Construction and analysis of the ceRNA network hsa_circ_0031968/miR-3611/GCG in lung adenocarcinoma
Introduction
Lung cancer (LC) has the highest incidence among all cancer types and is associated with a high fatality rate (1). Lung adenocarcinoma (LUAD) is the primary histopathological type (2). Clinically, surgery is the main form of treatment for LUAD patients, with radiotherapy, chemotherapy, immunotherapy, and targeted therapy as supplementary treatments. Although high-resolution computed tomography has improved the early diagnosis of LUAD (3), patient survival and prognosis has not been significantly enhanced. In addition, malignant tumor cells are prone to invasion and metastasis (4) and will continue to evolve during the treatment process, which can further lead to drug resistance and treatment failure (5). Therefore, it is essential to further our understanding of the competitive endogenous RNA (ceRNA) regulatory mechanism in LUAD.
Circular RNAs (circRNAs), microRNAs (miRNAs), and long non-coding RNAs (lncRNAs) are prominent members of non-coding RNAs (ncRNAs) and play vital roles in all aspects of human cancer through gene regulation (6). Our team’s previous research focused on the regulatory mechanism of the ceRNA regulatory network involved in lncRNA in LUAD, and we have confirmed that the Linc01833/miR-519e-3p/S100A4 axis can significantly promote the proliferation, invasion and metastasis of LUAD (7). There are not many studies on the mechanism of the ceRNA regulatory network involved in circRNA in LUAD. Therefore, to understand the role of the ceRNA regulatory network in LUAD as much as possible, our study will focus on exploring the relevant functions and mechanisms of circRNA. circRNA can be widely present in all organs and tissues of the human body, but is mainly located in the cytoplasm (8). circRNA is an entirely closed-loop structure with no 5'-3' polarity and no polyA tail. This structure means circRNAs are resistant to RNA exonuclease degradation, and are thus more stable than linear RNA (9). circRNAs contain a wealth of miRNA response elements (MREs), which can sponge a large amount of miRNA, thereby realizing the regulation of gene functions (10). In addition, it can also function through base pairing (11) or as a protein scaffold (12). circRNA is abnormally expressed in some types of tumors, such as bladder cancer (13), breast cancer (14), liver cancer (15), gastric cancer (16), and colon cancer (17). The ceRNA network, composed of circRNAs/miRNA/mRNA, has been demonstrated to exert a regulatory role in various tumors. For example, circRNA-LARP4 binds miR-424-5p through sponge adsorption to inhibit the proliferation of gastric cancer (18). circ-00001666 participates in the carcinogenesis of colorectal cancer by competing with the C-X-C motif chemokine receptor 5 (CXCR5) in combination with miR-1229 (19). The circRNA pleiotrophin/miR-122/SOX6 axis can promote the tumorigenesis of glioma cells (20). Thus, there appears to be an intricate interaction between the many circRNAs, miRNAs, and mRNA molecules in the human body. Although there has been much focus on circRNAs and their underlying mechanisms in LC (21,22), the role of circRNAs and the ceRNA network in LUAD still requires further investigation.
This study explored the regulatory role of the competitive interaction between circRNAs, miRNAs, and mRNAs in LUAD. This information may facilitate the identification of novel LUAD biomarkers and the development of new treatment strategies. The Gene Expression Omnibus (GEO) data was used to establish a ceRNA network and the circRNAs related to LUAD were selected for bioinformatics analysis. The Cancer Genome Atlas (TCGA) database for used for further verification. We finally successfully constructed a ceRNA regulatory network: hsa_circ_0031968/miR-3611/GCG, which can be used as new insights of LUAD biomarkers. The results of functional enrichment analysis can also provide new directions for understanding the occurrence and development of LUAD. In short, our research is based on mature bioinformatics technology, through rigorous statistical analysis combined with preliminary verification of clinical sample data, to provide reliable new clues for the early diagnosis and personalized treatment of LUAD patients. A flow chart detailing the research procedure is shown in Figure 1.
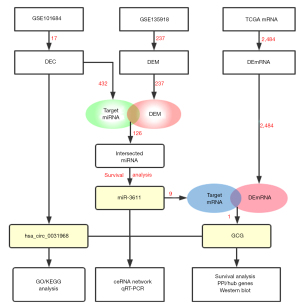
We present the following article in accordance with the MDAR reporting checklist (available at https://dx.doi.org/10.21037/atm-21-5854).
