A radiomics model combined with XGBoost may improve the accuracy of distinguishing between mediastinal cysts and tumors: a multicenter validation analysis
Introduction
Mediastinal cysts (MCs) are rare, usually benign lesions which mainly occur during embryonic development. They consist of 3 main subtypes: bronchogenic cysts, pleuropericardial cysts, and enteric or duplication cysts (1-3). MCs are generally asymptomatic, unless compressive symptoms develop due to a large tumor size (4,5). MCs convert to malignancies in very few cases; therefore, in affected patients, surveillance could be an alternative to surgical resection (6). Some study suggested potential clinical model for the prediction of MCs (7,8), however, it is difficult to differentiate between nonneoplastic MCs and more aggressive mediastinal tumors (MTs) such as thymomas or thymic carcinomas, especially in cases of half-solid and soft-tissue-like lesions (9,10).
Chest radiography is routinely used for the detection and diagnosis of mediastinal lesions and usually shows a well-delimited, homogeneous, spherical mediastinal image (11,12). With fluid intensity signals on T1 and T2 sequences, magnetic resonance imaging (MRI) provides a better definition of cysts (13,14) and offers superior visualization of invasion to neighboring structures than that afforded by computed tomography (CT) (15). A previous study showed that the rate of correct first-choice diagnosis of MCs using CT, MRI, and both methods was 61%, 56%, and 67%, respectively, indicating considerable scope for improvement (16). Mediastinal fine-needle aspiration cytology is a fairly specific technique for the diagnosis of mediastinal masses in patients for whom invasive diagnostic surgery is not feasible (17,18). However, it is not routinely recommended due to the potential risks of damage to adjacent organs. Thus, in clinical practice, differentiating MCs remains challenging due to their particular location.
With the rapid development of radiomics, high-order image features are being used to describe the textural characteristics of mediastinal lesions on medical images. By combining image-filtering and feature-extraction methods, it is possible to extract a large number of high-order radiomic features from CT images. Studies have shown significant radiomic parameters correlating to thymic tumor histology, such as skewness, kurtosis, and entropy (19). However, due to the similar appearances of MCs and MTs on CT images, even traditional machine learning models have difficulties in achieving satisfactory discriminatory performance.
Ensemble learning is often used to integrate multiple machine learning models for better prediction of complex medical images with a very large number of image features. Each model in ensemble learning uses a part of the entire feature set, and this approach is usually better than a single model in terms of model performance and model generalizability. Our study aimed to explore a novel model using radiomics combined with eXtreme Gradient Boosting (XGBoost), an advanced type of boosting algorithm belonging to the ensemble learning family, for the diagnosis of anterior mediastinal masses, thus providing a prediction tool for invasive medical decisions. We present the following article in accordance with the STARD reporting checklist (available at https://dx.doi.org/10.21037/atm-21-5999).
Methods
Patient eligibility
Patients with anterior mediastinal lesions admitted to Shanghai Pulmonary Hospital between October 2014 and January 2017 were enrolled in the study. Only patients diagnosed by radiologists using Digital Imaging and Communications in Medicine (DICOM) data retrieved from Shanghai Pulmonary Hospital were included, and no missing data was found. Consecutive patients with pathological examination from surgical resection were included. All cases and patient information were collected as a training set to develop the diagnostic model. Cases between January 2017 and September 2018 were collected prospectively as a test dataset. A total of 592 patients (289 male/303 female) with anterior mediastinal lesions were enrolled in the study (age range, 18–83 years). Fifty-six patients (9.5%) complained of compression symptoms. Another dataset comprising patient information of 87 patients with anterior mediastinal lesions from Huashan Hospital of Shanghai Fudan University Medical College between January 2017 and April 2019 was collected for model validation. All procedures performed in this study involving human participants were in accordance with the Declaration of Helsinki (as revised in 2013).
Patients’ personal information was anonymized before being used for data analysis. This study protocol was approved by the institutional review board of Shanghai Pulmonary Hospital (L20-379, Shanghai, China). Patients’ written informed consent was waived by the institutional review board because of the retrospective nature of the study.
