Self-supervised learning assisted diagnosis for mitral regurgitation severity classification based on color Doppler echocardiography
Introduction
Mitral regurgitation (MR) is the most common valve lesion worldwide and is a growing public health problem. Moderate-to-severe MR is associated with significant morbidity and mortality (1-4). Since new methods to treat MR are becoming increasingly available, and such interventions significantly reduce mortality and morbidity (5), screening and rapid diagnosis becomes ever more important.
Color Doppler echocardiography is a primary clinical tool for diagnosing and quantifying MR. However, the quantitative assessment of MR severity is challenging, since the standard methods recommended by current American Society of Echocardiography (ASE) (6) and European Society of Cardiology (ESC) guidelines (7) employ either proximal isovelocity surface area (PISA) or stroke volume (SV) to measure regurgitation volume and effective regurgitant orifice area (EROA), respectively. These methods require multi-step processes and are time-consuming and associated with large inter-observer variability. However, in clinical practice, Wang et al. (8,9) reported that ~90% of echocardiographers only use visual assessment of the color Doppler MR jet to grade MR severity and that guideline-recommended quantitative methods were used relatively infrequently. However, visual evaluation relies on the experience of the echocardiographer and it is difficult to evaluate borderline lesions, even for senior echocardiographers. The required quantitative measurements can be helpful, but in actual clinical practice are time-consuming to obtain and are not in widespread use. Therefore, automated algorithms which can provide quantitative indexes have the potential to improve accuracy for grading MR severity in clinical practice.
Deep-learning (DL) based methods, which are automatic and plug-and-play, already proved to be efficient in performing various computer vision tasks, including automatic image feature extraction from medical images (10,11). Moghaddasi et al. (12) developed models with high sensitivity and specificity for MR quantification from apical 4-chamber (A4C) two-dimensional (2D) echocardiographic video views but their methods failed to incorporate information from color Doppler videos. To the best of our knowledge, no prior study developed a DL plug-and-play model to automatedly analyze color Doppler videos for MR diagnosis and quantification.
However, as a data-driving technique, the requirement of manual annotation of videos by experts to train DL models is time-consuming and laborious; this has led to the emergence of self-supervised learning (SSL) algorithms (13) to enhance the learning capability through exploration of large amounts of unlabeled data. Several studies have utilized SSL for analysis of medical images (14,15). However, this approach has not been used for analysis of color Doppler echocardiographic videos.
Accordingly, the primary aim of this study was to develop an SSL model for 2D color Doppler video feature extraction and MR jet segmentation and investigate whether the SSL model could improve diagnostic accuracy of physicians with varying degrees of training in the interpretation of echocardiographic studies in practice. Furthermore, the secondary aim of our study was to assess the feasibility of directly using the semi-quantitative indicators extracted by the artificial intelligence (AI) model to grade MR severity.
This study was reported following the Standards for Reporting Diagnostic Accuracy (STARD) reporting checklist (available at https://dx.doi.org/10.21037/atm-21-3449).
Methods
Study design and patients
The overall process of patient selection is detail in Figure 1. We constructed a retrospective cohort of 2,766 consecutive echocardiographic studies of patients with MR diagnosed based on clinical criteria from two hospitals in Beijing, China (584 studies from the First Medical Center and 2,182 studies from the Fourth Medical Center of the Chinese PLA General Hospital) from September 1, 2019 and September 1, 2020. Inclusion criteria were as follows: (I) be obtained from patients ≥18 years old; (II) mild, moderate or severe MR be listed in the clinical echocardiographic report; and (III) at a minimum, the study included an A4C color Doppler (A4C-CDI) video clip. From these, we first established the test dataset by selecting studies that included all views required for quantitative assessment of MR severity according to strict adherence to society guidelines (SV method); namely parasternal long-axis-2d, A4C-mitral valve-2d, A4C-mitral valve-pulse wave, A4C-mitral valve-continuous wave, A5C-aortic valve-pulse wave. Of the original 2,766 studies in the database, only 148 studies fulfilled all these criteria. MR severity of the remaining studies were classified according to the diagnosis included in the echocardiographic report; these studies were used to develop the segmentation algorithm for the A4C-CDI video clips. The diagnoses of these latter studies were only used to stratify studies during random sampling to achieve matched proportions of studies with mild, moderate and severe MR within the training, validation and test datasets. By design, we aimed for a 4:1:1 ratio in the number of studies in the training, validation and test datasets. Since the number of studies in the test dataset was constrained at 148, this mandated a training set of 592 studies and a validation set of 148 studies; these studies were randomly selected from among the remaining 2,618 available studies. As noted, these studies were selected to achieve consistent proportions of cases with mild, moderate and severe disease as in the test dataset (numbers detailed in Figure 1). Finally, 592 echocardiograms deemed to have normal heart size and function without MR or other disease were selected from the Fourth Medical Center of Chinese PLA General Hospital for inclusion into an additional pre-training dataset which was used only for the purpose of feature extraction; namely, for training the model to segment the left ventricular (LV) and left atrium (LA) contours.
