resection is currently the only potential therapeutic cure for EC (5). However, nearly 20–25% of newly diagnosed EC patients present with distant organs metastases, and only a small group of these advanced patients have the opportunity to undergo surgery (6). Further, about 50% of EC patients with resectable tumors experience recurrence within 2 years (7). Therefore, better clinical prognosis parameters and new therapies for treatment are required. In the past decades, biochemical and genomic investigation for tumor cells have been studied to distinguish patient subpopulations with different prognoses and therapeutic responses, and to identify potential drug targets (8). However, the malignant phenotype of cancer is defined not only by the original activity of cells, but also by immune cells that are replenished and activated in tumor-related microenvironments. The tumor microenvironment plays an important role in tumor formation and progression (9). Tumor-infiltrating immune cells (TIICs), such as T cells, B cells, dendritic cells (DC), macrophages, neutrophils, monocytes, and mast cells, could modulate tumor progression and stimulate many new therapeutic targets (10). Moreover, many studies have reported the important role of TIICs for tumor development. For example, high expression of TIICs in the cancer nest indicates unfavorable clinical prognostic outcomes in some cancers including renal cell carcinoma, melanoma, colorectal cancer, and so on (11-13). The number and distribution of immune cells is the most important factor for determining tumor fate, including inhibition or promotion of tumorigenesis, cell metastasis, cell migration, and tumor angiogenesis (14). Each tumor can potentially exhibit an internal distribution of immune cells that is heterogeneous in location. The distribution of immune cells can also be heterogeneous in comparisons between patient-matched primary tumor and metastasis, or among various metastases derived from the same patient. For example, the majority of CD8+T cells are cytotoxic T lymphocytes generally believed to play a major role in directing immune responses to tumors. The presence of CD8+T cell infiltrates in the vast majority of cancers is associated with longer patient survival (15). Additionally, tumor-infiltrating mast cells (TIM) are a subgroup of innate immune cells, which have been shown to have a deep immunomodulatory role in cancer progression, including angiogenesis, reconstitution of the cancer microenvironment, and interactions with other immune cells (16). However, TIICs and their role in EC have not yet been fully investigated.
Recently, the Cell-type Identification by Estimating Relative Subsets of RNA Transcripts (CIBERSORT) algorithm has been well used as a metagenomic approach (17). It is based on a deconvolution algorithm which evaluates the relative variation in expression of a series genes compared to that of all other genes. So, the density and composition of tumor immune cells could be better analyzed by using the CIBERSORT approach. On account of the superior function of CIBERSORT, its application aroused more attention for studying the heterogeneity of cancer cells. In our study, by using CIBERSORT, we quantified 22 TIICs subgroups of immune cells response in EC to study the relationship between TIICs with molecular cell subsets, clinical survival, and other clinical characteristics. A much more comprehensive analysis of the molecular mechanisms and underlying immune microenvironment is needed to further progress in combating ESCC. This study is expected to provide profound insights for the complex association between tumor immune cell heterogeneity, molecular subtypes, and tumorigenicity in ESCC. In addition, this report shows a novel immunotherapy strategy for ESCC. We present the following article in accordance with the REMARK reporting checklist (available at https://dx.doi.org/10.21037/atm-21-5881).
Methods
Data acquisition
Firstly, the RNA-seq data [fragments per kilobase of transcript per million mapped reads (FPKM) values] of ESCC cohort were downloaded from The Cancer Genome Atlas (TCGA) website (https://cancergenome.nih.gov/). Secondly, we used Perl software to merge the data. The Voom standardized method was used to normalize the RNA-seq profiles here (mean-variance modelling at the observational level). The criteria for exclusion were as follows: pathological diagnosis was not EC; clinical data did not have sufficient information (age of patients, gender, tumor (T) staging, survival time and status).
Evaluation of tumor-infiltrating immune cells
The CIBERSORT method of analyzing gene data was used to calculate 22 human naive immune cell subtypes, then identify the features of 547 marker genes to assess the scores of every cell type. In order to ensure the accuracy of the results, the CIBERSORT method obtains the deconvoluted P value of every sample by using Monte Carlo methods. Standardized processing of data sets for gene expression was conducted by uploading to the CIBERSORT website (https://cibersort.stanford.edu/index.php), which runs using 1,000 aligned default signature matrices.