Image acquisition
All patients in Shanghai Pulmonary Hospital underwent nonenhanced and arterial-phase enhanced CT examinations with the Definition AS CT scanner (Siemens Healthineers, Erlangen, Germany). The entire chest of the patients was scanned, and all scans were obtained with patients in the supine position. For the purpose of the scan, nonionic contrast material was injected through the antecubital vein at a rate of 3.5 mL/s [1.5 mL/kg of body weight, a total of 90 to 120 mL, iohexol 300 mgI/mL (Omnipaque, GE Healthcare, Waukesha, WI, USA)]. The arterial phase scans were obtained at 30 s after injection of the contrast medium. The imaging parameters for CT were as follows: helical mode at a tube voltage of 120 kVp, tube current of 300 mA, collimation thickness of 5 mm, reconstruction thickness of 0.625 mm, rotation speed of 0.6 s, and helical pitch of 1.375. At Huashan Hospital, a Siemens CT scanner and Philips CT scanner (Eindhoven, the Netherlands) were used with similar parameters.
Radiological and pathological examinations
DICOM data were independently reviewed by a radiologist who was different from the one who prepared the original clinical report for the patient. For the pathological examination, surgically resected specimens were fixed in formalin, embedded in paraffin, sectioned with a microtome, and stained with hematoxylin and eosin (H&E). All available tumor slides stained with H&E were independently reviewed by 2 different pathologists who were blinded to the clinical outcomes.
Region of interest (ROI) delineation and radiomic feature extraction
A three-dimensional (3D) morphology of the mediastinal lesions was sketched by the aforementioned radiology expert based on two-dimensional (2D) contours on each frame of the CT images using the OsiriX medical image workstation. Indeterminate cases were settled by a third radiology expert. Based on the precise contour information obtained, a specific 3D ROI was reconstructed for each mediastinal lesion (Figure 1). The images were filtered, and from the ROIs of the filtered images, different types of radiomic features were determined, including histograms, morphological features, and various high-order textural features.
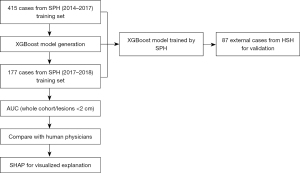
Machine learning framework
The training dataset was used to build an XGBoost model to classify MCs and MTs in the test dataset. Ten fivefold cross-validation experiments were performed using the training data, with each repetition based on random ordering of the training set. For each fivefold cross-validation, one-fifth of the samples were reserved as validation samples, and the remaining samples were used for training. The XGBoost model was fitted to each training sample to classify MT lesions on the CT images of the corresponding validation samples.
When building the XGBoost model, a large number of radiomic features were extracted. Among these, some features may have been unrelated to the prediction results or been interdependent, which could have led to the following consequences: (I) long processing time, and (II) low generalizability. Therefore, feature selection was needed to eliminate irrelevant or redundant features. Feature selection refers to the collection of a subset of features from all original features to reduce run time and train a better model.
For tree-based models, a statistical metric called ‘information gain’ is usually used to evaluate the ability of a feature to classify the data samples. The greater the information gain, the more powerful the feature for distinguishing different types of data samples. In the cross-validation experiments, the XGBoost model was independently trained, and the importance of all radiomic features was determined based on their information gain, followed by selection of the top 50% important features. Thereafter, the intersection of the selected important features was considered from 10 random divisions of data and a stable subset was obtained as the result of feature selection.
Visualization of the prediction
An XGBoost model often contains tens or hundreds of boosting trees, which highly reduces the interpretability of the model. To understand the inherent causality of model complexity, SHapley Additive exPlanations (SHAP) was used to analyze the relationship between the features and output in the XGBoost ‘black box’. SHAP analysis evaluated the SHAP value of each feature of the training sample, which represented the sensitivity of that feature to changes in model output. By linearly decomposing the prediction result into the effect of each feature, the importance of features could be calculated and the role of different features in the model could be visualized.
