Identification of ubiquitinated substrate proteins and their gene expression patterns in lung adenocarcinoma
Introduction
Lung cancer is the second most common cancer in males and females. Due to advancements in detection and treatment, its mortality rate has been decreasing over time. However, it remains the leading cause of cancer-related deaths worldwide (1). Lung adenocarcinoma (LUAD) is the most common type of lung cancer and more effective approaches are required to improve LUAD patients’ prognosis. Given advancements in genomics, our understanding of LUAD initiation and progression continues to increase. Consequently, potential therapeutic targets are being discovered (2) and targeted therapies are promising (3). Additionally, the rise of immunotherapy has greatly improved patient prognosis (4).
Recently, the focus on gene or protein expression shifted to other regulatory mechanisms, such as DNA methylation (5), histone modification (6), regulation of non-coding RNAs (7), and protein posttranslational modification (PTM). PTMs can rapidly respond to changes in the internal environment, being essential for different biological processes regulation (8).
Ubiquitination is a dynamic protein PTM, which affects multiple biological processes (9). For example, ubiquitination can change protein cellular localization (10), and histone ubiquitination can influence gene transcription (11). However, the most studied aspect of PTM involves the proteasome—a complex that recognizes ubiquitinated substrate proteins and degrades them and thus changes protein expression (12,13). In cancers, protein ubiquitination regulates the proliferation, migration, invasion (10,14), and metabolic reprogramming of cancer cells (15). An essential role for protein ubiquitination in immune-related pathways regulation has also been reported (16); this ubiquitination can perturb the tumors’ immune microenvironment (17). Given the robust regulatory function of protein ubiquitination on numerous pathways and its great potential in cancer therapy, anti-tumor therapeutic strategies that target ubiquitination have caught researchers’ attention (18-20).
Regarding LUAD, various ubiquitination-related E3 ligases have been demonstrated to affect biological processes. For example, ubiquitin-specific-processing protease 7 (USP7)-monoubiquitinated histones can epigenetically regulate cancer cell ferroptosis (21). Also, ubiquitin-conjugating enzyme E2 O (UBE2O) can ubiquitinate and degrade MAX interactor 1 (MXI1) to promote LUAD progression and radioresistance (22). Moreover, Cul3-ROC1 (CRL3)BTBD9 suppresses LUAD cell migration by targeting tumor necrosis factor-alpha-induced protein 1 (TNFAIP1) (23). The ubiquitination act through substrate proteins regulation on tumor cells. Although a global analysis of ubiquitination in lung squamous cell carcinoma (LUSC) has been reported (24), there are no analogous studies in LUAD. Therefore, it is crucial to explore the LUAD ubiquitination landscape.
We used an anti-K-ε-GG-based ubiquitination peptide enrichment combined with liquid chromatography-mass spectrometry (25) to obtain LUAD ubiquitylome data. A series of analyses were performed to reveal the LUAD protein ubiquitination landscape and unique ubiquitinated peptide motifs were found. Additionally, we focused on the analysis of genes encoding ubiquitinated substrate proteins and found different expression patterns. These expression patterns revealed heterogeneity between tumor samples and identified differences in the tumor immune infiltration microenvironment. Finally, we specifically analyzed the differences between genes encoding ubiquitinated substrate protein expression patterns and constructed a predictive model for patients’ overall survival, which can be used as a reference for LUAD clinical treatment. Although many articles have reported on the regulatory mechanism of ubiquitination in LUAD, our study is the first systematic analysis of the ubiquitination landscape in LUAD through ubiquitylome and proteomics and identified the characteristic motifs of the ubiquitinated substrate peptides. In addition, further genomics studies have also revealed the heterogeneity of the ubiquitination landscape in LUAD and provided new methods for the detection of patient prognosis. This study aimed to address the lack of LUAD ubiquitylome studies and to provide new perspectives for future research and clinical treatments. We present the following article in accordance with the MDAR reporting checklist (available at https://dx.doi.org/10.21037/atm-21-5645).
Methods
Tissue specimens
All tissue specimens were obtained from the Department of Thoracic Surgery of the Second Affiliated Hospital of Harbin Medical University. Patients with early operable lung nodules were included in the study, and those who were inoperable were excluded. Lung nodule tissues were randomly collected during surgery. After surgical resection, the pathologist confirmed five pairs of LUAD and adjacent normal lung tissues were preserved in liquid nitrogen. Patients’ clinical information is detailed in Table 1. All patients provided written informed consent and the Ethics Committee of the Second Affiliated Hospital of Harbin Medical University approved this study (KY2021-166). All procedures performed in this study involving human participants were in accordance with the Declaration of Helsinki (as revised in 2013).
Table 1
ID | Gender | Age | Pathology | Degree | Location | Size (mm) |
---|---|---|---|---|---|---|
1 | Male | 57 | Adenocarcinoma | Low | Right upper lobe | 15×12 |
2 | Female | 63 | Adenocarcinoma | Middle | Left upper lobe | 10×10 |
3 | Female | 57 | Adenocarcinoma | Middle | Right middle lobe | 8×10 |
4 | Male | 49 | Adenocarcinoma | High | Left lower lobe | 10×4 |
5 | Male | 57 | Adenocarcinoma | High | Right upper lobe | 15×10 |
Protein extraction and digestion
Samples were thoroughly ground into powder in a mortar precooled with liquid nitrogen. Four volumes of lysis buffer (1% Triton X-100, 1% protease inhibitor, 50 µM PR-619) (P0013; Beyotime, China) were added to each sample group and then lysed via sonication. Samples were centrifuged (12,000 g/10 min at 4 °C), and the supernatant was transferred into new tubes. Protein concentration was determined using a bicinchoninic acid assay (BCA) kit (23225; Thermo Fisher). An equal protein mass from each sample was withdrawn for enzymatic hydrolysis. Volumes were adjusted using the lysis buffer. Then, trichloroacetic acid (20%) was slowly added to the mixture, which was vortexed and precipitated for 2 h at 4 °C. Afterward, the mixture was centrifuged (4,500 g/5 min), and the supernatant was discarded. The pellet was washed (2–3 times) with precooled acetone and subsequently dried. Tetraethylammonium bromide was added at a final concentration of 200 mM. The pellet was dispersed using sonication, and trypsin was added at a ratio of 1:50 overnight. Dithiothreitol was added to a final concentration of 5 mM and reduced at 56 °C for 30 min. Finally, iodoacetamide was added to a final concentration of 11 mM and incubated for 15 min at room temperature.