Methods
Data source
The LUAD-related data sets from the GEO database (https://www.ncbi.nlm.nih.gov/geo) were analyzed. The eligible circRNA data set (GSE101684) was obtained from the GPL21825 platform and included 4 LUAD samples and 4 normal adjacent tissue samples. The eligible miRNA data set (GSE135918) was obtained from the GPL18058 platform and included data from 5 LUAD tissues and 5 normal adjacent tissue samples. In addition, clinical information and gene expression profiles of LUAD patients were obtained from the TCGA database (https://portal.gdc.cancer.gov). Finally, 594 cases were examined, including 535 LUAD tissues and 59 adjacent normal tissues. Clinical data including age, gender, T stage, N stage, survival time, and survival status were collated.
A total of 30 LUAD patients who did not receive any treatment prior to their operation at the Thoracic Surgery Department of the First Affiliated Hospital of Zhengzhou University were enrolled. Samples of the postoperative tumor tissues and normal adjacent tissues were obtained and examined by quantitative real-time polymerase chain reaction (qRT-PCR) and Western blot analysis. Informed consent was obtained from all patients. The study conformed to the provisions of the Declaration of Helsinki (as revised in 2013). All content and processes strictly comply with relevant requirements and have been reviewed and approved by the Ethics Review Committee of Life Sciences, Zhengzhou University, China.
Identification of the differentially expressed genes and differentially expressed miRNAs (DEMs)
The DEMs and differentially expressed circRNAs (DECs) in the LUAD samples were screened using the GEO2R tool (http://www.ncbi.nlm.nih.gov/geo/geo2r). The “limma” and “edger” packages in the R language were used to identify the differentially expressed mRNAs (DEmRNAs). The |log2FC| threshold and P value were adjusted according to the amount of data. The miR-TarBase (http://mirtarbase.cuhk.edu.cn/php/index.php) database was used to identify potentially significant mRNAs. The R language “gplots” (https://bioconductor.org/packages/gplots/) package and “heatmap” (https://bioconductor.org/packages/heatmap/) package was used to generate volcano plots for all DECs, DEMs, and DEmRNAs.
Construction of the ceRNA network
The circBase database (http://www.circbase.org) was used to obtain the parental genes of the DECs and the locus in the genome. The cancer-specific circRNA database (CSCD) (http://gb.whu.edu.cn/CSCD/) provides information related to the basic structure of DECs, including the MREs, RNA binding proteins (RBPs), and open reading frames (ORFs). The CSCD was used to draw the circRNA circle diagrams, screen the target miRNA of the circRNAs, and determine the function of the circRNA of interest. The Venn analysis (https://bioinformatics.psb.ugent.be/webtools/Venn/) was used to analyze and detect the miRNAs that overlap with the DEM, and record them as miRNAs of interest. Cytoscape 3.7.2 was used to construct a visualized network diagram of circRNAs/miRNAs. Finally, the data in the TCGA database was examined to determine the role of the miRNAs of interest in LUAD. TargetScan (http://www.targetscan.org), miRTarBase (http://www.mirtarbase.org), and miRDB (http://www.mirdb.org) were used to identify candidate mRNA molecules. Candidate target DEmRNA molecules were identified from LUAD patients in the TCGA database and Venn analysis was used to determine the final target gene.
Gene Ontology (GO) and Kyoto Encyclopedia of Genes and Genomes (KEGG) enrichment analysis
To determine the effects of circRNAs in the pathophysiological process of LUAD and identify the critical signaling pathways involved, the circRNA target miRNAs were further analyzed for the potential gene set. The “clusterProfiler” (https://bioconductor.org/packages/clusterProfiler/) package in the R language was used to perform enrichment analysis on the gene set. GO functional enrichment analysis shows the biological functions of different genes and KEGG signaling pathway enrichment analysis assesses the degree of gene enrichment in different pathways. The DAVID V6.8 database (https://david.abcc.ncifcrf.gov/) was used to annotate genes and proteins while visualizing them. A P value <0.05 was considered statistically significant.
Clinical correlation analysis and construction of the protein-protein interaction (PPI) network
The expression profile of the final target gene and its correlation with survival prognosis and other clinical characteristics was examined in LUAD patients using the TCGA database. The Kaplan-Meier method was used for survival analysis and the STRING database (https://string-db.org/) was used to establish a PPI network of target genes. Cytoscape 3.7.2 was used for visualization and the CytoHubba was used to screen the hub genes that may interact with the final target mRNA.
qRT-PCR
qRT-PCR was performed on the postoperative specimens of 36 LUAD patients to verify the expression of the hsa_circ_0031968/miR-3611/GCG axis. Total RNA was extracted from tissue samples using Trizol reagent (Invitrogen, Carlsbad, CA, USA) according to the manufacturer’s protocols. The High Capacity cDNA Reverse Transcription Kit (Thermo Fisher Scientific) was used to synthesize complementary DNA (cDNA). The reverse transcription of miRNA was performed using the PrimeScript RT Reagent Kit (Takara Bio Inc., Shiga, Japan). The SYBR Green master mix was used to amplify the cDNA using the qRT-PCR system (Applied Biosystems, CA, USA). Glyceraldehyde 3-phosphate dehydrogenase (GAPDH) and RNU6 (U6) were used for standardization. The 2−ΔΔCT method was adopted to calculate the relative expression of RNA molecules. All samples were analyzed as triplicates. The detailed information of all primers is shown in Table 1.
