Development and validation of the EHS-COPD model to predict sex-specific risk of chronic obstructive pulmonary disease (COPD) in older Chinese adults: Hong Kong’s Elderly Health Service Cohort
Introduction
Chronic obstructive pulmonary disease (COPD) and its complications are major causes of morbidity, mortality, and hospitalizations worldwide (1). In China, more than 86 million of ~633.0 million adults aged 40+ years were estimated to have COPD in 2014–2015, with the total cost of COPD ranging from US $1,964 to $3,449 per patient, representing 33–40% of the average household income (2-4). In Hong Kong, ~0.5% of the non-institutionalized population aged 15+ years had COPD in 2014–2015 (~43.5% were older adults aged 65 years or above), which resulted in ~2.7% of all registered deaths in 2017 (5). COPD is associated with substantial morbidity, increased healthcare use, disability, and death (1,6). Up to ~70% of COPD cases are undiagnosed and the prevalence of COPD is increasing globally (7-9), raising the possibility that earlier identification of those at high risk of COPD for suitable interventions could be beneficial.
Screening for COPD is not currently recommended in adults (10), because there is no direct evidence showing benefits of screening asymptomatic adults for COPD using either questionnaires or office-based screening via pulmonary function testing. With recent clinical trials demonstrating that smoking cessation (11-13) and pharmaceutical interventions (12,14-16) in people with mild or moderate COPD can ameliorate the decline of lung function and delay the risk of COPD exacerbations, identifying people at high risk of developing COPD using risk prediction models for targeted interventions might help reduce the burden of COPD.
To date, several risk prediction models have been developed for COPD, which either incorporate well-established risk factors (e.g., age and smoking) (17,18) or are restricted to asthma patients (19) from specific cohorts, limiting their application to the general population. In China, risk prediction models for COPD, including environmental, population, and genetic attributes (20) or peak expiratory flow measurement (21), are recommended for screening people at high risk. However, the inclusion of genetics or hard-to-measure factors limits their use in primary care. Existing risk prediction models do not always generalize to new settings because of health-related differences between populations. For example, the most widely used risk prediction models developed in Western settings, e.g., the Framingham cardiovascular disease score (22) and the Framingham diabetes score (23), have not always been applicable in a Chinese setting even after recalibration (24).
In this study, we first assessed the performance of an existing risk prediction model developed by Kotz et al. (17) in a Western setting, which uses four risk factors (i.e., age, smoking, prior asthma, and deprivation), in a Chinese setting. Secondly, we investigated whether a new model to predict COPD risk within the next 5 years in older Chinese adults (65+ years) performed better, based on a large population-based cohort, i.e., Hong Kong’s Elderly Health Services (EHS) Cohort. We followed the Transparent reporting of a multivariable model for individual prognosis or diagnosis (TRIPOD) guidance for development and reporting of a new model (available at https://dx.doi.org/10.21037/atm-21-3270) (25).
Methods
Study design and data source
The EHS cohort is a large, contemporary, prospective cohort study of older Chinese adults aged 65+ years in Hong Kong enrolled at 18 territory-wide Elderly Health Centres. Baseline and follow-up data were provided by the Department of Health, as detailed elsewhere (26). Specifically, the study aimed to promote understanding of aging in a global context and to take advantage of Hong Kong as a sentinel for Chinese populations currently experiencing very rapid economic development. The initial cohort enrolled ~66,820 participants from July 1998 to December 2001 at the Elderly Health Centres. All older adults in Hong Kong were encouraged to enroll at a nominal annual fee (22,26). At the centres, nurses and doctors provided physical check-ups and health assessments using clinical examinations and structured interviews, including demographics, lifestyle, socio-economic position, health status, self-rated health, physical functioning, social contact, depressive symptoms, and cognitive functioning. Self-reports were supplemented by clinical diagnoses based on medical history, if available. The participants were followed up at subsequent visits. Vital status was ascertained via record linkage to the Hong Kong Deaths Registry. Up to December 31, 2012, ~136,309 participants were enrolled in the EHS cohort, which was about 13.6% of the Hong Kong population aged 65+ years in 2012. This study excluded participants with existing COPD at baseline or those with >50% missing values for all predictors. For each participant, the entry date was the date of the baseline health assessment. Participants were censored at the earliest of date of death, attrition, or study end date (December 31, 2015). We randomly allocated two-thirds of the participants to the derivation cohort and the remainder to the validation cohort.
Outcomes
The outcome was the 5-year predicted risk of COPD. Self-reported new COPD cases were identified during follow-up visits. The time to event was measured from entry to date of self-report of a new COPD case.
Candidate risk predictors
We first assessed the performance of the model developed by Kotz et al. (17) using similar risk predictors [i.e., age, a measure of deprivation (Carstairs index), asthma, and smoking history]. We also selected additional potential risk predictors, available for the EHS cohort, from the relevant literature (Box 1).
Table 1
Characteristics | Derivation cohort | Validation cohort | |||||
---|---|---|---|---|---|---|---|
Men (n=32,222) | Women (n=58,911) | Overall (n=91,133) | Men (n=15,690) | Women (n=28,999) | Overall (n=44,689) | ||
Age (years), mean (SD) | 72.18 (5.20) | 71.68 (5.37) | 71.86 (5.31) | 72.11 (5.12) | 71.69 (5.40) | 71.84 (5.31) | |
Type of housing | |||||||