Ubiquitinated peptide enrichment
Peptides were dissolved in IP buffer (100 mM NaCl, 1 mM ethylenediamine tetraacetic acid (EDTA), 50 mM tris(hydroxymethyl) aminomethane (tris)-HCl, and 0.5% NP-40; pH 8.0) (87788; Thermo Fisher), transferred to a prewashed K-ε-GG binding resin (PTM-1104, Hangzhou Jingjie Biotechnology, Hangzhou, China), placed on a rotating shaker (4 °C), and gently shaken and incubated overnight. After incubation, the resin was washed four times with IP buffer and twice with deionized water. Finally, the resin-bound peptides were eluted three times using 0.1% trifluoroacetic acid eluent. The eluent was collected and freeze-dried under a vacuum. Then, desalination was performed according to the C18 ZipTips (Z720070, Millipore, Burlington, MA, USA) instructions for liquid chromatography-mass spectrometry analysis.
Liquid chromatography-mass spectrometry
The trypsin-digested peptides were dissolved in formic acid (0.1%), separated, and purified using a nanoElute UHPLC system (Burker, Billerica, MA, USA). Next, peptides were injected into a capillary ion source for ionization, and mass spectrometry was performed using the tims-TOFPro (Burker, Billerica, MA, USA)). The experimental procedures have been previously reported (25).
Results obtained from mass spectrometric analysis were searched using Maxquant (version 1.6.6.0), and we used the Homosapiens_9606 dataset from Uniprot (https://www.uniprot.org). We added common protein contaminants to the dataset to calculate the false positive rate (FDR) caused by random matching. The FDR was used to eliminate the protein contamination effect on the results. Trypsin/P was specified as a cleavage enzyme, and up to four missing cleavages were allowed. The minimum peptide length was set to seven amino acid residues; the maximum peptide modifications number was set to five, and GlyGly (K) was designated as a fixed modification. The quantification method was selected for label-free quantification, and the FDR was set to 1% for both protein and peptide-spectrum match identification.
Proteome, ubiquitylome, and gene mutation analysis
Principal component analysis (PCA) and identification of differentially expressed ubiquitinated residues were performed using the “ggplot2” R package (https://cran.r-project.org/web/packages/ggplot2). All heatmaps were plotted using the “pheatmap” R package (https://cran.r-project.org/web/packages/pheatmap). The “survival” R package was used to plot the survival curves (https://cran.r-project.org/web/packages/survival). For ubiquitinated motifs analysis, we used “momo” (https://meme-suite.org/meme/tools/momo), an online tool based on the “Motif-x” algorithm. All histograms were plotted using GraphPad Prism 8. The LUAD messenger RNA (mRNA) expression data were obtained from The Cancer Genome Atlas (TCGA) (https://portal.gdc.cancer.gov/). The “maftools” R package was used for gene mutation analysis (https://bioconductor.org/packages/maftools/).
Western blotting
Tissues or cells were homogenized and lysed using RIPA buffer, and the supernatant was extracted via centrifugation at 14,000 g for 5 min. Protein concentrations were determined using the BCA kit. After adjusting to the appropriate protein concentrations, loading buffer was added and heated to 100 °C for 6 min. Electrophoresis was performed using 10% acrylamide gels, and the proteins were transferred to polyvinylidene difluoride (PVDF) membranes at 250 mA for 120 min. After blocking with 5% skimmed milk for 1 h at room temperature, the membranes were incubated overnight at 4 °C with their corresponding primary antibodies. In this study, the primary antibodies were anti-SLC1A5 (ab237704, 1:1,000; Abcam), anti-beta actin (ab6276, 1:20,000; Abcam), and anti-ubiquitin (#58395S,1:1000; Cell Signaling Technology). The PVDF membranes were washed three times with TBST for 15 min and incubated for 1 h at room temperature with secondary antibodies of the corresponding species. The secondary antibodies (ab6721, ab6728, 1:10,000) were purchased from Abcam. The BeyoECL Star ECL kit (P0018AS; Beyotime, China) was used to image the western blots.
Immunoprecipitation
The immunoprecipitation kit (88805; Thermo Fisher) was used for the immunoprecipitation analysis. Briefly, the tissue was washed three times using pre-chilled phosphate-buffered saline, lysed for 1 h using NP40 lysate homogenate, and centrifuged at 14,000 g for 10 min to isolate the supernatant. A BCA kit was used to determine protein concentrations and to retain a fraction as the input group. The inclusion of the target protein in cell lysis was determined via western blotting analysis of the input group. The co-immunoprecipitation steps followed the kit’s instructions. To ensure equal sample sizes for the immunoblotting analysis, the protein concentration of the immunoprecipitated eluate was determined using the BCA kit. SLC1A5 ubiquitination levels were detected using the anti-ubiquitin antibody at the time of immunoblotting.
Identification of prognosis-related genes encoding ubiquitinated substrate proteins
Prognostic associated genes were screened in the eight Gene Expression Omnibus (GEO) datasets pooled data using univariate Cox regression and a P value <0.01 was considered as indicating statistical significance. Then, all genes were screened again using least absolute shrinkage and selection operator (LASSO) regression. Univariate Cox regression was performed using the “survival” and “survminer” R packages (https://cran.r-project.org/web/packages/survminer). For LASSO regression, the “glmnet” R package was used (https://cran.r-project.org/web/packages/glmnet).
Gene expression patterns identification and tumor immune infiltrating microenvironment analysis
The “ConsensusClusterPlus” R package was used for expression pattern identification between tumor samples (https://bioconductor.org/packages/ConsensusClusterPlus/). The optimal number of clusters was determined using the proportion of ambiguously clustered pairs (PAC) algorithm (26). The immune and stromal score of each sample was first assessed with the “ESTIMATE” algorithm. Then, the infiltration of common 22 immune cells in was assessed using the “CIBERSORT” algorithm. Group contrast boxplots were prepared using the “ggpubr” (https://cran.r-project.org/web/packages/ggpubr) and “ggplot2” R packages.
Tumor hallmark enrichment analysis
The “GSEABase” and “GSVA” R packages and hallmark gene sets (h.all.v7.4.symbols.gmt) were obtained from MSigDB (https://www.gsea-msigdb.org) and used for enrichment analysis. The contrast of enrichment scores was based on prognostically related gene expression patterns.
Prognostic model constr uction of and drug sensitivity analysis
UB_Score model was constructed via multivariate Cox regression:
Internal and external validation was performed on the GEO and TCGA datasets using receiving operator characteristic (ROC) curves. The prognostic model ROC curve was prepared using the “pROC” R package. The nomogram was drawn using the “rms” R package (https://cran.r-project.org/web/packages/rms). For the drug sensitivity analysis, we used the pRRophetic algorithm (27) to estimate each sample’s sensitivity to the drug-using ridge regression.