Survival analysis
We used the Kaplan-Meier method to analysis the relationship between immune subgroups cell and overall survival (OS). We then screened the prognosis of each cell subpopulation in 22 human immune cell phenotypes. The Kaplan-Meier method was applied by the survfit method in “survival” package in R software (https://www.r-project.org/). We also explored the association between the LM22 immune cell matrix and tumor-node-metastasis (TNM) stage.
Immune cell classification was detected by immunohistochemistry (IHC)
Tissue samples were collected from 50 ESCC patients who underwent surgery in the Harbin Medical University Cancer Hospital from January 2012 to December 2012. The clinical data was reliable and complete, and the patients had not received anticancer treatment before surgery. All participants were followed up for 5 years. The study was approved by ethics committee of Harbin Medical University Cancer Hospital (Registration number 2021-49-IIT), and written consent was provided by patients or their relatives before participating in this study. The study was conducted in accordance with the Declaration of Helsinki (as revised in 2013). Clinical staging of EC patients was based on the 2017 National Comprehensive Cancer Network (NCCN) guidelines for EC staging criteria. IHC was performed according to manufacturer’s instructions with appropriate antibodies against tryptase (ab329383, diluted 1/1,000, Abcam, Cambridge, MA, USA), CD8 (ab329383, Abcam, diluted 1/1,000). Phosphate-buffered saline (PBS) took the place of the first antibody as negative control.
Evaluation of immunostaining
The number of mast cells was assessed in 3 non-overlapping high-power fields (HPFs; ×400; 0.08 mm2) with typical tryptase staining, and relative mean value was used for subsequent analysis. The intensity of CD8+T cell was recorded as the mean number of CD8+ positive/HPF from 3 randomized fields, respectively. The expression of immune cells defined as low and high expression was calculated by the percentage of per 100 cells. The evaluation of specimens was analyzed by 2 investigators independently who were blinded to the clinical information.
Statistical analysis
Patients with CIBERSORT P values <0.05 were included in this study. All eligible tissue samples were split into 2 groups: ESCC cancer tissues and adjacent tissues. The expression of immune cells was analyzed in each sample respectively. To investigate the relationship among the cells of 22 immune subgroups, we calculated the Pearson correlation by R software. Log-rank statistic method was applied to analyze the differences of OS time with Kaplan-Meier plots. A heatmap was produced with the R package “Complex Heatmap”. A P-value of <0.05 was considered statistically significant.
The infiltration of mast cells and CD8+T cell in the cancer nest were divided into 2 groups: low expression and high expression, according to the median mast cell percentage. Statistical analyses were performed using the software SPSS 17.0 (IBM Corp., Armonk, NY, USA) and GraphPad Prism 8 (GraphPad Software Inc., La Jolla, CA, USA). The correlation between immune cells and clinicopathological data was estimated using Pearson’s chi-square correlation analysis. The Cox proportional hazards regression model was applied to perform univariate and multivariate analyses, and the parameters demonstrating a statistically significant effect on OS in the univariate analysis were included in the multivariate analysis. Analysis of the correlation between mast cells and other immune cells in EC was made by Spearman correlation. The P values <0.05 were considered to indicate statistical significance.
Results
The landscape of immune infiltration in EC
Using the CIBERSORT method, we demonstrated the difference of immune cells infiltration between 3 para-cancerous tissues and 82 tumor tissues in 22 subgroups of immune cells. The findings obtained from 82 EC patients are summarized in Figure 1A. We identified several different correlations of LM22 immune cells between ESCC tumor tissues and all samples (including para-cancerous tissues and tumor tissues). Therefore, we hypothesized that the change of infiltration degree of immune cells directly reflects the difference in immunity between EC tumor tissues and adjacent tissues (Figure 1B). Furthermore, we used unsupervised hierarchical clustering based on 22 immune cells subsets, and specimens were clearly separated into 2 discrete groups (Figure 1C). Apparently, the share of EC immune subgroup cells was significantly different between tumors and normal samples. The proportions of monocyte and macrophages M0 were significantly higher in normal tissues than EC cancer tissues (P=0.047 and 0.047, respectively). While T follicular helper cells, T cells CD8, natural killer (NK) cells activated, NK cells resting, mast cells resting, and B cell naive, mast cells, T cells CD4 memory resting, B cells memory, plasma cells, T cells CD4 naive, T cells CD4 memory resting, T cells CD4 memory activated, T cells regulatory (Tregs), T cells gamma delta, macrophages M1, macrophages M2, DC resting, DC activated, eosinophils, and neutrophils were not obviously altered between groups (Figure 2, Table 1). In conclusion, our study demonstrated that different immune cell subgroups and cell heterogeneity in EC closely regulated the clinical process and maintained the clinical significance.