Public or aided | 14,221 (44.1) | 26,488 (45.0) | 40,709 (44.7) | 6,935 (44.2) | 13,016 (44.9) | 19,951 (44.6) | |
Private (rented) | 1,336 (4.1) | 2,347 (4.0) | 3,683 (4.0) | 693 (4.4) | 1,147 (4.0) | 1,840 (4.1) | |
Private (self-owned) | 15,517 (48.2) | 27,154 (46.1) | 42,671 (46.8) | 7,484 (47.7) | 13,360 (46.1) | 20,844 (46.6) | |
Others | 1,146 (3.6) | 2,920 (5.0) | 4,066 (4.5) | 578 (3.7) | 1,476 (5.1) | 2,054 (4.6) | |
No. of missing values | 2 (0.0) | 2 (0.0) | 4 (0.0) | NA | NA | NA | |
Public assistance | |||||||
Yes | 4,301 (13.3) | 8,187 (13.9) | 12,488 (13.7) | 2,005 (12.8) | 4,053 (14.0) | 6,058 (13.6) | |
No | 27,915 (86.6) | 50,717 (86.1) | 78,632 (86.3) | 13,685 (87.2) | 24,942 (86.0) | 38,627 (86.4) | |
No. of missing values | 6 (0.0) | 7 (0.0) | 13 (0.0) | 0 (0.0) | 4 (0.0) | 4 (0.0) | |
Education | |||||||
Illiterate | 2,089 (6.5) | 19,994 (33.9) | 22,083 (24.2) | 990 (6.3) | 9,790 (33.8) | 10,780 (24.1) | |
Literate but no formal education | 2,948 (9.1) | 9,659 (16.4) | 12,607 (13.8) | 1,428 (9.1) | 4,845 (16.7) | 6,273 (14.0) | |
Primary | 14,638 (45.4) | 19,280 (32.7) | 33,918 (37.2) | 7,152 (45.6) | 9,353 (32.3) | 16,505 (36.9) | |
Secondary or above | 12,539 (38.9) | 9,962 (16.9) | 22,501 (24.7) | 6,118 (39.0) | 5,006 (17.3) | 11,124 (24.9) | |
No. of missing values | 8 (0.0) | 16 (0.0) | 24 (0.0) | 2 (0.0) | 5 (0.0) | 7 (0.0) | |
Body mass index (kg/m2) | |||||||
<18.5 | 1,593 (4.9) | 2,707 (4.6) | 4,300 (4.7) | 827 (5.3) | 1,332 (4.6) | 2,159 (4.8) | |
18.5–<23.0 | 11,021 (34.2) | 19,030 (32.3) | 30,051 (33.0) | 5,403 (34.4) | 9,512 (32.8) | 14,915 (33.4) | |
23.0–<25.0 | 8,188 (25.4) | 13,040 (22.1) | 21,228 (23.3) | 3,794 (24.2) | 6,494 (22.4) | 10,288 (23.0) | |
25.0+ | 11,415 (35.4) | 24,132 (41.0) | 35,547 (39.0) | 5,665 (36.1) | 11,657 (40.2) | 17,322 (38.8) | |
No. of missing values | 5 (0.0) | 2 (0.0) | 7 (0.0) | 1 (0.0) | 4 (0.0) | 5 (0.0) | |
Smoking status | |||||||
Never smoker | 12,864 (39.9) | 52,572 (89.2) | 65,436 (71.8) | 6,351 (40.5) | 26,017 (89.7) | 32,368 (72.4) | |
Ex-smoker | 13,710 (42.5) | 3,813 (6.5) | 17,523 (19.2) | 6,576 (41.9) | 1,744 (6.0) | 8,320 (18.6) | |
Current smoker | 5,569 (17.3) | 1,715 (2.9) | 7,284 (8.0) | 2,737 (17.4) | 813 (2.8) | 3,550 (7.9) | |
No. of missing values | 79 (0.2) | 811 (1.4) | 890 (1.0) | 26 (0.2) | 425 (1.5) | 451 (1.0) | |
Alcohol use | |||||||
Never drinker | 21,645 (67.2) | 53,081 (90.1) | 74,726 (82.0) | 10,589 (67.5) | 26,174 (90.3) | 36,763 (82.3) | |
Ex-drinker | 3,156 (9.8) | 1,842 (3.1) | 4,998 (5.5) | 1,521 (9.7) | 905 (3.1) | 2,426 (5.4) | |
Social drinker | 4,440 (13.8) | 3,324 (5.6) | 7,764 (8.5) | 2,109 (13.4) | 1,610 (5.6) | 3,719 (8.3) | |
Moderate drinker | 1,686 (5.2) | 410 (0.7) | 2,096 (2.3) | 854 (5.4) | 202 (0.7) | 1,056 (2.4) | |
Excessive drinker | 1,288 (4.0) | 251 (0.4) | 1,539 (1.7) | 615 (3.9) | 104 (0.4) | 719 (1.6) | |
No. of missing values | 7 (0.0) | 3 (0.0) | 10 (0.0) | 2 (0.0) | 4 (0.0) | 6 (0.0) | |
Physical activity (hours per week) | |||||||
None | 4,215 (13.1) | 7,104 (12.1) | 11,319 (12.4) | 2,000 (12.7) | 3,494 (12.0) | 5,494 (12.3) | |
<1.5 | 2,516 (7.8) | 4,943 (8.4) | 7,459 (8.2) | 1,258 (8.0) | 2,442 (8.4) | 3,700 (8.3) | |
1.5–<3.0 | 5,017 (15.6) | 10,185 (17.3) | 15,202 (16.7) | 2,515 (16.0) | 4,880 (16.8) | 7,395 (16.5) | |
3.0–<4.5 | 3,983 (12.4) | 7,157 (12.1) | 11,140 (12.2) | 1,844 (11.8) | 3,482 (12.0) | 5,326 (11.9) | |
4.5+ | 16,485 (51.2) | 29,513 (50.1) | 45,998 (50.5) | 8,073 (51.5) | 14,699 (50.7) | 22,772 (51.0) | |
No. of missing values | 6 (0.0) | 9 (0.0) | 15 (0.0) | 0 (0.0) | 2 (0.0) | 2 (0.0) | |
Family history of cardiovascular diseases | |||||||
No | 15,333 (47.6) | 28,691 (48.7) | 44,024 (48.3) | 7,445 (47.5) | 14,060 (48.5) | 21,505 (48.1) | |
Yes | 2,715 (8.4) | 5,655 (9.6) | 8,370 (9.2) | 1,417 (9.0) | 2,858 (9.9) | 4,275 (9.6) | |
No. of missing values | 14,174 (44.0) | 24,565 (41.7) | 38,739 (42.5) | 6,828 (43.5) | 12,081 (41.7) | 18,909 (42.3) | |
Hypertension (yes) | 702 (2.2) | 1,322 (2.2) | 2,024 (2.2) | 337 (2.1) | 586 (2.0) | 923 (2.1) | |
Asthma (yes) | 24 (0.1) | 85 (0.1) | 109 (0.1) | 15 (0.1) | 32 (0.1) | 47 (0.1) | |
Recent falls | |||||||
Yes | 274 (0.9) | 922 (1.6) | 1,196 (1.3) | 134 (0.9) | 474 (1.6) | 608 (1.4) | |
No | 31,939 (99.1) | 57,978 (98.4) | 89,917 (98.7) | 15,556 (99.1) | 28,517 (98.3) | 44,073 (98.6) | |
No. of missing values | 9 (0.0) | 11 (0.0) | 20 (0.0) | NA | 8 (0.0) | 8 (0.0) | |
Hospital admission (yes) | |||||||
Yes | 4,554 (14.1) | 7,075 (12.0) | 11,629 (12.8) | 2,189 (14.0) | 3,442 (11.9) | 5,631 (12.6) | |
No | 27,666 (85.9) | 51,835 (88.0) | 79,501 (87.2) | 13,501 (86.0) | 25,557 (88.1) | 39,058 (87.4) | |
No. of missing values | 2 (0.0) | 1 (0.0) | 3 (0.0) | NA | NA | NA | |
Physical functioning | |||||||
Normal | 30,509 (94.7) | 54,021 (91.7) | 84,530 (92.8) | 14,858 (94.7) | 26,571 (91.6) | 41,429 (92.7) | |
Poor | 1,588 (4.9) | 4,672 (7.9) | 6,260 (6.9) | 770 (4.9) | 2,316 (8.0) | 3,086 (6.9) | |
No. of missing values | 125 (0.4) | 218 (0.4) | 343 (0.4) | 62 (0.4) | 112 (0.4) | 174 (0.4) | |
Cognitive function | |||||||
Normal | 31,268 (97.0) | 54,246 (92.1) | 85,514 (93.8) | 15,272 (97.3) | 26,708 (92.1) | 41,980 (93.9) | |
Poor | 889 (2.8) | 4,557 (7.7) | 5,446 (6.0) | 390 (2.5) | 2,245 (7.7) | 2,635 (5.9) | |
No. of missing values | 65 (0.2) | 108 (0.2) | 173 (0.2) | 28 (0.2) | 46 (0.2) | 74 (0.2) | |
Depressive symptoms | |||||||
No/fewer | 30,446 (94.5) | 53,643 (91.1) | 84,089 (92.3) | 14,850 (94.6) | 26,430 (91.1) | 41,280 (92.4) | |
More | 1,714 (5.3) | 5,143 (8.7) | 6,857 (7.5) | 824 (5.3) | 2,512 (8.7) | 3,336 (7.5) | |
No. of missing values | 62 (0.2) | 25 (0.2) | 187 (0.2) | 16 (0.1) | 57 (0.2) | 73 (0.2) | |
Self-rated health status | |||||||
Better | 19,794 (61.4) | 33,412 (56.7) | 53,206 (58.4) | 9,701 (61.8) | 16,383 (56.5) | 26,084 (58.4) | |
Normal | 2,262 (7.0) | 4,108 (7.0) | 6,370 (7.0) | 1,043 (6.6) | 1,959 (6.8) | 3,002 (6.7) | |
Poor | 10,126 (31.4) | 21,302 (36.2) | 31,428 (34.5) | 4,932 (31.4) | 10,621 (36.6) | 15,553 (34.8) | |
No. of missing values | 40 (0.1) | 89 (0.2) | 129 (0.1) | 14 (0.1) | 36 (0.1) | 50 (0.1) |
Values are numbers (percentages) of participants unless stated otherwise. NA, not available; SD, standard deviation.