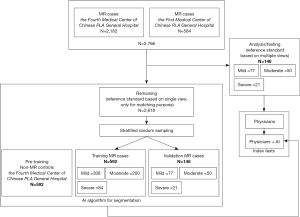
The study was registered the Chinese clinical trial registry (ChiCTR2000030278). The study was conducted in accordance with the Declaration of Helsinki (as revised in 2013). This study was approved by Ethical Review of Scientific Research Projects of the Medical Ethics Committee of the Chinese PLA General Hospital, for the use of deidentified echocardiographic and patient demographic data (No. S2019-319-01). Individual consent for this retrospective analysis was waived.
Test methods
Technical details and preprocessing of echocardiograms
Each echocardiographic study included A4C-CDI video clips, all of which were acquired by Phillips echocardiography machine (iE-elite and 7C with transducer S5-1 and X5-1; Phillips, Andover, MA, USA) using a Nyquist limit of 50 to 60 cm/s. These video clips were identified automatically from each study using a previously developed and validated view classification algorithm having an accuracy of >90% (10). All original DICOM images and associated clinical reports were obtained for analysis in this study.
For a given study, two expert echocardiographers selected the frame with the maximum MR jet area. They manually segmented the LA area and MR jet using LabelMe (16). These manually selected frames and segmentation labels were used as the ground truth for training the segmentation network.
Automatic self-supervised feature extraction
An MR jet recognition and segmentation algorithm was trained for feature extraction, which we named color doppler SSL, or “CD-SSL”. This algorithm consists of two stages: (I) a novel SSL model for color Doppler echocardiography feature extraction; and (II) multi-task transfer learning algorithm for MR recognition and segmentation. The technical details of this SSL algorithm are provided in the Appendix 1.
For the first stage, we developed a novel proxy task, which consists of structure recovery and color transform toleration to force the network to deeply exploit color-correlated and transformation-invariant information from the color Doppler echocardiography videos without information on MR classification (Figure 2). In this step, 1,184 color Doppler echocardiography videos (592 normal samples and 592 MR samples) were employed in pre-training the network via self-supervised manner to obtain high quality feature representation; the MR cases 308 mild, 200 moderate, and 84 severe samples.
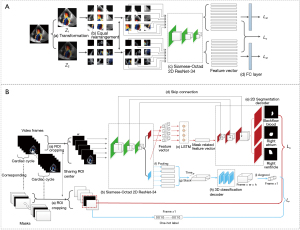
For the second stage, the videos of all MR cases, along with their segmentation annotations, were fed into the pre-trained network for the MR and LA area contours segmentation task. In the training process, 148 samples were utilized as validation dataset for tuning parameters (e.g., learning rate, batch size, optimization strategy). After a large number of iterations, we obtained a validated model for testing.
Finally, the network was employed for testing, which included the 148 MR samples. In the end, five measurements were derived from the color Doppler segmentation images (see Figure 3 for details), including MR jet length, MR jet area, LA length, LA width, LA area. Based on these measurements, we considered six candidate indexes of MR severity, including MR jet length/LA length, MR jet length, LA width, LA area, MR jet area and MR jet area/LA area. These indexes were provided to physicians to assist them in making clinical diagnoses as detailed in the next section.