Statistical analysis
The student’s t-test was used for comparisons between two groups. For comparisons among multiple groups, a one-way ANOVA was used. For all analyses, a P value <0.05 was considered statistically significant. In this article, * represents P value <0.05, ** represents P value <0.01, and *** represents P value <0.001.
Results
Proteomics and ubiquitylome analysis of LUAD ubiquitinated proteins
Following previously published methods for high-throughput liquid chromatography-mass spectrometry (25), we performed proteomic and ubiquitylome assays on five pairs of LUAD and normal lung tissues obtained from clinical samples (https://cdn.amegroups.cn/static/public/atm-21-5645-1.xlsx and https://cdn.amegroups.cn/static/public/atm-21-5645-2.xlsx). To ensure high confidence in the results, we filtered the obtained spectra using a localization probabilities criterion >0.75. Accordingly, we identified 7,330 proteins (6,400 were quantifiable); i.e., quantitative information on protein expression was present in at least one normal and LUAD tissue pair (Figure 1A).
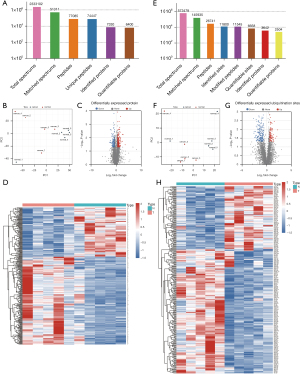
The PCA of all protein expressions revealed that LUAD and normal tissues can be approximately divided into two categories (Figure 1B), demonstrating the technical reproducibility of our data. After a log2 transformation (Log2FoldChange >1.5; P value <0.05) of quantitative protein expression values of all proteins identified, 194 were upregulated and 110 were downregulated in LUAD (Figure 1C). Figure 1D presents the heatmap of all differentially expressed proteins.
Regarding ubiquitylome, we identified 6,658 protein ubiquitylation sites, distributed among 2,504 proteins (Figure 1E). Similarly, the PCA also confirmed data reproducibility (Figure 1F). Of all identified ubiquitination sites, 192 were upregulated and 97 were downregulated (Figure 1G). A quantitative heatmap of all differentially expressed ubiquitination sites is shown in Figure 1H.
Identification of LUAD ubiquitination motifs
We plotted a heat map of 20 amino acids near the lysine modification residues. Isoleucine, lysine, valine, and alanine were commonly distributed near the modified residues (Figure 2A). Also, we evaluated the characteristic motifs of LUAD ubiquitination peptides using the Motif-X algorithm (Figure 2B) (28). According to the scores derived from Motif-X, xxxxxxxxxx_K_LxxxxxxRxx, xxxxxxxxxA_K_xIxxxxxxxx, and xxxxxxxxxx_K_xLxAxxxxxx were the top three ranked characteristic motifs (Figure 2C). They differed completely from previously published LUSC ubiquitination motifs (24), suggesting that the LUAD characteristic ubiquitination motifs are unique.
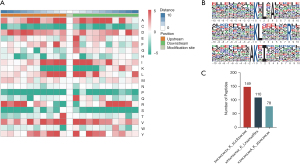
Functional analysis of differentially expressed ubiquitinated proteins
To determine which pathways are regulated by proteins undergoing ubiquitination, we first performed GO/KEGG functional enrichment analyses for all ubiquitinated proteins (Figure 3A). The results revealed that these ubiquitinated proteins regulate numerous biological processes important for lung cancer development and progression, including immune system regulation, protein localization, transmembrane transport of drugs, cell cycle, and various metabolic processes.
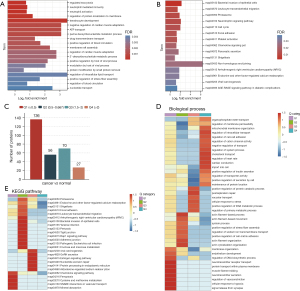
To explore the correlation between protein ubiquitination levels and pathway enrichment, we divided all proteins into four groups according to their differences in modification levels (Figure 3B). Group Q1 was defined as a tumor/normal ratio <0.5 and represented significantly lower levels of ubiquitination in tumors, while Q4 was defined as a ratio >2 and represented a significantly higher level of ubiquitination in tumors. The remaining proteins were divided into the slightly lower ubiquitination modification group (Q2) and the slightly higher ubiquitination modification group (Q3) (Figure 3C). Then, GO/KEGG enrichment analyses were performed separately for the proteins of each group. The heat maps of the results are shown in Figure 3D,3E.
The GO analysis indicated that Q1 category proteins with significantly reduced ubiquitination mainly participated in regulating protein transport receptors within the plasma membrane and in the cellular response to hypoxia, It is well-known that under hypoxic conditions, cancer cells will reorganize their metabolic processes to promote cell growth, survival, proliferation, and long-term maintenance, an effect known as the Warburg effect (29). In contrast, Q4 category proteins with significantly higher ubiquitination were enriched in the regulation of intracellular transport and cell-cell adhesion pathways.
The KEGG enrichment analysis revealed that proteins with significantly reduced ubiquitination (Q1) are involved in both cysteine metabolism and the ferroptosis pathway. Cysteine is the main raw material in the intracellular synthesis of glutathione which, under the action of glutathione peroxidase 4 (GPX4), constitutes the main intracellular antioxidant system resisting the accumulation of lipid reactive oxygen species (ROS). This process is closely associated with the ferroptosis pathway (30). Accordingly, ubiquitination events in LUAD likely influence the ferroptosis pathway.
Identification of ferroptosis-related ubiquitination proteins
To identify ferroptosis-pathway-regulating proteins undergoing ubiquitination in LUAD, we searched the ubiquitylome data with ferroptosis regulatory proteins from the FerrDb database (http://www.zhounan.org/ferrdb/) (Figure 4A). Overall, we identified nine differentially expressed ubiquitinated peptides from six ferroptosis-related proteins (CYBB, SCP2, SLC1A5, DUOX1, KRAS, and CAV1) in LUAD (Figure 4B-4F). CYBB, SCP2, SLC1A5, and DUOX1 are ferroptosis promoters with low ubiquitination levels, whereas KRAS is a ferroptosis promoter with high levels of ubiquitination. Notably, CAV1 was the only ferroptosis suppressor identified and compared to normal tissue, its ubiquitination levels vary at different residues. The ubiquitination sites of these proteins are described further in Table S1.