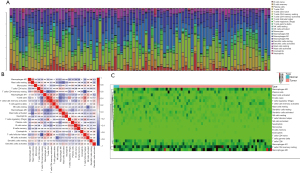
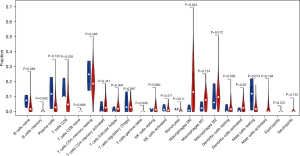
Table 1
Immune cells subtype | P value | Normal means | Tumor means |
---|---|---|---|
B cells naive | 0.084 | 0.070557 | 0.035588 |
B cells memory | 0.562 | 0.000000 | 0.002712 |
Plasma cells | 0.102 | 0.122489 | 0.044495 |
T cells CD8 | 0.076 | 0.140727 | 0.074117 |
T cells CD4 naive | 0.899 | 0.000000 | 0.000342 |
T cells CD4 memory resting | 0.385 | 0.242939 | 0.193597 |
T cells CD4 memory activated | 0.341 | 0.022688 | 0.042714 |
T cells follicular helper | 0.304 | 0.010991 | 0.027262 |
T cells regulatory (Tregs) | 0.867 | 0.054269 | 0.037650 |
T cells gamma delta | 0.648 | 0.000000 | 0.001149 |
NK cells resting | 0.664 | 0.015108 | 0.023257 |
NK cells activated | 0.471 | 0.021748 | 0.017728 |
Monocytes | 0.011 | 0.017861 | 0.005462 |
Macrophages M0 | 0.024 | 0.017902 | 0.173596 |
Macrophages M1 | 0.124 | 0.030822 | 0.080277 |
Macrophages M2 | 0.512 | 0.088587 | 0.116314 |
Dendritic cells resting | 0.509 | 0.016874 | 0.030458 |
Dendritic cells activated | 0.29 | 0.009988 | 0.034592 |
Mast cells resting | 0.073 | 0.116450 | 0.028685 |
Mast cells activated | 0.194 | 0.000000 | 0.023151 |
Eosinophils | 0.537 | 0.000000 | 0.001433 |
Eosinophils | 0.132 | 0.000000 | 0.005422 |
CIBERSORT, Cell-type Identification by Estimating Relative Subsets of RNA Transcripts; ESCC, esophageal squamous cell carcinoma.
Differential infiltration analysis of immune cell fraction in stage subgroup
We further explored the relationships between tumor stage and immune cells subclasses. The majority of T cells gamma delta was mainly focused on the earlier stage EC patients (Stage I/II) (P=0.037, Figure 3 and Figure S1). Whereas the majority of T follicular helper cells, monocyte, T cells CD8, NK cells resting, NK cells activated, macrophages M0, mast cells resting, mast cells activated, and B cell naive, B cells memory, plasma cells, T cells CD4 naive, T cells CD4 memory resting, T cells CD4 memory activated, T cells regulatory (Tregs), macrophages M1, macrophages M2, DC resting, DC activated, Eosinophils, and neutrophils did not correlate with the EC tumor stage.
Identification of prognostic LM22 immune cell subtypes
Moreover, we used Kaplan-Meier plot and log-rank test to analyze related immune cell subsets mentioned earlier, and this study showed that mast cells activated was associated with less favorable outcome (P=0.047, Figure 4 and Figure S2). The remaining cell subtypes were not significantly associated with OS of EC patients.