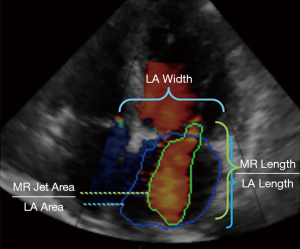
AI enhancement of physician diagnoses
We evaluated whether availability of the AI segmentation algorithm results could improve the diagnostic accuracy of physicians. For this analysis, A4C-CDI videos of the test dataset were provided to 9 physicians, who visually assessed the severity of MR based on their own experience. These 9 physicians had different years of experience, including 3 junior physicians (1–3 years), 3 physicians with intermediate experience (4–10 years) and 3 senior physicians (>10 years). Next, in a separate blinded manner, these same A4C-CDI videos were provided to the 9 physicians along with the values of the 6 indexes generated by the AI model, and they were asked to provide another assessment of MR severity. We compared the diagnostic accuracy of the physicians with and without the support of AI results. Care was taken to blind physicians from their original assessments and from ground truth to ensure independence of the two reads.
Establishing ground truth reference standard for MR classification in the test dataset
Each echocardiogram had an electronic clinical report that was used as the basis for the initial diagnosis of MR according to the 2017 ASE Recommendations for Noninvasive Evaluation of Native Valvular Regurgitation (7) confirming the presence, severity, and etiology of MR. Mild MR was defined as a central Doppler jet area <20% LA area and a vena contracta <0.3 cm. Moderate MR was defined as a central MR jet area of 20%–50% of LA area or late systolic eccentric MR jet MR, a vena contracta <0.7 cm, a regurgitant volume <60 mL and an EROA <0.40 cm2. Severe MR was defined as a central MR jet area >50% of LA area or holosystolic eccentric jet MR, a vena contracta ≥0.7 cm, a regurgitant volume ≥60 mL and an EROA ≥0.40 cm2. Moderate and severe MR was considered as positive disease status, non-moderate or severe was considered as negative.
Each of these measurements was made by two expert physician echocardiographers and the average of the values provided by the two experts was taken as reference standard. The expert physician echocardiographers who determine the reference standard were not those physicians who performed the index tests.
Sample size calculation
The sample size of the main analysis was determined by methods detailed by Alonzo et al. (17). The sensitivity and specificity with which physicians made accurate diagnoses of MR severity without support from AI were assumed to be 0.7, and we expected a 1.2-fold increase in performance (i.e., sensitivity and specificity to increase to 0.84) with the support of the AI algorithm. Based on a significance level of 0.05 and power of 0.8, the desired sample size consisted of 56 positive and 56 negative studies. Considering all studies in the main analysis dataset will be evaluated by 9 physicians, the sample size can be even reduced with this study design. So the 148 MR samples included in the main analysis were sufficient.
Statistical analysis
Continuous variables are expressed as median and interquartile range, or counts and percentages, as appropriate. The diagnostic performance of the physicians was assessed by sensitivity and specificity. These performance measures and their confidence intervals were estimated with generalized estimating equation (GEE) model, which can deal with clustered data for multiple readers (18). The diagnosis performance was also assessed in each experience group. The diagnostic performance of the indexes generated by the AI segmentation algorithm was presented with receiver operating characteristic (ROC) curve and the area under the ROC curve (AUC). Statistical analyses were conducted using R software (version 3.6.1) and packages geepack and pROC.
Results
Patient characteristics
The clinical and echocardiographic characteristics of patients included in this study are summarized in Table 1. In the 148 patients included in the main analysis, the median age was 71 (IQR: 61, 81), 98 (66.2%) were male, 21 (14.2%) had severe MR and 50 (33.8%) had moderate MR.