Table 1
Gene | Forward | Reverse |
---|---|---|
Hsa_circ_0031968 | 5'-TCATTCATGCCTTCTGCAAC-3' | 5'-CCAGAGCAGATCACGACTGA-3' |
MiR-3611 | 5'-GTGAAGAAAGAAATTCTTAGCGGC-3' | 5'-CTCTACAGCTATATTGCCAGCCAC-3' |
GCG | 5'-CTGAAGGGACCTTTACCAGTGA-3' | 5'-CCTGGCGGCAAGATTATCAAG-3' |
GAPDH | 5'-GACAGTCAGCCGCATCTCTT-3' | 5'-ACCAAATCCGTTGACTCCGA-3' |
U6 | 5'-CTCGCTTCGGGCAGCACAT3' | 5'-AACCGCTTCACGAATTTGCGT-3' |
Western blot analysis
Total protein was extracted from LUAD cells with RIPA buffer (Roche, Branchburg, NJ, USA) containing protease inhibitors. The extracted protein was separated by 12% sodium dodecyl sulphate polyacrylamide gel electrophoresis (SDS-PAGE) and transferred to polyvinylidene fluoride membranes, and blocked with Tris-buffered saline containing 5% bovine serum albumin at room temperature for 1 hour. Membranes were then wash three times with phosphate-buffered saline (PBS) and incubated at 4 °C overnight with primary anti-rabbit antibody against GCG (1:1,000, CST, USA) and GAPDH (1:1,000, CST, USA). After washing with PBS, the membranes were incubated for 2 hours with a secondary peroxidase-conjugated goat anti-rabbit IgG at room temperature. The Odyssey machine and ECL reagents were used to visualize the protein bands. GAPDH was used as an internal reference to standardize the results.
Statistical analysis
Data were processed and analyzed using R language (version 4.1.1), SPSS (version 23.0), and GraphPad Prism (version 8.0). We are using the chi-square test to compare the clinical features of different groups. P<0.05 was considered statistically significant.
Results
Identification of the DECs, DEMs, and DEmRNAs
To confirm the potential function of circRNAs and construct the interaction network between circRNAs and miRNAs, the DECs were first identified. The GEO2R tool revealed that 17 DECs, including 2 upregulated and 15 downregulated DECs, were significantly correlated with the occurrence and progression of LUAD (Figure 2A,2B). Analysis of the miRNA data set GSE135918 from the GPL18058 platform demonstrated that 237 DEMs were closely related to LUAD (Figure 2C,2D). Examination of the LUAD-related mRNA data obtained from the TCGA database revealed a total of 2,484 DEmRNAs, including 1,982 upregulated mRNAs and 502 downregulated mRNAs (Figure 2E,2F).
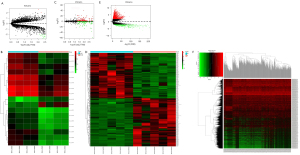
Identification of the circRNAs/miRNA interaction
The circBase database was used to identify the parent genes of these 17 DECs and their locus in the genome. The CSCD database contained details for 10 of the 17 circRNAs and their circle maps are shown in Figure 3A. Based on the concept of the ceRNA network, the CSCD and circRNA interactome (CRI) were used to successfully identify a total of 432 potential target miRNAs related these 10 circRNAs. Venn analysis determined that there were 126 miRNAs of interest, of which, 115 were upregulated and 11 were downregulated (Figure 3B-a). Furthermore, multiple pairs of circRNA/miRNA relationship were identified, including hsa_circ_0031968/miR-3611/miR-548, hsa_circ_0001955/miR-1236/miR-4713, and hsa_circ_0000317/miR-4458/miR-196 (Figure 3B-b).
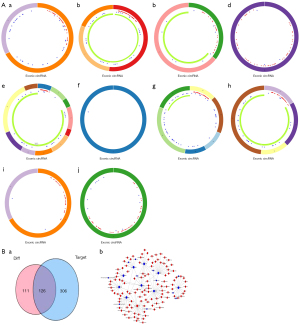
Selection of the final target miRNA
To further identify a more specific target miRNA for the ceRNA network, clinically relevant information of LUAD patients was obtained from the TCGA database and analyzed (Figure 4). It is noteworthy that miR-3611, which is regulated by hsa_circ_0031968, significantly affected the survival of LUAD patients. The survival rate of patients with low miR-3611 expression was considerably better than that of patients with high miR-3611 expression (P<0.05). Therefore, miR-3611 was considered the final target miRNA in this study and hsa_circ_0031968 was considered the final target circRNA.