CD8+T cells infiltration negatively correlates with TIM
According to CIBERSORT analysis, patients with high TIM had a significantly less favorable prognosis than those with low infiltration. To detect the possible role of mast cells in EC, we applied an indirect comparison by IHC analysis. The mast cells fraction in cancer tissues was identified by tryptase molecule positive staining. We analyzed mast cell percentage per 100 total cells in each tissue. High intra-tumoral mast cells infiltration was significantly associated with late cancer stages (Figure 5A). Furthermore, by IHC we also found that mast cells were concentrated in cancer tissues (Figure 5B), showing a vital role of mast cells in ESCC microenvironment. We also conducted IHC analysis to investigate the association between mast cells infiltration and CD8+T cells in ESCC patients. We found that high mast cells infiltration specimens were frequently accompanied with lower CD8+T cells expression (Figure 5B). In addition, mast cell infiltration was significantly negatively related to CD8+T cells, (P<0.001, Figure 5C). These findings revealed that high level CD8+T cell infiltration was also present in patients with lower level mast cells.
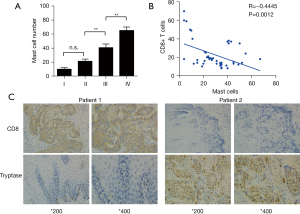
TIM and CD8+T cells are associated with prognosis of in EC patients
Next, we evaluated the clinical relevance of mast cell infiltration and CD8+T cells of esophagus cancer patients. Comparing patients with high expression (≥19.5% median level) versus low expression (<19.5%) TIM, the OS rates were higher for those with lower percentages of mast cells group (Figure 6A). We found that low expression patients showed better disease-free survival (DFS; Figure 6B). The median CD8+T cell percentage was 22.15%, so CD8+T of less than or equal to 22.15% was considered as low expression and CD8+T of more than 12.15% was defined as high expression. Survival analysis curves also showed high percentage of CD8+T cells to be a significantly good prognostic factor for OS (P<0.001, Figure 6C), but not for DFS (Figure 6D). We further calculated the relationship between different mast cells infiltration and CD8+T cells for patient OS. We found low TIM and high CD8+T cell cases showed remarkably better OS and DFS (Figure 6E,6F).
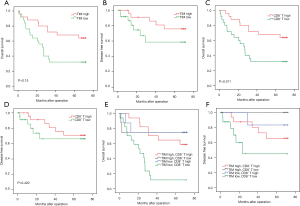
The association between mast cells and clinical pathology data of 50 ESCC patients was also detected (Table 2). In addition, our study revealed correlation between high mast cells infiltration and late T stage (P=0.042). Further research revealed no significant correlation between the percentage of mast cells and other clinicopathological data, such as age (P=0.544), TNM stage (P=0.626), cancer differentiation grade (P=0.152).
Table 2
Characteristic | Numbers | TIM infiltration | CD8+T infiltration | |||||
---|---|---|---|---|---|---|---|---|
High | Low | P | High | Low | P | |||
Age (years) | 0.544 | 1.000 | ||||||
≤60 | 34 | 18 | 16 | 17 | 17 | |||
>60 | 16 | 7 | 9 | 8 | 8 | |||
Differentiation | 0.152 | 0.774 | ||||||
Well | 21 | 8 | 13 | 10 | 11 | |||
Moderate/poor | 29 | 17 | 12 | 15 | 14 | |||
Tumor location | 0.713 | 0.269 | ||||||
Upper | 9 | 4 | 5 | 6 | 3 | |||
Medial/lower esophagus | 41 | 21 | 20 | 19 | 22 | |||
pT classification | 0.042 | 0.221 | ||||||
T1–2 | 7 | 1 | 6 | 2 | 5 | |||
T3–4 | 43 | 24 | 19 | 23 | 20 | |||
Lymph node metastasis classification | 0.564 | 0.564 | ||||||
Negative | 20 | 9 | 11 | 11 | 9 | |||
Positive | 30 | 16 | 14 | 14 | 16 | |||
TNM stage | 0.626 | 0.470 | ||||||
I | 11 | 6 | 5 | 7 | 4 | |||
II | 13 | 5 | 8 | 5 | 8 | |||
III | 26 | 14 | 12 | 13 | 13 |
TIM, tumor-infiltrating mast cells; TNM, tumor node metastasis.