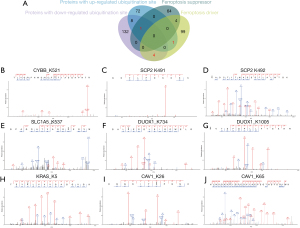
Construction of the ubiquitin–proteasome system (UPS) regulatory network based on the ubiquitinated ferroptosis-related proteins in LUAD
To elucidate the UPS regulatory network of the ubiquitinated proteins, we obtained protein interaction data from the BioGRID database (https://thebiogrid.org/), which was screened using the ubiquitinated regulatory enzymes from the IUUCD database (Figure 5A-5F).
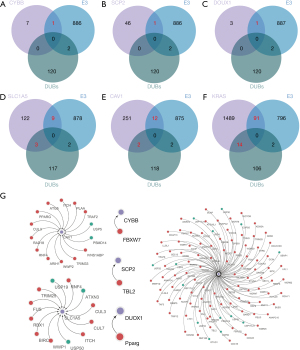
The constructed ubiquitination regulatory network consisting of CYBB, SCP2, SLC1A5, DUOX1, KRAS, and CAV1 is shown in Figure 5G. Among them, KRAS, CAV1, and SLC1A5 are the most extensively studied proteins in LUAD. KRAS has been reported to promote the development of lung cancer in response to OTU deubiquitinase 1 (31), suggesting that KRAS ubiquitination events in LUAD cells are significant. CAV1 has been observed to interact with PSMD14 (32), which was in our constructed regulatory network and therefore further validates its accuracy. Finally, SLC1A5 has been reported to promote lung cancer cell proliferation, invasion, and metastasis (33,34). It can be an independent prognostic biomarker in LUAD patients (35), and its high expression is associated with poor survival (36).
With respect to epigenetic regulation, a circular RNA, circ-LDLRAD3, has been found to upregulate SLC1A5 expression to promote lung cancer cell progression (37). Further, the epigenetic silencing of microRNA-137 in lung cancer enhances SLC1A5 expression to promote cancer cell growth (38). Consequently, we next focused on SLC1A5 ubiquitination and performed a validation experiment.
SLC1A5 is regulated by ubiquitination events in LUAD
We first analyzed our proteomic data from the clinical tissue samples, which revealed that SLC1A5 protein expression was higher in tumors than in normal tissues, although this difference was not statistically significant (Figure 6A). Due to the small sample size of our experiment, we also analyzed the LUAD proteomics data from the CPTAC database (https://cptac-data-portal.georgetown.edu/). Here, we found that SLC1A5 protein expression was significantly higher in LUAD tissues (Figure 6B). Moreover, the Immunol staining data from the Human Protein Atlas database (39-41) (https://www.proteinatlas.org/) confirmed the elevated SLC1A5 protein expression observed in tumors compared to normal tissue (Figure 6C). We also performed western blotting to validate the higher expression of SLC1A5 in tumors (Figure 6D).
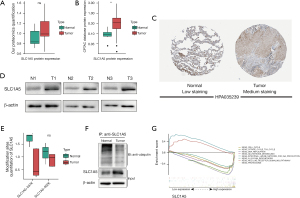
We predicted that ubiquitination may regulate the protein expression levels of SLC1A5. By analyzing the liquid chromatography-mass spectrometry data of all ubiquitinated peptides, we found two types of ubiquitinated peptides that may originate from the SLC1A5 protein. Quantitative analysis of SLC1A5 ubiquitination levels revealed that SLC1A5 is ubiquitinated at lower levels in tumors (Figure 6E). After lysing cancerous and normal tissues, SLC1A5 was purified via immunoprecipitation, after which its ubiquitination levels were quantified using western blot (Figure 6F). The results indicated that SLC1A5 ubiquitination levels in tumors was lower than in normal tissues, suggesting that in LUAD, deubiquitinases play a significant role in regulating SLC1A5 ubiquitination. To investigate whether SLC1A5 also regulates other pathways, we divided the samples into two groups according to SLC1A5 expression levels. The results from the GSEA enrichment analysis indicated that the up-regulation of immune-related pathways results from reduced SLC1A5 expression, which suggests that low SLC1A5 expression may improve the immune microenvironment of tumors. In the high SLC1A5 expression group (Figure 6G), the proteasome and glutamine metabolic pathway enrichment analyses support our findings that SLC1A5 is a ferroptosis-associated protein regulated by ubiquitination. Moreover, the high SLC1A5 expression group was also enriched in N-glycosylation-related pathways, which is consistent with previously published reports of N-glycosylation in SLC1A5 (42). In conclusion, our experiments indicate that SLC1A5 is an essential ferroptosis-regulating protein that in LUAD is regulated by protein ubiquitination.
Genomic analysis of LUAD ubiquitinated proteins and identification of prognostic genes
To better understand the LUAD ubiquitinated substrate proteins obtained above, we performed a series of analyses of the mRNAs encoding these proteins. First, we checked the mutations of these genes in the TCGA database. We found that KRAS, AHNAK, HUWE1, and UBR4 had the highest mutation frequency (Figure 7A). Additionally, in the cBioportal database, we found poor overall survival in patients with mutations in genes encoding ubiquitinated substrates (Figure 7B). It has been reported that wild-type and mutant KARS encoding protein ubiquitination substrates possess different responsiveness to ubiquitinase (43). Targeted ubiquitination of proteins encoded by mutant oncogenes may become a new strategy for cancer treatment. Therefore, understanding mutations in genes encoding ubiquitinated substrate proteins is important.
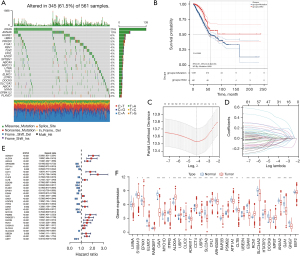
To explore the relationship between these ubiquitinated substrate-encoding genes and patient survival, we collected eight datasets containing patient survival status from the GEO database (GSE11969, GSE13213, GSE29016, GSE30219, GSE31210, GSE42127, GSE50081, and GSE72094) and used univariate Cox regression to obtain prognostically relevant genes (P value <0.01) (https://cdn.amegroups.cn/static/public/atm-21-5645-3.xlsx). To increase the credibility of prognostically related genes, LASSO was used for further screening. Thirty-two prognostically relevant ubiquitinated substrate protein-coding genes were obtained (Figure 7C,7D). The hazard ratio of these genes on prognosis is shown in Figure 7E. Finally, we contrasted these genes’ expressions in tumorous and normal samples (Figure 7F).