Table 1
Characteristics | Analysis/test | Training | Validation | Total |
---|---|---|---|---|
Patient number | 148 | 592 | 148 | 888 |
Age (years), median [IQR] | 71 [61, 81] | 69 [59, 78] | 65 [57, 79] | 69 [59, 79] |
Male, n (%) | 98 (66.2) | 386 (65.2) | 101 (68.2) | 585 (65.9) |
Etiology, n (%) | ||||
Primary MR | 8 (5.4) | 30 (5.1) | 12 (8.1) | 50 (5.6) |
Secondary MR | 140 (94.6) | 562 (94.9) | 136 (91.9) | 838 (94.4) |
Comorbidities, n (%) | ||||
Hypertension | 84 (56.8) | 320 (54.1) | 82 (55.4) | 486 (54.7) |
Hyperlipidemia | 35 (23.6) | 156 (26.4) | 32 (21.6) | 223 (25.1) |
Diabetes | 27 (18.2) | 115 (19.4) | 30 (20.3) | 172 (19.4) |
Coronary heart disease | 65 (43.9) | 272 (45.9) | 77 (52.1) | 414 (46.6) |
Myocardial infarction | 27 (18.2) | 143 (24.2) | 38 (25.6) | 208 (23.4) |
HCM | 4 (2.7) | 7 (1.2) | 4 (2.7) | 15 (1.9) |
DCM | 2 (1.4) | 10 (1.7) | 2 (1.4) | 14 (1.6) |
Lesion severity, n (%) | ||||
Mild | 77 (52.0) | 308 (52.0) | 77 (52.0) | 462 (52.0) |
Moderate | 50 (33.8) | 200 (33.8) | 50 (33.8) | 300 (33.8) |
Severe | 21 (14.2) | 84 (14.2) | 21 (14.2) | 126 (14.2) |
Echocardiographic, median [IQR] | ||||
LVEF (%) | 56 [38, 60] | 52 [39, 59] | 55 [36, 59] | 54 [38, 59] |
LVEDV (mL) | 107 [90, 144] | 114 [90, 139] | 118 [105, 147] | 107 [90, 144] |
LVESV (mL) | 48 [36, 80] | 54 [38, 78] | 59 [42, 85] | 53 [38, 78] |
LVEDD (mm) | 47 [43, 53] | 48 [45, 54] | 49 [45, 55] | 48 [45, 54] |
LA (mm) | 40 [34, 44] | 40 [35, 43] | 41 [36, 44] | 40 [35, 44] |
RA (mm) | 32 [30, 36] | 33 [30, 36] | 33 [30, 35] | 33 [30, 36] |
RV (mm) | 31 [28, 34] | 32 [29, 34] | 32 [30, 35] | 32 [29, 34] |
MR, mitral regurgitation; HCM, hypertrophic cardiomyopathy; DCM, dilated cardiomyopathy; LVEF, left ventricular ejection fraction; LVEDV, left ventricular end-diastolic volume; LVESV, left ventricular end-systolic volume; LVEDD, left ventricular end-diastolic dimension; LA, left atrium; RA, right atrium RV, right ventricular.
Evaluation of the AI segmentation model
We first evaluated the performance of our automatic segmentation framework. Examples of automated 2D color Doppler MR jet area segmentation (green line) and left atrial area segmentation (blue line) are shown in Figure 3. The performance of the framework, summarized in Table 2, yields 89.2% and 85.3% average segmentation dice similarity coefficient (DICE) in the validation and test datasets, which achieved 6.2% and 8.1% (absolute) improvements compared to a conventional Residual U-shape Network (ResNet-UNet) model, respectively.
Table 2
Dataset | Method | Max frame recognition ACC | DICE | |||
---|---|---|---|---|---|---|
MR jet | LA | AVG | DICE↑ | |||
Validation | ResNet-UNet | 92.0 | 0.811 | 0.848 | 0.829 | – |
CD-SSL | 93.1 | 0.863 | 0.920 | 0.892 | 0.063 | |
Test | ResNet-UNet | 93.5 | 0.767 | 0.776 | 0.772 | – |
CD-SSL | 95.9 | 0.821 | 0.884 | 0.853 | 0.081 |
“ResNet-UNet” indicates residual U-shaped network, which is our baseline model; “CD-SSL” indicates our segmentation framework which elaborates SSL; “max frame recognition ACC” indicates the max MR jet area frame recognition accuracy; “DICE” indicates segmentation DICE coefficient; “AVG” and “DICE↑” indicate the average dice coefficient of MR and LA and the improvement compared to the conventional ResNet-UNet model. ACC, accuracy; DICE, dice similarity coefficient; MR, mitral regurgitation; LA, left atrium; AVG, average; ResNet-UNet, Residual U-shape Network; CD-SSL, color doppler self-supervised learning.
Our framework relied on identification of the video frame with the maximum MR jet area. This process was automated by training the network in an end-to-end manner based on reference standards tagged by experts. To evaluate the reproducibility of this framework, we randomly choose a segment of 16 continuous frames, one of which contained the maximum jet area as determined by the expert grader and re-tested the algorithm. We run 10 such simulations on each of the 148 videos in the test dataset. Our model achieved an overall accuracy of 95.9% (±0.1) for identifying the frame with the maximum MR jet area.