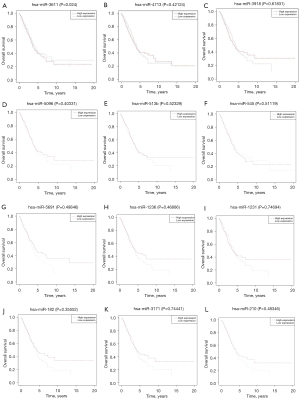
Enrichment analysis
The biological functions of hsa_circ_0031968 were further examined. Through the CSCD, 46 potential target miRNAs were identified. After intersection with the DEMs, a total of 32 miRNAs were obtained. Using the three databases TargetScan, miRTarBase, and miRDB, a total of 342 mRNA molecules were identified as candidate genes. GO functional enrichment analysis showed that these genes were enriched in SMAD binding (Figure 5A-a). KEGG signaling pathway enrichment analysis revealed that these genes were enriched in miRNAs related to cancer and significantly enriched in signaling pathways that the regulate pluripotency of stem cells and cellular senescence, as well as the wnt and p53 signaling pathways (Figure 5A-b and Figure 5B). The above results confirmed that the ceRNA network involving hsa_circ_0031968 plays an essential regulatory role in LUAD.
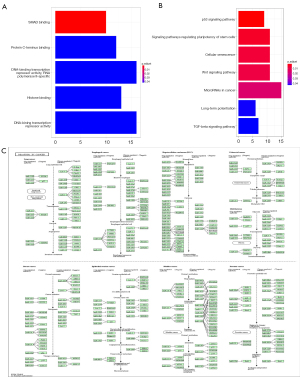
Construction of ceRNA network
Analysis of the TargetScan, miRTarBase, and miRDB databases identified 9 target genes of miR-3611. These 9 target genes and the 2484 DEmRNAs produced a unique crossover point at the proglucagon gene (GCG) (Figure 6A). This was verified by analysis of data in the TCGA database showing that the expression of GCG was significantly downregulated in LUAD samples. These results confirmed a ceRNA network consisting of hsa_circ_0031968/miR-3611/GCG in LUAD patients (Figure 6B). The expression of hsa_circ_0031968 and GCG was significantly downregulated in LUAD, while miR-3611 expression was significantly elevated in LUAD and was negatively correlated with patient survival. Furthermore, the RBPs and ORFs of hsa_circ_0031968 were examined and provide the basis for future studies (Tables 2,3).
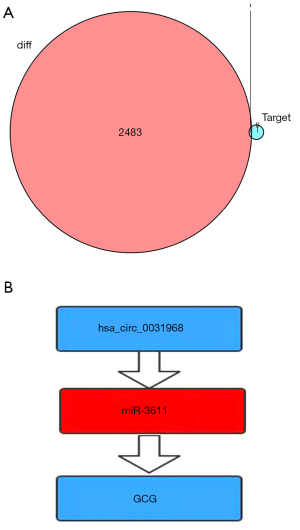
Table 2
ID | RBP |
---|---|
chr14:53331118|53331571 | FUS_Human_GSE43308_HITS-CLIP |
chr14:53331118|53331571 | eIF4AIII_Human_GSE40778_HITS-CLIP |
chr14:53331118|53331571 | PTB_Human_GSE42701_HITS-CLIP |
chr14:53331118|53331571 | U2AF65_Human_E-MTAB-1371_iCLIP |
chr14:53331118|53331571 | UPF1_Human_GSE47976_iCLIP |
chr14:53331118|53331571 | AGO2_Human_GSE42701_HITS-CLIP |
chr14:53331118|53331571 | HuR_Human_GSE28865_PAR-CLIP |
chr14:53331118|53331571 | IGF2BP3_Human_GSE21918_PAR-CLIP |
chr14:53331118|53331571 | IGF2BP1_Human_GSE21918_PAR-CLIP |
chr14:53331118|53331571 | IGF2BP2_Human_GSE21918_PAR-CLIP |
chr14:53331118|53331571 | AGO2_Human_GSE28865_HITS-CLIP |
chr14:53331118|53331571 | AGO2_Human_GSE21918_PAR-CLIP |
chr14:53331118|53331571 | LIN28A_Human_GSE44615_PAR-CLIP |
chr14:53331118|53331571 | LIN28B_Human_GSE44615_PAR-CLIP |
RBPs, RNA-binding proteins.