Correlation of different prognosis-related ubiquitination protein-encoding genes expression patterns with cancer hallmarks and immune infiltration
Our consensus cluster-based method grouped the expression patterns of prognosis-related ubiquitination protein-encoding genes in all tumor samples from the GEO datasets to mine heterogeneity. In all, 1,244 tumor samples were classified into “UB_Cluster1” and “UB_Cluster2” after clustering (Figure 8A). When we performed survival analysis of these two expression patterns, we found that there was a significant difference between them, and that “UB_Cluster1” overall survival was worse (Figure 8B). Survival differences in this clustering pattern are similarly reflected in the TCGA-LUAD dataset (Figure S1). Figure 8C shows the expression of prognosis-related ubiquitination protein-encoding genes in the two patterns. To further investigate the reason for the survival difference between the two expression patterns, we performed gene set variation analysis (GSVA)-based enrichment analysis of cancer hallmarks for the clusters (Figure 8D). The MYC pathway is one of the major pathways regulating the cell cycle (44), and we found that it was widely activated in “UB_Cluster1”, indicating that tumor cells have a more active cell cycle. Moreover, G2M and E2F checkpoints enrichment also confirmed the “UB_Cluster1” active cell cycle. The epithelial-mesenchymal transition (EMT) pathway had a high enrichment level in “UB_Cluster1”. EMT also plays an important role in lung cancer progression, metastasis, and drug resistance. Altogether, our results indicated that tumors have a more active cell cycle and EMT profile, which may be responsible for the shorter “UB_Cluster1” overall survival.
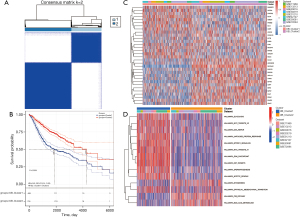
Next, we evaluated the immune and stromal scores in both clusters using the ESTIMATE algorithm (Figure 9A-9D). We found that samples in the “UB_Cluster2” had higher immune and stromal scores, as well as reduced tumor purity—a feature defined in the previous study as an “immunity high” subtype, which has higher sensitivity to immunotherapy and chemotherapy (45). Additionally, most of the immune cell infiltration in “UB_Cluster2” samples were observed to be higher after scoring the immune infiltrating cells in each tumor sample by single-sample gene set enrichment analysis (ssGSEA) (Figure 9E).
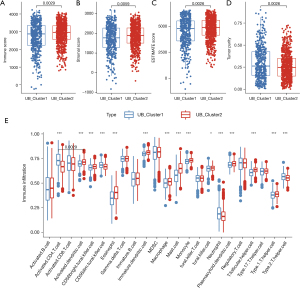
Patterns differential analysis and UB_Score model construction
To thoroughly investigate the differences between the two expression patterns, we identified differentially expressed genes between clusters. We identified 31 differentially expressed genes using a Log |Foldchange >1.5| and a P value <0.001 as criteria. Twenty-nine of them were upregulated and two were downregulated in the “UB_Cluster2” (Figure 10A). Next, we performed a univariate Cox regression analysis of these genes in the full dataset and found that all of them were associated with patients’ overall survival. We found that only two genes (KRT6A and SERPINB5) had hazard ratios >1, and that they were downregulated in the “UB_Cluster2” (Figure 10B), which could explain its better overall survival (Figure 4B). Similarly, we found two Database of Essential Genes (DEG) expression patterns in the samples, “DEG_ClusterA” and “DEG_ClusterB” (Figure 10C). Also, there were survival differences between the DEG expression patterns, and “DEG_ClusterB” overall survival was poor (Figure 10D). Additionally, we plotted the expression of these differentially expressed genes and found that “UB_Cluster1” with poor prognosis possessed a high coincidence rate with “DEG_ClusterB” (Figure 10E). Finally, to quantify the relationship between this differential expression pattern and overall survival, we constructed a quantitative value formula (UB_Score) for the DEGs expression pattern using multivariate Cox regression with these differentially expressed genes (Figure 10F,10G).
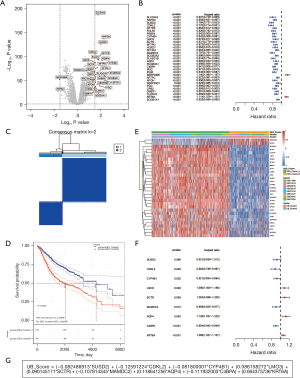
Evaluation and validation of the UB_Score model for prognosis prediction and nomogram construction
We set the GEO-dataset as the training group and calculated UB_Score values of all samples with the model obtained above. The median value of all sample scores was the cut-off value, and samples were divided into high-and low-score groups (Figure 11A). After comparing the two groups' survival time, we found that the high-score group’s survival was worse compared to that of the low-score group (Figure 11B). The UB_Score model predictive effect ROC curve on patients’ prognosis showed a good predictive effect (Figure 11C). Furthermore, we validated the model in the TCGA-LUAD dataset and results showed that the UB_Score model had a good predictive ability (Figure 11D,11E). After introducing other clinical indicators, we performed univariate (Figure 11F) and multivariate (Figure 11G) Cox regression analyses, and the UB_Score P value was <0.001. This demonstrated that the UB_Score is an independent prognostic factor for LUAD patients. Moreover, the area under the curve (AUC) of the UB_Score model ROC curve was greater than that of other clinical indicators. This indicates that our model is superior to other clinical indicators to predict LUAD patients’ prognosis (Figure 11H). Finally, the nomogram of UB_Score and other clinical indicators was constructed (Figure 11I). The calibration curves show that our constructed nomogram has a good predictive value (Figure S2).
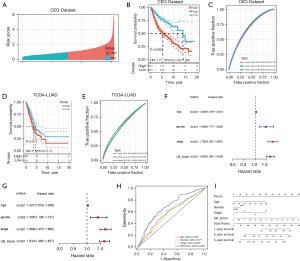
UB_Score can be used as a reference for LUAD clinical treatment
We compared PD1 and CTLA4 expression between the two patient groups (high or low UB_score) (Figure 12A,12B). Results showed that the expression of these two immune checkpoints was significantly higher in the high UB_score group. This indicates that timely immunotherapy in patients with a high UB_score improves survival. Additionally, it has been reported that the combination of PD1 and CTLA4 inhibitors leads to better results (46). We also evaluated the sensitivity of common chemotherapy and targeted therapy drugs in the two groups (Figure 12C-12H). Results showed that the high UB_Score group had a low half maximal inhibitory concentration (IC50) to multiple drugs, indicating that patients in this group are more sensitive when using these drugs. Therefore, our findings suggest that the constructed UB_Score model can be used as a clinical treatment guide for LUAD patients, and that timely clinical intervention may lead to better survival outcomes.