The results revealed that TNM stage [hazard ratio (HR) =1.787, 95% confidence interval (CI): 1.047 to 3.052, P=0.033], CD8+T cells expression (HR =2.744, 95% CI: 1.217 to 6.186, P=0.015), and mast cells expression (HR =2.649, 95% CI: 1.173 to 5.980, P=0.019) were correlated with OS by univariate analyses. There was no significant correlation between survival and age, tumor location, node, T stage, or differentiation. Moreover, multivariate analysis indicated that TNM stage (HR =1.867, 95% CI: 1.082 to 3.220, P=0.025) was an independent prognostic factor for OS (Table 3).
Table 3
Variables | Univariate analysis | Multivariate analysis | |||
---|---|---|---|---|---|
HR (95% CI) | P value | HR (95% CI) | P value | ||
Age | 1.657 (0.749, 3.669) | 0.213 | |||
Tumor location | 0.478 (0.506,4.274) | 1.471 | |||
Node | 1.434 (0.639, 3.219) | 0.382 | |||
T stage | 0.847 (0.292, 2.459) | 0.760 | |||
TNM stage | 1.787 (1.047, 3.052) | 0.033 | 1.867(1.082,3.220) | 0.025 | |
Differentiation | 0.602 (0.277, 1.310) | 0.201 | |||
TIM infiltration | 2.649 (1.173, 5.980) | 0.019 | 0.079 | ||
CD8+T infiltration | 2.744 (1.217, 6.186) | 0.015 | 0.052 |
CI, confidence interval; HR, hazard ratio; OS, overall survival; TIM, tumor-infiltrating mast cells; TNM, tumor node metastasis.
In summary, this study demonstrated that high mast cells percentage is related to tumor progression and reduced survival time of ESCC patients.
Discussion
The detection of immune cells as biomarkers in cancer immune microenvironment has become a meaningful method for determining immunotherapy therapeutic strategies for cancer patients. The number and distribution of immune cells is the most important factor for determining tumor fate, including inhibition or promotion of tumorigenesis, cell metastasis, cell migration, and tumor angiogenesis (18). However, the immune microenvironment in different cancer types is complicated and heterogeneity (19). Recent studies have shown that tumors can avoid host immune killing responses by expressing abnormal immune checkpoints including programmed cell death protein 1 (PD-1), programmed death-ligand 1 (PD-L1), PD-L2, and T cell immunoreceptor with Ig and ITIM domains (TIGIT) (20). In order to improve treatment for EC patients, heterogeneous measurement and evaluation of immune relative checkpoint molecules which influence patient prognosis is vital. In our research, we used CIBERSORT to analyze the different expressions of immune subgroup cells infiltration in EC and adjacent normal tissue, and showed that there was a significant difference of immune cell expression between the tumor and normal group. Therefore, CIBERSORT can be used to determine the differences in immune microenvironment between cancer groups and adjacent normal tissue. The different fractions of monocyte and macrophages M0 in normal tissues compared to EC tumor tissues revealed that different infiltration of immune cells and the heterogeneity in EC may regulate tumor molecular processes and indicate meaningful clinical values.
Mast cells have shown positive on the prognosis of many tumors as a subgroup of immune cells (21). According to a recent study, mast cells inhibit tumor growth and promote apoptosis in breast cancer by regulating interleukin-4 (IL-4) (22). However, the function and regulation effects of microenvironment mast cells in human EC are still unclear. A recent study shows there appears to be a correlation between the number of mast cells and tumor angiogenesis in patients with esophageal squamous cell carcinoma. In the current work, we found that TIMs were positively related to EC progression.
The proportion of mast cells in the tumor significantly increased in the later stage of EC, and a high proportion of mast cells were shown to be positively correlated with a lower survival rate of EC patients. We also found that the proportion of tumor microenvironment mast cell was increased in the late stage of EC. High mast cells expression in EC patients was positively correlated with worse survival time. Pathological function of immune mast cells in human EC microenvironment reveals a new immune escaping system for cancer progression. Our results also confirmed findings of previous reports which showed that mast cells were a biomarker to predict clinical patient outcome.
In the current study, we conducted IHC staining of tryptase and revealed that high level expression of mast cells was related to more advanced T stage. In addition, a high level of mast cells expression was shown to be a prognostic factor for OS in EC patients. Although many researchers have shown that mast cells are associated with less favorable patient outcome, its detailed mechanism of microenvironment interaction with cancer cells remains unknown. In gallbladder cancer, TIM could activate CD8+ cells and regulate the immune environment (23). Bulfone-Paus et al. revealed that mast cells could recruit CD8+T cells by regulating CCL3 in the cancer environment (24). In this report, we revealed that TIM infiltration was negatively related with CD8+T cells in EC. This may account for the heterogeneity, although further research is required for confirmation.