Statistical analysis
Finally, to evaluate the performance of the XGBoost model, the predicted result was compared with the pathologically confirmed lesion category. The accuracy of the model classification was noted, and the receiver operating characteristic (ROC) curve of the prediction results was plotted. The ROC curve described the relationship between the sensitivity and specificity of the classification when the model had different decision cut-offs. If the model was more robust and accurate, the area under the ROC curve (AUC) would be closer to the unit. We thus performed 2 sets of experiments. First, we evaluated the results of the cross-validation experiments. The accuracy and AUC were used to measure the prediction of the XGBoost model, and the confidence intervals (CIs) of the analysis were also determined. Next, we obtained an additional data set of 87 images from another hospital, which was used as an independent test set to evaluate the model. The accuracy and AUC were also used to measure the generalizability of the model.
In addition, we reported the analysis results of the radiologists by comparing them with the ROC curve of the prediction results with the XGBoost model.
Results
Detailed patient characteristics and features extracting
Our study included 322 MC cases (101 thymic cysts, 6 pericardial cysts, 144 bronchogenic cysts, and 71 other cysts, such as mixed type), and 270 MTs (237 thymomas, including 23 type A, 125 type B, and 89 type AB; and 33 thymic carcinomas) (Table 1). A total of 415 cases (~70%) were analyzed for the training data set, and 177 cases (~30%) were accumulated for the internal training set (Figure 2).
Table 1
Variable | Value |
---|---|
Gender, n (%) | |
Male | 289 (48.8) |
Female | 303 (51.2) |
Median age [range], years | 58 [18–83] |
MC, n (%) | |
Simple cyst | 174 (54.0) |
Pericardial cyst | 4 (1.2) |
Bronchogenic cyst | 144 (44.7) |
MT, n (%) | |
Low-risk | 146 (54.1) |
High-risk | 124 (45.9) |
Median diameter [range], cm | 3.1 [0.7–22] |
Diameter <2 cm, n (%) | |
Yes | 134 (22.6) |
Symptoms, n (%) | |
Yes | 56 (9.5) |
Low-risk: type A, AB, B1 thymoma; high-risk: type B2 thymoma and thymic carcinoma (type C). MC, mediastinal cyst; MT, mediastinal tumor.
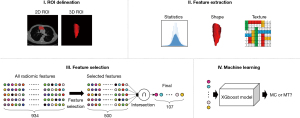
We extracted 934 features for each image via image filtering and radiomics analysis. In the fivefold cross-validation experiments, we obtained the 107 most important features by intersecting the top 500 features from all 934 radiomic features from 10 random divisions of the dataset. Twenty examples of these features are shown in Figure 3A,3B. The top 5 most important features were the 90th percentile Hounsfield unit (HU) value, the average HU value, the median HU value, the energy value (proportional to the square of the HU value), and the minimum value for area after filtering by the Laplacian of Gaussian operator.
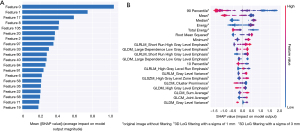
Repeatability test
To evaluate the variability of manual segmentation of mediastinal lesions, 37 patients’ CT images were randomly selected and the lesion boundaries were manually delineated for a second time. The Dice coefficient reached 0.963, which represented high repeatability (low interobserver variability) between the 2 results of the same volumetric image.
XGBoost classification model construction and validation
The XGBoost classification model was established using 107 selected radiomics features. In the fivefold cross-validation experiments, the XGBoost model achieved an accuracy of 0.960 on the training set and 0.924 on the test set. Figure 4A shows the ROC (yellow curve) of the XGBoost model, and the shaded area corresponds to the 95% CI, with an AUC of 0.972 (95% CI: 0.948–0.995). The asterisk in Figure 4A indicates that the accuracy rate of radiologists was 0.820 when reading only the CT image to determine whether the lesion was an MC or an MT. The reading of the radiologists was below the lower limit of the 95% CI for the prediction results of the model.
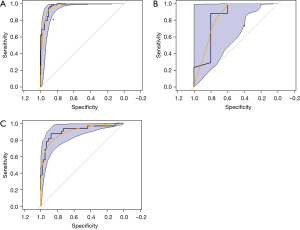
For lesions smaller than 2 cm, the accuracy of XGBoost reduced slightly. Figure 4B shows the corresponding ROC curve (yellow), with an AUC of 0.835 (95% CI: 0.543–1.000). The accuracy of radiologists was 0.667 (indicated by the asterisk). The reading of the radiologists was within the lower limit of the 95% CI for the prediction results of the model.