Missing data
Potential risk predictors with more than 50% missing values were excluded. Multiple imputation was used to impute risk predictors with ≤50% missing values assuming any missing values of the risk predictors were missing at random (49,50). We conducted ten imputations because this has relatively high efficiency, accounting for the large datasets and computing power (50). Rubin’s rules were used to combine coefficients accounting for missing data (50). Imputed values were used for derivation and testing of all the risk prediction models.
Validation and recalibration of the Kotz COPD risk prediction model (17)
We first assessed the performance of the original and recalibrated Kotz risk prediction models (17) using similar risk predictors. We recalibrated both models to survival in the EHS cohort because life expectancy is longer in Hong Kong than in the UK (51). We also replaced a composite measure of deprivation, the Carstairs index (52), with five levels based on seven measures (i.e., income deprivation, employment deprivation, education, skills and training deprivation, health deprivation, disability, crime, barriers to housing and services and living environment deprivation) with an index of deprivation based on the type of housing, education level, and public assistance (i.e., Comprehensive Social Security Assistance) as these are more relevant and contextually specific indices in Hong Kong, especially for older adults (53). Furthermore, due to the relatively low prevalence of asthma (~0.13%, n=175) at study entry, we excluded this risk predictor from the recalibrated Kotz model.
Statistical analysis for development of a new COPD risk prediction model
We secondarily investigated whether a new model to predict COPD risk within the next five years in older Chinese adults (65+ years) performed better than did the Kotz model. We used a sex-specific extended Cox proportional hazards regression to estimate the coefficients for each potential risk predictor in the derivation cohort. Risk predictors were selected using backward elimination as recommended for constructing clinical prediction models (54). The proportional hazards assumption was tested for each potential risk predictor, with additional adjustment for other risk predictors, using Schoenfeld residuals before model development (55). Non-linear relations for continuous risk predictors were detected, and the appropriate relation via fractional polynomial terms was identified based on Martingale residuals (56). Significant interactions between age and the risk predictors were included in the final models. The risk of COPD was calculated using the following equation:
where S0(t) is the baseline survival at time t, which was estimated using the observed survival in the derivation cohort; Xi and Xj(t) are respectively time-invariant and time-varying risk predictors. We considered three periods (i.e., 0–4.9, 5.0–9.9, 10+ years) for possible time-varying effects of age and smoking.
Model validation
We assessed the predictive performance of the original Kotz model in the whole EHS cohort and of the recalibrated Kotz model and the new model (EHS-COPD) in the validation cohort. Measures assessing predictive performance [i.e., integrated Brier score (IBS) and explained variance (R2)], discrimination (i.e., D statistic and Harrel’s C statistic), and calibration [i.e., calibration-in-the-large (or calibration intercept) and calibration slope] were computed (57). Of these, IBS was calculated by integrating the Brier score for all the entire follow-up periods, which quantifies the mean squared error of the difference between the predicted and the observed survival probability. The observed survival probability derived from the Kaplan-Meier estimator was considered as the benchmark value (), in which an IBS of zero indicates a perfect model (58). Based on the IBS, we further computed the explained variance using . We calculated the D statistic (which quantifies the prognostic separation between the COPD and non-COPD cases) and Harrell’s C statistic (which quantifies the probability of correct ordering in terms of shorter time to event for the participant with higher predicted risk for a randomly selected pair of participants) to evaluate the discriminative ability, in which a higher value indicates better model discrimination. Calibration was illustrated by comparing the mean 5-year predicted risk with the observed risk. The calibration slope was estimated to evaluate the agreement between predicted and observed risk. A value of 1 for the calibration slope and 0 for the calibration in the large parameter suggest perfect calibration, whereas a value for the calibration slope diverging from 1 indicates a poorer agreement. The performance measures were estimated based on each imputed validation cohort and combined using inverse variance weighting (59).
Risk stratification
Since there is no clear threshold for classifying the risk of developing COPD; we calculated sensitivity, specificity, and positive and negative predicted values of the EHS-COPD model at the top 5%, 10%, and 50% estimated risk of COPD over 5 years to stratify the participants into four groups: high, moderate, mild, and low, as in a previous similar study (60).
All statistical analyses were conducted using R software (version 3.6.3, https://cran.r-project.org/) (61).
Ethical review
The EHS Cohort was conducted with the Institutional Review Board of the University of Hong Kong/Hospital Authority Hong Kong West Cluster (HKU/HA HKW IRB) ethical approval. This study is an analysis of routinely collected data; informed (non-written) consent was obtained by the participants implicitly agreeing to their data being used for research by using the service. This study conformed to the Declaration of Helsinki (as revised in 2013).
Data availability
Currently, the data are not publicly available; we would welcome collaborations and research proposals.
Results
Overall study population
We identified a total of 136,309 participants aged 65 years or older in the EHS cohort. We excluded 487 (0.36%) participants who had COPD at baseline. We included 135,822 participants (47,912 men and 87,910 women) when assessing the performance of the original and recalibrated Kotz models and also to derive the sex-specific EHS-COPD models. Two-thirds of the participants (32,222 men and 58,911 women) were randomly allocated to the derivation cohort, with the remaining one-third (15,690 men and 28,999 women) allocated to the validation cohort, as shown in Figure 1.
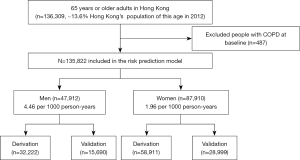
Baseline characteristics
Table 1 shows sex-specific baseline characteristics of the participants in both the derivation and validation cohorts. In the derivation cohort, the mean age was 72.2 years for men and 71.7 years for women. The distribution of type of housing, public assistance, physical activity, family history of cardiovascular diseases, existing hypertension, hospital admission, and recent falls were comparable between men and women. Women were more likely than men to be illiterate or obese, based on Asian BMI cut-offs (62) (BMI 25.0+ kg/m2), and to have poor physical functioning, poor cognitive functioning, depressive symptoms, or poor self-rated health. Furthermore, 59.8% of men and 9.4% of women were ex- or current smokers, while 32.8% of men and 9.9% of women were ever drinkers.
Incidence rates of COPD
Table 2 shows the number of incidence cases, person-years of follow-up, and incidence rates by sex, age, and observation period. Overall, 3,823 COPD cases were observed, with 2,610 COPD cases in 0.92 million person-years of follow-up in the derivation cohort and 1,213 COPD cases in 0.45 million person-years of follow-up in the validation cohort. The incidence rate of COPD was 2.27 times [95% confidence interval (CI): 2.13–2.42] higher in men than in women, and increased rapidly during the first 2 years of follow-up and decreased afterwards (Figure S1). The original Kotz model had about a 2 times higher crude incidence rate of COPD [5.53 per 1,000 person-years (95% CI: 5.46–5.60)] than that in the EHS cohort (2.78 per 1,000 person-years, 95% CI: 2.69–2.87). The median follow-up time for the derivation cohort was 10.0 years [interquartile range (IQR), 6.3–15.0 years], with 75,543 (82.9%) participants having 5+ years of follow-up and 44,198 (48.5%) participants having 10+ years of follow-up. In the validation cohort, the median follow-up time was 10.0 years (IQR, 6.4–15.0 years), with 37,096 (83.0%) participants having 5+ years of follow-up and 21,890 (49.0%) participants having 10+ years of follow-up.