MR indexes generated by AI segmentation model
The six quantitative indexes generated by the AI segmentation model, including MR jet length/LA length, MR jet length, LA width, LA area, MR jet area and MR jet area/LA area, had significantly different distributions among MR severity groups (box plots shown in Figure 4, all P<0.001). If these indexes were used individually for diagnosing the severity of MR, they also yielded outstanding performance. The AUCs of MR jet length/LA length (AUC =0.951), MR jet length (AUC =0.953), MR jet area (AUC =0.952) and MR jet area/LA area (AUC =0.951) were all above 0.95. However, LA width (AUC =0.683) and LA area (AUC =0.713) performed less well (Figure 5).
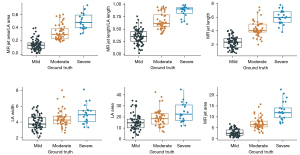
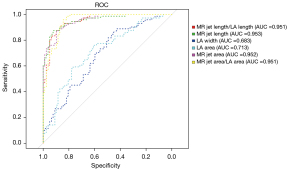
Diagnostic accuracy of physicians without and with support of AI segmentation model
When physicians made the judgement of MR grade solely based on visual assessment of the 2D color Doppler videos, they achieved a sensitivity of 77.0% (95% CI: 70.9–82.1%) and a specificity of 91.5% (95% CI: 87.8–94.1%). When physicians were supported by the AI segmentation model, the sensitivity increased to 86.7% (95% CI: 80.3–91.2%) and specificity did not change significantly, remaining at 90.5% (95% CI: 86.7–93.2%).
Availability of AI segmentation model results improved the sensitivity of grading moderate and severe MR in physicians, regardless of years of experience (Table 3). However, it was only for senior physicians with >10 years of experience where the specificity of grading increased, in this case from 93.9% (95% CI: 92.4–95.2%) to 95.7% (95% CI: 93.4–97.2%).
Table 3
Physician group | Sensitivity (%) (95% CI) | Specificity (%) (95% CI) | |||
---|---|---|---|---|---|
Without AI | With AI | Without AI | With AI | ||
All physicians (n=9) | 77.0 (70.9–82.1) | 86.7 (80.3–91.2) | 91.5 (87.8–94.1) | 90.5 (86.7–93.2) | |
Junior physicians (n=3) | 84.0 (72.6–91.3) | 95.8 (94.3–96.9) | 87.4 (78.9–92.8) | 84.4 (82.2–86.4) | |
Intermediate physicians (n=3) | 77.5 (68.7–84.3) | 85.4 (76.1–91.6) | 93.1 (89.7–95.4) | 91.3 (89.3–93.0) | |
Senior physicians (n=3) | 69.5 (68.7–70.2) | 78.9 (74.7–82.5) | 93.9 (92.4–95.2) | 95.7 (93.4–97.2) |
AI, artificial intelligence; MR, mitral regurgitation.
Discussion
The current study reveals two main technological advances in automated analysis of color Doppler videos. First, we developed and validated our SSL method for automated color Doppler video feature extraction, which considers the characteristic of color Doppler echocardiography and therefore offers an effective and automatic tool for medical-related feature extraction. With this model, we were able to accurately and reliably select and segment the frame of a video containing the maximum MR jet area. Second, we performed head-to-head comparisons among a group of physicians with different years of experience, and showed that availability of AI segmentation model results can improve the diagnostic performance of physicians.
Several prior studies (19-21) have reported automated methods for grading severity of MR. Those methods utilize three-dimensional (3D) full-volume color Doppler transthoracic echocardiography (FVCD). However, they rely on commercially available software packages which are only incorporated into the latest hardware versions which are not widely available in most clinical settings. In contrast, our model which is based on an SSL algorithm is plug-and-play and therefore has the potential for widespread application, independent of echocardiographic hardware.
In recent years, the use of AI for interpreting medial images has developed rapidly, and some studies report automatic disease identification and diagnosis with comparable accuracy to those of experienced physicians (22-25). In addition, AI avoids interobserver variability, which inherently yields reproducible results. More specifically, previous studies have employed AI platforms to investigate image analysis of echocardiograms. For instance, Zhang et al. (26) developed an algorithm for automated view identification, image segmentation, quantification of structure and function, and detection of 3 diseases. Ouyang et al. (27) developed video-based algorithms for segmenting the left ventricle, estimating ejection fraction and assessing cardiomyopathy. Huang et al. (28) tested a DL network to automate the recognition of regional wall motion abnormalities. However, those approaches are based on standard 2D echocardiography and lack the ability to capture information contained in the color aspects of the color Doppler echocardiographic videos. To overcome these limitations, we developed an SSL-based model to extract features from color Doppler echocardiograms which automatically provides segmentation prediction and indexes.