Table 3
ORF | Term |
---|---|
lcl|ORF1 | MDGSLQISLQRQDHGGQFLQLRSSEYSFLSEDAAFKPRSSVNTRADHD |
lcl|ORF2 | MIPVAEGMNEIWLRCDNEKQYAHWMAACRLASKGKTMADSSYNLEVQNILSFLKMQHLNPDPQLIPEQITTDITPECLVSPRYLKKYKNKQ |
lcl|ORF3 | MFFIVTTKPDFIHAFCNWNQEFNVKFLA |
lcl|ORF4 | MSAMVLPLEANLQAAIQCAYCFSLSQRSQISFMPSATGIRSLMLNFWPEMFTSGVTSH |
lcl|ORF5 | MVLTEDLGLNAASSERKEYSELLSCKNCPPWSCLWRLICRLPSSVHTVFHCHNEARFHSCLLQLESGV |
ORF, open reading frame.
Survival analysis and clinical correlation analysis
Relevant data in the TCGA database were analyzed to examine the biological effects of GCG in LUAD and its possible mechanisms of action. Kaplan-Meier survival analysis revealed that survival was significantly improved in patients with high GCG expression compared to patients with low GCG expression (P<0.05; Figure 7A). In addition, the expression of GCG was significantly reduced in patients with T2 LUAD compared to patients with T1 staging (P<0.05). Although the GCG expression levels of other staging subgroups were not statistically different, the median GCG expression for each subgroup gradually decreased with increasing T stage (Figure 7B-a). However, the expression of GCG was not significantly different between patients of different ages, genders, nor different N stages (Figure 7B-b-d).
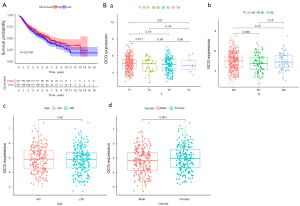
Construction of the PPI network
The final PPI network of the target gene was constructed using data from the STRING (v11.0) database and CytoHubba plug-in was used to identify the hub genes in the PPI network. The results highlighted the critical role of GCG in the pathophysiological process of LUAD and identified 17 hub genes, including cholecystokinin (CCK) and calcitonin-related polypeptide alpha (CALCA), that showed significant interact with GCG (Figure 8).
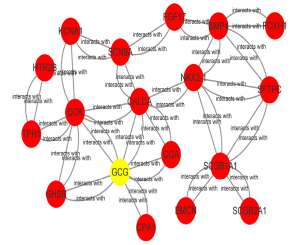
qRT-PCR and Western blot analysis results
qRT-PCR analysis in 36 paired LUAD samples demonstrated that hsa_circ_0031968 and GCG expression were significantly downregulated in LUAD tissues compared to normal adjacent tissues by 1.294±1.038 fold and 0.454±0.758 fold, respectively. Conversely, miR-3611 expression in LUAD samples was upregulated by 0.889±0.716 fold compared to normal adjacent tissues (Figure 9A-9C). These results were further validated by Western blot analyses which showed a significant decrease in GCG protein expression in LUAD samples compared to normal adjacent tissues (P<0.01; Figure 9D).
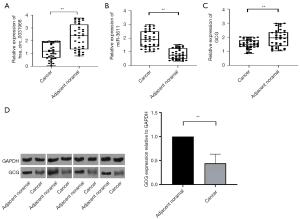
Discussion
Despite significant progress in the early detection and treatment of LUAD, many patients still suffer from poor prognosis (23). With advances in sequencing technology, bioinformatics, and molecular biology, circRNAs have been identified as ideal targets for the early detection, diagnosis, and treatment of tumors due to its high stability and remarkable temporal and spatial specificity (24). The main mechanism of action of circRNAs is through the ceRNA regulatory network that involves miRNA sponge adsorption (25-28). This study used bioinformatics techniques on relevant data from the GEO and TCGA databases to screen for essential genes involved in LUAD and to construct a potential regulatory model. This information may lead to the discovery of potential new biological markers and treatments for patients with LUAD.