Table 2
Characteristics | Derivation cohort | Validation cohort | |||||
---|---|---|---|---|---|---|---|
Incidence cases | Person years | Incidence rate per 1,000 person-years (95% CI) | Incidence cases | Person years | Incidence rate per 1,000 person-years (95% CI) | ||
Men | 1,352 | 302,230 | 4.47 (4.24–4.72) | 657 | 148,465 | 4.43 (4.09–4.78) | |
Women | 1,258 | 618,752 | 2.03 (1.92–2.15) | 556 | 305,396 | 1.82 (1.67–1.98) | |
Age (years) | |||||||
65–69 | 995 | 405,158 | 2.46 (2.31–2.61) | 462 | 200,740 | 2.30 (2.10–2.52) | |
70–74 | 874 | 295,446 | 2.96 (2.77–3.16) | 398 | 144,575 | 2.75 (2.49–3.04) | |
75–79 | 489 | 149,370 | 3.27 (2.99–3.58) | 224 | 72,337 | 3.10 (2.70–3.53) | |
80–84 | 180 | 53,417 | 3.37 (2.90–3.90) | 100 | 27,444 | 3.64 (2.96–4.43) | |
85+ | 72 | 17,591 | 4.09 (3.20–5.15) | 29 | 8,753 | 3.31 (2.22–4.76) | |
Time period (years) | |||||||
0.0–4.9 | 2,005 | 429,709 | 4.67 (4.46–4.87) | 912 | 210,796 | 4.33 (4.05–4.62) | |
5.0–9.9 | 453 | 673,190 | 0.67 (0.61–0.74) | 208 | 331,560 | 0.63 (0.54–0.72) | |
10.0+ | 152 | 637,778 | 0.24 (0.20–0.28) | 93 | 315,885 | 0.29 (0.24–0.36) | |
Overall | 2,610 | 920,982 | 2.83 (2.73–2.94) | 1,213 | 453,861 | 2.67 (2.52–2.83) |
CI, confidence interval.
Potential risk predictors
Table S1 shows the coefficients and adjusted hazard ratios for the recalibrated Kotz model for men and women in the derivation cohort. Table 3 shows the coefficients and adjusted hazard ratios for the EHS-COPD model for men and women in the derivation cohort. The EHS-COPD model included time-varying variables (i.e., age and smoking status) and time-invariant variables (i.e., education level, public assistance, alcohol use, BMI, physical activity, existing hypertension, recent falls in the past 6 months, cognitive function, and self-rated health status). The other potential risk predictors did not meet the inclusion criteria in the final model because of extremely low or high prevalence.
Table 3
Characteristics | Men | Women | |||
---|---|---|---|---|---|
Coefficients | Adjusted HR (95% CI)* | Coefficients | Adjusted HR (95% CI)* | ||
Age | |||||
Time period: 0.0–4.9 years | 0.0112 | 1.01 (1.01–1.02) | 0.0081 | 1.01 (1.00–1.01) | |
Time period: 5.0–9.9 years | −0.0077 | 0.99 (0.98–1.00) | −0.0113 | 0.99 (0.98–1.00) | |
Time period: 10.0+ years | −0.0319 | 0.97 (0.95–0.98) | −0.0191 | 0.98 (0.96–1.00) | |
Smoking status | |||||
Time period: 0.0–4.9 years | |||||
Never smoker | Reference | Reference | |||
Ex-smoker | 0.4868 | 1.63 (1.55–1.71) | 0.7780 | 2.18 (2.05–2.31) | |
Current smoker | 1.0053 | 2.73 (2.59–2.89) | 1.0978 | 3.00 (2.79–3.22) | |
Time period: 5.0–9.9 years | |||||
Never smoker | Reference | Reference | |||
Ex-smoker | 0.5285 | 1.70 (1.54–1.87) | 1.0375 | 2.82 (2.48–3.21) | |
Current smoker | 1.072 | 2.92 (2.63–3.24) | 1.6642 | 5.28 (4.61–6.04) | |
Time period: 10.0+ years | |||||
Never smoker | Reference | Reference | |||
Ex-smoker | 0.5000 | 1.65 (1.41–1.93) | 1.0660 | 2.90 (2.28–3.71) | |
Current smoker | 1.1787 | 3.25 (2.76–3.83) | 1.4618 | 4.31 (3.28–5.67) | |
Public assistance (yes) | 0.2302 | 1.26 (1.20–1.32) | 0.2236 | 1.25 (1.19–1.31) | |
Alcohol use | |||||
Never drinker | Reference | ||||
Ex-drinker | 0.3786 | 1.46 (1.39–1.53) | 0.0176 | 1.02 (0.94–1.11) | |
Social drinker | 0.3271 | 1.39 (1.33–1.45) | 0.1105 | 1.12 (1.05–1.19) | |
Moderate drinker | 0.2116 | 1.24 (1.16–1.32) | −0.2036 | 0.82 (0.67–0.99) | |
Excessive drinker | −0.0786 | 0.92 (0.84–1.01) | −1.7951 | 0.17 (0.09–0.31) | |
Body mass index (kg/m2) | |||||
<18.5 | 0.4515 | 1.57 (1.47–1.68) | 0.4666 | 1.59 (1.49–1.71) | |
18.5–<23.0 | Reference | Reference | |||
23.0–<25.0 | −0.0541 | 0.95 (0.91–0.99) | −0.1573 | 0.85 (0.81–0.90) | |
25.0+ | −0.0962 | 0.91 (0.87–0.95) | 0.0110 | 1.01 (0.97–1.05) | |
Physical activity (hours per week) | |||||
None | Reference | Reference | |||
0.1–<1.5 | −0.2346 | 0.79 (0.74–0.85) | −0.2693 | 0.76 (0.71–0.83) | |
1.5–<3.0 | −0.0561 | 0.95 (0.90–1.00) | 0.0793 | 1.08 (1.02–1.15) | |
3.0–<4.5 | −0.8430 | 0.43 (0.40–0.47) | −0.9286 | 0.40 (0.36–0.43) | |
4.5+ | −0.2890 | 0.75 (0.71–0.78) | −0.0592 | 0.94 (0.89–0.99) | |
Hypertension (yes) | 0.5051 | 1.66 (1.52–1.81) | 0.8540 | 2.35 (2.17–2.54) | |
Recent falls (yes) | −0.9162 | 0.40 (0.30–0.53) | 0.2171 | 1.24 (1.10–1.40) | |
Cognitive function (poor) | −0.3933 | 0.67 (0.59–0.77) | −0.2269 | 0.80 (0.74–0.86) | |
Self-rated health | |||||
Better | 0.1113 | 1.12 (1.04–1.20) | 0.0315 | 1.03 (0.96–1.11) | |
Normal | Reference | Reference | |||
Poor | 0.1925 | 1.21 (1.17–1.26) | 0.1741 | 1.19 (1.15–1.23) |
*, well-established predictors in EHS-COPD are selected with P value <0.05. EHS-COPD, chronic obstructive pulmonary disease (COPD) risk prediction model based on Hong Kong’s Elderly Health Services (EHS) Cohort; CI, confidence interval.