Prior studies have described SSL algorithms for natural color image feature extraction for medical imaging applications, such as Jigsaw puzzle (29) and RotNet (30). Other medical-image-based SSL methods, such as Rubik’s Cube+ (31), Models Genesis (14) and distance prediction methods (32) are ad-hoc methods proposed and applied in specific imaging modalities such as MRI or CT. In contrast, our CD-SSL considers information regarding image color and cardiac structure of color Doppler echocardiograms, thus offering a specialized and effective tool for MR severity grading.
As noted, a key feature of our method is the reliable identification of the frame containing the maximum MR jet area, which is the cornerstone of accurate grading of MR severity. The robustness of this feature was demonstrated by challenging the algorithm by randomly shifting the location of the selected frame in the video sequence. This feature of the algorithm can therefore eliminate the variability of manual selection in clinical practice.
We also investigated the potential use of individual quantitative indexes generated by the AI segmentation model from Color Doppler echocardiography, in detecting moderate and severe MR. In the clinical setting, most echocardiographers often evaluate MR severity based on visual assessment using semi-quantitative assessments of parameters such as MR jet area/LA area and MR jet length/LA length. However, it is difficult for clinicians to reliably identify the frame containing the maximum jet area and to accurately quantify and assign MR severity from these indexes, particularly for many borderline lesions. An automatic and reliable tool to diagnosis the presence and assess severity of MR would offer many advantages to improve diagnostic accuracy and workflow efficiency. The current study showed that several indexes, such as MR jet length/LA length, MR jet length, MR jet area and MR jet area/LA area, determined automatically by our DL algorithm yielded excellent diagnostic performance and may therefore be useful as diagnostic markers or candidate variables for clinical prediction models.
Limitations
The current findings need to be considered within the context of several limitations. First, grading of MR severity based on society guidelines requires analyses based on multiple 2D color, continuous and pulse wave Doppler signals. However, in clinical practice, as in our overall database, images from all required views of sufficient quality are not always available. Accordingly, by design, our algorithm relied only on the A4C view, which was reliably obtained in the overall dataset. While this limited our analysis to metrics of MR derivable from segmentation of LA and MR contours, we demonstrated that this approach yielded excellent results when compared with thorough analyses by experts based on a larger number of metrics obtained from multiple views which are required by society guidelines. Notably, all views required for such thorough analysis were available in only a ~5% (148/2,766) of echocardiograms obtained in our real-world retrospective analysis. Overall, the results showing the excellent performance of our segmentation model and index quantification algorithms demonstrate the effectiveness of this approach. Nevertheless, the DL architecture developed for the present study could readily be trained and applied to other views if so desired.
Further related to model training, our algorithm employed SSL pre-training to overcome the need for a large number of labeled images, and thus markedly reduced the burden of manual annotation.
As noted, the test dataset was selected based on the availability of sufficient views to calculate EROA. Accordingly, this may represent a biased sample with a different distribution of MR severity compared to the overall population of patients with MR. This could reduce model performance in practice. Thus, further external validation in prospective cohort studies is required.
Finally, the “black box” nature of a DL algorithm poses potential difficulties in acceptance in real-world application due to the interpretation problem, i.e., the lack transparency in how the diagnoses are made. Therefore, the current algorithm was specifically designed to provide quantitative indexes to assistant clinicians, rather than to replace physicians by making the MR classification by itself.
Conclusions
In conclusion, this study introduced and validated a new, practical, accurate, plug-and-play AI-based approach based on a single 2D color Doppler echocardiographic view for assisting physicians in MR severity grading that can be easily implemented in clinical practice. The feature extractor model was developed via an SSL approach to obtain feature representations of the disease by considering the specific characteristics of medical images, e.g., color Doppler echocardiography. The results show that this model can improve the diagnostic performance of physicians.
Acknowledgments
Funding: This research received support from the Beijing Natural Science and Technology Foundation (7202198) and Ministry of Industry and Information Technology of China (2020-0103-3-1).