Conclusions
In summary, we used the CIBERSORT deconvolution algorithm to analyze LM22 immune subgroups cells and reveal the overall information of EC immune microenvironment landscape. Our study has also revealed an important aspect in predicting EC patient clinical outcome. We revealed that TIM infiltration level is related to cancer progression and can independently predict worse OS. The TIM infiltration is related to higher accumulation of CD8+T immune cells, which reveals an immune regulating function of TIM in the EC immune microenvironment and might provide better guidance for clinical immunotherapy planning.
Acknowledgments
Funding: This study was supported by National Natural Science Foundation of China (No. 82172786 to Jianqun Ma; No. 81903120 to Hang Yin; No. 81902317 to Xiaoyuan Wang), Young Innovative Talents Training Program of Higher Education Institutions in Heilongjiang Province (No. UNPYSCT-2020161 to Hang Yin), Haiyan Foundation of Harbin Medical University Cancer Hospital (No. JJQN2019-01 to Xiaoyuan Wang and No. JJZD2020-13 to Hang Yin), Postdoctoral Foundation of Heilongjiang Province (No. LBH-Z18224 to Xiaoyuan Wang and No. LBH-Z20076 to Hang Yin), the Natural Science Fund of Heilongjiang (No. YQ2021H024 to Hang Yin and No. LH2019H041 to Xiaoyuan Wang), China Postdoctoral Science Foundation (No. 2018M641861 to Xiaoyuan Wang, No. 2020M681117 to Hang Yin and No. 2021T140170 to Hang Yin), and Young Talents Foundation of Harbin Medical University Cancer Hospital (No. BJQN2019-06 to Xiaoyuan Wang and No. BJQN2020-01 to Hang Yin).
Footnote
Reporting Checklist: The authors have completed the REMARK reporting checklist. Available at https://dx.doi.org/10.21037/atm-21-5881
Data Sharing Statement: Available at https://dx.doi.org/10.21037/atm-21-5881
Conflicts of Interest: All authors have completed the ICMJE uniform disclosure form (available at https://dx.doi.org/10.21037/atm-21-5881). The authors have no conflicts of interest to declare.
Ethical Statement: The authors are accountable for all aspects of the work in ensuring that questions related to the accuracy or integrity of any part of the work are appropriately investigated and resolved. The study was approved by ethics committee of Harbin Medical University Cancer Hospital (registration number 2021-49-IIT). The study was conducted in accordance with the Declaration of Helsinki (as revised in 2013). Written consent was provided by patients or their relatives before participating in this study.
Open Access Statement: This is an Open Access article distributed in accordance with the Creative Commons Attribution-NonCommercial-NoDerivs 4.0 International License (CC BY-NC-ND 4.0), which permits the non-commercial replication and distribution of the article with the strict proviso that no changes or edits are made and the original work is properly cited (including links to both the formal publication through the relevant DOI and the license). See: https://creativecommons.org/licenses/by-nc-nd/4.0/.