Figures S2,S3 show the smoothed hazard ratios for the time-varying effects of age and smoking status including and excluding education level, public assistance, alcohol use, BMI, physical activity, existing hypertension, recent falls, cognitive function, and self-rated health status in men and women, respectively. For each of these potential risk predictors, the hazard changed over time from study entry.
Model validation
Table 4 shows the performance of the original Kotz model, the recalibrated Kotz model, and the EHS-COPD model in predicting the 5-year risk of COPD in men and women. Overall the values of D and C statistics were greater in women than in men except for the C statistic for the recalibrated Kotz model, suggesting that both the original and the recalibrated Kotz model performed relatively better in women than in men. However, the performance of the EHS-COPD model for predicting 5-year risk of COPD was better than those of the original and the recalibrated Kotz models in both sexes, as indicated by the smaller IBS, larger R2, higher D statistic, and the higher Harrell’s C statistic.
Table 4
Statistics | Men | Women |
---|---|---|
Original Kotz model* | ||
Integrated Brier Score | ||
Benchmark model | 0.0367 | 0.0177 |
Original Kotz model | 0.0364 | 0.0176 |
R2 (%) | 0.82 | 0.56 |
D statistic | 1.614 (1.591 to 1.636) | 2.220 (2.193 to 2.248) |
Harrell’s C | 0.603 (0.598 to 0.607) | 0.684 (0.677 to 0.692) |
Calibration slope | 0.391 (0.287 to 0.495) | N.A. |
Calibration-in-the-large | 0.003 (−0.004 to 0.011) | N.A. |
Recalibrated Kotz model** | ||
Integrated Brier Score | ||
Benchmark model | 0.0370 | 0.0164 |
Recalibrated Kotz model | 0.0364 | 0.0163 |
R2 (%) | 1.62 | 0.61 |
D statistic | 2.458 (2.420 to 2.497) | 2.472 (2.429 to 2.515) |
Harrell’s C | 0.666 (0.659 to 0.673) | 0.657 (0.649 to 0.665) |
Calibration slope | 0.737 (0.692 to 0.782) | 1.437 (1.312 to 1.562) |
Calibration-in-the-large | −0.005 (−0.007 to −0.002) | −0.011 (−0.013 to −0.008) |
EHS-COPD*** | ||
Integrated Brier Score | ||
Benchmark model | 0.0370 | 0.0164 |
EHS-COPD | 0.0298 | 0.0150 |
R2 (%) | 19.46 | 8.54 |
D statistic | 23.147 (23.097 to 23.187) | 21.420 (21.374 to 21.465) |
Harrell’s C | 0.930 (0.928 to 0.932) | 0.928 (0.925 to 0.930) |
Calibration slope | 2.126 (1.952 to 2.301) | 3.928 (3.663 to 4.193) |
Calibration-in-the-large | −0.018 (−0.024 to −0.012) | −0.018 (−0.021 to −0.015) |
*, the baseline survival at 5 years was 0.9700 for men and 0.9842 for women in the original Kotz model; **, the baseline survival at 5 years was 0.9703 for men and 0.9835 for women in the recalibrated Kotz model; ***, the baseline survival at 5 years was 0.9720 for men and 0.9845 for women in the EHS-COPD model. N.A., not available. The performance of the original Kotz model was assessed using the whole EHS cohort, whilst those of the recalibrated Kotz and EHS-COPD models were assessed using the validation cohort. Due to the limited predicted risk using the original Kotz model, only 2 risks were calculated, inducing 2 subgroups so that the calibration slope and the calibration-in-the-large could not calculated. EHS-COPD, chronic obstructive pulmonary disease (COPD) risk prediction model based on Hong Kong’s Elderly Health Services (EHS) Cohort; CI, confidence interval.
Compared with the Kaplan-Meier estimator (), the predictive error of IBS for the recalibrated Kotz model was 0.0364 for men. It explained 1.62% of the variation in COPD risk in the validation cohort. The D statistic was 2.458 and Harrell’s C statistic was 0.666. The corresponding values in women were 0.0163, 0.61%, 2.472, and 0.657. The recalibrated Kotz model performed better than the original Kotz model for men, but performed worse for women. In contrast, the newly developed EHS-COPD model outperformed the original and recalibrated Kotz models in both sexes. Similar results were also observed for calibration slope and calibration in the large (Table 4).
Furthermore, based on the EHS-COPD risk prediction model, the 5-year predicted risk of COPD was 0.114 for men and 0.042 for women, similar to the observed 5-year risk (i.e., 0.152 for men and 0.073 for women). Figure S4 depicts the observed risk and mean predicted risk of developing COPD at 5 years by 20th predicted risk in the validation cohort for men and women, respectively. The EHS-COPD model tended to underestimate the 5-year risk of developing COPD. Overall, the EHS-COPD model had better performance in men than in women.
Risk stratification
Table 5 shows sensitivity, specificity, positive and negative predictive values for the 5-year risk of COPD in the validation cohort. Table S2 shows the characteristics of participants in the validation cohort classified into the following four groups according to EHS-COPD model:
Table 5
Threshold | Risk threshold (%) | True-positive count | False-positive count | False-negative count | True-negative count | Sensitivity (%) | Specificity (%) | Positive predictive value (%) | Negative predictive value (%) |
---|---|---|---|---|---|---|---|---|---|
Top 1% | 0.1434 | 112 | 336 | 800 | 43,440 | 12.31 | 99.23 | 25.07 | 98.19 |
Top 2% | 0.1057 | 213 | 676 | 699 | 43,100 | 23.34 | 98.46 | 23.93 | 98.40 |
Top 3% | 0.0853 | 308 | 1,006 | 604 | 42,770 | 33.81 | 97.70 | 23.45 | 98.61 |
Top 4% | 0.0722 | 391 | 1,334 | 521 | 42,442 | 42.83 | 96.95 | 22.65 | 98.79 |
Top 5% | 0.0626 | 446 | 1,662 | 466 | 42,114 | 48.88 | 96.21 | 21.15 | 98.91 |
Top 6% | 0.0544 | 497 | 1,987 | 415 | 41,789 | 54.49 | 95.46 | 20.02 | 99.02 |
Top 7% | 0.0473 | 541 | 2,264 | 371 | 41,512 | 59.38 | 94.83 | 19.30 | 99.11 |
Top 8% | 0.0418 | 564 | 2,532 | 348 | 41,244 | 61.83 | 94.22 | 18.21 | 99.16 |
Top 9% | 0.0380 | 590 | 2,793 | 322 | 40,983 | 64.75 | 93.62 | 17.46 | 99.22 |
Top 10% | 0.0348 | 616 | 3,058 | 296 | 40,718 | 67.56 | 93.02 | 16.79 | 99.28 |
Top 15% | 0.0254 | 782 | 4,341 | 130 | 39,435 | 85.83 | 90.08 | 15.28 | 99.67 |
Top 20% | 0.0199 | 871 | 5,437 | 41 | 38,339 | 95.54 | 87.58 | 13.81 | 99.89 |
Top 30% | 0.0112 | 907 | 6,161 | 5 | 37,615 | 99.45 | 85.93 | 12.83 | 99.99 |
Top 40% | 0.0074 | 912 | 6,646 | 0 | 37,130 | 100 | 84.82 | 12.07 | 100 |
Top 50% | 0.0057 | 912 | 6,671 | 0 | 37,105 | 100 | 84.76 | 12.03 | 100 |
- The high-risk group included 2,237 participants (i.e., 5.0% of 44,688), in the top 5% for risk of COPD in the next 5 years;
- The moderate-risk group included 2236 participants (i.e., 5.0% of 44,688), in the next 10% for risk of COPD in the next 5 years;
- The mild-risk group included 18,028 participants (i.e., 40.3% of 44,688), in next 50% for risk of COPD in the next 5 years;
- The low-risk group included the remaining 22,187 participants (i.e., 49.6% of 44,688).