Footnote
Reporting Checklist: The authors have completed the STARD reporting checklist. Available at https://dx.doi.org/10.21037/atm-21-3449
Data Sharing Statement: Available at https://dx.doi.org/10.21037/atm-21-3449
Peer Review File: Available at https://dx.doi.org/10.21037/atm-21-3449
Conflicts of Interest: All authors have completed the ICMJE uniform disclosure form (available at https://dx.doi.org/10.21037/atm-21-3449). FY reports funding from the Beijing Natural Science and Technology Foundation (7202198). DB reports being a consultant to BioMind. KH reported funding from Ministry of Industry and Information Technology of China (2020-0103-3-1). The other authors have no conflicts of interest to declare.
Ethical Statement: The authors are accountable for all aspects of the work in ensuring that questions related to the accuracy or integrity of any part of the work are appropriately investigated and resolved. The study was conducted in accordance with the Declaration of Helsinki (as revised in 2013). This study was approved by Ethical Review of Scientific Research Projects of the Medical Ethics Committee of the Chinese PLA General Hospital, for the use of deidentified echocardiographic and patient demographic data (No. S2019-319-01). Individual consent for this retrospective analysis was waived.
Open Access Statement: This is an Open Access article distributed in accordance with the Creative Commons Attribution-NonCommercial-NoDerivs 4.0 International License (CC BY-NC-ND 4.0), which permits the non-commercial replication and distribution of the article with the strict proviso that no changes or edits are made and the original work is properly cited (including links to both the formal publication through the relevant DOI and the license). See: https://creativecommons.org/licenses/by-nc-nd/4.0/.
References
- Enriquez-Sarano M, Akins CW, Vahanian A. Mitral regurgitation. Lancet 2009;373:1382-94. [Crossref] [PubMed]
- Go AS, Mozaffarian D, Roger VL, et al. Executive summary: heart disease and stroke statistics--2013 update: a report from the American Heart Association. Circulation 2013;127:143-52. [Crossref] [PubMed]
- Helmcke F, Nanda NC, Hsiung MC, et al. Color Doppler assessment of mitral regurgitation with orthogonal planes. Circulation 1987;75:175-83. [Crossref] [PubMed]
- Nkomo VT, Gardin JM, Skelton TN, et al. Burden of valvular heart diseases: a population-based study. Lancet 2006;368:1005-11. [Crossref] [PubMed]
- Kalavrouziotis D, Voisine P, Mohammadi S. Transcatheter Mitral-Valve Repair in Patients with Heart Failure. N Engl J Med 2019;380:1979-80. [PubMed]
- Zoghbi WA, Adams D, Bonow RO, et al. Recommendations for Noninvasive Evaluation of Native Valvular Regurgitation: A Report from the American Society of Echocardiography Developed in Collaboration with the Society for Cardiovascular Magnetic Resonance. J Am Soc Echocardiogr 2017;30:303-71. [Crossref] [PubMed]
- Baumgartner H, Falk V, Bax JJ, et al. 2017 ESC/EACTS Guidelines for the management of valvular heart disease. Kardiol Pol 2018;76:1-62. [Crossref] [PubMed]
- Wang A, Grayburn P, Foster JA, et al. Practice gaps in the care of mitral valve regurgitation: Insights from the American College of Cardiology mitral regurgitation gap analysis and advisory panel. Am Heart J 2016;172:70-9. [Crossref] [PubMed]
- Kamoen V, Calle S, El Haddad M, et al. Diagnostic and Prognostic Value of Several Color Doppler Jet Grading Methods in Patients With Mitral Regurgitation. Am J Cardiol 2021;143:111-7. [Crossref] [PubMed]
- Gandhi S, Mosleh W, Shen J, et al. Automation, machine learning, and artificial intelligence in echocardiography: A brave new world. Echocardiography 2018;35:1402-18. [Crossref] [PubMed]
- Al'Aref SJ, Anchouche K, Singh G, et al. Clinical applications of machine learning in cardiovascular disease and its relevance to cardiac imaging. Eur Heart J 2019;40:1975-86. [Crossref] [PubMed]
- Moghaddasi H, Nourian S. Automatic assessment of mitral regurgitation severity based on extensive textural features on 2D echocardiography videos. Comput Biol Med 2016;73:47-55. [Crossref] [PubMed]
- Kolesnikov A, Zhai X, Beyer L. Revisiting self-supervised visual representation learning. In: Proceedings of the IEEE/CVF conference on computer vision and pattern recognition. 2019:1920-9.