The hsa_circ_0031968/miR-3611/GCG axis was identified in LUAD samples and verified through qRT-PCR and Western blot experiments. LUAD samples showed low expression of hsa_circ_0031968 and GCG, but enhanced expression of miR-3611. The GO enrichment analysis of hsa_circ_0031968 suggested that the target mRNAs were enriched in SMAD binding. The SMAD family includes 8 members, SMAD1 to 9, which can function through multiple signaling pathways (29) to affect the pathophysiological processes of tumor cell proliferation, differentiation, migration, and apoptosis (30). Previous reports have confirmed that the SMAD proteins are associated with LC. For example, SMAD1 is related to LUAD differentiation and lymphatic metastasis (31), and SMAD2/3 can promote the growth and metastasis of LC (32). However, SMAD6, 7, and 9 are significantly downregulated in non-small cell lung cancer (NSCLC) (33). The KEGG pathway analysis showed that the target mRNAs are enriched primarily in miRNAs related to cancer, further confirming that the ceRNA regulation mechanism in hsa_circ_0031968 has excellent potential to play a critical regulatory role in the pathogenesis or progression of LUAD. In addition, the target mRNAs are also significantly enriched in signaling pathways that regulate the pluripotency of stem cells and cellular senescence, as well as the Wnt and p53 signaling pathways. Indeed, many genes can regulate the pathogenesis and progression of LC through these signaling pathways. For example, thyroid hormone receptor interacting protein 13 (TRIP13) and Pleckstrin homology like domain family A member 3 (PHLDA3) can promote the proliferation and invasion of LUAD by activating the Wnt signaling pathway (34,35). Retinoic acid inducible gene G (RIG-G) and tandem C2 domains nuclear (TC2N) can affect the proliferation and apoptosis of LC by regulating the activity of the p53 signaling pathway (36,37). Therefore, studying these signaling pathways may help to predict the progression of LUAD.
The GCG gene encodes a glucagon protein that stabilizes blood sugar in the body by increasing gluconeogenesis and reducing glycolysis (38). Studies have confirmed that tumors can lead to lower blood sugar levels. Hypoglycemia may represent a paraneoplastic symptom and is often associated with poor survival and prognosis (39). This current investigation demonstrated that the expression of GCG was reduced in LUAD samples, and patients with lower GCG expression showed poorer survival. Furthermore, with an increase in T staging, the expression of GCG showed a gradually decreasing trend. Therefore, GCG may be an effective novel biomarker in patients with LUAD. In addition, the 17 hub genes of GCG that were identified through the PPI network, including CALCA (40), CGA (41), CCK (42), GHSR (43), and NKX2-1 (44), have been demonstrated to directly or indirectly participate in LC. This further suggested that LUAD can be regulated by GCG.
In summary, this report successfully established the hsa_circ_0031968/miR-3611/GCG ceRNA network in LUAD samples. The significance of our research lies in the discovery and preliminary verification that hsa_circ_0031968 can play an essential regulatory role in the occurrence and development of LUAD through the miR-3611/GCG axis. This research provides novel insights into the identification of potential biomarkers and the pathogenesis of LUAD, thereby facilitating the early diagnosis and effective treatment of LUAD patients.
There were some limitations to this research. First, the predictive value of hsa_circ_0031968 is unclear and should be further verified through molecular biology or cellular experiments. Second, the sample size of the study was small, and the sample size of the microarray data set was limited. Further improvements and supplementation of data and information are warranted to improve the reliability of the conclusions.
Conclusions
This investigation successfully constructed the hsa_circ_0031968/miR-3611/GCG ceRNA regulatory network and provides novel insights into the pathogenesis of LUAD and may aid in the development of potential biomarkers and therapies for the management of patients with LUAD.
Acknowledgments
Funding: None.
Footnote
Reporting Checklist: The authors have completed the MDAR reporting checklist. Available at https://dx.doi.org/10.21037/atm-21-5854
Data Sharing Statement: Available at https://dx.doi.org/10.21037/atm-21-5854
Conflicts of Interest: All authors have completed the ICMJE uniform disclosure form (available at https://dx.doi.org/10.21037/atm-21-5854). The authors have no conflicts of interest to declare.
Ethical Statement: The authors are accountable for all aspects of the work in ensuring that questions related to the accuracy or integrity of any part of the work are appropriately investigated and resolved. The study conformed to the provisions of the Declaration of Helsinki (as revised in 2013). All content and processes strictly comply with relevant requirements and have been reviewed and approved by the Ethics Review Committee of Life Sciences, Zhengzhou University, China. Informed consent was obtained from all patients.
Open Access Statement: This is an Open Access article distributed in accordance with the Creative Commons Attribution-NonCommercial-NoDerivs 4.0 International License (CC BY-NC-ND 4.0), which permits the non-commercial replication and distribution of the article with the strict proviso that no changes or edits are made and the original work is properly cited (including links to both the formal publication through the relevant DOI and the license). See: https://creativecommons.org/licenses/by-nc-nd/4.0/.