We tested the model on an independent external data set containing 87 CT volumetric images from another hospital, and the AUC of the ROC curve was 0.910 (Figure 4C).
Visualization by SHAP
In addition to accuracies and AUCs, we used SHAP analysis to visualize the features used in the XGBoost model. Feature distribution was based on the classification results for the first 20 features (Figure 3B). Each row represents a feature, the horizontal axis of the table represents the SHAP values, and each dot represents a data sample. A redder color indicates a larger value of the feature itself, and a bluer color indicates a smaller value. The top-ranked features show significant differences in SHAP values for different lesion categories. For example, the 90th percentile of the HU value (tumor, 73.3±21.0, 95% CI: 70.8–75.8 vs. cyst, 40.1±20.4, 95% CI: 37.9–42.4) is the most important feature, and the blue dots are mainly gathered in the right portion of the axis, while the red dots mainly appear in the left portion.
Simple clinical classification model
We constructed a simple ‘clinical’ classification model based on logistic regression using the 90% percentile HU value, the presence or absence of symptoms, and tumor size. The accuracy of the model was 0.823 (AUC =0.894), slightly higher than the accuracy of 0.820 by radiologists. To further test the robustness of the 90% percentile HU value, we removed the symptoms and the tumor size features from the model. The accuracy of the model based solely on the 90% percentile HU value reached 0.809 (AUC =0.880), which indicated that the feature showed high accuracy and excellent robustness in the classification of mediastinal lesions in our experiment.
Discussion
To date, this study involved the largest cohort in a radiomics study of mediastinal lesion differentiation strengthened by multicenter validation. A previous study reported that MCs were correctly diagnosed by CT in 83% (10/12) of cases (20), similar to our study (87%), indicating that CT accuracy has been insufficient for a considerable number of patients. The combination of radiomics with XGBoost dramatically increased the accuracy to 97.2% in our internal dataset and 91% in the external dataset validation, indicating the exceptional stability of this novel diagnostic tool. We chose XGBoost as the primary classification model for this study due to the ‘black box’ nature of machine learning, which showed better interpretability than other machine learning methods. The datasets of the 2 different hospitals showed discrepancies in feature distribution due to differences in CT scanner and radiological parameters. We used adaptive instance normalization (21) to align the features of the 2 types of data so that the original model could be applied to CT images from other institutions, indicating that it had good potential to further improve the prediction value after training with big data. We believe a better accuracy might be achieved if the model could be trained using data from more institutions. The model suggested in this study had the best performance up to date, and the most important part is the feedback of the model to human radiologists, who may understand the logic behind the radiomics textures.
A precise preoperative differential diagnosis can facilitate the decision to perform surgery and also help identify the appropriate excision preoperatively, thereby indicating the optimal surgical approach. Cysts may only require removal around the border without extensive clearance, for which video-assisted thoracic surgery (VATS) would be sufficient, while thymomas should be removed thoroughly along with thymic fat tissues to reduce tumor recurrence or myasthenia gravis, which necessitates extensive thymectomy in the first attempt. Therefore, the combination of CT with our novel prediction model could greatly improve diagnostic accuracy, optimize the operative workload, and reduce the time and cost required for unnecessary MRI or positron emission tomography (PET)-CT detection.
An extraordinarily low accuracy for the diagnosis of lesions less than 2 cm exists in clinical practice. However, this problem was resolved by the model, which made us curious about the internal mechanisms. SHAP analysis was performed to show a series of diagnostic texture features, and the 90th percentile HU value ranked as the top radiological characteristic, which explained precisely the difficulty for radiologists in accurately diagnosing lesions less than 2 cm. For example, 10 points can be selected randomly for HU measurement, and solid lesions should be considered if the 90th percentile is greater than 42.4. The average HU value, which is commonly used by physicians, is not as reliable as the 90th percentile, as indicated by machine learning. Therefore, radiologists need to read multipoint CT HU values carefully when judging small lesions. We felt that the visualizations from machine learning could provide a window into artificial intelligence, allowing clinicians to understand the internal logic underlying the high accuracy of the machine. We developed an easy ‘clinical’ model using the 90% percentile HU value, the presence or absence of symptoms, and tumor size. The model showed excellent performance and could be introduced into clinical practice.