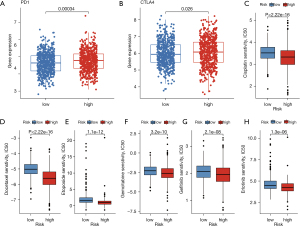
Discussion
It has been reported that protein degradation in mammals is mainly through two pathways, the autophagy-lysosome pathway, and the ubiquitin-proteasome pathway (18). E3 ubiquitinates are generally considered as regulators mediating protein degradation, and substrate proteins are degraded via the ubiquitin-proteasome system. But, when ubiquitin-proteasome regulation is unbalanced, a variety of biological processes that may induce the development of cancers are activated, mainly involving metabolic reprogramming, regulation of cell death, adaptation to stress response, regulation of signal transduction pathways, DNA damage repair (47), and tumor microenvironment changes (48), etc. In addition, deubiquitinating enzymes also stabilize oncogenic proteins by deubiquitinating modifications and play an important role in the development of a variety of tumors (49-51). Drug resistance is a major problem in the current clinical application of anticancer drugs. Overexpression of drug efflux proteins or low expression of drug influx proteins, as well as mutations in drug direct targets, are the main mechanisms by which cancer cells develop resistance to anticancer drugs (52). Ubiquitinase and deubiquitinase, therefore, make them effective therapeutic targets against drug resistance by epigenetic regulation of a variety of cancer-related pathways and their ability to regulate atypical drug targets such as transcription factors and skeleton proteins (53). It has been reported that deubiquitinase is abnormally activated in a variety of cancers, resulting in increased protein expression related to drug efflux and changes in drug target molecules (54), activating a variety of oncogenic pathways and forming drug resistance (55-57), so deubiquitinase has the potential to be developed as drug targets. It is therefore essential to study the ubiquitylome of cancers to provide new ideas for new drug target development.
Due to recent advances in mass spectrometry technology, our understanding of proteomics has grown and various protein PTMs have been discovered. To clarify the LUAD proteins undergoing ubiquitination, we performed ubiquitylome and proteomic analysis using a new mass spectrometry technique (25). Overall, we identified 11,545 ubiquitination sites among 3,642 LUAD proteins. Moreover, we identified for the first time unique ubiquitination peptides motifs in LUAD by analyzing the modified peptides. At the genetic level, we found different expression patterns of LUAD ubiquitinated protein-coding genes, revealed heterogeneity among tumor samples, and found that different expression patterns can lead to different tumor immune infiltration microenvironment landscapes. Finally, by analyzing the differences between expression patterns, we constructed a survival prediction model for LUAD patients, which can be used as a guide for clinical treatment.
Although ubiquitylome and proteomics analysis in LUSC have been reported (24), we are the first to systematically carry out LUAD proteomics and ubiquitylome. We added ion mobility to the original liquid chromatography-mass spectrometry analysis index (25), such that our results are more sensitive and accurate. We found that the characteristic amino acid motifs “xxxxxxxxxx_K_LxxxxxxRxx”, “xxxxxxxxxA_K_xIxxxxxxxx”, and “xxxxxxxxxx_K_xLxAxxxxxx” are prone to ubiquitination in LUAD. These motifs differ completely from previous LUSC-related reports. This difference may be due to the different pathogenetic molecular mechanisms and tumor properties between LUAD and LUSC. It is predicted that different lung cancer types have different protein ubiquitination landscapes, which has implications for LUAD treatment strategies targeting protein ubiquitination pathways. Previous studies reported that wild-type and mutant KRAS respond differently to ubiquitinase, thus providing new therapeutic strategies to target mutant LUAD oncogenic proteins (43). Therefore, we also studied mutations in genes encoding LUAD ubiquitinated substrate proteins to provide new insights for subsequent studies. Protein ubiquitination is a type of post-translational protein modification. Ubiquitinase often affects numerous biological processes by regulating substrate proteins. Therefore, we focused on the expression patterns of genes encoding substrate proteins and found differences between them regarding cancer hallmarks and the immune infiltration microenvironment. After expression patterns differential analysis, we constructed a prognostic model to quantify the relationship between expression patterns and prognosis, and our model had a good predictive ability. Moreover, in the immune checkpoint and drug sensitivity analysis for all samples, we observed that our model can be used as a guide for clinical lung cancer treatment.
In short, we are the first to systematically analyze LUAD ubiquitylome. We identified proteins with differential ubiquitination levels in LUAD compared to normal lung tissues, thus providing important insights for further investigation of the protein ubiquitination mechanism in LUAD. Additionally, gene-level analysis of these substrate proteins revealed heterogeneity among tumor samples. Then, we constructed a prognostic model to quantify the relationship between expression patterns and prognosis, which can be used as a guide for LUAD clinical treatment. However, this study is limited by the small ubiquitination sample size. Therefore, a study with a larger sample size would provide more detailed information on which to base clinical guidance. In future studies, we plan to study the protein ubiquitination regulatory mechanism in LUAD to expand its clinical treatment indications. This study provides the basis for the study of protein ubiquitination modification mechanisms in LUAD and provides a new perspective for the development of therapeutic agents based on ubiquitin-proteasome mechanisms to overcome existing drug resistance. In addition, we also identified different molecular subtypes of LUAD by genomics analysis, reflecting the heterogeneity among tumors and emphasizing the importance of individualized treatment. The constructed prognostic prediction model also provides a reference for timely intervention and treatment of patients, but its application requires targeted sequencing of the genes in the model, so its economic benefits and feasibility need to be further verified.
Conclusions
In this research, we used an anti-K-ε-GG-based ubiquitination peptide enrichment combined with liquid chromatography-mass spectrometry to obtain LUAD ubiquitylome data. A series of analyses were performed to reveal the protein ubiquitination landscape in LUAD, and unique ubiquitinated peptide motifs were found. Additionally, further analysis of their gene expression patterns was performed, and we found that patterns are related to multiple drug sensitivity. Our work addresses the lack of ubiquitylome studies in LUAD and provides new perspectives for subsequent research and clinical treatment.
Acknowledgments
Funding: National Key Research and Development Plan (No. 2017YFC1308700), the Ministry of Science and Technology of the People’s Republic of China.
Footnote
Reporting Checklist: The authors have completed the MDAR reporting checklist. Available at https://dx.doi.org/10.21037/atm-21-5645
Data Sharing Statement: Available at https://dx.doi.org/10.21037/atm-21-5645
Conflicts of Interest: All authors have completed the ICMJE uniform disclosure form (available at https://dx.doi.org/10.21037/atm-21-5645). The authors have no conflicts of interest to declare.