References
- Chen W, Zheng R, Baade PD, et al. Cancer statistics in China, 2015. CA Cancer J Clin 2016;66:115-32. [Crossref] [PubMed]
- Duma N, Santana-Davila R, Molina JR. Non-small cell lung cancer: epidemiology, screening, diagnosis, and treatment. Mayo Clin Proc 2019;94:1623-40. [Crossref] [PubMed]
- Horst C, Callister MEJ, Janes SM. Low-dose computed tomography screening: the (other) lung cancer revolution. Clin Oncol (R Coll Radiol) 2019;31:697-701. [Crossref] [PubMed]
- Torre LA, Bray F, Siegel RL, et al. Global cancer statistics, 2012. CA Cancer J Clin 2015;65:87-108. [Crossref] [PubMed]
- Vijayvergia N, Mehra R. Clinical challenges in targeting anaplastic lymphoma kinase in advanced non-small cell lung cancer. Cancer Chemother Pharmacol 2014;74:437-46. [Crossref] [PubMed]
- Wang Y, Xu R, Zhang D, et al. Circ-ZKSCAN1 regulates FAM83A expression and inactivates MAPK signaling by targeting miR-330-5p to promote non-small cell lung cancer progression. Transl Lung Cancer Res 2019;8:862-75. [Crossref] [PubMed]
- Zhang Y, Li W, Lin Z, et al. The Long Noncoding RNA Linc01833 Enhances Lung Adenocarcinoma Progression via MiR-519e-3p/S100A4 Axis. Cancer Manag Res 2020;12:11157-67. [Crossref] [PubMed]
- Su H, Tao T, Yang Z, et al. Circular RNA cTFRC acts as the sponge of MicroRNA-107 to promote bladder carcinoma progression. Mol Cancer 2019;18:27. [Crossref] [PubMed]
- Su M, Xiao Y, Ma J, et al. Circular RNAs in Cancer: emerging functions in hallmarks, stemness, resistance and roles as potential biomarkers. Mol Cancer 2019;18:90. [Crossref] [PubMed]
- Zhang K, Liu J, Song Q, et al. DNA nanosponge for adsorption and clearance of intracellular miR-21 and enhanced antitumor chemotherapy. ACS Appl Mater Interfaces 2019;11:46604-13. [Crossref] [PubMed]
- Tay Y, Rinn J, Pandolfi PP. The multilayered complexity of ceRNA crosstalk and competition. Nature 2014;505:344-52. [Crossref] [PubMed]
- Kim HH, Kuwano Y, Srikantan S, et al. HuR recruits let-7/RISC to repress c-Myc expression. Genes Dev 2009;23:1743-8. [Crossref] [PubMed]
- Jiang WD, Yuan PC. Molecular network-based identification of competing endogenous RNAs in bladder cancer. PLoS One 2019;14:e0220118. [Crossref] [PubMed]
- Zeng K, He B, Yang BB, et al. The pro-metastasis effect of circANKS1B in breast cancer. Mol Cancer 2018;17:160. [Crossref] [PubMed]
- Fang Z, Fan R, Lu Y, et al. Circular RNA hsa_circ_0002124 promotes hepatocellular carcinoma cell proliferation through the MAPK pathway. Transl Cancer Res 2019;8:367-78. [Crossref]
- Guan YJ, Ma JY, Song W. Identification of circRNA-miRNA-mRNA regulatory network in gastric cancer by analysis of microarray data. Cancer Cell Int 2019;19:183. [Crossref] [PubMed]
- Shi Y, Chen X, Xi B, et al. STAT3 activation regulated circ-STAT3.46 promote expression of IGF1R by sponging of miR-139-5p in human colon cancer. Transl Cancer Res 2019;8:2593-601. [Crossref]
- Zhang J, Liu H, Hou L, et al. Circular RNA_LARP4 inhibits cell proliferation and invasion of gastric cancer by sponging miR-424-5p and regulating LATS1 expression. Mol Cancer 2017;16:151. [Crossref] [PubMed]
- Song W, Fu T. Circular RNA-associated competing endogenous RNA network and prognostic nomogram for patients with colorectal cancer. Front Oncol 2019;9:1181. [Crossref] [PubMed]
- Chen C, Deng L, Nie DK, et al. Circular RNA Pleiotrophin promotes carcinogenesis in glioma via regulation of microRNA-122/SRY-box transcription factor 6 axis. Eur J Cancer Prev 2020;29:165-73. [Crossref] [PubMed]
- Meng S, Zhou H, Feng Z, et al. CircRNA: functions and properties of a novel potential biomarker for cancer. Mol Cancer 2017;16:94. [Crossref] [PubMed]
- Zong L, Sun Q, Zhang H, et al. Increased expression of circRNA_102231 in lung cancer and its clinical significance. Biomed Pharmacother 2018;102:639-44. [Crossref] [PubMed]
- Siegelin MD, Borczuk AC. Epidermal growth factor receptor mutations in lung adenocarcinoma. Lab Invest 2014;94:129-37. [Crossref] [PubMed]