Due to the relatively stable morphology and clear boundaries of MCs and MTs, the interobserver variability of manual segmentation was low, as was suggested in the results of repeatability. A mediastinal window was used for drawing the boundary of the tumor, and the influence of thymus tissue around the lesion could be avoided. In this study, we focused on the texture characteristics of the tumor, and so we did not include the lesion shape or its relationship with adjacent tissues into the machine learning calculation. Nevertheless, we believe that lesion shape has some value for differential diagnosis of the nature of lesions, and this should be addressed in future research. In addition, the ROIs in our study were manually delineated by radiologists. In future studies, we will use deep learning methods with computer vision to train an automated model to delineate the lesion ROI, allowing automatic delineation and recognition of thymic lesions, which will facilitate the transformation of this method into a real-world product of clinical value.
Although the proposed method yielded more accurate results, several limitations warrant further investigation. First, due to the inherent bias of the black box nature of radiomics, our findings still need to be verified prospectively by large-sample and multicenter data. However, our model was trained using collected data before 2018 and tested with cases collected subsequently, and so it could be considered a prospective observational study. Second, the proposed model did not show equal accuracy for data from another hospital (the accuracy reduced from 0.97 to 0.91 but was still higher than that obtained by radiologists). We will further explore more advanced feature alignment methods, such as deep learning-based generative models, to improve the classification accuracy from multicenter datasets. After developing automatic depiction tools, clinicians may have a feasible desktop tool for diagnostic assistance. In addition to identifying cysts and tumors, we will further identify low-risk and high-risk subtypes of thymoma, as well as anterior MCs and type B1 and B2 thymomas, so as to expand the application scenarios of the model. The number of patient samples in this study is too small, and a large sample study should be added for verification.
In conclusion, our study showed that radiomics combined with XGBoost may have significantly increased diagnostic accuracy for distinguishing MC from MT compared to the accuracy obtained by radiologists. We believe that this novel diagnostic model may optimize the surgical strategy for mediastinal lesions, especially those within 2 cm in size.
Acknowledgments
We would like to thank Oxford Science Editing for their editorial support.
Funding: This project was funded by the National Natural Science Foundation of China (81870008) and the Shanghai Municipal Health Commission Special Program for Clinical Research in Health Industry (201840175).
Footnote
Reporting Checklist: The authors have completed the STARD reporting checklist. Available at https://dx.doi.org/10.21037/atm-21-5999
Data Sharing Statement: Available at https://dx.doi.org/10.21037/atm-21-5999
Conflicts of Interest: All authors have completed the ICMJE uniform disclosure form (available at https://dx.doi.org/10.21037/atm-21-5999). The authors have no conflicts of interest to declare.
Ethical Statement: The authors are accountable for all aspects of the work in ensuring that questions related to the accuracy or integrity of any part of the work are appropriately investigated and resolved. All procedures performed in this study involving human participants were in accordance with the Declaration of Helsinki (as revised in 2013). This study protocol was approved by the institutional review board of Shanghai Pulmonary Hospital (L20-379, Shanghai, China). Patients’ written informed consent was waived by the institutional review board because of the retrospective nature of the study.
Open Access Statement: This is an Open Access article distributed in accordance with the Creative Commons Attribution-NonCommercial-NoDerivs 4.0 International License (CC BY-NC-ND 4.0), which permits the non-commercial replication and distribution of the article with the strict proviso that no changes or edits are made and the original work is properly cited (including links to both the formal publication through the relevant DOI and the license). See: https://creativecommons.org/licenses/by-nc-nd/4.0/.