In the high-risk group, the COPD incidence rate was 65.0 per 1,000 person-years, and the mean age was 74.6 years. In addition, 88.1% were men, 92.2% were either ex- or current smokers, 27.1% had public assistance, 14.2% were illiterate, 40.9% were ever drinkers, 15.7% had low BMI, 21.6% had no physical activity per week, 2.1% had existing hypertension, 0.8% had had 2 or more falls in the last 6 months, 4.0% had poor cognitive function, and 43.1% had poor self-rated health in comparison with the previous year.
Clinical examples
Table S3 shows four hypothetical participants as examples to illustrate how to use the EHS-COPD model.
Discussion
Principal findings
We assessed the performance of the original and the recalibrated Kotz models for predicting the risk of developing COPD within the next 5 years in older Chinese adults (65+ years) in Hong Kong. Neither model predicted the risk of developing COPD well. A newly developed EHS-COPD risk prediction model had a better performance in an independent validation cohort. It explained 19.5% and 8.5% of COPD risk for men and women, respectively. Moreover, the EHS-COPD model yielded an excellent overall performance, which outperformed the original and recalibrated Kotz models, especially for men.
Comparison with other studies
Incidence rate
The crude incidence rate of COPD, i.e., 2.78 per 1,000 person-years (95% CI: 2.69–2.87), in the EHS cohort (Figure S1) was lower than that in some previous studies (17,33) but comparable to that in others (63).
Risk predictors
The original Kotz model is largely driven by age (17), but in the EHS cohort, age did not predict COPD in men or women (Table S1). Ex- or current smoking did not predict COPD as strongly in the EHS cohort as in the original Kotz model, possibly due to the small number of cigarettes smoked per day in Hong Kong and the existence of other context-specific causes of COPD (Table 1), such as incense burning (64,65). As previously, low BMI, low socioeconomic position, and ever drinking all predicted COPD (8,32,37-44,66,67). Several markers of ill-health, such as poor cognitive function, recent falls, and self-rated health status, also predicted COPD. However, asthma did not predict COPD, possibly because of the relatively low prevalence of asthma among older adults in this setting (68).
Other risk prediction models
A recent review (27) identified 4 risk prediction models for COPD. However, one of them was designed for use in out-patients, and 3 of them were developed in Western settings.
Strengths and limitations
The COPD risk prediction model developed here has the advantages of incorporating time-varying risks of age and smoking status, a large sample with long follow-up, a population-based cohort, and minimal recall and response bias. Nevertheless, limitations exist. First, self-reported COPD cases were not validated against medical records or spirometry, but measurement errors should be random. Second, participants in the EHS cohort were volunteers, so they may not represent the entire population, limiting its applicability to those who are home-bound. Third, for feasibility, the model only included easy-to-assess risk predictors, but did not include other potentially significant COPD risk predictors that are unlikely to be available in a clinical setting, such as indoor/outdoor air pollution (31,69), occupational exposures (e.g., dust, chemical agents, and fumes) (70), genetic predisposition (71,72), measures of lung function (72), asthma and airway hyper-reactivity (73,74), and history of severe childhood respiratory infections (75,76). The model explained less variation for women (i.e., ~8.5%) than it did for men, possibly because women had low smoking prevalence. Lastly, recalibration of the EHS-COPD model might be needed for use in all Chinese adults.
Conclusions
An existing COPD prediction model, the Kotz model, had low discrimination and calibration in older Chinese adults (65+ years) in Hong Kong. However, a revised model, EHS-COPD, had excellent performance compared to the original and recalibrated Kotz models.
Risk predictors in the relevant literature |
Age (continuous variable) (8,17-19,27-35) |
Smoking status (never, ex-, or current smoker) (8,17-20,27-32,34-37) |
BMI (<18.5 kg/m2, 18.5–<23.0 kg/m2, 23.0–<25.0 kg/m2, 25.0+ kg/m2) (20,27,34,37-40) |
Existing asthma at study entry (17,28) |
Physical activity (none, 0.1–<1.5 h/week, 1.5–<3.0 h/week, 3.0–<4.5 h/week, 4.5+ h/week) (37,41-44) |
Recent falls (2 or more falls in the past 6 months) (45,46) |
Cognitive function (poor functioning defined as mini-mental state examination score ≤18 [illiterate]/20 [1–2 years schooling]/22 [2+ years schooling] or abbreviated mental test score ≤7 or simplified memory test without correctly answering all three questions) (47) |
Existing hypertension at study entry (48) |
Alcohol use (never, ex-, social, moderate, or excessive drinker) (37) |
Potential risk predictors available in the EHS cohort |
Education level (illiterate, literate but no formal education, primary, secondary or above) |
Family history of cardiovascular disease in either first- or second-degree relatives |
Hospital admission in the past 12 months |
Physical functioning [poor functioning defined as ADL >8, or IADL ≥6] |
Depressive symptoms (geriatric depression scale ≥8) |
Type of housing (public or aided housing, private rented housing, private self-owned housing, others) |
Public assistance (in receipt of Comprehensive Social Security Assistance) |
Self-rated health (health condition this year compared with last year) |
COPD, chronic obstructive pulmonary disease; BMI, body mass index; EHS, Elderly Health Services; ADL, activities of daily living score; IADL, instrumental activities of daily living score.
Acknowledgments
The authors thank the participants of Hong Kong’s Elderly Health Service Cohort, and the Hong Kong Department of Health for their collaboration.
Funding: None.
Footnote
Reporting Checklist: The authors have completed the transparent reporting of a multivariable model for individual prognosis or diagnosis (TRIPOD) guidance reporting checklist. Available at https://dx.doi.org/10.21037/atm-21-3270
Data Sharing Statement: Available at https://dx.doi.org/10.21037/atm-21-3270
Conflicts of Interest: All authors have completed the ICMJE uniform disclosure form (available at https://dx.doi.org/10.21037/atm-21-3270). The authors have no conflicts of interest to declare.
Ethical Statement: The authors are accountable for all aspects of the work in ensuring that questions related to the accuracy or integrity of any part of the work appropriately investigated and resolved. The EHS Cohort was conducted with the Institutional Review Board of the University of Hong Kong/Hospital Authority Hong Kong West Cluster (HKU/HA HKW IRB) ethical approval. This study is an analysis of routinely collected data; informed (non-written) consent was obtained by the participants implicitly agreeing to their data being used for research by using the service. This study also conformed to the provisions of the Declaration of Helsinki (as revised in 2013).
Open Access Statement: This is an Open Access article distributed in accordance with the Creative Commons Attribution-NonCommercial-NoDerivs 4.0 International License (CC BY-NC-ND 4.0), which permits the non-commercial replication and distribution of the article with the strict proviso that no changes or edits are made and the original work is properly cited (including links to both the formal publication through the relevant DOI and the license). See: https://creativecommons.org/licenses/by-nc-nd/4.0/.