- Ren G, Zhao Q, Yan C, et al. Circular RNA circZFR promotes tumorigenic capacity of lung cancer via CCND1. Transl Cancer Res 2020;9:3303-11. [Crossref]
- Fan Y, Xia X, Zhu Y, et al. Circular RNA expression profile in laryngeal squamous cell carcinoma revealed by microarray. Cell Physiol Biochem 2018;50:342-52. [Crossref] [PubMed]
- Bartel DP. MicroRNAs: target recognition and regulatory functions. Cell 2009;136:215-33. [Crossref] [PubMed]
- Mao W, Huang X, Wang L, et al. Circular RNA hsa_circ_0068871 regulates FGFR3 expression and activates STAT3 by targeting miR-181a-5p to promote bladder cancer progression. J Exp Clin Cancer Res 2019;38:169. [Crossref] [PubMed]
- Zhang M, Xia B, Xu Y, et al. Circular RNA (hsa_circ_0051240) promotes cell proliferation, migration and invasion in ovarian cancer through miR-637/KLK4 axis. Artif Cells Nanomed Biotechnol 2019;47:1224-33. [Crossref] [PubMed]
- Heldin CH, Miyazono K, ten Dijke P. TGF-beta signalling from cell membrane to nucleus through SMAD proteins. Nature 1997;390:465-71. [Crossref] [PubMed]
- Derynck R, Zhang YE. Smad-dependent and Smad-independent pathways in TGF-beta family signalling. Nature 2003;425:577-84. [Crossref] [PubMed]
- Gao YQ, Liu M, Zhang H. Expression profiles of SMAD1 protein in lung cancer tissues and normal tissues and its effect on lung cancer incidence. J Biol Regul Homeost Agents 2016;30:165-71. [PubMed]
- Tang YN, Ding WQ, Guo XJ, et al. Epigenetic regulation of Smad2 and Smad3 by profilin-2 promotes lung cancer growth and metastasis. Nat Commun 2015;6:8230. [Crossref] [PubMed]
- Zeng Z, Yang Y, Qing C, et al. Distinct expression and prognostic value of members of SMAD family in non-small cell lung cancer. Medicine (Baltimore) 2020;99:e19451. [Crossref] [PubMed]
- Li ZH, Lei L, Fei LR, et al. TRIP13 promotes the proliferation and invasion of lung cancer cells via the Wnt signaling pathway and epithelial-mesenchymal transition. J Mol Histol 2021;52:11-20. [Crossref] [PubMed]
- Lei L, Wang Y, Li ZH, et al. PHLDA3 promotes lung adenocarcinoma cell proliferation and invasion via activation of the Wnt signaling pathway. Lab Invest 2021;101:1130-41. [Crossref] [PubMed]
- Sun J, Wang X, Liu W, et al. Novel evidence for retinoic acid-induced G (Rig-G) as a tumor suppressor by activating p53 signaling pathway in lung cancer. FASEB J 2020;34:11900-12. [Crossref] [PubMed]
- Hao XL, Han F, Zhang N, et al. TC2N, a novel oncogene, accelerates tumor progression by suppressing p53 signaling pathway in lung cancer. Cell Death Differ 2019;26:1235-50. [Crossref] [PubMed]
- Inculet RI, Peacock JL, Gorschboth CM, et al. Gluconeogenesis in the tumor-influenced rat hepatocyte: importance of tumor burden, lactate, insulin, and glucagon. J Natl Cancer Inst 1987;79:1039-46. [PubMed]
- Iglesias P, Díez JJ. Management of endocrine disease: a clinical update on tumor-induced hypoglycemia. Eur J Endocrinol 2014;170:R147-57. [Crossref] [PubMed]
- Zheng Q, Min S, Zhou Q. Identification of potential diagnostic and prognostic biomarkers for LUAD based on TCGA and GEO databases. Biosci Rep 2021;41:BSR20204370. [Crossref] [PubMed]
- Gregorc V, Spreafico A, Floriani I, et al. Prognostic value of circulating chromogranin A and soluble tumor necrosis factor receptors in advanced nonsmall cell lung cancer. Cancer 2007;110:845-53. [Crossref] [PubMed]
- Moody TW, Nuche-Berenguer B, Moreno P, et al. CI-988 inhibits EGFR transactivation and proliferation caused by addition of CCK/gastrin to lung cancer cells. J Mol Neurosci 2015;56:663-72. [Crossref] [PubMed]
- Zhu J, Yao J, Huang R, et al. Ghrelin promotes human non-small cell lung cancer A549 cell proliferation through PI3K/Akt/mTOR/P70S6K and ERK signaling pathways. Biochem Biophys Res Commun 2018;498:616-20. [Crossref] [PubMed]
- Yang L, Lin M, Ruan WJ, et al. Nkx2-1: a novel tumor biomarker of lung cancer. J Zhejiang Univ Sci B 2012;13:855-66. [Crossref] [PubMed]
(English Language Editor: J. Teoh)