References
- Weng JC, Ma JP, Hao SY, et al. Intradural Extramedullary Bronchogenic Cyst: Clinical and Radiologic Characteristics, Surgical Outcomes, and Literature Review. World Neurosurg 2018;109:e571-80. [Crossref] [PubMed]
- Wang R, Li H, Jiang J, et al. Incidence, treatment, and survival analysis in mediastinal malignant teratoma population. Transl Cancer Res 2020;9:2492-502. [Crossref]
- Strollo DC, Rosado-de-Christenson ML, Jett JR. Primary mediastinal tumors: part II. Tumors of the middle and posterior mediastinum. Chest 1997;112:1344-57. [Crossref] [PubMed]
- Davis JW, Florendo FT. Symptomatic mediastinal thymic cysts. Ann Thorac Surg 1988;46:693-4. [Crossref] [PubMed]
- Cheng L, Huang A, Jiang K, et al. Urgent Surgical Approach to Mediastinal Cyst Causing Life-Threatening Tracheal Obstruction. Ann Thorac Surg 2018;105:e125-8. [Crossref] [PubMed]
- Kozu Y, Suzuki K, Oh S, et al. Single institutional experience with primary mediastinal cysts: clinicopathological study of 108 resected cases. Ann Thorac Cardiovasc Surg 2014;20:365-9. [Crossref] [PubMed]
- Jung W, Cho S, Yum S, et al. Differentiating thymoma from thymic cyst in anterior mediastinal abnormalities smaller than 3 cm. J Thorac Dis 2020;12:1357-65. [Crossref] [PubMed]
- Liu L, Lu F, Pang P, et al. Can computed tomography-based radiomics potentially discriminate between anterior mediastinal cysts and type B1 and B2 thymomas? Biomed Eng Online 2020;19:89. [Crossref] [PubMed]
- Almeida PT, Heller D. Anterior mediastinal mass. InStatpearls. Treasure Island (FL), 2020.
- Date H. Diagnostic strategies for mediastinal tumors and cysts. Thorac Surg Clin 2009;19:29-35. vi. [Crossref] [PubMed]
- Kawaguchi M, Kato H, Hara A, et al. CT and MRI characteristics for differentiating mediastinal Müllerian cysts from bronchogenic cysts. Clin Radiol 2019;74:976.e19-976.e25. [Crossref] [PubMed]
- Cangemi V, Volpino P, Gualdi G, et al. Pericardial cysts of the mediastinum. J Cardiovasc Surg (Torino) 1999;40:909-13. [PubMed]
- McAdams HP, Kirejczyk WM, Rosado-de-Christenson ML, Matsumoto S. Bronchogenic cyst: imaging features with clinical and histopathologic correlation. Radiology 2000;217:441-6. [Crossref] [PubMed]
- Murayama S, Murakami J, Watanabe H, et al. Signal intensity characteristics of mediastinal cystic masses on T1-weighted MRI. J Comput Assist Tomogr 1995;19:188-91. [Crossref] [PubMed]
- Shin KE, Yi CA, Kim TS, et al. Diffusion-weighted MRI for distinguishing non-neoplastic cysts from solid masses in the mediastinum: problem-solving in mediastinal masses of indeterminate internal characteristics on CT. Eur Radiol 2014;24:677-84. [Crossref] [PubMed]
- Tomiyama N, Honda O, Tsubamoto M, et al. Anterior mediastinal tumors: diagnostic accuracy of CT and MRI. Eur J Radiol 2009;69:280-8. [Crossref] [PubMed]
- Ryu HS, Koh JS, Park S, et al. Classification of thymoma by fine needle aspiration biopsy according to WHO classification: a cytological algorithm for stepwise analysis in the classification of thymoma. Acta Cytol 2012;56:487-94. [Crossref] [PubMed]
- Marcus A, Narula N, Kamel MK, et al. Sensitivity and specificity of fine needle aspiration for the diagnosis of mediastinal lesions. Ann Diagn Pathol 2019;39:69-73. [Crossref] [PubMed]
- Iannarelli A, Sacconi B, Tomei F, et al. Analysis of CT features and quantitative texture analysis in patients with thymic tumors: correlation with grading and staging. Radiol Med 2018;123:345-50. [Crossref] [PubMed]
- Weber T, Roth TC, Beshay M, et al. Video-assisted thoracoscopic surgery of mediastinal bronchogenic cysts in adults: a single-center experience. Ann Thorac Surg 2004;78:987-91. [Crossref] [PubMed]
- Huang X, Belongie S. Arbitrary style transfer in real-time with adaptive instance normalization. In: Proceedings of the IEEE International Conference on Computer Vision, 2017:1501-10.
(English Language Editor: A. Muijlwijk)