References
- GBD Chronic Respiratory Disease Collaborators. Prevalence and attributable health burden of chronic respiratory diseases, 1990-2017: a systematic analysis for the Global Burden of Disease Study 2017. Lancet Respir Med 2020;8:585-96. [Crossref] [PubMed]
- Fang L, Gao P, Bao H, et al. Chronic obstructive pulmonary disease in China: a nationwide prevalence study. Lancet Respir Med 2018;6:421-30. [Crossref] [PubMed]
- Srivastava K, Thakur D, Sharma S, et al. Systematic review of humanistic and economic burden of symptomatic chronic obstructive pulmonary disease. Pharmacoeconomics 2015;33:467-88. [Crossref] [PubMed]
- Wang C, Xu J, Yang L, et al. Prevalence and risk factors of chronic obstructive pulmonary disease in China (the China Pulmonary Health [CPH] study): a national cross-sectional study. Lancet 2018;391:1706-17. [Crossref] [PubMed]
- Mortality Statistics 2017 (Provisional). Hong Kong SAR: Department of Health and Census and Statistics Department.
- WHO. Chronic respiratory diseases. Available online: https://www.who.int/health-topics/chronic-respiratory-diseases#tab=tab_1 (accessed July 28, 2020).
- Mathers CD, Loncar D. Projections of global mortality and burden of disease from 2002 to 2030. PLoS Med 2006;3:e442. [Crossref] [PubMed]
- Beran D, Zar HJ, Perrin C, et al. Burden of asthma and chronic obstructive pulmonary disease and access to essential medicines in low-income and middle-income countries. Lancet Respir Med 2015;3:159-70. [Crossref] [PubMed]
- Diab N, Gershon AS, Sin DD, et al. Underdiagnosis and Overdiagnosis of Chronic Obstructive Pulmonary Disease. Am J Respir Crit Care Med 2018;198:1130-9. [Crossref] [PubMed]
- Guirguis-Blake JM, Senger CA, Webber EM, et al. Screening for Chronic Obstructive Pulmonary Disease: Evidence Report and Systematic Review for the US Preventive Services Task Force. JAMA 2016;315:1378-93. [Crossref] [PubMed]
- Tashkin D, Kanner R, Bailey W, et al. Smoking cessation in patients with chronic obstructive pulmonary disease: a double-blind, placebo-controlled, randomised trial. Lancet 2001;357:1571-5. [Crossref] [PubMed]
- Tønnesen P, Mikkelsen K, Bremann L. Nurse-conducted smoking cessation in patients with COPD using nicotine sublingual tablets and behavioral support. Chest 2006;130:334-42. [Crossref] [PubMed]
- Hilberink SR, Jacobs JE, Bottema BJ, et al. Smoking cessation in patients with COPD in daily general practice (SMOCC): six months' results. Prev Med 2005;41:822-7. [Crossref] [PubMed]
- Decramer M, Celli B, Kesten S, et al. Effect of tiotropium on outcomes in patients with moderate chronic obstructive pulmonary disease (UPLIFT): a prespecified subgroup analysis of a randomised controlled trial. Lancet 2009;374:1171-8. [Crossref] [PubMed]
- Tashkin DP, Celli BR, Decramer M, et al. Efficacy of tiotropium in COPD patients with FEV1 ≥ 60% participating in the UPLIFT® trial. COPD 2012;9:289-96. [Crossref] [PubMed]
- Zhou Y, Zhong NS, Li X, et al. Tiotropium in Early-Stage Chronic Obstructive Pulmonary Disease. N Engl J Med 2017;377:923-35. [Crossref] [PubMed]
- Kotz D, Simpson CR, Viechtbauer W, et al. Development and validation of a model to predict the 10-year risk of general practitioner-recorded COPD. NPJ Prim Care Respir Med 2014;24:14011. [Crossref] [PubMed]
- Higgins MW, Keller JB, Becker M, et al. An index of risk for obstructive airways disease. Am Rev Respir Dis 1982;125:144-51. [PubMed]
- Himes BE, Dai Y, Kohane IS, et al. Prediction of chronic obstructive pulmonary disease (COPD) in asthma patients using electronic medical records. J Am Med Inform Assoc 2009;16:371-9. [Crossref] [PubMed]
- Guo YI, Qian Y, Gong YI, et al. A predictive model for the development of chronic obstructive pulmonary disease. Biomed Rep 2015;3:853-63. [Crossref] [PubMed]
- Tian J, Zhou Y, Cui J, et al. Peak expiratory flow as a screening tool to detect airflow obstruction in a primary health care setting. Int J Tuberc Lung Dis 2012;16:674-80. [Crossref] [PubMed]
- Leung JY, Lin SL, Lee RS, et al. Framingham risk score for predicting cardiovascular disease in older adults in Hong Kong. Hong Kong Med J 2018;24:8-11. [PubMed]
- Xu L, Jiang CQ, Schooling CM, et al. Prediction of 4-year incident diabetes in older Chinese: recalibration of the Framingham diabetes score on Guangzhou Biobank Cohort Study. Prev Med 2014;69:63-8. [Crossref] [PubMed]
- Yang X, Li J, Hu D, et al. Predicting the 10-Year Risks of Atherosclerotic Cardiovascular Disease in Chinese Population: The China-PAR Project (Prediction for ASCVD Risk in China). Circulation 2016;134:1430-40. [Crossref] [PubMed]
- Collins GS, Reitsma JB, Altman DG, et al. Transparent reporting of a multivariable prediction model for individual prognosis or diagnosis (TRIPOD): the TRIPOD statement. BMJ 2015;350:g7594. [Crossref] [PubMed]
- Schooling CM, Chan WM, Leung SL, et al. Cohort Profile: Hong Kong Department of Health Elderly Health Service Cohort. Int J Epidemiol 2016;45:64-72. [Crossref] [PubMed]
- Matheson MC, Bowatte G, Perret JL, et al. Prediction models for the development of COPD: a systematic review. Int J Chron Obstruct Pulmon Dis 2018;13:1927-35. [Crossref] [PubMed]
- Mannino DM, Buist AS. Global burden of COPD: risk factors, prevalence, and future trends. Lancet 2007;370:765-73. [Crossref] [PubMed]
- López-Campos JL, Tan W, Soriano JB. Global burden of COPD. Respirology 2016;21:14-23. [Crossref] [PubMed]
- Ehteshami-Afshar S, FitzGerald JM, Doyle-Waters MM, et al. The global economic burden of asthma and chronic obstructive pulmonary disease. Int J Tuberc Lung Dis 2016;20:11-23. [Crossref] [PubMed]
- Eisner MD, Anthonisen N, Coultas D, et al. An official American Thoracic Society public policy statement: Novel risk factors and the global burden of chronic obstructive pulmonary disease. Am J Respir Crit Care Med 2010;182:693-718. [Crossref] [PubMed]
- Vestbo J, Hurd SS, Agustí AG, et al. Global strategy for the diagnosis, management, and prevention of chronic obstructive pulmonary disease: GOLD executive summary. Am J Respir Crit Care Med 2013;187:347-65. [Crossref] [PubMed]
- Gershon AS, Warner L, Cascagnette P, et al. Lifetime risk of developing chronic obstructive pulmonary disease: a longitudinal population study. Lancet 2011;378:991-6. [Crossref] [PubMed]
- Haroon S, Adab P, Riley RD, et al. Predicting risk of COPD in primary care: development and validation of a clinical risk score. BMJ Open Respir Res 2015;2:e000060. [Crossref] [PubMed]
- Su KC, Ko HK, Chou KT, et al. An accurate prediction model to identify undiagnosed at-risk patients with COPD: a cross-sectional case-finding study. NPJ Prim Care Respir Med 2019;29:22. [Crossref] [PubMed]