Ethical Statement: The authors are accountable for all aspects of the work in ensuring that questions related to the accuracy or integrity of any part of the work are appropriately investigated and resolved. The study was approved by the Ethics Committee of the Second Affiliated Hospital of Harbin Medical University (NO.: KY2021-166) and written informed consent was obtained from all patients. All procedures performed in this study involving human participants were in accordance with the Declaration of Helsinki (as revised in 2013).
Open Access Statement: This is an Open Access article distributed in accordance with the Creative Commons Attribution-NonCommercial-NoDerivs 4.0 International License (CC BY-NC-ND 4.0), which permits the non-commercial replication and distribution of the article with the strict proviso that no changes or edits are made and the original work is properly cited (including links to both the formal publication through the relevant DOI and the license). See: https://creativecommons.org/licenses/by-nc-nd/4.0/.
References
- Siegel RL, Miller KD, Fuchs HE, et al. Cancer Statistics, 2021. CA Cancer J Clin 2021;71:7-33. [Crossref] [PubMed]
- Halliday PR, Blakely CM, Bivona TG. Emerging Targeted Therapies for the Treatment of Non-small Cell Lung Cancer. Curr Oncol Rep 2019;21:21. [Crossref] [PubMed]
- Mayekar MK, Bivona TG. Current Landscape of Targeted Therapy in Lung Cancer. Clin Pharmacol Ther 2017;102:757-64. [Crossref] [PubMed]
- Martinez P, Peters S, Stammers T, et al. Immunotherapy for the First-Line Treatment of Patients with Metastatic Non-Small Cell Lung Cancer. Clin Cancer Res 2019;25:2691-8. [Crossref] [PubMed]
- Sandoval J, Mendez-Gonzalez J, Nadal E, et al. A prognostic DNA methylation signature for stage I non-small-cell lung cancer. J Clin Oncol 2013;31:4140-7. [Crossref] [PubMed]
- Chen Y, Liu X, Li Y, et al. Lung Cancer Therapy Targeting Histone Methylation: Opportunities and Challenges. Comput Struct Biotechnol J 2018;16:211-23. [Crossref] [PubMed]
- Osielska MA, Jagodziński PP. Long non-coding RNA as potential biomarkers in non-small-cell lung cancer: What do we know so far? Biomed Pharmacother 2018;101:322-33. [Crossref] [PubMed]
- Lin H, Caroll KS. Introduction: Posttranslational Protein Modification. Chem Rev 2018;118:887-8. [Crossref] [PubMed]
- Swatek KN, Komander D. Ubiquitin modifications. Cell Res 2016;26:399-422. [Crossref] [PubMed]
- Mansour MA. Ubiquitination: Friend and foe in cancer. Int J Biochem Cell Biol 2018;101:80-93. [Crossref] [PubMed]
- Weake VM, Workman JL. Histone ubiquitination: triggering gene activity. Mol Cell 2008;29:653-63. [Crossref] [PubMed]
- Lu Z, Hunter T. Degradation of activated protein kinases by ubiquitination. Annu Rev Biochem 2009;78:435-75. [Crossref] [PubMed]
- Foot N, Henshall T, Kumar S. Ubiquitination and the Regulation of Membrane Proteins. Physiol Rev 2017;97:253-81. [Crossref] [PubMed]
- Park HB, Kim JW, Baek KH. Regulation of Wnt Signaling through Ubiquitination and Deubiquitination in Cancers. Int J Mol Sci 2020;21:3904. [Crossref] [PubMed]
- Sun T, Liu Z, Yang Q. The role of ubiquitination and deubiquitination in cancer metabolism. Mol Cancer 2020;19:146. [Crossref] [PubMed]
- Shimizu Y, Taraborrelli L, Walczak H. Linear ubiquitination in immunity. Immunol Rev 2015;266:190-207. [Crossref] [PubMed]
- Chang SC, Ding JL. Ubiquitination and SUMOylation in the chronic inflammatory tumor microenvironment. Biochim Biophys Acta Rev Cancer 2018;1870:165-75. [Crossref] [PubMed]
- Wu T, Yoon H, Xiong Y, et al. Targeted protein degradation as a powerful research tool in basic biology and drug target discovery. Nat Struct Mol Biol 2020;27:605-14. [Crossref] [PubMed]
- Veggiani G, Gerpe MCR, Sidhu SS, et al. Emerging drug development technologies targeting ubiquitination for cancer therapeutics. Pharmacol Ther 2019;199:139-54. [Crossref] [PubMed]
- Popovic D, Vucic D, Dikic I. Ubiquitination in disease pathogenesis and treatment. Nat Med 2014;20:1242-53. [Crossref] [PubMed]
- Wang Y, Yang L, Zhang X, et al. Epigenetic regulation of ferroptosis by H2B monoubiquitination and p53. EMBO Rep 2019;20:e47563 [Crossref] [PubMed]
- Huang Y, Yang X, Lu Y, et al. UBE2O targets Mxi1 for ubiquitination and degradation to promote lung cancer progression and radioresistance. Cell Death Differ 2021;28:671-84. [Crossref] [PubMed]
- Li L, Zhang W, Liu Y, et al. The CRL3BTBD9 E3 ubiquitin ligase complex targets TNFAIP1 for degradation to suppress cancer cell migration. Signal Transduct Target Ther 2020;5:42. [Crossref] [PubMed]
- Lu M, Chen W, Zhuang W, et al. Label-free quantitative identification of abnormally ubiquitinated proteins as useful biomarkers for human lung squamous cell carcinomas. EPMA J 2020;11:73-94. [Crossref] [PubMed]
- Meier F, Brunner AD, Koch S, et al. Online Parallel Accumulation-Serial Fragmentation (PASEF) with a Novel Trapped Ion Mobility Mass Spectrometer. Mol Cell Proteomics 2018;17:2534-45. [Crossref] [PubMed]
- Șenbabaoğlu Y, Michailidis G, Li JZ. Critical limitations of consensus clustering in class discovery. Sci Rep 2014;4:6207. [Crossref] [PubMed]
- Geeleher P, Cox N, Huang RS. pRRophetic: an R package for prediction of clinical chemotherapeutic response from tumor gene expression levels. PLoS One 2014;9:e107468 [Crossref] [PubMed]