References
- Siegel RL, Miller KD, Jemal A. Cancer statistics, 2020. CA Cancer J Clin 2020;70:7-30. [Crossref] [PubMed]
- Lordick F, Mariette C, Haustermans K, et al. Oesophageal cancer: ESMO Clinical Practice Guidelines for diagnosis, treatment and follow-up. Ann Oncol 2016;27:v50-7. [Crossref] [PubMed]
- Huang J, Xu J, Chen Y, et al. Camrelizumab versus investigator's choice of chemotherapy as second-line therapy for advanced or metastatic oesophageal squamous cell carcinoma (ESCORT): a multicentre, randomised, open-label, phase 3 study. Lancet Oncol 2020;21:832-42. [Crossref] [PubMed]
- van Laarhoven HW. Is chemotherapy for advanced or metastatic oesophageal squamous cell carcinoma no longer needed? Lancet Oncol 2020;21:743-5. [Crossref] [PubMed]
- Shah MA, Kennedy EB, Catenacci DV, et al. Treatment of Locally Advanced Esophageal Carcinoma: ASCO Guideline. J Clin Oncol 2020;38:2677-94. [Crossref] [PubMed]
- Aversa JG, Diggs LP, Hagerty BL, et al. Trends of Clinician Adherence to Evidence-Based Recommendations for Multidisciplinary Oncology Care for Patients With Esophageal Cancer. JAMA Oncol 2020;6:1290-2. [Crossref] [PubMed]
- Arnold M, Ferlay J, van Berge Henegouwen MI, et al. Global burden of oesophageal and gastric cancer by histology and subsite in 2018. Gut 2020;69:1564-71. [Crossref] [PubMed]
- Prensner JR, Enache OM, Luria V, et al. Noncanonical open reading frames encode functional proteins essential for cancer cell survival. Nat Biotechnol 2021;39:697-704. [Crossref] [PubMed]
- DeBerardinis RJ. Tumor Microenvironment, Metabolism, and Immunotherapy. N Engl J Med 2020;382:869-71. [Crossref] [PubMed]
- Kennedy LB, Salama AKS. A review of cancer immunotherapy toxicity. CA Cancer J Clin 2020;70:86-104. [Crossref] [PubMed]
- Donskov F, von der Maase H. Impact of immune parameters on long-term survival in metastatic renal cell carcinoma. J Clin Oncol 2006;24:1997-2005. [Crossref] [PubMed]
- Jensen TO, Schmidt H, Møller HJ, et al. Intratumoral neutrophils and plasmacytoid dendritic cells indicate poor prognosis and are associated with pSTAT3 expression in AJCC stage I/II melanoma. Cancer 2012;118:2476-85. [Crossref] [PubMed]
- Rao HL, Chen JW, Li M, et al. Increased intratumoral neutrophil in colorectal carcinomas correlates closely with malignant phenotype and predicts patients' adverse prognosis. PLoS One 2012;7:e30806 [Crossref] [PubMed]
- Väyrynen SA, Zhang J, Yuan C, et al. Composition, Spatial Characteristics, and Prognostic Significance of Myeloid Cell Infiltration in Pancreatic Cancer. Clin Cancer Res 2021;27:1069-81. [Crossref] [PubMed]
- Petitprez F, de Reyniès A, Keung EZ, et al. B cells are associated with survival and immunotherapy response in sarcoma. Nature 2020;577:556-60. [Crossref] [PubMed]
- Somasundaram R, Connelly T, Choi R, et al. Tumor-infiltrating mast cells are associated with resistance to anti-PD-1 therapy. Nat Commun 2021;12:346. [Crossref] [PubMed]
- Gentles AJ, Newman AM, Liu CL, et al. The prognostic landscape of genes and infiltrating immune cells across human cancers. Nat Med 2015;21:938-45. [Crossref] [PubMed]
- Nixon AB, Schalper KA, Jacobs I, et al. Peripheral immune-based biomarkers in cancer immunotherapy: can we realize their predictive potential? J Immunother Cancer 2019;7:325. [Crossref] [PubMed]
- McGranahan N, Swanton C. Clonal Heterogeneity and Tumor Evolution: Past, Present, and the Future. Cell 2017;168:613-28. [Crossref] [PubMed]
- Cader FZ, Schackmann RCJ, Hu X, et al. Mass cytometry of Hodgkin lymphoma reveals a CD4+ regulatory T-cell-rich and exhausted T-effector microenvironment. Blood 2018;132:825-36. [Crossref] [PubMed]
- Ribatti D, Crivellato E. Mast cells, angiogenesis, and tumour growth. Biochim Biophys Acta 2012;1822:2-8. [Crossref] [PubMed]
- Gooch JL, Lee AV, Yee D. Interleukin 4 inhibits growth and induces apoptosis in human breast cancer cells. Cancer Res 1998;58:4199-205. [PubMed]
- Bo X, Wang J, Wang C, et al. High infiltration of mast cells is associated with improved response to adjuvant chemotherapy in gallbladder cancer. Cancer Sci 2020;111:817-25. [Crossref] [PubMed]
- Bulfone-Paus S, Bahri R. Mast Cells as Regulators of T Cell Responses. Front Immunol 2015;6:394. [Crossref] [PubMed]