- Salvi S. Tobacco smoking and environmental risk factors for chronic obstructive pulmonary disease. Clin Chest Med 2014;35:17-27. [Crossref] [PubMed]
- Chen W, Sin DD, FitzGerald JM, et al. An Individualized Prediction Model for Long-term Lung Function Trajectory and Risk of COPD in the General Population. Chest 2020;157:547-57. [Crossref] [PubMed]
- Harik-Khan RI, Fleg JL, Wise RA. Body mass index and the risk of COPD. Chest 2002;121:370-6. [Crossref] [PubMed]
- Grigsby MR, Siddharthan T, Pollard SL, et al. Low Body Mass Index Is Associated with Higher Odds of COPD and Lower Lung Function in Low- and Middle-Income Countries. COPD 2019;16:58-65. [Crossref] [PubMed]
- Park HJ, Cho JH, Kim HJ, et al. The effect of low body mass index on the development of chronic obstructive pulmonary disease and mortality. J Intern Med 2019;286:573-82. [Crossref] [PubMed]
- Troosters T, van der Molen T, Polkey M, et al. Improving physical activity in COPD: towards a new paradigm. Respir Res 2013;14:115. [Crossref] [PubMed]
- Gimeno-Santos E, Frei A, Steurer-Stey C, et al. Determinants and outcomes of physical activity in patients with COPD: a systematic review. Thorax 2014;69:731-9. [Crossref] [PubMed]
- Garcia Aymerich J. Physical activity and COPD development. Time to advocate. Thorax 2019;74:831-2. [Crossref] [PubMed]
- Hansen GM, Marott JL, Holtermann A, et al. Midlife cardiorespiratory fitness and the long-term risk of chronic obstructive pulmonary disease. Thorax 2019;74:843-8. [Crossref] [PubMed]
- Roig M, Eng JJ, MacIntyre DL, et al. Falls in people with chronic obstructive pulmonary disease: an observational cohort study. Respir Med 2011;105:461-9. [Crossref] [PubMed]
- Hakamy A, Bolton CE, Gibson JE, et al. Risk of fall in patients with COPD. Thorax 2018;73:1079-80. [Crossref] [PubMed]
- von Siemens SM, Perneczky R, Vogelmeier CF, et al. The association of cognitive functioning as measured by the DemTect with functional and clinical characteristics of COPD: results from the COSYCONET cohort. Respir Res 2019;20:257. [Crossref] [PubMed]
- Chaouat A, Naeije R, Weitzenblum E. Pulmonary hypertension in COPD. Eur Respir J 2008;32:1371-85. [Crossref] [PubMed]
- Schafer JL. Multiple imputation: a primer. Stat Methods Med Res 1999;8:3-15. [Crossref] [PubMed]
- Rubin DB. Multiple imputation for nonresponse in surveys. New Jersey: John Wiley & Sons; 2004.
- The World Bank. (2019) Life expectancy at birth, total (years) - Hong Kong SAR, China. Available online: https://data.worldbank.org/indicator/SP.DYN.LE00.IN?locations=HK (accessed 22 June, 2021).
- Carstairs V, Morris R. Deprivation and health in Scotland. Health Bull (Edinb) 1990;48:162-75. [PubMed]
- Services HS. Housing for the Elderly 2018 Available online: https://www.gov.hk/en/residents/housing/publichousing/elderlyhousing.htm (accessed 22 June, 2021)
- Chowdhury MZI, Turin TC. Variable selection strategies and its importance in clinical prediction modelling. Fam Med Community Health 2020;8:e000262. [Crossref] [PubMed]
- Grambsch PM, Therneau TM. Proportional hazards tests and diagnostics based on weighted residuals. Biometrika 1994;81:515-26. [Crossref]
- Royston P, Ambler G, Sauerbrei W. The use of fractional polynomials to model continuous risk variables in epidemiology. Int J Epidemiol 1999;28:964-74. [Crossref] [PubMed]
- Steyerberg EW, Vickers AJ, Cook NR, et al. Assessing the performance of prediction models: a framework for traditional and novel measures. Epidemiology 2010;21:128-38. [Crossref] [PubMed]
- Gerds TA, Cai T, Schumacher M. The performance of risk prediction models. Biom J 2008;50:457-79. [Crossref] [PubMed]
- Riley RD, Ensor J, Snell KI, et al. External validation of clinical prediction models using big datasets from e-health records or IPD meta-analysis: opportunities and challenges. BMJ 2016;353:i3140. [Crossref] [PubMed]
- Hippisley-Cox J, Coupland C. Development and validation of QMortality risk prediction algorithm to estimate short term risk of death and assess frailty: cohort study. BMJ 2017;358:j4208. [Crossref] [PubMed]
- R Core Team. R: A Language and Environment for Statistical Computing. Vienna, Austria: R Foundation for Statistical Computing; 2019.
- Expert Consultation WHO. Appropriate body-mass index for Asian populations and its implications for policy and intervention strategies. Lancet 2004;363:157-63. [Crossref] [PubMed]
- Afonso AS, Verhamme KM, Sturkenboom MC, et al. COPD in the general population: prevalence, incidence and survival. Respir Med 2011;105:1872-84. [Crossref] [PubMed]
- Zhang Z, Tan L, Huss A, et al. Household incense burning and children's respiratory health: A cohort study in Hong Kong. Pediatr Pulmonol 2019;54:399-404. [Crossref] [PubMed]
- Wong A, Lou W, Ho KF, et al. Indoor incense burning impacts cognitive functions and brain functional connectivity in community older adults. Sci Rep 2020;10:7090. [Crossref] [PubMed]
- Celli BR, Cote CG, Marin JM, et al. The body-mass index, airflow obstruction, dyspnea, and exercise capacity index in chronic obstructive pulmonary disease. N Engl J Med 2004;350:1005-12. [Crossref] [PubMed]
- Garcia-Aymerich J, Gómez FP, Benet M, et al. Identification and prospective validation of clinically relevant chronic obstructive pulmonary disease (COPD) subtypes. Thorax 2011;66:430-7. [Crossref] [PubMed]
- Chan-Yeung M, Lai CK, Chan KS, et al. The burden of lung disease in Hong Kong: a report from the Hong Kong Thoracic Society. Respirology 2008;13:S133-65. [Crossref] [PubMed]
- Salvi SS, Barnes PJ. Chronic obstructive pulmonary disease in non-smokers. Lancet 2009;374:733-43. [Crossref] [PubMed]
- Paulin LM, Diette GB, Blanc PD, et al. Occupational exposures are associated with worse morbidity in patients with chronic obstructive pulmonary disease. Am J Respir Crit Care Med 2015;191:557-65. [Crossref] [PubMed]
- Stoller JK, Aboussouan LS. Alpha1-antitrypsin deficiency. Lancet 2005;365:2225-36. [Crossref] [PubMed]
- Hunninghake GM, Cho MH, Tesfaigzi Y, et al. MMP12, lung function, and COPD in high-risk populations. N Engl J Med 2009;361:2599-608. [Crossref] [PubMed]
- Silva GE, Sherrill DL, Guerra S, et al. Asthma as a risk factor for COPD in a longitudinal study. Chest 2004;126:59-65. [Crossref] [PubMed]
- McGeachie MJ. Childhood asthma is a risk factor for the development of chronic obstructive pulmonary disease. Curr Opin Allergy Clin Immunol 2017;17:104-9. [Crossref] [PubMed]
- Kim V, Han MK, Vance GB, et al. The chronic bronchitic phenotype of COPD: an analysis of the COPDGene Study. Chest 2011;140:626-33. [Crossref] [PubMed]
- de Marco R, Accordini S, Marcon A, et al. Risk factors for chronic obstructive pulmonary disease in a European cohort of young adults. Am J Respir Crit Care Med 2011;183:891-7. [Crossref] [PubMed]