- Cheng A, Grant CE, Noble WS, et al. MoMo: discovery of statistically significant post-translational modification motifs. Bioinformatics 2019;35:2774-82. [Crossref] [PubMed]
- Liberti MV, Locasale JW. The Warburg Effect: How Does it Benefit Cancer Cells? Trends Biochem Sci 2016;41:211-8. [Crossref] [PubMed]
- Li J, Cao F, Yin HL, et al. Ferroptosis: past, present and future. Cell Death Dis 2020;11:88. [Crossref] [PubMed]
- Baietti MF, Simicek M, Abbasi Asbagh L, et al. OTUB1 triggers lung cancer development by inhibiting RAS monoubiquitination. EMBO Mol Med 2016;8:288-303. [Crossref] [PubMed]
- Wang B, Xu X, Yang Z, et al. POH1 contributes to hyperactivation of TGF-β signaling and facilitates hepatocellular carcinoma metastasis through deubiquitinating TGF-β receptors and caveolin-1. EBioMedicine 2019;41:320-32. [Crossref] [PubMed]
- Hassanein M, Hoeksema MD, Shiota M, et al. SLC1A5 mediates glutamine transport required for lung cancer cell growth and survival. Clin Cancer Res 2013;19:560-70. [Crossref] [PubMed]
- Cormerais Y, Massard PA, Vucetic M, et al. The glutamine transporter ASCT2 (SLC1A5) promotes tumor growth independently of the amino acid transporter LAT1 (SLC7A5). J Biol Chem 2018;293:2877-87. [Crossref] [PubMed]
- Shimizu K, Kaira K, Tomizawa Y, et al. ASC amino-acid transporter 2 (ASCT2) as a novel prognostic marker in non-small cell lung cancer. Br J Cancer 2014;110:2030-9. [Crossref] [PubMed]
- Csanadi A, Oser A, Aumann K, et al. Overexpression of SLC1a5 in lymph node metastases outperforms assessment in the primary as a negative prognosticator in non-small cell lung cancer. Pathology 2018;50:269-75. [Crossref] [PubMed]
- Xue M, Hong W, Jiang J, et al. Circular RNA circ-LDLRAD3 serves as an oncogene to promote non-small cell lung cancer progression by upregulating SLC1A5 through sponging miR-137. RNA Biol 2020;17:1811-22. [Crossref] [PubMed]
- Dong J, Xiao D, Zhao Z, et al. Epigenetic silencing of microRNA-137 enhances ASCT2 expression and tumor glutamine metabolism. Oncogenesis 2017;6:e356 [Crossref] [PubMed]
- Uhlen M, Zhang C, Lee S, et al. A pathology atlas of the human cancer transcriptome. Science 2017;357:eaan2507 [Crossref] [PubMed]
- Uhlén M, Fagerberg L, Hallström BM, et al. Proteomics. Tissue-based map of the human proteome. Science 2015;347:1260419 [Crossref] [PubMed]
- Thul PJ, Åkesson L, Wiking M, et al. A subcellular map of the human proteome. Science 2017;356:eaal3321 [Crossref] [PubMed]
- Console L, Scalise M, Tarmakova Z, et al. N-linked glycosylation of human SLC1A5 (ASCT2) transporter is critical for trafficking to membrane. Biochim Biophys Acta 2015;1853:1636-45. [Crossref] [PubMed]
- Shukla S, Allam US, Ahsan A, et al. KRAS protein stability is regulated through SMURF2: UBCH5 complex-mediated β-TrCP1 degradation. Neoplasia 2014;16:115-28. [Crossref] [PubMed]
- Zajac-Kaye M. Myc oncogene: a key component in cell cycle regulation and its implication for lung cancer. Lung Cancer 2001;34:S43-6. [Crossref] [PubMed]
- Xu F, Chen JX, Yang XB, et al. Analysis of Lung Adenocarcinoma Subtypes Based on Immune Signatures Identifies Clinical Implications for Cancer Therapy. Mol Ther Oncolytics 2020;17:241-9. [Crossref] [PubMed]
- Paz-Ares L, Ciuleanu TE, Cobo M, et al. First-line nivolumab plus ipilimumab combined with two cycles of chemotherapy in patients with non-small-cell lung cancer (CheckMate 9LA): an international, randomised, open-label, phase 3 trial. Lancet Oncol 2021;22:198-211. [Crossref] [PubMed]
- Senft D, Qi J, Ronai ZA. Ubiquitin ligases in oncogenic transformation and cancer therapy. Nat Rev Cancer 2018;18:69-88. [Crossref] [PubMed]
- Deng L, Meng T, Chen L, et al. The role of ubiquitination in tumorigenesis and targeted drug discovery. Signal Transduct Target Ther 2020;5:11. [Crossref] [PubMed]
- Wu C, Luo K, Zhao F, et al. USP20 positively regulates tumorigenesis and chemoresistance through β-catenin stabilization. Cell Death Differ 2018;25:1855-69. [Crossref] [PubMed]
- Wang X, Xia S, Li H, et al. The deubiquitinase USP10 regulates KLF4 stability and suppresses lung tumorigenesis. Cell Death Differ 2020;27:1747-64. [Crossref] [PubMed]
- Hong K, Hu L, Liu X, et al. USP37 promotes deubiquitination of HIF2α in kidney cancer. Proc Natl Acad Sci U S A 2020;117:13023-32. [Crossref] [PubMed]
- Narayanan S, Cai CY, Assaraf YG, et al. Targeting the ubiquitin-proteasome pathway to overcome anti-cancer drug resistance. Drug Resist Updat 2020;48:100663 [Crossref] [PubMed]
- Lu J, Qian Y, Altieri M, et al. Hijacking the E3 Ubiquitin Ligase Cereblon to Efficiently Target BRD4. Chem Biol 2015;22:755-63. [Crossref] [PubMed]
- Tanguturi P, Kim KS, Ramakrishna S. The role of deubiquitinating enzymes in cancer drug resistance. Cancer Chemother Pharmacol 2020;85:627-39. [Crossref] [PubMed]
- Zhang Q, Zhang ZY, Du H, et al. DUB3 deubiquitinates and stabilizes NRF2 in chemotherapy resistance of colorectal cancer. Cell Death Differ 2019;26:2300-13. [Crossref] [PubMed]
- Zhang Q, Wang W, Gao Q. β-TRCP-mediated AEBP2 ubiquitination and destruction controls cisplatin resistance in ovarian cancer. Biochem Biophys Res Commun 2020;523:274-9. [Crossref] [PubMed]
- Yan Y, Xu Z, Huang J, et al. The deubiquitinase USP36 Regulates DNA replication stress and confers therapeutic resistance through PrimPol stabilization. Nucleic Acids Res 2020;48:12711-26. [Crossref] [PubMed]
(English Language Editor: B. Meiser)