- Zhou Z, Sodha V, Pang J, et al. Models Genesis. Med Image Anal 2021;67:101840. [Crossref] [PubMed]
- Bai W, Chen C, Tarroni G, et al. Self-supervised learning for cardiac MR image segmentation by anatomical position prediction. In: International Conference on Medical Image Computing and Computer-Assisted Intervention. Cham: Springer, 2019:541-9.
- Russell BC, Torralba A, Murphy KP, et al. LabelMe: a database and web-based tool for image annotation. Int J Comput Vis 2008;77:157-73. [Crossref]
- Alonzo TA, Pepe MS, Moskowitz CS. Sample size calculations for comparative studies of medical tests for detecting presence of disease. Stat Med 2002;21:835-52. [Crossref] [PubMed]
- Genders TS, Spronk S, Stijnen T, et al. Methods for calculating sensitivity and specificity of clustered data: a tutorial. Radiology 2012;265:910-6. [Crossref] [PubMed]
- Son JW, Chang HJ, Lee JK, et al. Automated quantification of mitral regurgitation by three dimensional real time full volume color Doppler transthoracic echocardiography: a validation with cardiac magnetic resonance imaging and comparison with two dimensional quantitative methods. J Cardiovasc Ultrasound 2013;21:81-9. [Crossref] [PubMed]
- Heo R, Son JW, Ó Hartaigh B, et al. Clinical Implications of Three-Dimensional Real-Time Color Doppler Transthoracic Echocardiography in Quantifying Mitral Regurgitation: A Comparison with Conventional Two-Dimensional Methods. J Am Soc Echocardiogr 2017;30:393-403.e7. [Crossref] [PubMed]
- Jeganathan J, Knio Z, Amador Y, et al. Artificial intelligence in mitral valve analysis. Ann Card Anaesth 2017;20:129-34. [Crossref] [PubMed]
- Das N, Topalovic M, Janssens W. Artificial intelligence in diagnosis of obstructive lung disease: current status and future potential. Curr Opin Pulm Med 2018;24:117-23. [Crossref] [PubMed]
- Rajkomar A, Dean J, Kohane I. Machine Learning in Medicine. N Engl J Med 2019;380:1347-58. [Crossref] [PubMed]
- Ehteshami Bejnordi B, Veta M, Johannes van Diest P, et al. Diagnostic Assessment of Deep Learning Algorithms for Detection of Lymph Node Metastases in Women With Breast Cancer. JAMA 2017;318:2199-210. [Crossref] [PubMed]
- Esteva A, Kuprel B, Novoa RA, et al. Dermatologist-level classification of skin cancer with deep neural networks. Nature 2017;542:115-8. [Crossref] [PubMed]
- Zhang J, Gajjala S, Agrawal P, et al. Fully Automated Echocardiogram Interpretation in Clinical Practice. Circulation 2018;138:1623-35. [Crossref] [PubMed]
- Ouyang D, He B, Ghorbani A, et al. Video-based AI for beat-to-beat assessment of cardiac function. Nature 2020;580:252-6. [Crossref] [PubMed]
- Huang MS, Wang CS, Chiang JH, et al. Automated Recognition of Regional Wall Motion Abnormalities Through Deep Neural Network Interpretation of Transthoracic Echocardiography. Circulation 2020;142:1510-20. [Crossref] [PubMed]
- Noroozi M, Favaro P. Unsupervised learning of visual representations by solving jigsaw puzzles. In: European conference on computer vision. Cham: Springer, 2016:69-84.
- Gidaris S, Singh P, Komodakis N. Unsupervised representation learning by predicting image rotations. arXiv 2018:1803.07728.
- Zhu J, Li Y, Hu Y, et al. Rubik's Cube+: A self-supervised feature learning framework for 3D medical image analysis. Med Image Anal 2020;64:101746. [Crossref] [PubMed]
- Spitzer H, Kiwitz K, Amunts K, et al. Improving cytoarchitectonic segmentation of human brain areas with self-supervised siamese networks. In: International Conference on Medical Image Computing and Computer-Assisted Intervention. Cham: Springer, 2018:663-71.